The Rise of AI-Driven Strategic Portfolio Management Balancing Risk and Reward in 2024
The Rise of AI-Driven Strategic Portfolio Management Balancing Risk and Reward in 2024 - AI Algorithms Reshape Risk Assessment Models
AI algorithms are fundamentally altering how financial institutions assess risk, moving away from the traditional methods that have long been the standard. By processing vast quantities of unstructured data, AI can pinpoint potential hazards and evaluate risks with greater accuracy than ever before. This capability translates to more efficient operations and reduced costs, as many routine tasks become automated. Furthermore, the incorporation of predictive analytics fosters a more dynamic and adaptable risk management system.
While this evolution is promising, concerns remain about establishing confidence and ensuring ethical considerations remain paramount as AI technologies rapidly evolve. The financial sector must carefully manage the balance between the potential benefits of AI and the inherent risks it presents to ensure its responsible integration. It's a crucial task to navigate these new waters, ensuring that the drive for innovation doesn't compromise established ethical standards within the field.
The surge in AI's capabilities, especially with advancements like ChatGPT, is fundamentally reshaping how we think about risk assessment within portfolio management. AI algorithms can sift through massive quantities of historical data and contemporary market signals in real-time, providing a much finer-grained predictive capacity than traditional approaches that tend to rely on more straightforward, linear relationships. This leads to a more comprehensive view of potential risks.
Machine learning is particularly impactful in this area. It allows us to uncover non-linear relationships between diverse factors, bringing to light hidden risks that might elude traditional models. Furthermore, by blending in insights from behavioral finance, AI algorithms are capable of anticipating market distortions that stem from human emotions, which leads to more nuanced risk evaluations.
We're also seeing a shift towards more dynamic risk management. AI algorithms can dynamically adjust risk parameters in response to changing market conditions, enabling a more agile and responsive approach. This is a marked difference from traditional strategies that predominantly depend on historical averages.
Moreover, AI offers new methods for stress-testing portfolios. The ability to simulate different economic scenarios allows for a more detailed understanding of how a portfolio might respond under duress, exposing potential weaknesses in risk exposure. Beyond conventional data, AI can now incorporate alternative data streams, like social media sentiment or satellite imagery, to enrich risk assessment models and improve the timeliness and practical relevance of the insights generated.
This ability to process diverse data sources also reveals systemic risks that can span multiple interconnected financial instruments. This is something that traditional models frequently miss because they often operate in isolated compartments. Evidence suggests that portfolios managed with AI-enhanced risk assessment generally exhibit reduced volatility during market fluctuations in contrast to those using established techniques.
This enhanced speed and capacity of AI algorithms to crunch data and generate risk assessments can significantly accelerate decision-making. This could be a game changer, especially in markets where speed is paramount. Beyond immediacy, the capacity of AI to learn and adapt means that risk assessment models are constantly evolving. This makes them more robust and less susceptible to unforeseen disruptions, a significant improvement over static models that require human intervention to remain accurate.
The Rise of AI-Driven Strategic Portfolio Management Balancing Risk and Reward in 2024 - Machine Learning Enhances Asset Allocation Strategies
Within the expanding field of AI-driven portfolio management, machine learning is taking on a significant role in refining asset allocation strategies. By analyzing enormous datasets, machine learning algorithms uncover intricate patterns and relationships that standard methods often overlook, thereby leading to more effective asset allocation decisions. This capability helps in maximizing returns while also enhancing risk management through real-time insights into market movements and price trends.
The power of machine learning extends to forecasting asset performance, automatically adjusting portfolios, and even automating trading decisions. While this technology holds immense promise for shaping the future of investing, there are critical issues that need attention. The ethical implications of relying on algorithms for such crucial tasks, as well as the need for transparency and control over automated decision-making, are significant considerations that require ongoing examination. The continued advancement of machine learning techniques is poised to broaden their impact within investment strategies, but the complexities of responsible integration must be thoughtfully navigated.
Machine learning offers a more nuanced approach to asset allocation by uncovering intricate, non-linear relationships within asset returns—something traditional statistical methods often miss. This allows for potentially more precise predictions of asset price movements, which can translate into more effective allocation strategies.
Beyond risk identification, the integration of machine learning opens up a new world of investment opportunities. It enables us to analyze a wider range of data, such as consumer behavior trends or global political events, to potentially spot otherwise hidden investment prospects.
Research suggests that portfolios built using machine learning techniques can achieve higher returns compared to those based on traditional methods—potentially 2-5% annually in some cases. This indicates that machine learning algorithms might be more adept at anticipating future market movements.
The capacity of machine learning algorithms to process data in real-time enables near-instant adjustments to asset allocation in response to shifts in market conditions. This responsiveness is crucial in today's rapidly evolving markets, where even slight delays can result in substantial losses.
Machine learning algorithms excel at multi-factor analysis, allowing for a more comprehensive assessment of various performance drivers and their interplay. This improves the precision of asset selection by ensuring a balanced approach that also mitigates exposure to correlated risks.
By analyzing correlations between asset classes more deeply, machine learning helps us refine diversification strategies. It's not just about having a mix of different assets; it's about creating a portfolio optimally balanced against interconnected systemic risks.
Studies indicate that using machine learning in asset allocation can not only mitigate portfolio declines during market downturns but also lead to a higher Sharpe Ratio. This means better risk-adjusted returns, which is a critical objective for any investor.
Reinforcement learning, a subfield of machine learning, can be applied to dynamic portfolio management. This allows investment strategies to evolve over time through a process of trial and error, continuously refining and optimizing returns through experience.
Machine learning models can identify how macroeconomic factors influence specific asset classes. This can improve predictions about how assets might behave in different economic environments, fostering better risk management during periods of uncertainty.
While some machine learning models are sometimes considered 'black boxes', we can use explainable AI techniques to gain insights into their decision-making processes. This helps increase transparency and fosters greater trust in automated asset allocation strategies.
The Rise of AI-Driven Strategic Portfolio Management Balancing Risk and Reward in 2024 - Natural Language Processing Improves Market Sentiment Analysis
Natural Language Processing (NLP) is significantly improving how we analyze market sentiment. By being able to extract insights from the sheer volume of public opinion and feedback found online, businesses and financial institutions can gain a much deeper understanding of the prevailing market mood. This is valuable across many industries, but particularly in finance where understanding public sentiment can inform strategic decisions. Recent advancements in NLP have made it easier to categorize and interpret complex sentiment data, which helps to pinpoint subtle shifts in market behavior. This is important for investment strategies that attempt to capitalize on news-driven changes in sentiment, as NLP tools can now better link the expression of opinion with market movements. While powerful, we must acknowledge that using advanced AI in this way presents challenges related to transparency and potential algorithmic biases. As such, it's vital that these technologies are used responsibly and with a keen eye towards ethical considerations.
### Natural Language Processing Improves Market Sentiment Analysis
Natural Language Processing (NLP) has become increasingly important in understanding market sentiment. It allows us to analyze text data from sources like news and social media to gain a more nuanced understanding of investor and consumer opinion, which can be invaluable for decision-making in investment and marketing strategies. NLP's ability to break down text into individual components, such as words and phrases (tokenization), and identify key entities like companies or people (named entity recognition) provides a level of detail that simpler keyword-based approaches often miss. This kind of granular analysis allows us to get a richer understanding of the subtleties of expressed emotions.
Sentiment analysis, a core part of NLP, is transforming how different sectors—from finance and social media to healthcare and even disaster response—approach data analysis. Modern NLP advancements allow for a more sophisticated categorization of sentiments, moving beyond simple positive/negative labels to grasp complex situations more accurately. This is critical as we try to understand the massive volumes of data generated daily.
Deep learning, a type of machine learning, has the potential to leverage these sentiment insights in trading strategies. It's adept at sifting through vast datasets and discerning complex patterns hidden in financial data, potentially uncovering connections between news sentiment and market shifts. Algorithms designed for analyzing the sentiment of financial news are increasingly being used to assess these relationships.
The use of AI, particularly machine learning and NLP, is becoming more common in marketing, helping to develop market intelligence and improve efficiency. It's clear from reports that AI, including NLP, is improving marketing productivity and overall effectiveness. In fact, analyses show that AI techniques have numerous applications across various industries, with marketing standing out as a field that can greatly benefit from these innovative tools.
Standard NLP methods used in marketing include techniques like Latent Dirichlet Allocation (LDA) for topic modeling and lexicon-based approaches for sentiment analysis. But the future of NLP in marketing is likely to lean towards more sophisticated approaches, like embedding-based methods and advanced language models. These tools have the potential to enhance accuracy and insight considerably.
However, these developments haven't been without their own challenges. While NLP is increasingly good at sorting through tons of information and even detecting nuances like fear or joy within text, there are still challenges. Sarcasm and more complex language can be hard for current models to interpret, which can lead to inaccuracies in the resulting sentiment assessments. It's important to acknowledge these limitations as we continue to push NLP forward.
Despite these issues, NLP is a critical part of advanced market analysis. It enables rapid, large-scale sentiment analysis, opening up avenues for uncovering previously hidden relationships between sentiment and market dynamics. It remains to be seen how effectively these insights can be integrated into actual trading strategies, but the ongoing improvements to NLP models are undeniably significant. As NLP technology matures and computational power increases, we can expect to see even more sophisticated and accurate market sentiment analysis, possibly leading to new frontiers in both investment and trading practices.
The Rise of AI-Driven Strategic Portfolio Management Balancing Risk and Reward in 2024 - Automated Trading Systems Gain Sophistication
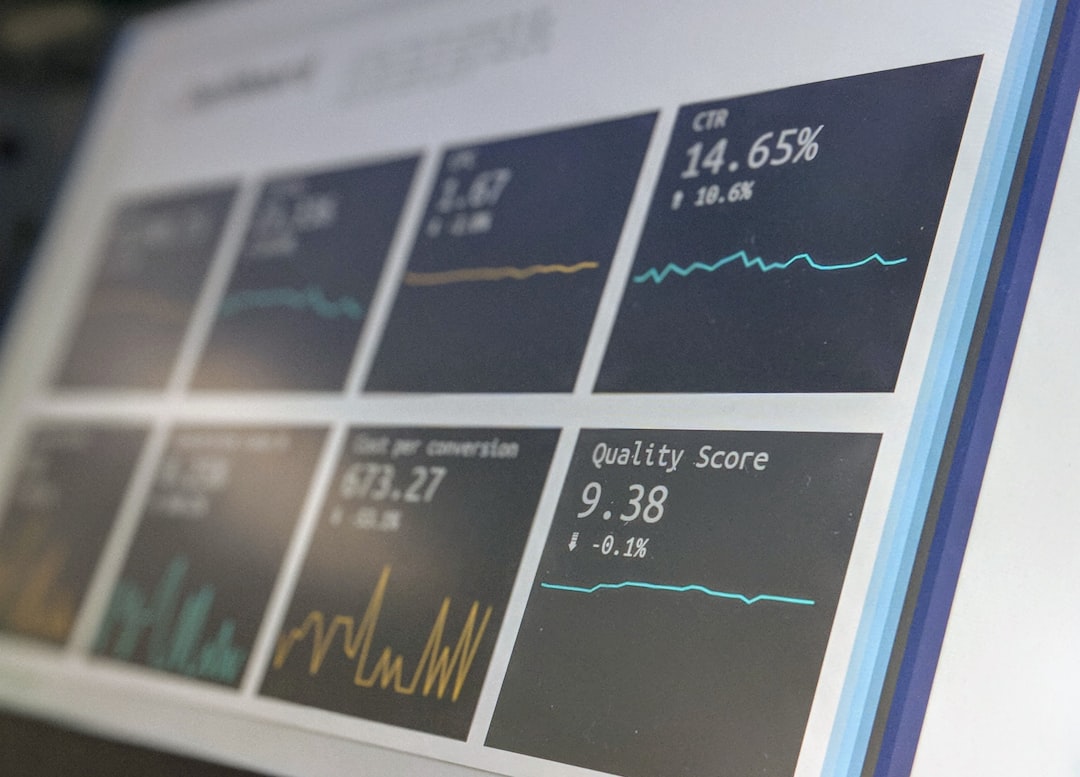
Automated trading systems are becoming increasingly sophisticated, employing advanced AI to refine trading decisions and manage risk within financial markets. These systems can now process data in real-time, leading to more flexible trading strategies that adapt quickly to changing market situations. The availability of sophisticated analytics, including risk-to-gain ratios and win rates, offers traders a more precise understanding of their trading performance. However, with this increasing sophistication come crucial questions about regulations and the ethical considerations of automated trading. As we progress into 2024, effectively balancing innovation with responsible management within the financial sector will be a major challenge.
The realm of automated trading systems is undergoing a significant transformation, moving beyond basic, pre-programmed rules to embrace more complex machine learning models. These systems are becoming increasingly adept at adapting to market conditions in real-time without human intervention, a shift driven by the continuous advancement of AI. We're seeing a notable trend towards algorithms that operate at extremely high speeds, often measured in fractions of a millisecond. This level of speed allows them to exploit minuscule price discrepancies before others, leading to a fundamentally different market environment characterized by faster trading cycles and higher volatility.
A fascinating development is the incorporation of crowdsourced data, such as social media commentary or opinions from online investment forums, into the decision-making process of automated trading systems. While this added data layer enhances predictive abilities, it also raises legitimate concerns about data reliability and the potential biases embedded in public sentiment. The use of reinforcement learning techniques further amplifies the ability of these systems to adapt. Essentially, these trading systems learn from their successes and failures, constantly evolving their strategies in a way that mimics the behavior of biological organisms adapting to an environment.
We're also witnessing the development of more intricate multi-stage trading strategies. These sophisticated algorithms are capable of simultaneously buying and selling various assets in different markets, aiming to optimize trade execution and reduce market impact. The integration of behavioral economic principles adds another layer of depth to these systems. By understanding how human emotions and biases impact trading decisions, algorithms can better anticipate and potentially exploit predictable market anomalies that result from irrational behavior, particularly in volatile market conditions.
Furthermore, algorithms are becoming more skilled at identifying correlations across different markets, leading to increasingly comprehensive and sophisticated cross-asset strategies. The ability to connect equity, currency, and commodity markets opens up a wider range of opportunities and risk mitigation strategies. However, this complexity also poses significant challenges for financial regulators. It can be difficult to trace the activities of complex algorithms, making it challenging to ensure fair market practices.
In a democratizing trend, several retail trading platforms are now implementing automated trading models that mimic strategies traditionally employed by sophisticated hedge funds. This allows everyday investors to potentially compete more effectively with established institutional players. Another future possibility lies in the realm of quantum computing. While still in its early stages, its potential to dramatically change automated trading is already evident. Quantum algorithms could drastically improve the speed and complexity of trading strategies by addressing computations that are currently impossible. This could lead to a fundamental shift in the field of automated trading if quantum technologies continue to develop.
This evolution of automated trading systems presents a fascinating landscape of both opportunity and challenge. While AI offers the possibility of greater efficiency, optimization, and potentially more equitable access to sophisticated trading tools, it's crucial to carefully consider the implications of such complex and autonomous systems. The interplay between innovation, market dynamics, and regulatory oversight will likely be a defining characteristic of the financial world for years to come.
The Rise of AI-Driven Strategic Portfolio Management Balancing Risk and Reward in 2024 - Ethical Considerations in AI-Driven Investment Decisions
The increasing integration of AI in investment decisions necessitates a heightened focus on ethical considerations. Ensuring the data used to train AI models is accurate and unbiased is crucial, as is validating the models themselves to prevent flawed or misleading outcomes. Transparency in how AI algorithms reach their conclusions is paramount, enabling stakeholders to understand and trust the investment process. Efforts to minimize biases within AI systems are critical for fair and equitable investment practices, and ethical frameworks are needed to guide decisions. These frameworks should prioritize principles like accountability, fairness, and maintaining the confidentiality of sensitive investor information. The push for ethical AI isn't just about following regulations; it's about cultivating trust in the financial ecosystem and ensuring that advancements in AI benefit investors without sacrificing core ethical principles. Successfully managing the challenges and complexities of AI in finance will play a key role in building a sustainable and trustworthy future for the industry as it navigates rapid technological change.
The increasing use of AI in investment decisions raises a number of ethical questions we need to consider. One major concern is the lack of transparency in how AI algorithms arrive at their conclusions. Investors and regulators are rightly demanding more clarity about how these systems operate, as opaque systems can easily lead to unfair trading practices or biased outcomes. We simply don't want to see AI-powered investment decisions made without understanding why.
Furthermore, AI models are often trained on historical data, and this can be problematic. If the training data reflects existing biases within the financial system or broader society, the AI may unintentionally amplify those biases in its investment decisions, potentially leading to unfair treatment of specific asset classes or investors. This suggests that the quality and nature of the data used to train AI systems must be carefully vetted and understood.
While we design AI to limit human error, it's important to remember that AI struggles to fully grasp the complexity of human emotions, especially in financial markets. This inability to fully capture and account for emotional decision-making could lead to flawed investment choices, particularly during times of economic uncertainty. It is a reminder that the human element remains important even in an era of advanced AI.
The rise of automated trading has fundamentally altered market dynamics. These systems, in their pursuit of maximizing profit, can cause instability in financial markets, particularly during times of stress. Their lightning-fast reactions can create cascading sell-offs or create unpredictable price distortions. This rapid response capability introduces a new kind of risk into the system and might necessitate adjustments to how markets are regulated.
AI excels at optimizing portfolio performance, but it's not without potential drawbacks. When a system over-optimizes, it can inadvertently lead to a situation where investors react in very similar ways to certain market events, kind of like a herd mentality. This is risky as it can potentially create conditions for sudden, sharp market downturns, highlighting the need for diversification in both investment strategies and the AI models themselves.
As AI investment strategies become more commonplace, it's becoming challenging for regulators to keep up with the rapidly evolving technology. Striking a balance between allowing innovation to flourish and ensuring proper oversight to protect investors is a complex issue that needs continuous attention. We have to ensure AI enhances, not undermines, the integrity of financial systems.
The sophistication of AI algorithms can also be exploited for less ethical purposes. For instance, certain traders might find ways to exploit flaws in automated systems for personal gain, potentially creating unfair advantages. This raises questions about the responsibility of AI developers to create robust systems that are less susceptible to this type of manipulation.
The increasing use of AI in finance requires gathering large amounts of data, including personal information. This raises important privacy concerns. How we ensure that this data is handled ethically and protected is a vital part of implementing these new technologies. Building trust requires demonstrating responsible data practices.
We also need to address the issue of misinformation and its potential impact on investor sentiment. When AI systems rely on social media or news feeds, there's a risk of reacting to incorrect information. This potential for misinformation to lead to impulsive trading underscores the need for robust mechanisms to verify the quality of data used for making investment decisions.
Finally, as AI takes on more decision-making responsibilities, questions of accountability will only become more pressing. If an AI-driven investment fails, who is responsible? This highlights the need for careful re-evaluation of ethical frameworks in the finance industry, particularly as we shift from a model where humans are primarily responsible for decisions to a model where AI plays a more significant role.
All in all, it's clear that AI-driven portfolio management is a powerful tool with the potential to transform finance for the better. However, as we advance, we need to ensure that it is developed and implemented responsibly, with a careful eye toward the ethical considerations it presents. This will require ongoing collaboration among researchers, developers, investors, regulators, and the wider community to ensure a fair and sustainable financial system for the future.
The Rise of AI-Driven Strategic Portfolio Management Balancing Risk and Reward in 2024 - Data Privacy Challenges in AI Portfolio Management
The increasing reliance on AI in portfolio management, particularly in 2024, brings to light significant data privacy concerns. While AI enhances risk assessment and decision-making, the use of personal data in these sophisticated algorithms raises questions about how this data is handled and protected. Meeting the requirements of evolving data privacy regulations, especially when dealing with sensitive data and the potential for AI-driven herding behavior that can destabilize markets, poses a major hurdle for portfolio managers. There's a growing worry that existing legal frameworks may not be equipped to fully address the privacy implications of rapidly advancing AI technologies. As investment strategies become more reliant on AI-driven insights, it's crucial that ethical considerations and robust governance structures are central to managing the complexities of data privacy in this new landscape. Only through a careful balancing act can we responsibly navigate the exciting but potentially perilous waters of AI in finance.
The widespread use of AI in managing investment portfolios is leading to new complexities surrounding data privacy. One emerging issue is the challenge of managing data across international borders, as differing privacy laws create potential legal snags. For instance, if a financial firm stores data in a place with weaker privacy protections, it might be in violation of stricter regulations elsewhere. This becomes more significant as financial markets become increasingly interconnected.
Another concern is the possibility that AI models trained on historical data might unintentionally inherit existing biases in financial systems or wider society. This can result in unfair or discriminatory outcomes for certain types of investments or investors, raising ethical flags. We need to be more mindful of how these AI systems are built to minimize such risks.
With the increased reliance on AI comes a greater risk of data breaches. Since these systems often handle highly sensitive client information, a successful breach could cause major financial harm and severely damage trust in the institution. This suggests that security protocols for AI-based systems need careful consideration and improvement.
As AI models increasingly share data to enhance their predictive capabilities, there's a potential for unintended consequences. For example, firms inadvertently sharing sensitive information could inadvertently influence market dynamics in a way that benefits some players unfairly or facilitates collusion. This prompts concerns about competition and how we manage the use of shared information.
A lot of AI systems in finance operate like "black boxes." This means that we don't always understand the reasoning behind the decisions these systems make. This opacity makes it difficult for investors and regulators to verify the fairness of trading decisions and makes it harder to ensure compliance with the rules. This lack of transparency can be a barrier to wider adoption of AI in financial services.
Algorithmic trading, powered by AI, can bring increased speed and automation to market interactions. However, this can also lead to market manipulations and volatile trading activity. This raises questions about the ethics of automated systems that operate before humans have a chance to intervene, potentially requiring new safeguards.
Because many AI models depend on personal information, it can be challenging to ensure individuals fully understand how their data is being used. Without clear communication and informed consent, financial institutions risk violating privacy standards. This emphasizes the need for more transparent data practices.
Currently, the development and implementation of AI is outpacing the creation of relevant regulations. This gap could lead to a situation where data practices are not properly controlled, creating a risk to data privacy and the security of investor information. This suggests that a more agile approach to regulation may be needed.
AI can be fooled by misinformation, such as false social media trends or fabricated financial news. This vulnerability can lead to impulsive trades based on false information, potentially creating significant instability in the market. Developing mechanisms to filter and validate the information used to make investment decisions is critical.
Finally, there's a growing concern about the potential for AI to re-identify individuals even when data sets are anonymized. This reinforces the need for institutions to employ stronger safeguards to protect the sensitive information they collect and process. The need for careful management of privacy, even with anonymization, is critical as AI models become more sophisticated.
More Posts from :