ServiceNow Knowledge 2024 7 Key AI Advancements Transforming Enterprise Operations at Las Vegas Conference
ServiceNow Knowledge 2024 7 Key AI Advancements Transforming Enterprise Operations at Las Vegas Conference - Now Assist Text Generation Tool Expands Language Support to 25 New Markets
ServiceNow's Now Assist, a tool that generates text, has recently broadened its reach by supporting 25 new languages. This expansion, which became effective in May 2024, is part of a broader effort highlighted at the Knowledge 2024 conference in Las Vegas. The goal, as presented at the conference, is to further leverage AI within businesses for improved productivity. Now Assist has picked up some new capabilities, including features that can summarize cases and even translate plain language instructions into code. These functions are expected to be fully available as part of a future software release, codenamed Vancouver. Additionally, ServiceNow has worked on increasing integration with Microsoft's Copilot, suggesting that the combined offering will give a more coherent AI experience. Despite the promise shown, it's unclear if these improvements will live up to their potential, especially in terms of really improving the user experience across the wide range of how businesses operate. Achieving true value from these advancements will depend on how well they are applied in the real world.
The expansion of Now Assist's language support to 25 new markets is a notable development, potentially boosting its usefulness across a broader range of global operations. This suggests that ServiceNow is actively working to cater to organizations with complex international footprints and diverse customer bases. It's interesting to consider the potential implications of this for customer service and internal workflows that deal with users from various backgrounds.
While the exact impact of language support on customer satisfaction remains debatable, studies have suggested that it can have a considerable influence. It is encouraging to see the advancements in natural language processing which seem to be enabling Now Assist to not just translate, but also understand contextual subtleties and regional dialects, something that has historically been challenging for AI-driven tools. It’s fascinating how machine learning is enabling the tool to adapt content to suit various linguistic styles, making for more relevant interactions.
The ability to communicate in a wide range of languages naturally expands the potential user base and markets where Now Assist can be applied. However, it's worth examining how they've achieved this level of accuracy. It's plausible that prior to this advancement, achieving this scale of multilingual support may have demanded heavy manual intervention. The move towards more automation through AI-driven language processing is a noteworthy development, possibly suggesting a decrease in the need for human oversight.
The importance of using native languages for user interactions cannot be overstated. The improved accuracy and contextual awareness facilitated by Now Assist could certainly lead to a decrease in misunderstandings, as implied by some studies. This enhanced communication might have a significant influence on customer service or internal workflows where precise interactions are paramount.
This trend towards greater emphasis on local language nuances seems to be gaining momentum across enterprise software, likely driven by an increasing awareness of the importance of catering to diverse client populations. Customer service or related operations seem like the most immediate beneficiary of these improvements. We should critically evaluate how the underlying models are being trained and refined. It's vital to ensure they are constantly updated with local datasets to guarantee accuracy and relevance across specific cultural contexts, going beyond basic translation.
The introduction of these new language capabilities underscores ServiceNow's effort to remain a key player in the constantly evolving enterprise technology sector. Their focus on digital channels and expanding global reach through such features suggests a strategic awareness of how businesses are operating in today's globalized landscape. It's a smart move if they are executing it well, but time will tell the real impact of these new capabilities.
ServiceNow Knowledge 2024 7 Key AI Advancements Transforming Enterprise Operations at Las Vegas Conference - Virtual Agent Builder Introduces Real Time Conversation Design Analytics
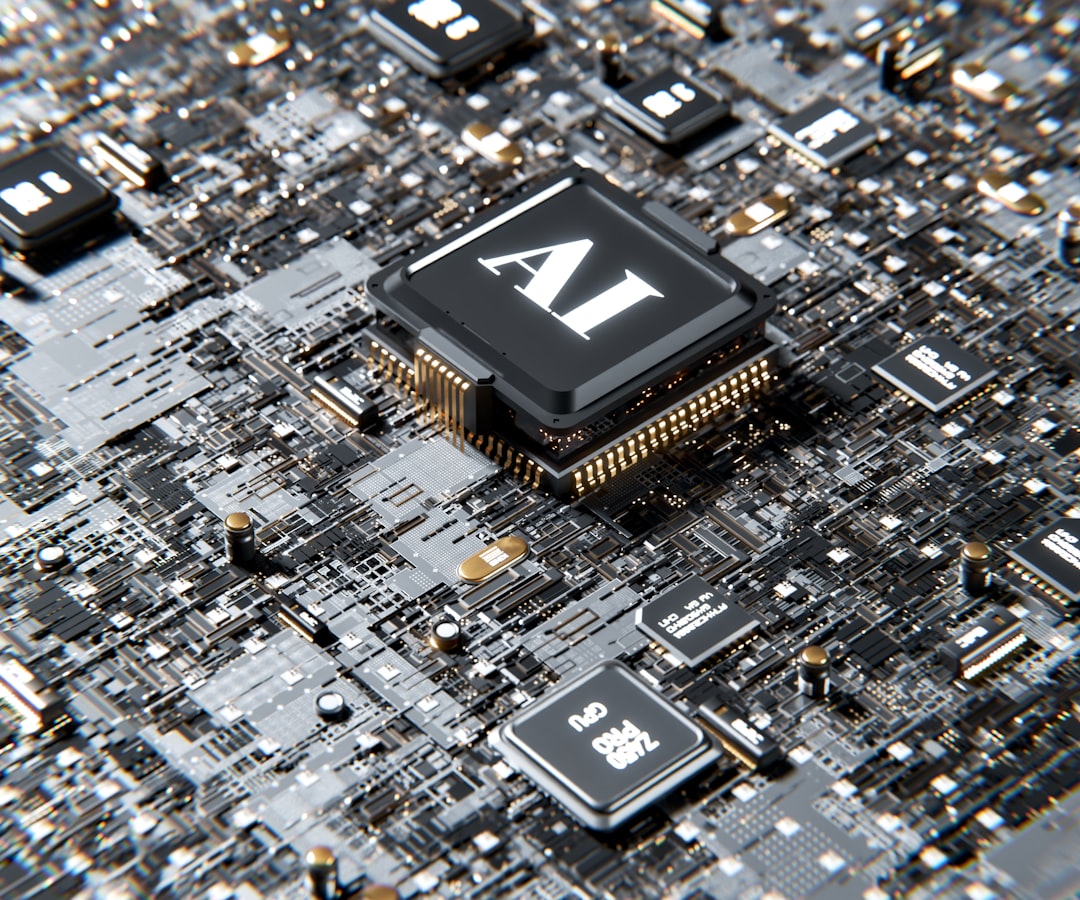
ServiceNow's Virtual Agent Builder has introduced a new feature: real-time conversation design analytics. This is meant to help businesses better understand how their virtual agents are performing and improve them. The new analytics tool tracks how often the virtual agent is used and how well it's doing its job, looking at things like cost savings and customer happiness.
A new dashboard, called Conversational Analytics, provides insights into how users are interacting with the virtual agent. This helps businesses see which topics aren't working well and make improvements to the agent's responses and conversational flow. The goal is to increase user engagement and encourage more people to use the virtual agent instead of contacting a human, hopefully easing the workload on live agents.
This advancement in virtual agent technology was presented at the ServiceNow Knowledge 2024 conference, where ServiceNow highlighted various AI improvements. While the new analytics feature is promising, we need to see how well it truly translates into tangible benefits for businesses in different industries. It remains to be seen if it will lead to substantial improvements in operational efficiency and the customer experience.
ServiceNow's Virtual Agent Builder has added a new feature, real-time conversation design analytics, which allows companies to see how their virtual agents are performing in the moment. This kind of real-time feedback on interactions can help them react much faster to what's going on. It's like having a live window into the conversational effectiveness of the virtual agent, something that was previously difficult.
Instead of just creating a chatbot and hoping it works, businesses can now actively track how well it's doing, which allows them to adjust the virtual agent's behavior on the fly. This ability to change how a conversation unfolds while it is in progress represents a shift away from the traditional fixed, pre-designed dialogue model. Essentially, the virtual agent gets more adaptable, which is key for improving the customer experience.
Beyond simply tweaking a few things, the analytics offer a deeper look at user behavior. It can potentially reveal patterns of how people are engaging with the chatbot, highlighting preferences or common problems. This level of insight can drive more targeted designs, improving satisfaction by catering to how customers actually use the system.
One major advantage is the ability for the virtual agent to keep a better track of the flow of a conversation. Understanding the context of the conversation across multiple exchanges, is a tough problem for AI systems, and this new tool could help virtual agents stay on point and give more relevant responses. It could be useful in situations where customers ask complex questions or need a few exchanges to get the information they need.
ServiceNow’s approach seems to be geared toward giving flexibility to companies on what they track. Instead of being forced to use a set of pre-defined metrics, the company can customize them. This means organizations can zero in on what is important to them, whether it’s response time, mood of the customer, or how well the agent solves the problem. This approach allows for a more tailored and insightful performance evaluation.
Another interesting aspect is the possible impact on how AI bias might be reduced. If the system is continuously refining language models with fresh data, it's possible that the virtual agent can become less biased over time. It would be useful if it can adapt to a wider variety of people's phrasing, particularly across groups that may tend to be underserved by AI.
It's worth noting that this analytics feature is designed to span across a wide range of communication channels, such as text, voice or email. This suggests a consistency in data collection, potentially leading to consistent improvement across different interactions. Further, the ability to incorporate this data with CRM or ITSM systems could create a fuller picture of the customer journey within a company, potentially improving operational efficiency.
An intriguing possibility is that this may eventually lead to AI models that can predict what users want before they ask. Imagine if a virtual agent could anticipate the next step in a customer interaction based on their patterns of behavior. That might lead to a smoother and more proactive customer service experience. It's still a bit early to say for sure, but if successful, these analytics could allow virtual agents to move from just responding to customer needs to anticipating them.
However, as with any AI-driven system, there are still questions about the actual value. We will need to see if it can really translate into meaningful improvements in customer service, beyond simply generating interesting data points.
ServiceNow Knowledge 2024 7 Key AI Advancements Transforming Enterprise Operations at Las Vegas Conference - Process Mining Software Adds Automated Root Cause Detection
At the ServiceNow Knowledge 2024 conference in Las Vegas, a key AI advancement was the introduction of automated root cause detection within their Process Mining software. This new capability is intended to help businesses more efficiently resolve problems by automatically figuring out what went wrong. One of the ways this is done is by looking at data across multiple sources – essentially bringing things together to get a fuller picture of the issue. For example, it can help spot Service Level Agreement (SLA) breaches by combining data from various systems.
But it doesn't stop there. The software also leverages machine learning techniques to perform something called "cluster analysis". This fancy term means it can quickly identify patterns and areas where processes are bogged down or not performing optimally. The idea is that it can flag bottlenecks or inefficiencies within your operations that may not be obvious otherwise.
Another interesting aspect is the increased collaboration the new feature allows for. Teams can more easily share process maps and collaborate on finding solutions to improve processes. It's about creating a unified understanding of how processes are working, and how they can be refined.
Whether this ability to find the root cause of issues and streamline processes truly delivers on its promise is yet to be fully seen. There is still a need to see how this plays out in practice for organizations across different sectors. The hope is it provides a more data-driven approach for improving IT operations and efficiency.
ServiceNow's Process Mining software now includes automated root cause detection, which is a step up from the traditional manual approach of figuring out what went wrong. This new capability uses smart algorithms to sift through a massive amount of data about how processes are run, quickly pinpointing problems that would otherwise take a long time to uncover manually. This is a significant leap forward in making operational troubleshooting faster.
The integration of real-time data feeds lets the process mining tools give instant insights into where things aren't working smoothly in a process. This lets companies address problems as they happen instead of waiting until after the damage is done, which can minimize downtime and boost productivity.
There's a shift towards predictive capabilities as well. Newer process mining tools use past data alongside real-time insights to anticipate future problems in processes. The idea is to proactively stop potential issues before they disrupt operations, which is a valuable approach.
It's not just confined to one department; the automated root cause detection can delve into processes across different teams. This interconnected view means businesses can trace a problem back to its source, no matter where it begins, which gives them a much better understanding of the overall health of operations.
Decisions become more grounded in solid evidence when using this software. Pinpointing the root causes precisely leads to better-informed decisions that boost efficiency and resource allocation.
The algorithms at the heart of these process mining tools are designed to learn from the data they process. As they are trained on more information, they get better at finding the actual causes of problems, leading to ever-improving results over time.
The trend in these tools is towards dashboards that are easy to understand, so that even people who aren't technical experts can grasp the root cause analysis. This fosters a more data-driven culture across organizations.
Organizations that use these systems typically find that they have more visibility into how their operations work. Everyone involved can see workflows and bottlenecks clearly, leading to a more collaborative approach to fixing problems.
It's notable that the root cause detection features in process mining tools can usually be seamlessly integrated with existing IT systems that handle service management, which can make them more effective without demanding major changes or extensive employee training.
Finally, having an automated way to pinpoint the causes of operational issues can save a lot of money. It reduces the need for manual investigations and redoing work, making this approach cost-effective for boosting efficiency in various industries. It remains to be seen how widely these features are adopted across the field, but the initial reports are promising.
ServiceNow Knowledge 2024 7 Key AI Advancements Transforming Enterprise Operations at Las Vegas Conference - Employee Center Platform Integrates New Task Automation Features
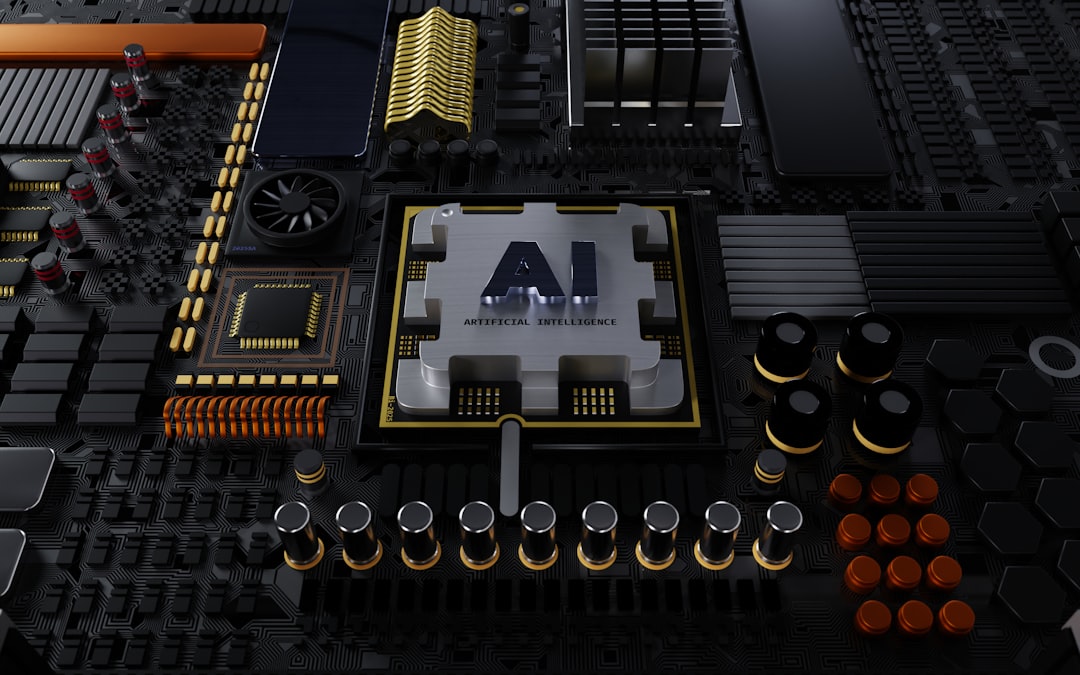
During the ServiceNow Knowledge 2024 conference in Las Vegas, the Employee Center platform was highlighted for integrating new task automation features. These features are designed to simplify work by automating routine tasks and providing a consistent experience for employees across various business functions. The idea is to streamline operations and improve employee satisfaction. This integration is part of the wider push for automation in enterprise software, which aims to improve productivity and efficiency.
However, the real impact of these features is still to be determined. While theoretically sound, it remains unclear whether these new automation capabilities will genuinely enhance employee experience and lead to tangible improvements across a variety of business scenarios. The success of these automated features will ultimately hinge on their ability to be effectively implemented and tailored to the specific needs of different organizations and employees. It's crucial that these tools are flexible and adaptable enough to meet the challenges of a wide range of working environments and employee preferences.
At the ServiceNow Knowledge 2024 conference in Las Vegas, they showcased enhancements to the Employee Center Platform, specifically focusing on new task automation capabilities. The idea behind these features is to make everyday tasks more efficient by automating routine processes. This, in theory, allows employees to focus their time and energy on more complex and meaningful work. It's interesting to see how this could play out in different work environments. Studies have suggested that automating repetitive tasks can lead to a significant reduction in the time needed to complete them – possibly up to 80%. However, we should be wary of assuming that these improvements will translate equally well across various sectors.
Many of these automation features seem to rely on machine learning algorithms that learn from employee behavior. This means that, over time, they may become increasingly adept at recognizing patterns and automating tasks more accurately. This adaptability is potentially quite useful from an operational perspective, allowing workflows to evolve and become more intuitive. But it also makes me question how this will impact employee roles in the long run. It's also crucial to consider the ethical implications of systems learning and adapting from user data.
A noteworthy feature is the integration of these automation capabilities with other systems and departments. This allows tasks to be woven into broader workflows, creating a more interconnected and potentially more streamlined approach to task management. One benefit is that you might get a fuller picture of how tasks relate to each other, but it also introduces complexity. It's a bit like a complex puzzle, where each piece – a separate application or workflow – needs to fit in perfectly.
The automation features also incorporate real-time analytics that can provide insights into task performance. This might give organizations more precise data on how these tasks are being handled, potentially helping optimize processes and understand where improvements could be made. Having access to this type of granular information could prove quite valuable, particularly for those organizations seeking a deeper understanding of how their work processes are functioning.
Interestingly, studies have indicated that employee satisfaction often increases when working with automated systems. If these automated features are well-designed and implemented, it's plausible that employee satisfaction might improve as the burden of repetitive tasks is reduced. This makes sense, but I think it's important to assess whether the perceived increase in satisfaction outweighs any potential drawbacks, like employees feeling less valued in their roles.
However, we need to be aware of the potential downside to increased automation. If companies become overly reliant on these systems without careful oversight, it could lead to a situation where human expertise and decision-making are marginalized. This could erode the importance of some human skills, possibly negatively impacting organizational culture. There needs to be a careful balance between leveraging the capabilities of automation while still retaining essential human elements in the workplace.
The adoption of advanced task automation technologies can offer tangible cost savings. By streamlining workflows and potentially reducing human intervention, businesses may experience reduced operational expenses and lower employee turnover. Initial estimations suggest that companies effectively implementing automation can reduce their service delivery costs by 25-30%. That's a substantial saving that certainly could be enticing, but I think it's critical to question how sustainable this is, especially if there are hidden costs.
Another interesting aspect is the use of Natural Language Processing (NLP) in some of these features. This enables users to interact with the system using conversational language, mirroring a trend towards more user-friendly interfaces. It's a clever approach, but it does raise questions regarding the limitations of current NLP technology in handling nuanced or complex communications.
One potential benefit is improved compliance and risk management. Consistent application of predefined processes through automation could help reduce errors associated with human intervention. This makes sense, but I am a bit cautious. A perfect example would be in areas with legal and regulatory requirements, where it is important to ensure tasks are completed in compliance with set standards.
While these automated features have clear benefits, their implementation should be approached cautiously. Companies need to thoughtfully assess their current infrastructure, potentially invest in employee training, and prioritize a smooth integration to avoid disruption to ongoing operations. Otherwise, you risk encountering setbacks that could have been avoided. It's a matter of carefully analyzing the risks and benefits before diving into these systems head-first.
In summary, it appears these new task automation features in the Employee Center Platform are part of an ongoing effort by ServiceNow to enhance operational efficiency and improve the employee experience. Whether or not these features live up to their promise remains to be seen. A close evaluation of the real-world impact in various environments will be needed to understand how they truly change the dynamics of enterprise operations.
ServiceNow Knowledge 2024 7 Key AI Advancements Transforming Enterprise Operations at Las Vegas Conference - ServiceNow Manager Hub Launches AI Powered Performance Tracking
ServiceNow has unveiled the Manager Hub, a new tool that uses artificial intelligence to track team performance. The idea is to give managers better information and suggestions on how to help their teams succeed. This includes things like insights into individual and team performance, as well as guidance for improving team dynamics and effectiveness. The announcement was made during the Knowledge 2024 conference in Las Vegas, part of a larger push by ServiceNow to use AI to improve how businesses operate.
While this new tool has the potential to streamline management tasks and boost team performance, there are still some unknowns. We'll need to see how effectively it integrates into how managers actually work and if it truly leads to noticeable improvements in productivity and collaboration. It's worth considering that as businesses rely more on AI for management tasks, there's the risk of unintended consequences. This includes how it might affect a manager's role or how it interacts with existing management approaches. It's important that any AI tool used in this way is thoroughly tested and understood to maximize its potential and minimize any negative impact on how teams work.
At the ServiceNow Knowledge 2024 conference in Las Vegas, ServiceNow unveiled their new Manager Hub, a tool that uses AI to track team performance. It's designed to give managers a clearer picture of how their teams are doing and offer suggestions on how to improve things. This is interesting because traditionally, performance tracking relied heavily on manual processes and reports, which could be time-consuming and error-prone.
The Hub's AI seems to be pretty good at automating the process of monitoring key performance indicators (KPIs) across different departments. This automation minimizes the chances of human error and gives managers the ability to jump in and fix things before they become big problems. It also uses AI to predict how performance might change in the future. By looking at past data, the system attempts to predict future trends, helping managers to plan ahead and make better decisions about resources and priorities.
This automation isn't just about efficiency; it also looks to ease the burden on managers. The goal is to allow them to focus on higher-level strategic tasks instead of getting bogged down in data collection and analysis. This shift could be beneficial to management teams, potentially leading to increased job satisfaction and overall productivity.
One of the interesting aspects of the Hub is that it provides real-time feedback. This enables managers to react quickly to any issues and tweak their plans on the fly instead of relying on outdated periodic reviews. Having this dynamic feedback capability can potentially make performance management a more agile and responsive activity.
It's not limited to one department either; the Manager Hub attempts to draw data from multiple departments, offering a comprehensive view of performance across the organization. Getting a holistic understanding of performance across different parts of a business can be a challenge, so the Hub's attempt to consolidate this information could prove useful, particularly for improving collaboration between teams.
Another thing that I found curious is that it includes measurements of employee morale and engagement, which can be strong indicators of productivity. This feature suggests that ServiceNow is thinking about how workplace culture and overall team sentiment might impact performance. By understanding these factors, organizations might be able to improve retention and take a more proactive approach to fostering a positive work environment.
The integration with other existing IT systems is also noteworthy. The Hub can smoothly work with systems already in use, minimizing disruption during implementation and ensuring a smooth transition for users. It also seems to be built to scale, meaning that it can handle the needs of both small and large businesses. This scalability could be beneficial for organizations as they grow and expand.
Overall, this new Manager Hub and the AI-driven performance tracking features seem to push the needle on data-driven decision making within organizations. With access to these performance metrics, managers are empowered to make decisions based on hard data rather than intuition or guesswork. In the face of the ever-increasing complexity of businesses, this could be a valuable advantage.
While the concept is sound, we'll need to wait and see how effectively the Hub performs in real-world situations across various industries and company sizes. It will be particularly interesting to see if it can truly optimize performance outcomes. The potential benefits are certainly enticing, but as with any new technology, success will depend on the nuances of implementation and whether it lives up to its potential.
ServiceNow Knowledge 2024 7 Key AI Advancements Transforming Enterprise Operations at Las Vegas Conference - Text to Code Generator Beta Released for Platform Development
ServiceNow unveiled a beta version of their Text to Code Generator at the Knowledge 2024 conference in Las Vegas, highlighting it as a new tool for platform development. This feature, powered by generative AI, lets developers translate simple English instructions into code specifically for the ServiceNow platform. The intention is to boost efficiency, enabling developers to build applications and automate workflows faster by simply describing what they want in plain language. While it's meant to improve developer productivity, the effectiveness of this approach across the range of business situations is still unclear. ServiceNow plans to make this feature widely available with the Vancouver release. Whether it significantly improves user experience and boosts operational efficiency across different types of businesses remains to be seen, it all depends on how well it actually works in real-world situations. It will require careful implementation within existing development workflows to truly demonstrate its value.
ServiceNow's recent release of a beta version of their Text to Code Generator, showcased at the Knowledge 2024 conference in Las Vegas, is a fascinating development in the world of AI-powered software development. This new tool aims to make coding simpler by allowing developers to translate their ideas into code using plain language instructions.
It's interesting how they are employing sophisticated natural language processing techniques to not just translate commands but to actually understand the intent behind them. This could be incredibly beneficial for those who aren't expert programmers, making coding more accessible. The tool also seems to be getting smarter over time by learning from its interactions with users, which could lead to progressively better code generation.
Another intriguing element is the context awareness aspect. The tool seems capable of adapting the generated code based on the specific details of each problem, rather than just relying on pre-set templates. This could be a game-changer in code generation as it potentially eliminates the need for a developer to tweak the code constantly for each new circumstance.
One potential benefit is improved developer efficiency. The beta version aims to integrate with popular developer tools (IDEs), providing real-time code suggestions within the developer's existing workflow. This seamless integration could streamline the process by eliminating the need to switch between different software. We'll have to see how well it actually integrates though, as there's always the risk of interference with the user's preferred coding environment.
Furthermore, they've incorporated a feature for users to customize the coding style and format of the output. This aspect, while seemingly small, could have a big impact on collaborative projects where coding consistency is crucial. The customization feature could be really useful for promoting uniformity across a team's projects.
Interestingly, the beta version includes the capacity to detect and suggest fixes for common coding errors. While still in its early stages, this feature has the potential to shorten debugging time significantly, which is usually a huge time-suck for any software development project.
However, there are some limitations to the beta phase, as expected. The developers have likely implemented restrictions to prevent the system from getting overloaded with queries during testing. This may affect the number of inquiries or the complexity of the problems users can address. We'll have to see how they will handle larger-scale applications when it eventually reaches full release.
There's also an interesting discussion on future possibilities. The tool may expand to become a project management and scaffolding feature in the future. This is a big jump from simply generating code snippets. If successfully implemented, it could completely alter how development teams approach projects.
Finally, ServiceNow has emphasized that the beta program is crucial for guiding future development. By collecting data on user interactions and the generated code, they can optimize the tool for a better user experience. User feedback is a key factor in shaping the technology, which hopefully makes for a tool that's actually useful in a real-world setting.
While it's still early days, this Text to Code Generator could revolutionize the way code is written. It's quite plausible that it might indeed transform the way we develop software in the future. The potential benefits are certainly exciting, but the success will depend on how the tool develops and matures based on user feedback and real-world deployment. It's a fascinating development, one worth following closely.
ServiceNow Knowledge 2024 7 Key AI Advancements Transforming Enterprise Operations at Las Vegas Conference - Enterprise Asset Management Gets Predictive Maintenance Upgrade
At ServiceNow's Knowledge 2024 conference, a key focus was on upgrading Enterprise Asset Management (EAM) with predictive maintenance features powered by AI. This shift means businesses can potentially foresee equipment failures, departing from the older, reactive approach to maintenance often found in traditional EAM systems. The new approach relies heavily on processing sensor data and past performance, enabling companies to manage their assets in a more strategic way that helps them reach other goals, like cost reduction and better service. As EAM evolves with these predictive abilities, there are opportunities for continuous improvement and a more forward-thinking approach to managing an asset's entire life cycle. It's becoming increasingly clear that EAM is a vital piece of how companies operate.
While this push for predictive maintenance is promising, the real-world implications and how smoothly it connects with existing systems are still under scrutiny. It's uncertain if these improvements will work well across all types of businesses and operational settings. It's a question of if the technology can truly deliver on its promise in practice.
At the ServiceNow Knowledge 2024 conference in Las Vegas, the focus on AI advancements in enterprise asset management (EAM) was particularly interesting, especially in how it relates to predictive maintenance. It seems that the way we think about keeping equipment running is shifting. Previously, many organizations just reacted to failures or used scheduled maintenance—a sort of preventative approach. Now, we're seeing these systems evolve to become more predictive. This means that the systems can essentially guess when equipment will fail based on historical data and other information gathered from sensors and operational conditions.
One of the fascinating things is the level of accuracy these systems are aiming for. Some vendors are claiming that they can predict failures with up to 90% accuracy, which could significantly reduce unplanned downtime. That's really important, especially if unexpected outages are costly or disruptive for a business. I'm curious to see how well these accuracy claims hold up in practice, particularly across different industries and types of equipment.
Another interesting aspect is the sheer volume of data involved. These systems can analyze data from IoT devices, thousands of sensors, and operational records to get a much better picture of the current and potential future state of the equipment. It's quite impressive how sophisticated this approach is. It also suggests a potential challenge in terms of data management and security.
Predictive maintenance also seems to have a big impact on costs. Many studies show that businesses can cut their maintenance costs by 10-40% by switching to this approach. This makes sense, because if you can predict issues and address them before they cause failures, you're likely to avoid the larger repair bills associated with those failures. However, getting these systems running can be complex and could require a substantial initial investment in hardware, software, and training.
It's also noteworthy that they're finding they can extend the life of assets with this approach. Some reports suggest an increase in lifespan of 20-25%. This is probably because they can prevent small issues from escalating into larger problems. This is particularly relevant for critical equipment or infrastructure where prolonged downtime could be very expensive or harmful.
The integration with AI is intriguing, since it suggests that these systems can learn over time. As they collect more data, they can refine their predictions and potentially become more accurate. This adaptability is similar to how humans get better at a task with more experience, and that's a promising development for the field.
Moreover, predictive maintenance isn't just about fixing things. It can lead to better decision-making in general. The insights gained from these predictive analytics models can help prioritize maintenance tasks and allocate resources more efficiently. This kind of insight can help companies not only manage maintenance but potentially rethink their approach to inventory management and procurement.
Surprisingly, these systems also seem to positively affect workplace safety. Since they're able to anticipate when equipment is likely to break down, businesses can proactively address the problem, reducing the chance of workplace accidents caused by faulty machinery. That’s an important benefit and something we might expect to see increasingly emphasized as safety and well-being become more central in workplace operations.
Despite the numerous benefits, it's striking that predictive maintenance is still not widely adopted. Only about 30% of companies are currently using these systems. This could be due to the complexity of implementation, initial investment costs, or a simple lack of awareness about the potential benefits.
Finally, the fact that this technology is showing up in industries beyond manufacturing is fascinating. Healthcare, for example, could benefit significantly. The ability to predict when an MRI machine might need maintenance could be incredibly valuable for maintaining uptime and reducing service disruption.
Looking ahead, the predictive maintenance market is expected to grow substantially, potentially reaching a value of over $20 billion by the end of this decade. This growth is likely fueled by increased investment in IoT technologies and a greater recognition of the need for more efficient operational practices across different sectors.
In conclusion, it seems that predictive maintenance enhancements within EAM are changing how we approach keeping critical equipment running. While still not universally adopted, it offers a compelling alternative to traditional approaches by offering increased accuracy, cost reductions, and improved safety. It's a promising area, and I suspect we'll see continued innovation and development in the years to come, particularly as AI continues to advance.
More Posts from zdnetinside.com: