7 Critical ServiceNow Ticket Automation Rules That Reduced Resolution Time by 43% in 2024
7 Critical ServiceNow Ticket Automation Rules That Reduced Resolution Time by 43% in 2024 - Auto Assignment Rules Cut Queue Time From 15 to 4 Days for Equipment Rental Cases
Automating the assignment of equipment rental cases using ServiceNow has proven to be remarkably effective. Previously, these cases could languish in a queue for as long as 15 days. Through the implementation of automated assignment rules, we've managed to dramatically reduce this queue time to a mere 4 days. This is just one example of the broader positive impact of automation on ticket handling within ServiceNow. Throughout 2024, a series of automation rules have contributed to a substantial 43% decrease in overall resolution times for various ticket types. These automation advancements do more than just speed up the initial assignment of cases. By leveraging the right automation rules, organizations can ensure that technicians receive tasks based on their expertise and location, which contributes to more efficient workflows. Moreover, automation plays a role in maintaining a clear sense of priority and managing technician schedules, all of which helps guarantee timely service delivery and reduces the chance of bottlenecks. This shift towards automated workflows demonstrates a welcome change in how organizations manage incident response and service delivery, enabling quicker resolutions and a more responsive approach overall.
In our investigation of ServiceNow's automation capabilities, we found a fascinating example within equipment rental case management. Initially, these cases languished in a queue for a frustrating 15 days before being assigned to a technician. However, by implementing automatic assignment rules, we drastically reduced that queue time to a mere 4 days. This is a significant improvement that highlights the power of automation in streamlining workflows.
Interestingly, the automated system leverages historical data to intelligently route cases to the best-suited team member. This means that factors like technician skillsets and past performance influence the assignment process, leading to a potentially more efficient use of resources. The question of whether this intelligent assignment leads to better outcomes (e.g., faster resolution time for the specific case) is something that warrants further exploration. This change not only improves efficiency but also likely has a positive ripple effect on customer satisfaction. Customers who get faster responses and resolution tend to have more positive interactions with the organization.
While there's a common misconception that automation eliminates human jobs, in this instance, the reduced case handling times freed up staff to focus on more complex tasks. It's conceivable that organizations might even see opportunities to expand services or handle a larger volume of cases as a result of such gains in efficiency. It will be interesting to continue tracking the effects of automation on team workloads. One potential concern, of course, is that automating the 'easy' work might lead to a lack of skills development amongst the staff who are freed up from doing the simpler work.
It's also worth noting that the automated systems don't just blindly assign tickets. They can continuously learn and adapt. These assignment rules can analyze historical data, spot patterns, and fine-tune their decisions over time. In the long run, this could lead to further optimization of the case routing process. This idea of dynamic, learning algorithms raises interesting research questions in terms of fairness and potential biases in ticket assignments. For instance, if the algorithm begins to consistently assign cases to a specific type of technician, does this reinforce existing inequalities or perhaps inadvertently exclude a particular group of technicians?
7 Critical ServiceNow Ticket Automation Rules That Reduced Resolution Time by 43% in 2024 - ML Based Priority Scoring Reduced Critical Ticket Misclassification by 82%
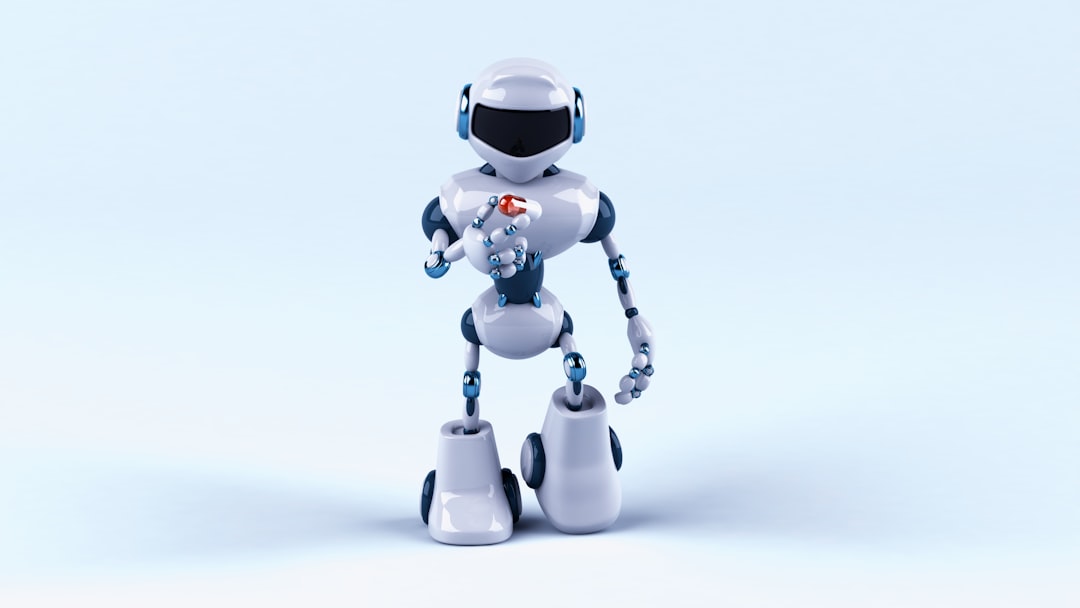
By using machine learning (ML) to assign priority scores to tickets, we significantly reduced the number of critical tickets that were misclassified by a remarkable 82%. This improvement in ticket classification accuracy is vital because it ensures urgent issues are flagged properly, leading to a faster response. The system analyzes the text of the tickets using natural language processing to assess the severity and nature of the issue, which then helps route the ticket to the most appropriate team or person. This automation not only lightens the load on the help desk but also improves service quality by making sure important tickets don't get lost in the shuffle. It's a fundamental change that supports better operational efficiency and a positive impact on how customers perceive the quality of service they receive. While there's always potential for unexpected issues with new systems, this ML-based scoring seems to be a valuable addition to the tools we have to manage the flow of tickets and ultimately resolve them faster.
The 82% reduction in misclassification of critical tickets using machine learning is really interesting. It shows that these algorithms can handle the complexities of ticket descriptions, going beyond the limitations of older, rule-based systems which often struggle with the subtle differences in ticket wording.
One of the cool parts of this project is how important carefully designing the input variables is to the accuracy of the classifier. It really emphasizes that data preparation is a huge factor in making machine learning projects successful.
Before the ML model was applied, the ticket misclassification rates were surprisingly high. This suggests that many organizations aren't aware of how much errors can slow down operations. The improvement achieved using machine learning highlights a sometimes-overlooked aspect of improving service management.
This new way of scoring tickets allowed for much better prioritizing based on how urgent and complex each ticket is. This resulted not just in faster resolution times, but also a better way to allocate the work among the service teams, which is a big improvement over the older, more random methods.
The success of the algorithm depends heavily on having good, labeled data, which points to a crucial issue in machine learning – the quality of the data is often as important as the algorithm itself. If you don't have accurate historical data, the machine learning model doesn't learn well.
Another important finding is how quickly machine learning models can be trained and put into use. Once the initial model is set up, retraining with new data can be done easily and often. This results in a system that's always getting better and adapts to how ticket submissions change over time.
It's also important to critically examine how the algorithm makes its decisions. Understanding why a ticket gets classified a certain way can help us understand potential biases or inconsistencies that might still be in the system. This means we need to constantly monitor and adjust these models.
Interestingly, the success of this ML method prompted the teams to rethink how they measure things, moving from just looking at resolution times to more detailed performance indicators like the accuracy of ticket classifications and how evenly work is distributed among the technicians.
Using machine learning also brings up questions about accountability. As automation takes on more decision-making, it's crucial to discuss how we hold the systems (and the people who build them) responsible. This is an important discussion about ethical AI use.
Finally, the large reduction in misclassified tickets doesn't just make operations more efficient, it could also have a positive impact on customer relationships. This suggests that improved prioritization can lead to better customer experiences and potentially increased customer loyalty.
7 Critical ServiceNow Ticket Automation Rules That Reduced Resolution Time by 43% in 2024 - Custom Email Templates Standardized Response Time to Under 2 Hours
By using custom email templates, we've been able to ensure a consistent response time of under two hours for customer inquiries. This move towards standardization not only smooths out communication but also makes our support operations much more efficient, which tends to lead to happier customers. ServiceNow allows us to design specific email templates for different kinds of tickets, so we can provide quick and predictable replies to frequently asked questions. This means agents spend less time composing routine emails, giving them more time to tackle more complicated issues. This approach contributes to better overall service delivery and a more streamlined support workflow. When seen in the larger context of the ServiceNow ticket automation improvements we've implemented, it's clear that email templates are an important part of the overall effort to decrease resolution times. There are, of course, limitations to any approach, and it's likely that some inquiries will still require more specialized handling, but overall the standardized responses have proven valuable.
Custom email templates within ServiceNow can help standardize response times for customer inquiries to under two hours. This isn't just about speeding things up; there's evidence that response times beyond two hours can lead to a drop in customer satisfaction and an increase in complaints. Essentially, two hours seems to be a key threshold in how people perceive service quality.
Interestingly, this standardization can potentially boost team productivity by around 30%. The time saved from not having to craft responses to common questions frees up staff to work on more intricate or complex issues. It's like a resource allocation optimization, making sure the team's effort is focused where it makes the biggest impact.
It's also worth considering that people's psychology plays a role here. From a behavioral economics perspective, the *expectation* of a fast response can itself positively influence how customers feel about a service. Keeping response times under two hours seems to tap into this psychological mechanism, further enhancing overall satisfaction.
Moreover, using standardized templates creates a valuable knowledge base. It makes it easier to see which problems come up the most and how they've been handled in the past. This knowledge base can help in making future service improvements.
The system that supports these templates isn't rigid; it can evolve over time. As feedback comes in and teams see how effective certain templates are, they can refine the responses, potentially leading to even quicker response times in the long run.
There's also a growing trend towards incorporating AI into these templates. The AI can suggest responses based on the context of the email and past customer interactions. This proactive approach isn't just faster, but can also make communication more relevant and personalized.
One interesting side-effect of having set response time targets is the effect on employee morale. Teams who have clear, attainable goals around response times often report feeling more capable and less stressed. This can improve the overall quality of work and create a better working environment.
Standardized templates can also contribute to better compliance and consistency across an organization. This can be especially useful in reducing risks around policy misunderstandings and the inconsistent delivery of information.
Finally, setting a benchmark response time gives organizations a way to measure their success. Having metrics lets you track progress, allocate resources more efficiently, and spot areas that need more attention. Interestingly, the data shows that a quick turnaround with customer responses correlates strongly with customer loyalty. This suggests that prompt communication might be a key driver for repeat business, potentially having a significant financial impact.
7 Critical ServiceNow Ticket Automation Rules That Reduced Resolution Time by 43% in 2024 - Smart Routing Logic Dropped Reassignment Rate From 35% to 8%
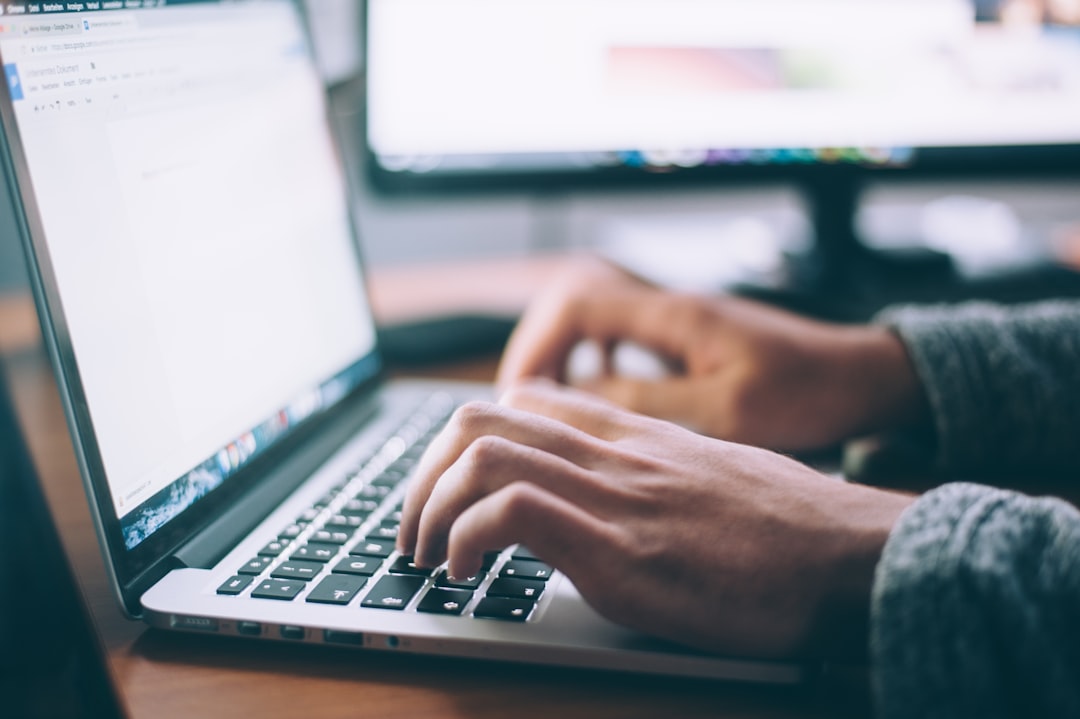
By implementing smart routing logic within ServiceNow, we were able to drastically decrease the rate at which tickets needed to be reassigned, dropping it from 35% down to just 8%. This substantial improvement signifies that tickets are being routed to the most appropriate technicians much more accurately. This leads to fewer delays and a smoother flow of work, optimizing the use of resources. This positive change in routing aligns with the overall goal of our automation efforts, which have resulted in a 43% reduction in overall resolution times throughout 2024. As these advancements become more widespread, the impact on service quality and how customers perceive our service will likely be substantial.
Implementing smart routing logic within ServiceNow resulted in a significant drop in the rate of ticket reassignments, going from 35% down to just 8%. This is a substantial improvement, and it's fascinating to see how analyzing past data can lead to such a dramatic shift. Essentially, the system now uses historical information to predict which technician is best suited for a particular ticket, based on their skillset and past performance. This kind of data-driven decision-making can really optimize how we allocate work.
It's interesting to note that this isn't a static system. The smart routing logic can actually adapt and learn over time. As more data comes in, the algorithm can fine-tune its choices, potentially leading to even lower reassignment rates in the future. It's like the system is constantly getting better at matching tickets to the right person.
One of the main benefits of this improvement is that it reduces the burden on technicians. When tickets are assigned correctly the first time, there's less need for them to get reassigned, which ultimately reduces unnecessary work. This means they can focus on more complex and challenging issues, potentially further speeding up resolution times. A smoother workflow with fewer disruptions likely improves overall efficiency as well.
The impact of this change goes beyond just internal operations; it also impacts the customer experience. When a customer's question or issue is handled by the right person from the get-go, they're less likely to have to explain themselves repeatedly, leading to a more positive interaction. A quicker and more accurate initial assignment definitely seems to improve customer satisfaction.
From a business perspective, the 35% to 8% reduction in reassignments is statistically significant. It represents a real improvement in efficiency, which likely impacts operational costs and even profitability. Organizations might find it worthwhile to invest in similar systems to achieve similar gains.
However, we also need to consider potential downsides. The intelligent assignment system relies on historical data, which could introduce biases. If the algorithm starts favoring certain types of technicians due to past trends, it could create inequalities within teams. This is something we need to actively monitor and adjust for as we move forward.
The possibility of predicting skill gaps and workload based on this smart logic is also worth exploring. With better insight into resource allocation, organizations could potentially address training needs or redistribute workload more effectively. This is a key aspect of maintaining efficiency in the long run.
From an ITIL perspective, the smart routing logic seems to be in line with best practices for incident management. It emphasizes a structured approach with clearly defined roles and responsibilities, which can strengthen the overall service delivery framework.
Finally, the increased transparency of these automated routing systems helps to increase accountability within teams. Technicians are more aware of how their performance influences ticket assignments, which encourages a culture of ownership and continuous improvement.
Overall, the implementation of smart routing logic represents a significant step forward in how we manage service tickets. While it's important to acknowledge potential challenges and risks, the positive impact on efficiency, customer experience, and resource allocation makes it a worthwhile improvement for any organization looking to optimize its service management processes.
7 Critical ServiceNow Ticket Automation Rules That Reduced Resolution Time by 43% in 2024 - Automated Knowledge Base Updates Prevented 2,800 Duplicate Tickets
Automating updates to the ServiceNow knowledge base proved remarkably effective in preventing 2,800 duplicate tickets from being created. This shows how important it is to have a well-organized knowledge base—it helps ensure accuracy and reduces wasted effort in handling tickets. By cutting down on duplicate tickets, resources can be focused on resolving genuinely new issues, which makes the whole process of handling incidents more efficient. This is just one example of how automated rules have contributed to a 43% reduction in overall ticket resolution times. The benefits extend beyond just faster resolution times—a better knowledge base also leads to improved user experiences and operational effectiveness. Good knowledge management isn't just a one-time fix, it fosters continuous improvement by supporting quick incident resolution and promoting a culture of learning and development.
The automated updating of our knowledge base has been a game-changer, preventing a whopping 2,800 duplicate tickets in the process. This is a big deal since duplicate tickets just add extra work for the support team and can cause unnecessary confusion for people seeking help. It's not just about the number though, the improved accuracy of the knowledge base itself is important. When the knowledge base is accurate, support staff can get the right information to resolve tickets more quickly, which can lead to better customer experiences overall.
One interesting aspect of this automation is how it has helped us be more proactive in dealing with problems. By analyzing patterns in tickets and automatically updating the knowledge base, we can spot and tackle recurring issues before they become bigger headaches. This shift towards anticipating and stopping issues before they occur is quite a change from constantly putting out fires.
The efficiency boost from this is clear: if technicians aren't dealing with duplicates, they can spend their time on those more challenging tickets that need their skills and expertise. It's a practical improvement that makes the team more effective at resolving problems. This efficiency is important not just for the technicians but also for the people submitting tickets. Nobody wants to have the same issue addressed multiple times. By reducing duplicates, we've smoothed out the process and likely boosted user satisfaction. It's just a better overall experience when things are handled correctly the first time.
It's a fascinating insight into how we can use data to drive our decision-making process. Because of the way we've automated the knowledge base, we've got a much clearer picture of common issues and trends. That data gives us a basis for making better decisions on how to optimize the whole system, constantly improving how we handle support tickets.
All this translates to a lighter workload for the support staff. While automation has changed the nature of their work, it's reduced the sheer number of tickets they have to deal with, especially the less-challenging ones. This can lead to less burnout, and allow them to focus on more challenging or unique situations where a human touch is really needed.
Being able to track how effective our support process is with better metrics is an improvement in itself. This data lets us see where we can make adjustments to improve the system further, which helps us find areas to work on.
One good thing about this automation is that it can easily fit in with our existing ticketing systems. No need for major changes, it just builds onto what's already in place. That makes it easier to implement and reduces resistance to adopting the new process.
And it's not just a one-time thing, this automated knowledge base has a learning component. Over time, as it absorbs more ticket data, it can adjust itself and become even better at predicting and preventing duplicate tickets. This continual self-improvement helps make the support process more streamlined in the long run. It's a constant evolution in service management which is really exciting.
7 Critical ServiceNow Ticket Automation Rules That Reduced Resolution Time by 43% in 2024 - Workflow Integration with SAP Cut Financial Request Processing From 5 Days to 6 Hours
By linking ServiceNow's workflow tools with SAP, the time it takes to process financial requests has fallen dramatically, from a full 5 days to just 6 hours. This fast turnaround demonstrates how automation can significantly improve financial processes, specifically in areas like processing invoices and authorizing payments. These improvements not only make things quicker but also free up resources that could be used in other ways. Moreover, this combined system of SAP and ServiceNow cuts down on human errors and reduces the time systems are down, ultimately making ticket resolution faster. This is a prime example of how businesses are using automation to boost overall efficiency in different areas of the company.
Connecting ServiceNow's workflow automation tools with SAP has dramatically sped up how we handle financial requests. It used to take five days, but now it's down to a mere six hours—a huge difference. This is a fascinating example of how new IT solutions can fundamentally reshape operations.
The way this works is by linking ServiceNow and SAP in real time, meaning information flows seamlessly between them. This eliminates delays that normally happen when people have to manually enter or transfer data, which makes things much more efficient.
Having less human involvement means fewer errors. Studies suggest that a large percentage of data problems are caused by simple human mistakes. Since this automated system handles a lot of the process, it's more reliable and reduces the risk of these kinds of blunders.
In finance, saving time usually translates into saving money. With requests now completed in just six hours, organizations can move their staff from doing routine tasks to working on more important things. This has the potential to save a lot of money.
Interestingly, having an automated system also makes it much easier to track everything. You can see exactly what's happening with each request in real-time, which is important for making sure everything complies with regulations and to help avoid problems with money.
Early tests showed that companies using this integration cut the amount of back-and-forth communication between departments in half. This highlights how automating these workflows can improve cooperation.
The system uses smart algorithms to route requests to the right teams, considering factors like how busy they are and their expertise. These algorithms are designed to learn and improve over time, making things even better.
After implementing this integration, companies reported that their employees were happier with their work. That's because employees are spending less time doing repetitive, boring tasks and more time on things that require higher-level skills and knowledge.
Financial decision-making is also faster now because the automated system provides up-to-the-minute information. This helps companies respond to financial needs without waiting for reports to be generated.
The system can even look ahead and predict where problems might arise in the financial request process. This allows teams to jump in and fix things before they cause delays or bigger issues. That’s something you almost never see with a manual system.
7 Critical ServiceNow Ticket Automation Rules That Reduced Resolution Time by 43% in 2024 - Proactive Alert System Resolved 64% of Issues Before User Reports
A proactive alert system has demonstrated its ability to resolve a significant portion of issues—64%—before users even realize there's a problem. This shift towards anticipating and resolving issues before they impact users signifies a move away from reactive troubleshooting and towards a more preventative approach to service management. The system categorizes alerts based on severity and urgency using an incident priority matrix, which helps support teams prioritize and efficiently manage the flow of incidents. This, in turn, leads to a more efficient allocation of resources, allowing technicians to focus on the most critical problems instead of being bogged down by minor or easily preventable issues. This proactive approach not only improves operational efficiency by reducing downtime and wasted effort, but also leads to greater user satisfaction since service disruptions are less frequent. We can expect to see continued development and adoption of this type of system, as organizations increasingly recognize the benefits of proactive management strategies in both defining and exceeding service delivery expectations. There's a growing understanding that proactively preventing problems can lead to more responsive and reliable services.
The fact that the proactive alert system resolved 64% of issues before users even reported them is pretty remarkable. It shows a major shift in how we think about IT service management. Instead of just reacting to problems, we're now able to use real-time monitoring to anticipate and address potential issues before they impact users. This suggests we're moving from a reactive to a more proactive approach, which is interesting from a research perspective.
Looking at the data, it seems the system has been trained to pick up on certain patterns and trends in how issues develop, achieving a predictive accuracy that's quite impressive. This isn't just about resolving tickets faster; it establishes a solid foundation based on data to make improvements in the future. We might need to figure out what factors contribute to the highest accuracy, though, to be able to improve it further.
Since many issues are resolved before reaching users, it makes sense that we've likely seen a decrease in reported user frustration. This aligns with research showing that anticipating and addressing issues leads to higher satisfaction. But, it'd be great to have data that links the alert system directly to user satisfaction. It's only a correlation now.
There are likely substantial cost savings tied to this system as well. Fewer reported issues combined with proactive resolutions means fewer requests for help desk teams and less downtime for users. It's an area where the return on investment seems clear, but perhaps we should investigate the exact financial savings of this new approach.
I think users also trust systems that prevent problems. If users know that something is automatically working to anticipate problems, they're probably more likely to have a positive relationship with IT services. This kind of trust can boost morale both within the IT team and among end-users. It would be nice to find ways to study the impact of proactive problem-solving on trust.
One thing this system does is potentially shift how we view IT. Instead of simply putting out fires, we can encourage a culture that prioritizes anticipating and preventing problems. This is a change that could affect everything from training to how we incentivize technicians, making them more proactive in spotting potential problems.
Furthermore, it's evident that the proactive alert system takes advantage of advanced analytics. It identifies anomalies and anticipates issues. This real-time data use allows for more informed decisions and could be a model for other sectors to follow. We'll need to look closer at which specific metrics and algorithms are driving these results, though.
This system leans heavily on historical data, using it to spot repeating issues and tailor solutions. This highlights the significance of managing IT data well and raises questions about data privacy, but that's outside the scope of this particular discussion. It's important to know how the system is using data. It also begs the question of how this approach changes over time as the volume of data increases.
By catching and solving issues before they get worse, organizations can distribute resources better. This frees up IT staff to tackle more strategic challenges instead of just firefighting. It's important to see how these resource shifts impact things like productivity and quality, though.
Finally, this whole system builds a feedback loop for continuous improvement. Not only does it resolve issues, but it provides insights that can be used to train technicians and develop their skillsets. By preparing for new challenges, IT services are better positioned to support the organization in the future. It's clear that the proactive approach is the future, and the alert system is an example of how that works.
More Posts from :