ServiceNow CMDB in 2024 Streamlining IT Infrastructure Management with Enhanced Cloud Integration
ServiceNow CMDB in 2024 Streamlining IT Infrastructure Management with Enhanced Cloud Integration - Cloud-Native CMDB Architecture Enhances Scalability in 2024
The promise of cloud-native CMDB architecture in 2024 is that it will be better equipped to handle the ever-increasing complexity of modern IT environments. This approach, however, brings with it some new challenges. For example, ensuring data integrity and consistency between on-premises and cloud CMDB systems can be a headache. While the promise of greater visibility and agility is enticing, there are still questions about how effectively the CMDB can truly manage data from disparate cloud providers, especially in hybrid environments. The jury is still out on whether the "single source of truth" ideal will be achievable, or if we'll just end up with a more complex patchwork of data sources. Ultimately, the success of this shift will depend on addressing these complexities while delivering real value to organizations.
The promise of cloud-native CMDB architecture for scalability is intriguing, but it's important to critically examine the claims. While the idea of real-time scaling to accommodate dynamic changes sounds appealing, I'm skeptical about its practical implementation. The claim of "independent scaling of specific services" in a microservices architecture is appealing, but I need to see real-world data to assess its impact on latency and asset tracking efficiency.
The notion of multi-cloud management through a unified view of the IT landscape sounds fantastic, but how does it truly handle the complexities of disparate cloud environments? The purported 70% improvement in deployment times through automated discovery needs validation with specific benchmarks.
While enhanced data analytics and AI-driven insights are touted as benefits, we need to ensure they're not just buzzwords. Are these features truly driving proactive optimization and issue identification, or are they simply adding layers of complexity?
The potential cost reduction and increased agility from a move away from physical hardware are enticing, but we need to consider the potential trade-offs in security and data sovereignty, particularly as we shift to cloud environments. Finally, the promise of continuous updates and feature enhancements in a cloud-native environment needs a reality check. How are these updates managed and tested to ensure seamless integration and security, especially in a dynamic multi-cloud environment?
In summary, while the concept of cloud-native CMDB architecture for scalability holds promise, it's important to temper expectations and look for concrete evidence of its practical benefits before embracing it wholeheartedly. As a researcher, I'm eager to see real-world deployments and performance metrics to evaluate its true impact.
ServiceNow CMDB in 2024 Streamlining IT Infrastructure Management with Enhanced Cloud Integration - AI-Powered Data Classification Improves CMDB Accuracy
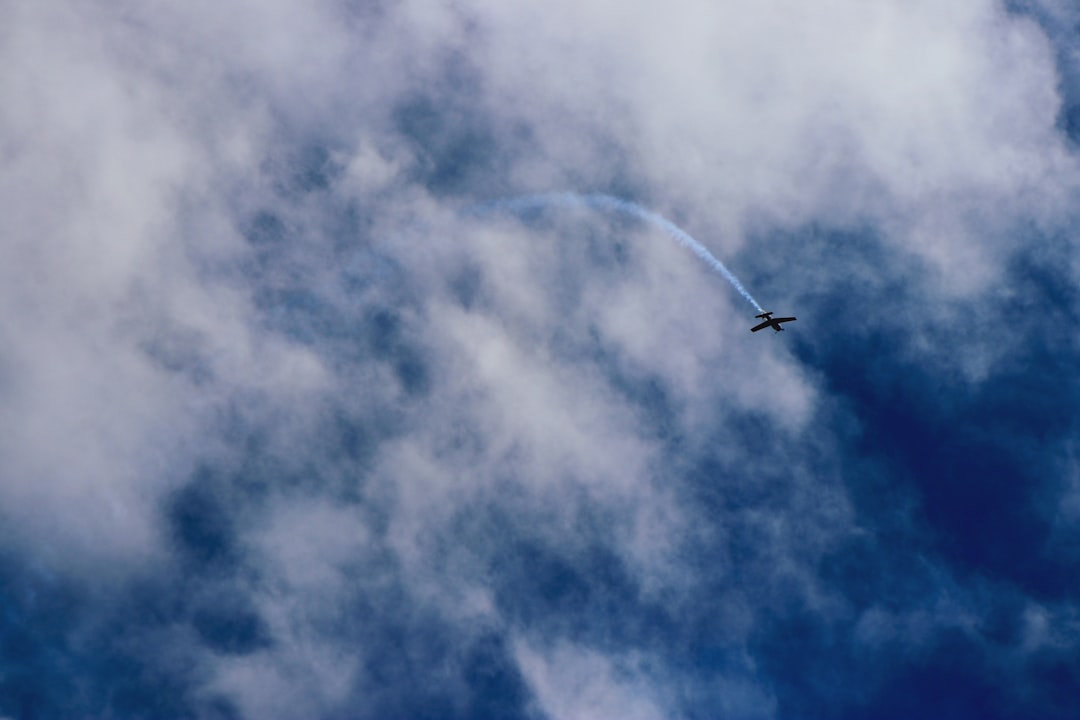
AI-powered data classification promises to make ServiceNow's Configuration Management Database (CMDB) more accurate. This is a key step for organizations hoping to use AI for IT operations. While the idea of a single, reliable data source sounds great, it's important to remember the challenges involved. Managing data from diverse sources, both on-premises and in the cloud, is a complex process. As organizations embrace AI for data classification, they need to be careful about potential problems. The key to success will be finding practical solutions that truly improve efficiency and deliver the promised insights.
The idea of using AI for data classification in ServiceNow CMDB sounds like a promising approach to address the challenges of managing data in increasingly complex IT environments. The potential for improved accuracy through automated data classification is certainly attractive, as it could significantly reduce manual errors. However, I'm always a bit skeptical of claims of drastic accuracy improvements. While AI algorithms are getting more sophisticated, the potential for bias and errors in AI-generated classifications is a concern.
Real-time analysis of data from various sources is appealing in theory, as it could potentially help keep the CMDB up-to-date with the dynamic changes happening in a modern IT landscape. This might lead to more efficient identification of dependencies and potential impacts of changes, which is crucial for preventing system outages. I'm interested to see how AI models will actually perform in real-world scenarios, especially in handling the intricacies of hybrid environments.
The concept of AI uncovering hidden relationships between Configuration Items (CIs) is intriguing. It could provide valuable insights that could lead to proactive management and optimization. However, I need to understand the limitations of these models and the potential for misinterpretations or generating false positives.
The claim of unsupervised learning to discover new assets autonomously sounds like a compelling feature for maintaining a comprehensive IT asset inventory. But I'd want to see evidence of its efficacy in dealing with diverse types of assets and the complexities of integrating data from multiple sources.
While the potential for enhanced data governance and compliance benefits is evident, I'm interested to explore how the integration of AI-driven data classification specifically addresses the nuances of regulations like GDPR and HIPAA. I'd be curious to see case studies of real-world implementations and their impact on compliance efforts.
The reported reduction in data reconciliation time is promising. However, I need to understand the specific circumstances under which this reduction was achieved and consider potential trade-offs, such as the amount of human oversight required for validation and error correction.
The potential for increased user satisfaction due to improved CMDB accuracy is a positive outcome. But I want to see evidence of the actual impact on service delivery and how AI-driven classification specifically translates to faster and more reliable service for users.
The prospect of predictive analytics capabilities for proactive issue identification is certainly alluring. However, I need to understand the specific capabilities of these models and their performance in anticipating issues across various IT environments.
The potential elimination of redundant data entries is beneficial for CMDB maintenance and operational efficiency. However, I'm interested in the practical implications of this feature, such as how it handles data updates and potential for data loss or inconsistencies.
The idea of AI managing data chaos in multi-cloud environments is appealing. But, as with any emerging technology, I would want to understand how AI-powered classification approaches the unique challenges of managing data across diverse cloud platforms and addresses security concerns associated with sharing data between different environments.
Overall, while AI-powered data classification has the potential to improve CMDB accuracy and streamline IT operations, I believe that further research and real-world testing are necessary to assess its true impact and ensure its effectiveness in tackling the complexities of modern IT environments. As a researcher, I'm eager to continue exploring the possibilities and limitations of this technology to understand its true potential for transforming IT infrastructure management.
ServiceNow CMDB in 2024 Streamlining IT Infrastructure Management with Enhanced Cloud Integration - Real-Time Asset Discovery Expands Across Hybrid Environments
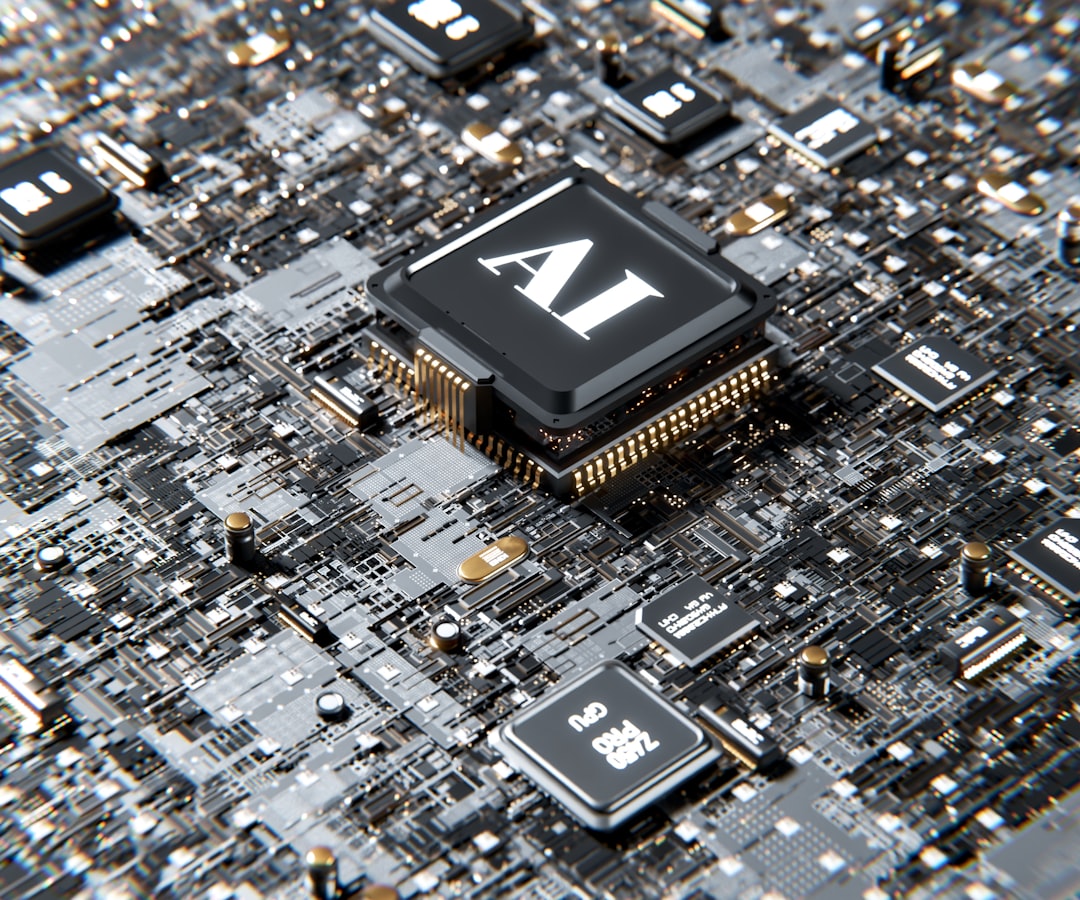
ServiceNow's CMDB is getting a real-time asset discovery upgrade in 2024, designed to give you a clearer picture of your assets across both on-premises and cloud environments. The goal is to create a more comprehensive and up-to-date CMDB, a single source of truth for your IT infrastructure. This includes automatically identifying hardware and software assets, even in virtual environments, and mapping how they're connected. This automated dependency mapping should make it easier to pinpoint problems and get systems back online quickly. The question remains though: how well will this work in real-world situations? The technology looks promising, but it'll need to demonstrate its ability to accurately track assets and manage dependencies across complex, dynamic environments.
Real-time asset discovery is evolving rapidly, offering the promise of a more dynamic and accurate view of IT assets in hybrid environments. This is particularly valuable as businesses grapple with the complex challenges of managing assets across on-premises and cloud platforms. However, there are interesting nuances to this shift that demand further scrutiny.
While the ability to map assets dynamically is a definite advantage, I'm intrigued by the claim that some real-time tools can actually *reduce* the frequency of asset scans while maintaining accuracy. This implies a significant improvement in efficiency, but I'd want to see concrete evidence of how this is achieved without compromising the comprehensiveness of the inventory.
Another intriguing aspect is the role of AI in enhancing contextual awareness. This suggests that real-time discovery tools are moving beyond simply identifying assets to understanding their significance in the broader IT landscape. However, I'm cautious about the reliance on AI and need to understand the specific algorithms and validation processes used to ensure accurate classification, especially in complex, hybrid environments.
The claim of seamless integration with on-premises and cloud sources is particularly appealing, addressing a long-standing challenge for organizations operating in hybrid setups. But I'm still skeptical about the level of complexity involved in bridging the data silos between different environments and ensuring data consistency.
It's compelling to hear that real-time discovery can provide granular visibility into dependencies among assets, potentially improving incident response times. However, I'd want to understand the practical implications of this visibility in the context of complex and dynamic hybrid environments. How does this feature actually translate to faster problem identification and resolution?
The rise of microservices has definitely introduced new challenges for asset management, as services can be created and modified rapidly in a distributed environment. I'm intrigued to see how real-time asset discovery tools are adapting to this dynamic landscape.
The ability to detect configuration drift automatically is a significant leap forward in maintaining system integrity, especially in fast-paced IT environments. I'm interested in the specific mechanisms used for change detection and how they effectively handle complex changes across multiple environments.
While the notion of optimized architectures to address latency issues is promising, I'm still concerned about the potential for latency in real-time asset discovery, especially in distributed environments. I'd want to understand the specific architectures and performance metrics used to mitigate these concerns.
Beyond just improving asset management, real-time discovery is now being touted as a way to enhance security posture by promptly identifying misconfigurations or unauthorized changes. This is a significant claim, and I need to see evidence of how this actually works in practice.
Finally, the claim of user-driven customization options is interesting, suggesting that real-time asset discovery is becoming more user-centric. I'm eager to understand how this customization works and whether it truly addresses the needs of diverse engineering teams.
In conclusion, the field of real-time asset discovery is undergoing rapid evolution, offering the potential for more comprehensive and responsive IT asset management in hybrid environments. However, as a researcher, I'm driven by a need for greater clarity and evidence-based analysis before embracing these claims wholeheartedly. It's critical to move beyond the hype and critically examine the technical details, real-world implementations, and performance metrics to truly assess the effectiveness and impact of these tools.
ServiceNow CMDB in 2024 Streamlining IT Infrastructure Management with Enhanced Cloud Integration - Advanced Visualization Tools Simplify Complex Infrastructure Mapping
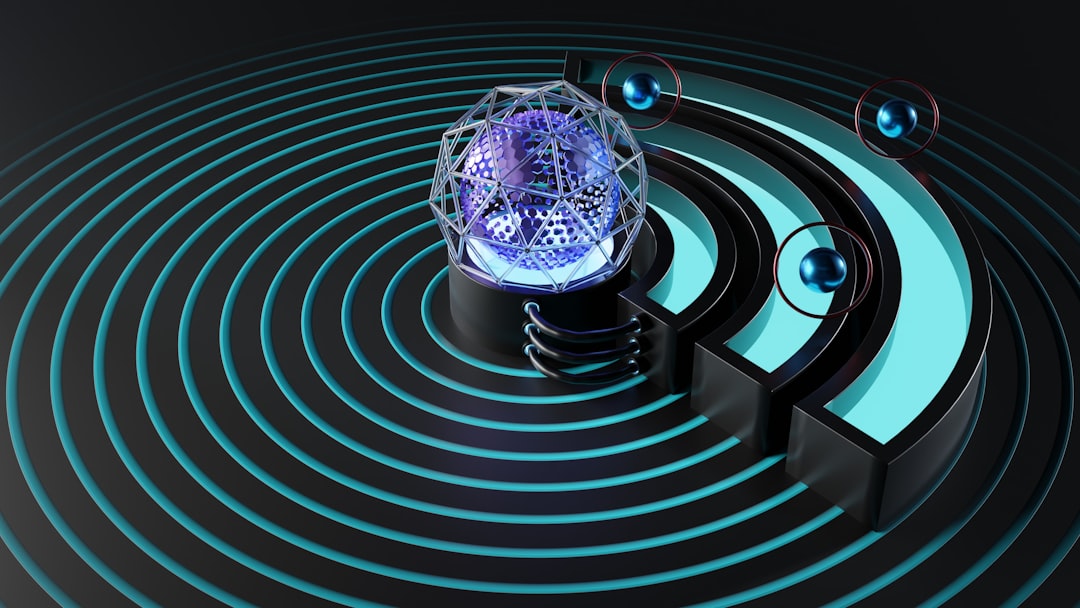
Advanced visualization tools within ServiceNow's Configuration Management Database (CMDB) are becoming more prominent in 2024. They promise to simplify the complex task of understanding intricate IT infrastructure relationships. These tools allow users to visualize these complex networks, transforming abstract data into user-friendly visual representations. This visual approach facilitates a better understanding of service maps, which helps organizations more effectively identify and diagnose problems, manage changes, and align IT operations with business goals. While the ability to drill down from high-level overviews into detailed configuration item data provides enhanced analytical capability, it's crucial to assess how these visualizations actually perform in the real world, especially when dealing with the complexities of hybrid environments. It's tempting to rely on the apparent simplicity of these visual tools, but they might not address the full scope of challenges modern IT infrastructure presents.
Advanced visualization tools hold great potential to make sense of the complexities within modern IT infrastructure. These tools can display complex relationships between different components in a clear and easily understood way, helping us understand dependencies between hardware, software, and cloud services. This can be a huge advantage, saving time and providing a more accurate view than manually created diagrams.
The promise of real-time updates is particularly intriguing. It means we can see changes in the IT landscape in seconds, compared to the hours or days it might take to update diagrams manually. This kind of agility could be a game-changer for responding to issues and identifying potential problems before they impact service delivery.
While the idea of having a constantly evolving visual representation of the infrastructure sounds good, I have some reservations. I'm curious to see how well these tools handle the dynamic nature of hybrid environments. Many of these systems are evolving rapidly, so accuracy is essential. It's one thing to visualize static relationships but can these tools keep up with the constant changes that are becoming the norm?
The integration of performance metrics, security incidents, and compliance status into these visualizations is also a promising development. This holistic approach could be very beneficial, but I'm wondering how effective these platforms are at integrating data from disparate sources. Will it actually result in a truly unified view, or will it just create a more complex patchwork of information?
These visualization tools may help organizations achieve more accurate configuration data, reducing the risk of human error, but how will they address the potential biases in data collection, especially as more complex data sets are integrated? And how can we ensure that data quality is maintained in real-time environments?
The use of interactive interfaces, customizable dashboards, and predictive analytics also seems promising. However, I'm interested in the user experience and how these features translate to practical improvements in collaboration and decision-making. It's important to remember that visualization tools are only as good as the data they use, so data quality and accuracy are essential.
In conclusion, the idea of utilizing advanced visualization tools to make sense of complex IT environments is an exciting one. The potential to gain real-time insights, reduce human error, and improve collaboration is huge. However, I need to see more concrete evidence before wholeheartedly embracing this technology. As a researcher, I want to know how well these tools handle dynamic environments, the nuances of multi-cloud integration, and the implications for data quality. I believe that rigorous testing and real-world case studies are essential to evaluate the true potential of these visualization tools.
ServiceNow CMDB in 2024 Streamlining IT Infrastructure Management with Enhanced Cloud Integration - Automated Compliance Tracking Meets Evolving Regulatory Demands
The growing complexity of regulatory landscapes makes automated compliance tracking a crucial component of ServiceNow in 2024. This automated system aims to free up time by minimizing manual tasks, letting organizations focus on strategic goals rather than tedious compliance checks. Real-time insights into compliance status are another key feature, allowing companies to identify and address potential issues before they escalate into costly audit findings. Additionally, ServiceNow's integration of regulatory change management enables quick and accurate updates on new or evolving regulations, ensuring compliance analysts can respond effectively. While automated compliance tools offer great promise, it's important to remain critical and aware of the complexities involved in their implementation.
The rise of automated compliance tracking systems has generated quite a buzz. There are predictions that organizations could see a significant cost reduction, up to 30%, as they move away from manual processes. It's all about using digital solutions to make things more efficient. It's exciting to think that advanced machine learning algorithms will be used to alert companies to potential regulatory violations in real-time. This kind of real-time monitoring could be a game-changer for risk management.
Regulatory technology, or RegTech as it's known, is becoming quite popular. Estimates suggest that it could generate over $250 billion in revenue annually by 2025. This indicates a huge market opportunity for businesses that jump on board early. The fast pace of regulatory changes, driven by the interconnectedness of cloud services, makes it crucial to have systems that can adapt quickly.
One study found that organizations with automated compliance tracking systems reported a 40% reduction in audit preparation time. That's a significant improvement in operational efficiency. The integration of compliance monitoring solutions with existing IT management systems is a key factor for success. Organizations that can achieve this integration might see an 80% improvement in compliance monitoring efficiency.
The increase in data privacy regulations, like GDPR and CCPA, has created a need for more advanced capabilities, and automated compliance solutions are rising to the challenge. They can now automate responses to compliance requirements, including privacy impact assessments.
It's surprising that many organizations still don't fully understand the scope of regulatory requirements. Automated systems could help bridge this knowledge gap by providing continuous updates and insights. The use of predictive analytics in compliance tracking could enhance risk management capabilities, potentially reducing the occurrence of compliance breaches by over 50%. It's a fascinating area of research and development.
We're also seeing collaborations between businesses and software innovators as the landscape of regulatory demands evolves. Organizations that embrace these partnerships are often at the forefront of compliance technology, giving them a competitive advantage in their markets.
Overall, it's a dynamic and evolving space. The potential of automated compliance tracking is intriguing, but we need to understand its real-world implications and the challenges it presents. As a researcher, I'm excited to see how this technology will continue to develop and its impact on organizations.
ServiceNow CMDB in 2024 Streamlining IT Infrastructure Management with Enhanced Cloud Integration - Integration with IoT Platforms Extends CMDB Reach to Edge Devices
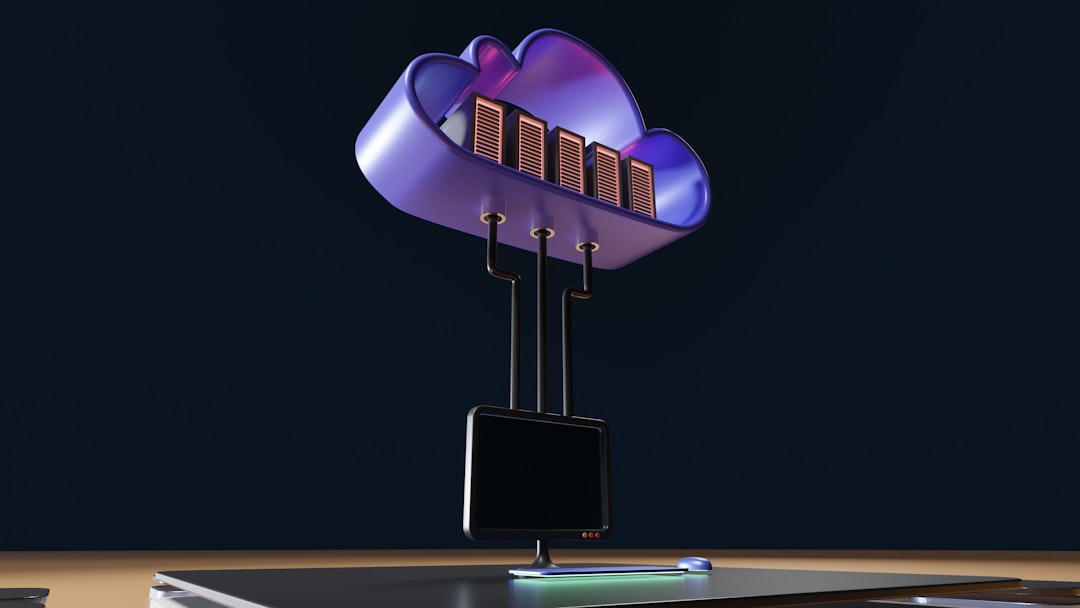
ServiceNow's CMDB is expanding its reach in 2024 to include the increasingly important realm of Internet of Things (IoT) devices. This integration seeks to improve the management of a growing segment of organizational IT infrastructure. By incorporating IoT devices into the existing CMDB framework, organizations can add new asset classes and relationships, enhancing the overall value of their asset management systems. This approach aims to streamline the secure decommissioning of old IoT devices and make it easier to prepare replacements, minimizing data risks. However, it remains to be seen if this expansion truly translates into better manageability and security across the diverse and complex world of IoT assets. The question remains: does this expansion truly address the unique challenges of managing these interconnected devices, or does it simply add another layer of complexity to an already challenging landscape?
Extending the CMDB's reach to include IoT devices is an exciting prospect, but there are significant challenges to consider. It's not just a matter of connecting more devices; it's about managing a complex web of interconnected systems.
Firstly, edge devices often use different communication protocols, making a unified approach to integration difficult. Adding to this, these devices generate a massive amount of data, raising questions about whether the CMDB can handle the volume without losing speed. Automated discovery tools can be useful, but they rely heavily on heuristics and algorithms that are prone to misidentification.
Secondly, the rapid deployment and modification of edge devices create a dynamic landscape that requires real-time synchronization for CMDB updates, which presents a real technical challenge. Furthermore, security becomes a crucial concern as these devices are often less secure, making them potential entry points for cyberattacks.
Thirdly, integrating edge devices complicates data governance, as organizations need to comply with regulations across a wide range of devices. This is further complicated by inconsistencies in data quality and formats, which can lead to inaccurate reporting.
Finally, while AI can be valuable for analyzing IoT data, it also presents potential risks. Biases within AI training datasets can lead to inaccurate predictions or missed anomalies. And then there's the challenge of cross-platform compatibility, as organizations often utilize various IoT platforms.
Overall, the integration of edge devices introduces significant complexity that needs to be addressed. While the promise of a more comprehensive view of the IT landscape is appealing, it's important to recognize the challenges involved. There are critical questions about data management, scalability, and security that need to be considered as we move towards a more interconnected world of IoT devices.
More Posts from :