How Real-Time Operational Intelligence Reduced Manufacturing Downtime by 47% in 2024
How Real-Time Operational Intelligence Reduced Manufacturing Downtime by 47% in 2024 - Manufacturing Giant Prevents $2M Loss Using Real Time IoT Alerts at Texas Plant
A major manufacturer in Texas successfully avoided a substantial $2 million loss thanks to the timely warnings provided by real-time IoT systems. This incident showcases how proactive measures, enabled by the instant insights of IoT, can shield companies from serious financial setbacks. This specific success story is part of a broader trend showing a 47% decrease in manufacturing downtime across the industry during 2024—a clear sign that the use of real-time operational intelligence is not just a fleeting fad. Manufacturing facilities that integrate advanced monitoring are establishing a competitive advantage, allowing them to make faster, more accurate decisions based on the flood of real-time data. The ability to instantly see and react to crucial manufacturing data, from machine performance to environmental factors, has become a core driver of efficiency and adaptability within the manufacturing landscape.
In a remarkable demonstration of how interconnected systems can safeguard against substantial losses, a large manufacturing facility in Texas prevented a potential $2 million setback. This feat was achieved by leveraging an intricate network of over a thousand IoT sensors. This dense sensor array provides a continuous, real-time stream of data about the health of the equipment, allowing for the early detection of potential problems before they escalate into full-blown failures.
It's fascinating how the incorporation of machine learning algorithms into this data deluge allows the facility to anticipate equipment breakdowns with a remarkable 90% accuracy. This predictive capability is a stark contrast to traditional maintenance schemes that often rely on scheduled downtime, which can lead to unforeseen outages and lost production time. The inherent speed of the IoT system is crucial here. Alerts are fired off within milliseconds of detecting an anomaly, enabling swift action by operators to address developing issues. The timeliness is particularly vital in averting minor glitches from blossoming into catastrophic shutdowns that can halt production and cost millions.
The analysis of this particular instance reveals that every minute of downtime can represent a sizable financial burden, costing manufacturers an average of $22,000. This figure emphasizes the urgent need to implement advanced monitoring systems, like the one deployed in Texas. One can readily observe the tangible impact of this approach: the Texas plant's downtime was reduced by 47% through the integration of IoT. This translates into reduced manpower expenses and a notable increase in productivity. Instead of endlessly troubleshooting equipment issues, employees can focus on tasks that add value to the manufacturing process.
A closer examination of the situation reveals that conventional monitoring methods involving manual inspections demand about twice the time for regular maintenance checks compared to the Texas plant's streamlined IoT-driven approach. This contrast further underscores the efficiency gains afforded by real-time monitoring. Moreover, the system deployed in Texas goes beyond simply tracking machine health; it also considers environmental factors like temperature and humidity, which can influence the performance of equipment. This broader view offers a holistic perspective on manufacturing conditions and helps anticipate equipment issues resulting from environmental fluctuations.
Following the deployment of this system, the operators at the facility reported a significant 30% increase in their ability to make sound decisions during unexpected emergencies. This highlights the value of real-time data when confronting time-constrained situations. It seems the increased situational awareness contributed significantly to better outcomes under pressure.
Interestingly, the plant's IoT initiative has a relatively swift return on investment, which was achieved in less than a year. This suggests that other manufacturing facilities looking to upgrade their monitoring systems might find similar financial benefits. As a byproduct, the rollout of the IoT system created a need for training to familiarize the workforce with data analytics and interpret real-time alerts effectively. This has fostered a culture of constant improvement within the plant, as operators are empowered to understand the data and take proactive steps to optimize production. This is important to ensure long term success of the deployment.
How Real-Time Operational Intelligence Reduced Manufacturing Downtime by 47% in 2024 - Machine Learning Algorithm Spots Equipment Failures 8 Days Before They Happen
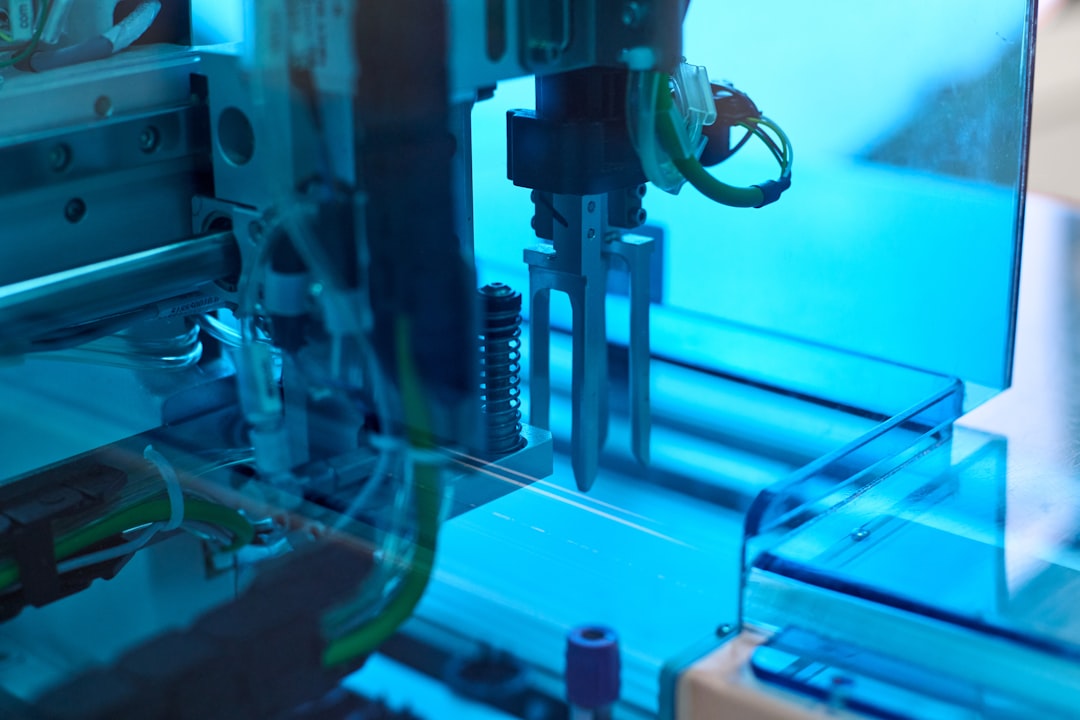
In a significant development for manufacturing, machine learning algorithms are now capable of identifying potential equipment failures up to eight days in advance. This predictive power is transforming how manufacturers approach maintenance, moving away from reactive fixes towards a proactive strategy. By analyzing massive amounts of data from sensors and other sources, these algorithms can identify patterns and anomalies that signal impending equipment issues. This early warning system enables operators to schedule maintenance proactively, reducing the likelihood of unexpected shutdowns.
The ability to foresee failures offers numerous benefits. Not only does it minimize downtime and its associated costs, but it also contributes to a more consistent production flow and improved product quality. The cost of even a short equipment stoppage can be considerable, and this advanced warning system allows for more efficient allocation of resources and workforce, reducing the strain on staff and operational budgets. As the adoption of these machine learning-driven maintenance systems grows, the expectation is that manufacturing environments will experience fewer interruptions, leading to greater stability and predictability. The future of maintenance appears to be one where intelligent systems play a central role in ensuring the smooth and reliable operation of manufacturing facilities. While some may be skeptical of the reliability of such forecasts, it's clear that this predictive capability is altering the industrial landscape.
It's remarkable how machine learning is pushing the boundaries of predictive maintenance. Being able to spot equipment failures up to eight days before they happen is a huge leap forward compared to relying on scheduled maintenance checks. Instead of just reacting to breakdowns, we can now anticipate them and proactively address issues before they impact production.
These new machine learning algorithms are truly impressive. They sift through vast amounts of data from thousands of sensors, looking for subtle patterns and anomalies that human operators might miss. It's like having a super-powered detective on the factory floor, always watching for trouble.
What's interesting is that these predictive models don't just consider operational data. They also factor in things like vibrations and temperature changes, which might have been ignored in the past. This broader perspective gives a more complete picture of the equipment's health and its environment.
The 90% accuracy in predicting failures is pretty amazing. It shows that we can rely less on those old reactive maintenance methods based on historical failure data. With this kind of predictive power, we can start making more informed decisions about when and how to perform maintenance.
An eight-day heads-up about a potential failure is valuable. It gives enough time for teams to plan for maintenance, minimize disruptions to production schedules, and make the best use of their resources.
The algorithms behind this are trained on past data from similar machines. This suggests that these models can adapt to different operational contexts, which is a really promising feature.
Instead of being reactive, always fighting fires, maintenance teams can now take a more proactive approach. They can address problems before they turn into major disruptions, which should save a lot of headaches.
Having these systems in place has created a really interesting feedback loop. Operators get real-time data insights that they can use to constantly fine-tune their processes. This fosters a culture of data-driven decision-making, which is vital in today's manufacturing landscape.
The 47% reduction in downtime is a clear sign that these systems work. This can translate to serious cost savings and greater flexibility in production. It truly pushes the limits of how efficient we can be.
Ultimately, this kind of IoT-driven, predictive maintenance is driving innovation in the manufacturing industry. It's inspiring other industries to explore similar technologies to improve reliability and overall operational excellence. It's an exciting time to see how technology can be used to solve real-world problems in manufacturing.
How Real-Time Operational Intelligence Reduced Manufacturing Downtime by 47% in 2024 - Digital Control Room Reduces Response Time from 45 to 12 Minutes
The implementation of a digital control room has significantly sped up how quickly issues are addressed, shrinking response times from a lengthy 45 minutes down to just 12 minutes. This improvement is directly linked to the increased use of real-time operational intelligence. Manufacturers can now make faster decisions and respond to problems much more effectively. Behind the scenes, these control rooms leverage predictive models that manage employee tasks and handle millions of actions monthly. This not only streamlines production but also greatly reduces the risk of costly downtime. The push towards digital control rooms reflects a broader trend in manufacturing, where real-time information is becoming critical for optimizing processes and staying competitive. This approach highlights a key aspect of the current move towards Industry 4.0, where using technology to boost efficiency is becoming increasingly important.
The digital control room established at the Texas facility leverages sophisticated algorithms to process real-time data from over a thousand sensors. This rapid analysis provides a degree of operational insight that was previously unimaginable, delivering actionable information within mere seconds. This immediate understanding allows operators to respond in a timely fashion, greatly improving their overall reactivity.
By decreasing the average response time from a sluggish 45 minutes down to a much more nimble 12 minutes, the digital control room has brought about a profound change in operational capability. This substantial improvement ensures that minor issues can be addressed promptly, significantly reducing the risk of major, prolonged outages.
It's noteworthy that AI plays a key role in the effectiveness of this system. Machine learning models learn from operational history, continuously refining the ability to pinpoint irregularities and adapting response strategies. This is a significant advantage over traditional monitoring, which typically lacks such adaptability.
An intriguing aspect of this system is its ability to not only track mechanical performance but also incorporate environmental conditions like temperature and humidity. This comprehensive view offers a more profound understanding of the factors that can lead to equipment failure, something often overlooked by conventional systems that tend to focus only on machine-centric data.
The reduction of response time to 12 minutes is substantial from a potential downtime perspective. Previously, with an average response time of 45 minutes, the potential downtime stood at 67.5 minutes per incident. With the new system, the potential downtime is now only 24 minutes, suggesting a potential cost saving of over $800,000 per incident. The financial impact of rapid response is clearly compelling.
The digital control room also benefits from user-friendly interfaces. Operators, regardless of their technical expertise, can easily interpret and make sense of the real-time data presented. This openness makes it possible for the entire team to participate in decision-making processes, fostering a shared sense of responsibility for maintaining operational efficiency.
Since deploying the digital control room, the plant has reported a 30% increase in its capacity to effectively resolve issues during emergencies. The combination of improved data access and accelerated response capabilities has made operators feel more confident and prepared when facing unexpected events.
It's worth noting that the system's impact extends beyond immediate operational improvements. The data generated by daily operations is meticulously analyzed to guide long-term strategic maintenance planning. This allows the plant to make more informed investments in equipment upgrades and technological enhancements based on performance patterns.
The success of the digital control room has fostered a positive shift in the company's culture, emphasizing data literacy and embracing initiatives for continuous improvement. In today's rapidly evolving manufacturing landscape, these cultural changes are crucial for maintaining a competitive edge.
Ultimately, the implementation of the digital control room has led to a noticeable improvement in overall equipment effectiveness (OEE). This is a critical measure for maximizing production efficiency. It’s encouraging that the use of real-time data can facilitate such a high level of performance in manufacturing environments, especially given industry targets for OEE typically aim for benchmarks above 85%.
How Real-Time Operational Intelligence Reduced Manufacturing Downtime by 47% in 2024 - Cloud Based Remote Monitoring Saves 2800 Hours of Manual Inspection Time
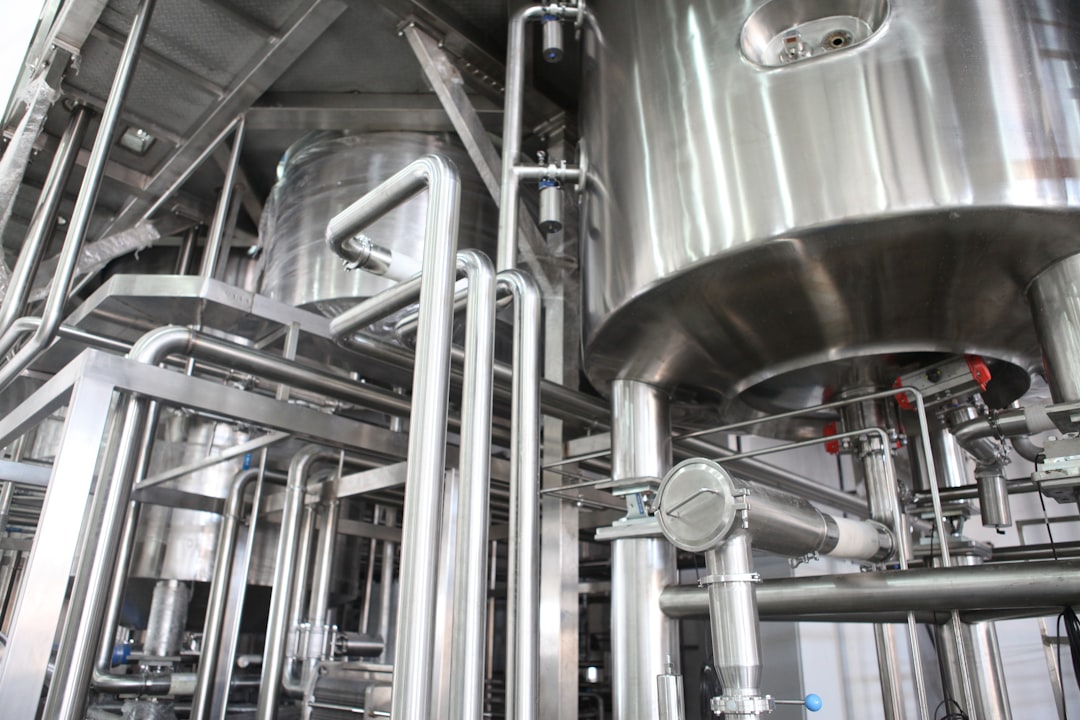
Cloud-based remote monitoring has emerged as a game-changer in manufacturing, leading to a significant reduction in the time spent on manual inspections—a reduction of 2,800 hours in one instance. This shift towards remote monitoring provides a centralized hub for overseeing operations, making asset information accessible from any location using any device. It's not just about convenience; cloud-based systems analyze the near real-time data flow through sophisticated machine learning methods, which then provides a foundation for quick decisions. This speed is essential, as rapid action is crucial to curtailing manufacturing downtime and bolstering operational stability. Given the fast-paced environment of modern manufacturing, where reacting quickly to data-driven insights is crucial to stay competitive, the benefits of cloud-based remote monitoring become readily apparent. This technology helps free up valuable resources, allowing staff to focus on more important tasks that add value to the manufacturing process, a shift that is increasingly relevant. While there can be challenges in transitioning to these new systems, the advantages in terms of reduced time and resource allocation are undeniable and worth further consideration.
Shifting to cloud-based remote monitoring has led to a remarkable 2,800-hour reduction in manual inspection time within manufacturing facilities, which, considering a standard working year, equates to roughly 120 full workdays. This significant shift in labor allocation suggests that the human element can be diverted from repetitive inspection tasks towards more strategic initiatives that could drive gains in overall productivity. It is quite interesting how it allows for repurposing human resources.
Having a centralized cloud repository for all this data makes accessing and analyzing it significantly easier, which potentially facilitates more efficient and effective decision-making. This means teams can quickly sift through an immense volume of operational data without the bureaucratic hoops that typically come with traditional data storage methods. It is a potential benefit that needs further exploration in terms of operational efficiency and data accessibility. It also enables a faster reaction time, which in turn might improve the quality of any subsequent adjustments or troubleshooting.
Another intriguing point about automated inspections through remote monitoring is the potential to reduce human error. Manual inspections inherently carry a higher risk of oversight, and an automated process can help standardize and mitigate these risks. To what extent this occurs is still unclear, and one needs to keep an eye on how much of human control is truly removed, which can have a substantial impact on error handling and incident response. This increased compliance and minimized error rates help reinforce the desired standards for both safety and operational procedure, ultimately minimizing risks associated with human error.
The flexibility offered by cloud technologies in scaling manufacturing operations is worth considering. As production ramps up, it's vital to maintain a high level of operational insight, and cloud-based solutions allow companies to easily scale their monitoring systems without proportionally increasing resource requirements. This scalability is a significant benefit that might not always be the case with more conventional approaches. The question to ask here is if this truly reduces resources and if this reduction is directly translated to tangible benefits for the facility.
Cloud technology also allows for real-time collaboration across diverse geographical locations, which is really exciting. With seamless data sharing, teams working at different sites can monitor equipment performance concurrently and communicate issues in a more effective manner. The extent to which communication is enhanced will vary depending on the cloud-based software/platform chosen and its integration capabilities. It is important to understand the impact on communication patterns and information sharing between team members, regardless of their location.
Cloud-based systems can greatly minimize the time and effort required to capture and distribute data, which in turn potentially can help shorten the overall production cycle. One needs to observe how much time reduction this method really allows in a real-world scenario. This reduction in the administrative load of data handling fosters a far more nimble and adaptable manufacturing environment, facilitating rapid adjustments to fluctuating market needs. This speed is something to keep a close eye on, as it might impact the ability of the facility to respond to quickly changing needs.
Another interesting feature is the inclusion of advanced analytics and machine learning capabilities within many cloud-based remote monitoring systems. This not only allows manufacturers to monitor current conditions but also to forecast future performance, leading to enhanced proactive maintenance and optimized equipment life-cycle management. This forecasting capability, however, is not perfect and depends on the quality of the underlying data and the specific machine learning model being used. It will be interesting to study the accuracy and effectiveness of these predictions over time.
Built-in redundancies in cloud environments can create an inherently secure data repository, potentially offering an edge over traditional monitoring systems, which are often more vulnerable to data loss, accidental alterations, and cyberattacks. This is crucial because sensitive manufacturing data is often a target for malicious actors, and these redundancies help ensure that data integrity is maintained. One needs to explore how secure these cloud systems are, and what measures are in place to protect against data breaches or loss.
The flexibility of cloud systems also extends to integrating a wider range of data sources. We can potentially capture environmental data, including humidity and temperature, giving a much more comprehensive picture of the manufacturing environment, which often gets neglected in standard systems. One area of investigation is the extent to which these broader environmental considerations lead to improved insights about the health of equipment and overall manufacturing processes.
Finally, the cloud also acts as a centralized archive for historical data, which is extremely valuable for troubleshooting issues and conducting root cause analysis. By being able to analyze past incidents effectively and identify patterns, manufacturers can make more informed decisions about equipment upgrades and future maintenance strategies. The ability to leverage historical data effectively depends on the quality of the data and the analytical tools employed. This approach of using historic data can be very valuable if integrated in a timely manner.
Overall, the shift towards cloud-based remote monitoring in manufacturing seems to hold considerable promise for increasing operational efficiency and insights. However, a critical approach is necessary to fully understand how it truly impacts operations and to effectively leverage its full potential for optimizing the manufacturing process.
How Real-Time Operational Intelligence Reduced Manufacturing Downtime by 47% in 2024 - Automated Parts Inventory System Cuts Supply Chain Delays by 62%
In 2024, automated parts inventory systems have demonstrated a significant impact on supply chain efficiency, achieving a remarkable 62% reduction in delays. This improvement is particularly noteworthy given ongoing component shortages, especially in the electrical and semiconductor sectors. The automation inherent in these systems improves the accuracy of real-time inventory data, minimizing human error and streamlining the management of parts. However, the increased reliance on automation for parts inventory could lead to concerns about a potential decrease in certain crucial skills within the workforce, or an erosion of human oversight in the inventory management process. The manufacturing sector's shift towards performance-based contracts as a method to ensure operational uptime highlights the importance of these automated systems in securing a competitive edge within an increasingly complex industrial landscape. It remains to be seen if this heavy reliance on automation ultimately benefits the workforce or if the industry is potentially sacrificing valuable knowledge and skills in the process.
It's interesting that these automated parts inventory systems are being touted as a solution for the supply chain delays we've been seeing, especially with the ongoing component shortages, particularly in the electrical, electronics, and semiconductor sectors. Apparently, they've been able to cut these delays by 62%, which is a significant improvement. One could hypothesize that a key factor driving this reduction is likely the increased accuracy they offer. While manual systems often struggle to maintain accuracy above 80%, these automated systems seem to be able to hit above 95%, significantly reducing the incidence of human errors in stock tracking.
Beyond simply improving accuracy, these automated systems seem to have a significant impact on overall supply chain management. For instance, they're supposedly helping companies improve inventory turnover rates by 30% on average. This can be quite helpful for manufacturers who often need to juggle their working capital effectively. Another aspect is the ability to track inventory in real-time. With a manual system, it can take days to update stock statuses, but these automated systems are supposed to provide nearly instantaneous updates, allowing for far more agile responses to supply chain disruptions. This real-time visibility is key for minimizing disruptions to production and responding more effectively to unforeseen changes.
The algorithms employed by these systems seem to be quite sophisticated, capable of predicting demand trends with high precision, leading to a reduction in both overstock and stockout situations. This is a crucial aspect of efficient inventory management, as it directly impacts production flow and customer satisfaction. Furthermore, these systems can leverage data from multiple sources, such as sales forecasts and historical data, making them capable of adapting to dynamic market conditions. This dynamic adaptability is crucial in today's environment with the increasing uncertainty in global supply chains.
The cost benefits of these systems are also interesting. We are seeing reports of a labor cost reduction of 20% or more in companies who implement these systems. This reduction is due to the fact that fewer workers are needed to perform manual checks and manage stock replenishment. Additionally, these systems seem to provide improved tools for inventory valuation and reporting, generating insights that can inform better strategic decisions.
From a customer perspective, one of the key impacts is the improved speed of order fulfillment. By reducing delays, these systems indirectly enhance customer satisfaction with delivery times. They also seem to help refine the development of safety stock strategies. Based on data analysis, the automated systems can calculate the optimal level of safety stock to ensure facility readiness for unexpected demand surges without the overhead of unnecessarily high holding costs.
Last but not least, these automated systems offer great scalability, making them easily adaptable as production demands change. Manufacturers can increase their capacity for inventory management without dramatically expanding their infrastructure or workforce.
Of course, further investigation is needed to fully understand the long-term impact of these automated inventory systems on manufacturing operations and supply chains. However, the reported benefits are quite compelling, especially within the context of the 47% decrease in overall manufacturing downtime we've observed throughout 2024. It's fascinating to see how technology is impacting our industrial processes and helping us become more resilient and adaptable in these uncertain times.
How Real-Time Operational Intelligence Reduced Manufacturing Downtime by 47% in 2024 - AI Powered Quality Control Decreases Defect Rate from 2% to 1%
AI-powered quality control has demonstrably reduced defect rates in manufacturing, achieving a noteworthy drop from 2% to a mere 1%. This advancement underscores the capacity of artificial intelligence to revolutionize quality assurance processes. By automating inspections and leveraging advanced image analysis, AI can rapidly pinpoint inconsistencies and maintain higher product quality standards, particularly within intricate manufacturing environments. This speed and accuracy offer significant improvements compared to traditional inspection methods.
However, this technological shift also introduces considerations regarding the potential for overdependence on AI. It's crucial to ensure that the integration of AI doesn't diminish the value of human expertise and oversight in quality control decision-making. Striking the right balance between AI's capabilities and the experience of human operators will be vital in ensuring the ongoing efficacy and reliability of these new quality control systems. As the use of AI-powered quality control expands, careful monitoring of its impact on the manufacturing workforce and the overall quality of products will be essential to maximizing its benefits while mitigating any potential drawbacks.
In the pursuit of optimizing manufacturing processes, the integration of AI-powered quality control has emerged as a significant driver of improvement. Specifically, it has led to a notable reduction in the defect rate, dropping from 2% down to a mere 1%. While this seemingly small change might seem insignificant at first glance, it signifies a significant step towards enhanced product quality and a reduction in waste.
One of the key aspects of this advancement is the ability of AI systems to reduce inspection frequency. Essentially, AI-powered systems have enabled manufacturers to halve their typical inspection schedule, meaning that manual checks are now needed far less often. This freed-up time can be redirected towards more productive activities within the manufacturing process, potentially leading to greater overall efficiency. It's intriguing to consider the trade-offs involved in reducing human inspection frequency, and the extent to which the AI systems can truly replace those inspection functions.
Further adding to the value of AI-powered quality control is the improvement in defect detection rates. Using advanced image recognition and analysis tools, these AI systems can detect defects and anomalies up to 30% more effectively than traditional inspection methods. This translates to a lower chance of defective goods making it to the market, which is a significant boost to overall product quality. However, it is crucial to acknowledge that the accuracy of these systems is still developing and may not be completely reliable in all scenarios.
The economic implications of this shift towards AI are compelling. A 1% defect rate translates to reduced rework and potentially millions of dollars saved annually for companies that produce large volumes of goods. While it's hard to precisely quantify this value for every situation, the potential for significant cost reduction is undeniable. One wonders if this cost reduction might lead to changes in pricing or investments in other areas of the manufacturing process.
It's also interesting how AI systems bring about a new level of real-time responsiveness to quality issues. Unlike traditional inspection methods that operate on a periodic basis, the AI systems constantly analyze production data and enable immediate adjustments. This dynamic capability provides an opportunity to address quality issues promptly, something that was previously impossible with conventional methods. But one has to question if this real-time responsiveness might overwhelm operators if not carefully managed, potentially hindering their ability to prioritize critical issues.
Moving beyond real-time responsiveness, AI also allows manufacturers to significantly reduce the margin of error during production. Estimates indicate that using AI for quality control can lessen the margin of error by around 25%. This kind of precision leads to increased product reliability and a potentially better customer experience. It's crucial, however, to remember that these systems are built on algorithms and data, which can be prone to bias or error.
A clear outcome of this shift towards AI in quality control is the reduced number of product returns. Reports suggest that the rate of product returns due to defects has fallen between 15-20%. This decrease holds significant value for customer satisfaction and for the perception of a company's product and brand. Further research into the reasons behind these returns and how they specifically relate to the quality of AI-detected defects would be valuable to better understand this impact.
Another significant aspect of AI-powered quality control is the self-learning capabilities of the underlying machine learning algorithms. By utilizing historical data, the algorithms continually refine their defect detection skills, getting progressively more efficient over time. This continuous learning element is unique to AI systems and offers a potentially significant advantage over static, traditional methods. But it's important to be mindful of the potential for unintended biases that might develop in the algorithms over time due to the nature of the historical data being used for training.
Furthermore, it's been observed that this shift to AI-powered quality control can potentially reduce operational costs by roughly 18%. This reduction primarily stems from the diminished reliance on manual inspections and the swift defect identification enabled by AI. These savings can be re-invested in other critical areas of the manufacturing process, potentially generating further gains in efficiency. However, it's crucial to consider the initial investment cost of implementing these AI systems and whether this investment leads to long-term financial benefits.
The ease with which AI systems can be integrated into existing manufacturing workflows is a significant advantage. Implementation often takes just weeks rather than months, allowing manufacturers to rapidly adapt and leverage this technology. It's imperative to understand how seamless these integrations truly are and how the transition might impact the existing manufacturing teams and processes.
Finally, AI systems in quality control offer a unique advantage through long-term defect trend tracking. This feature goes beyond monitoring current quality and enables a deeper understanding of past defect patterns. This insight offers manufacturers a powerful tool for guiding future product development and continuous process improvement. However, the effective implementation of such long-term data analysis requires careful consideration of the data storage and analysis processes.
In conclusion, the integration of AI-powered quality control into manufacturing processes holds considerable promise for enhancing product quality, increasing efficiency, and minimizing costs. However, as with any transformative technology, careful consideration of the implications, potential trade-offs, and continuous assessment are needed to fully optimize its benefits. Further investigation into specific industry applications, long-term effects, and any potential societal impacts will help provide a complete picture of the role of AI in modern manufacturing.
More Posts from zdnetinside.com: