Artificial Intelligence in Business Management Software A 2024 Reality Check
Artificial Intelligence in Business Management Software A 2024 Reality Check - AI adoption rates in US businesses remain lower than expected
While the potential of artificial intelligence (AI) to reshape businesses continues to be a major topic, its adoption within US companies hasn't reached the levels many predicted. A recent study found that only about two-fifths of large organizations are currently actively using AI, suggesting a slower-than-expected rollout across various sectors. Although some businesses that have already integrated AI are looking to scale up their efforts, the wider adoption picture appears uneven. AI integration appears to be more prevalent in a handful of major cities and amongst larger companies, indicating many businesses are still cautious about fully implementing AI. Looking ahead, businesses will need to figure out how to overcome this hurdle and accelerate their AI adoption to capture the projected gains in operational efficiency and competitive edge AI is expected to bring in the coming years.
While AI's potential to revolutionize business operations is widely acknowledged, its adoption rate in US businesses remains surprisingly low. Studies show that only around 40% of businesses, particularly those with over 1,000 employees, are actively utilizing AI, far less than many initially predicted. Even among the early adopters, a large number are primarily focusing on the exploratory phases of AI use, suggesting a lack of full-scale integration.
This slower-than-expected adoption isn't uniform across the business landscape. Smaller businesses, in particular, are significantly trailing behind larger enterprises, indicating that the challenges of implementing AI might be amplified for smaller operations. This discrepancy likely stems from factors like access to resources and the perceived level of risk associated with AI implementation for different sized businesses.
Another key hurdle is workforce readiness and management's approach to addressing the associated anxieties. A notable portion of employees express reservations about AI's potential impact on their jobs, which inevitably creates hurdles for management teams trying to implement AI. The tension surrounding job displacement needs careful consideration as AI integration progresses.
Furthermore, data security and privacy issues are top concerns for many organizations, hindering wider adoption. The apprehension is understandable, as companies are naturally cautious about potentially sensitive data being exposed. Addressing these valid security anxieties is crucial to encourage the uptake of AI in various sectors.
Interestingly, there's a marked difference between executive awareness and strategic planning around AI. While most leadership recognizes AI's benefits, relatively few have a concrete strategy for putting it into action. This disconnect between acknowledgment and implementation raises questions about the commitment and preparedness for fully utilizing AI's capabilities.
Specific industries, such as healthcare and education, have demonstrated a comparatively slower pace of AI adoption. This could be attributed to the presence of legacy systems and established practices that resist the integration of new technology. Such ingrained institutional patterns may make it difficult to adapt and implement innovative approaches to leveraging AI.
We also see variations in AI adoption rates across different regions. The West Coast, with its robust technology infrastructure and higher investment levels, is leading the way in AI adoption. The Midwest, on the other hand, has lagged behind, suggesting that geographical factors play a significant role in shaping the environment for AI adoption and could inform policy decisions.
The lack of skilled professionals and a widening gap in relevant workforce training is also hindering AI implementation. Many businesses are struggling to locate individuals with the necessary expertise to operate and optimize AI technologies effectively, leading to delays or stalls in AI initiatives.
In addition to workforce constraints, many businesses find that their current systems are not optimally compatible with new, cutting-edge AI technologies. The issue of technological interoperability emphasizes the need for greater foresight when planning for long-term technology adoption and integration.
While AI adoption is proving to be more gradual than anticipated, the benefits are clearly apparent in those organizations that have successfully implemented it. These companies report improvements in decision-making speed and operational efficiency. However, many struggle to concretely measure and quantify these benefits, hindering the development of comprehensive justifications for future investments in AI. This lack of tangible evidence will need to be addressed if AI is to become more universally adopted.
Artificial Intelligence in Business Management Software A 2024 Reality Check - Generative AI use doubles among organizations in one year
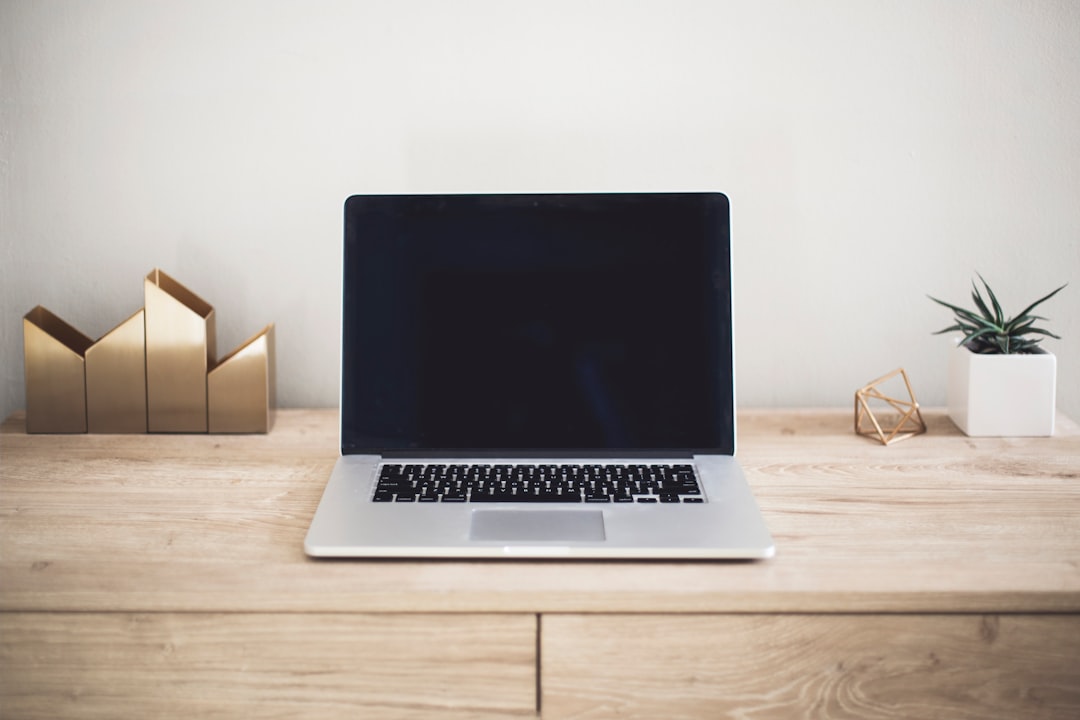
The landscape of business operations is being reshaped by generative AI, with its adoption experiencing a significant surge. Within a single year, the number of organizations regularly utilizing generative AI has nearly doubled, with over two-thirds now employing it in various aspects of their work. This growth is especially notable in areas like sales, where the rate of generative AI adoption has more than doubled. However, this quick uptake comes with growing concerns about potential drawbacks. The accuracy of generative AI outputs remains a significant worry, with a notable increase in businesses reporting concerns over inaccuracies. Alongside this, organizations are increasingly aware of the risks posed by generative AI, particularly when it comes to intellectual property. This growing focus on mitigating these risks signifies the complexities involved in implementing such transformative technologies. While the potential to streamline operations and change established business processes is undeniable, generative AI's integration into businesses is not occurring uniformly. Some businesses and industries have embraced its potential more readily than others, suggesting that navigating the unique challenges of integrating generative AI remains a key obstacle for many companies.
In just a year, the adoption of generative AI within organizations has nearly doubled, with a substantial 65% of respondents in a recent McKinsey survey reporting its regular use. This rapid increase suggests a growing recognition of the potential of generative AI across a wide range of business functions. The sales sector, in particular, saw a more than doubling of generative AI usage from the previous year, highlighting its increasing importance for driving business outcomes.
However, this surge in adoption is not without its challenges. Concerns about the accuracy of generative AI outputs have risen significantly, with 63% of respondents expressing such worries, up from 56% previously. This growing concern around potential inaccuracies is understandable given the inherent limitations of these tools in understanding and processing complex information. Further complicating matters are questions surrounding intellectual property rights and the potential for infringement when leveraging AI-generated content.
These risks are prompting organizations to focus on mitigating the potential downsides. A recent Capgemini report emphasizes how organizations are actively integrating generative AI, leading to significant shifts in how they operate. It's no longer a question of *if* organizations will adopt generative AI, but rather *how* they will navigate the inherent challenges.
A Deloitte study surveyed 1,982 business and technology leaders across a range of sectors and countries, confirming a global and widespread interest in exploring and deploying generative AI solutions. Gartner, known for its industry insights, forecasts that over 80% of enterprises will utilize generative AI technologies, models, or APIs by 2026. This is a staggering increase from the less than 5% in 2023, illustrating the momentum that generative AI has gained in such a short timeframe.
The speed at which organizations are considering and deploying generative AI, especially since its introduction to the wider public in late 2022 and early 2023, is notable. Organizations are looking at ways to maximize the value of generative AI by establishing reuse strategies for models and use cases. This desire for more efficient implementation suggests that the novelty phase is ending, and the focus is shifting to maximizing ROI. Major corporations are increasingly making public commitments to generative AI, reinforcing the perception that it is a critical aspect of future business operations. It appears the future of many businesses may be inextricably linked to their adoption and utilization of generative AI.
Artificial Intelligence in Business Management Software A 2024 Reality Check - Data foundations and governance become focal points for AI implementation
The successful implementation of AI within businesses is increasingly reliant on strong data foundations and governance. Companies are discovering that simply having AI tools isn't enough; they need well-managed data to fuel these tools effectively. This means addressing the many challenges associated with data management, such as ensuring the quality, security, and compliance of the data used for AI. Many organizations report difficulties in expanding their AI initiatives due to limitations in their existing data infrastructure. This emphasizes the importance of establishing solid data governance practices to reduce the inherent risks and unlock the full potential of AI. This concern is amplified in the age of generative AI, as the vast amounts of data and high-speed processing demands present unique challenges for data handling. Effectively managing data governance is proving to be crucial for firms looking to responsibly and effectively incorporate AI into their operations.
In the realm of AI implementation within business management software, we're seeing a growing realization that the foundations of data – how it's managed, governed, and accessed – are paramount. It's become increasingly clear that a strong data foundation is crucial for scaling AI efforts across a whole organization. In fact, the vast majority of organizations are grappling with this very challenge, facing difficulties in laying down the necessary groundwork for effective AI. This isn't entirely surprising, considering that AI's demands – processing enormous volumes of data at lightning speeds – necessitate a more strategic approach to data infrastructure than we've seen in the past.
One of the biggest challenges revolves around managing the complexities of data within today's hybrid cloud environments. Businesses need to grapple with issues of data access, ensuring the right people have the information they need for AI applications. Security remains a major worry, as organizations navigate the risks of handling and storing ever-increasing amounts of potentially sensitive information. And of course, the constantly evolving landscape of compliance regulations adds another layer of difficulty, particularly when handling personal information in various jurisdictions.
While we've seen a fairly widespread adoption of AI across various business units – around half of organizations have implemented AI in at least one area – the true potential of AI remains unrealized in many cases. Essentially, the organizations that are seeing the most benefits from AI are the ones that have a solid handle on their data governance. Ensuring the data used in AI initiatives is of high quality, readily accessible, and secure is a crucial element for success.
It's interesting to see that the increased focus on generative AI and large language models has amplified the importance of data quality and privacy in data governance. Many companies are realizing that poorly managed data sources – the infamous "data silos" that hinder data flow – can significantly undermine the effectiveness of AI systems. It's almost as if the very nature of AI, with its need for massive amounts of data, is forcing organizations to reconsider their historical approach to data management and find better solutions for integration. There's a clear message here: a well-defined and adaptive data strategy is critical to unlocking the full potential of AI within an organization and fostering responsible AI practices. It's not just about adopting AI, it's about having the foundational data infrastructure to support it, especially as we move toward more advanced forms of AI that require even more robust data foundations.
Artificial Intelligence in Business Management Software A 2024 Reality Check - Multimodal AI emerges as a significant trend in 2024
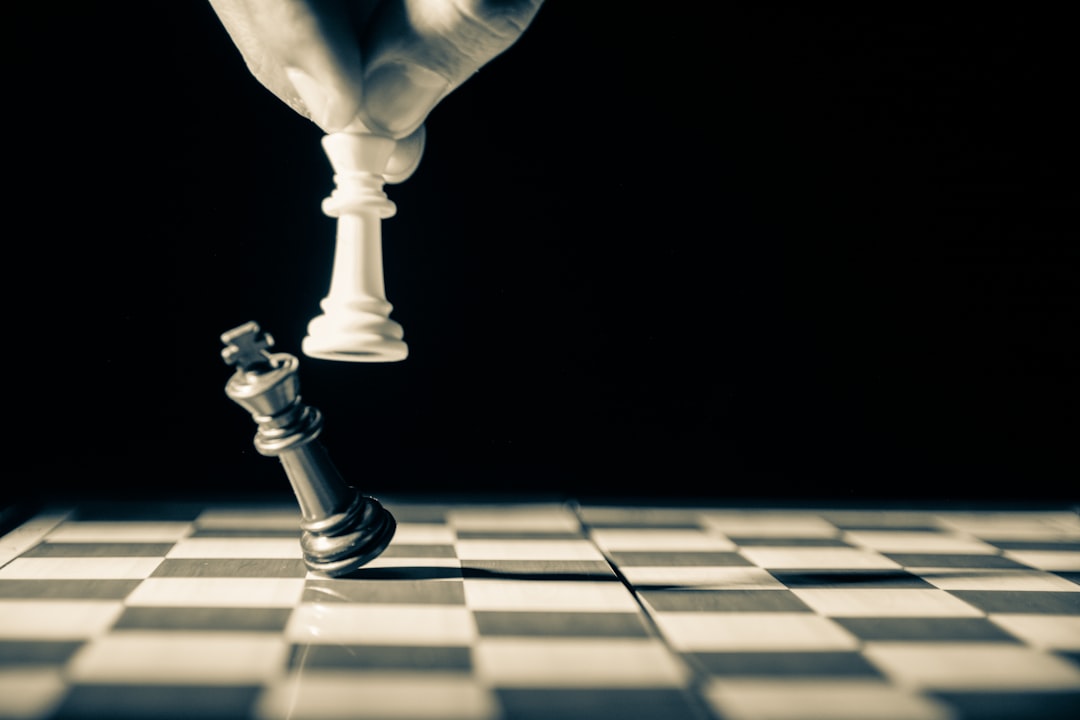
Multimodal AI has emerged as a noteworthy trend in 2024, characterized by its ability to process and integrate different forms of data, like text and images, and potentially audio in the future. This signifies a move towards AI that mirrors how humans communicate, enriching user experiences in areas like conversational and generative AI. Leading tech companies, like Microsoft, are spearheading development, displaying models that blend various data types. This development could also make AI tools more accessible and usable for everyday users, not just those with specialized tech backgrounds, as generative AI continues to evolve in its user-friendliness.
While the potential of multimodal AI to transform business practices is substantial, this advancement also brings to light important ethical considerations and regulatory concerns. It's critical that development and application of such advanced AI technologies are carefully considered, particularly as it becomes integrated into business management software. Areas like inventory, content creation, and recruitment are being impacted, with the potential for increased automation and data-driven decision-making across various industries. Multimodal AI showcases the broader trend of AI becoming increasingly integrated into our daily lives and workplaces. However, we must acknowledge the responsible development and usage of such powerful technologies. It's predicted that these changes will fundamentally alter the way humans interact with AI systems in the years to come, potentially driving higher levels of productivity and innovation.
Multimodal AI has emerged as a prominent trend in 2024, focusing on the integration of diverse data types—like text, images, and audio—into AI systems. The aim is to replicate human-like cognitive abilities, enabling a more holistic understanding of information and leading to potentially more insightful decision-making tools within businesses. This approach, however, comes with its own set of challenges.
Businesses that have begun using multimodal AI report improvements in operational efficiency, especially in areas like customer service. By understanding the context of customer interactions across various data points, they're achieving better outcomes and faster resolution of issues. The integration of multimodal AI into existing systems has proven tricky, however. It's difficult to smoothly merge various data sources, which often leads to bottlenecks and slows down the progress of developing AI products.
Early adopters in fields like e-commerce and healthcare have observed benefits in terms of faster product development cycles. By processing real-time feedback from diverse data streams, they're more readily responsive to market shifts and can adapt to consumer demand with more agility. But there are significant hurdles to overcome. One key issue is interpretability. As multimodal AI models grow in sophistication, understanding their internal decision processes can become incredibly difficult. This can lead to trust issues, particularly in regulated industries where transparency is vital.
The computational demands of multimodal AI are far greater than those of traditional AI. This places significant strain on businesses' IT infrastructure and can create a large financial burden. They have to carefully evaluate whether the potential gains outweigh the significant investment needed for hardware and cloud resources. Despite the buzz surrounding it, only a quarter of companies have implemented fully operational multimodal AI systems this year. Many are still in the experimental phase, indicating a gap between enthusiasm and actual deployment.
Security is a critical concern, as the integration of different data types exposes businesses to more attack vectors than traditional AI systems. This necessitates a more comprehensive security approach, going beyond piecemeal solutions to protect data holistically. Adding to the complexity are emerging regulations regarding data privacy and security. Companies implementing multimodal AI have to navigate a complex regulatory landscape and ensure their systems comply with varying jurisdictional requirements. This creates significant hurdles for timely implementation.
Beyond the technical challenges, multimodal AI is forcing discussions about broader ethical considerations. As AI models ingest a wider array of data types, the potential for bias across different data sources becomes more apparent. The need for responsible and ethical AI development is driving businesses to create clearer frameworks for ensuring accountability in their AI strategies. This is a necessary evolution in a field that is still grappling with the ethical ramifications of rapidly advancing technology.
Artificial Intelligence in Business Management Software A 2024 Reality Check - Real-time data processing enhances decision-making speed
The capacity to process data in real-time is increasingly vital for businesses aiming to make quicker decisions, particularly in dynamic business environments. AI's integration, including machine learning and natural language processing, empowers organizations to analyze massive datasets instantaneously. This allows businesses to react promptly to new opportunities and challenges. This not only equips management to handle high-stakes scenarios more effectively, but also encourages a mindset of adaptability and well-informed decisions across the organization. However, this dependence on real-time data processing isn't without its complications. Developing robust data governance and seamless integration strategies are necessary to maximize the benefits. As AI technologies continue to evolve, the capacity to make timely, informed decisions with real-time data is likely to become a crucial differentiator for business success.
The capability to process data in real-time is significantly impacting how businesses make decisions, especially in today's fast-paced environments. By leveraging AI technologies like machine learning and natural language processing, organizations can analyze vast quantities of information almost instantly. This shift from traditional, slower data analysis methods allows businesses to react much faster to changing market conditions, customer demands, and operational challenges. It's becoming clear that being able to react quickly is increasingly crucial for maintaining competitiveness.
While the potential for improvements in decision-making speed is considerable, it's not without challenges. Effectively integrating different data sources into a single, cohesive real-time system can be quite complex. Many businesses struggle with achieving seamless integration across various platforms and technologies, highlighting that the technology isn't a simple plug-and-play solution. There's a need to carefully consider the architecture of such systems and how various data sources will be combined.
Furthermore, the sheer volume of data generated and processed in real-time can strain existing IT infrastructure, and the costs associated with upgrading systems or adopting new technologies can be significant. Businesses need to evaluate if the projected gains outweigh the initial costs and ongoing maintenance of such sophisticated systems. It's not just about speed; it's about making sure that the infrastructure can support the demands of real-time processing effectively.
Despite these obstacles, the benefits of real-time data processing are compelling. Organizations employing these approaches often see improvements in decision accuracy, as well as a noticeable boost in employee engagement and productivity. It seems that when staff have access to current insights, they can make better informed choices, ultimately leading to improved performance and greater satisfaction with their work. This aspect is less often discussed in the context of real-time data, yet it appears to be a significant consequence of more agile data workflows.
In critical sectors like banking and healthcare, where quick decisions can be crucial, real-time processing has become essential. For instance, it's used to detect fraudulent transactions immediately and provide continuous patient monitoring, showcasing the importance of real-time information for safety-related decisions. As businesses look to further integrate AI into operations, real-time data processing will likely become increasingly important for fostering agility and responsiveness in various sectors. However, careful planning and a thoughtful approach to integrating multiple data sources are essential to unlocking the true potential of this evolving technology.
Artificial Intelligence in Business Management Software A 2024 Reality Check - Non-technical users gain access to AI experimentation tools
The availability of AI experimentation tools is expanding in 2024, particularly for those without a technical background. No-code and low-code platforms are making it easier for non-programmers to design and modify AI models. This wider access to AI is important as businesses are starting to see the benefits of a more diverse group of people involved in the development process. However, despite the ease of use, challenges remain. Businesses still need to wrestle with the concerns around data security and the accuracy of AI outputs. Also, it seems like many companies know they *should* use AI, but the actual application of these simpler tools into real-world decisions is lagging. It's a question of whether companies can effectively utilize these user-friendly AI tools to drive real improvements in their businesses.
It's fascinating how the AI landscape is evolving towards greater inclusivity. We're seeing a surge in AI experimentation tools specifically designed for individuals without a coding background. This shift towards user-friendly interfaces empowers business analysts, marketers, and others to directly engage with AI without needing extensive programming skills. They can now build rudimentary AI models with intuitive tools, often through drag-and-drop interfaces. This has the potential to accelerate the product development process, allowing for faster iteration and feedback loops.
Furthermore, the accessibility of pre-trained AI models is making it significantly easier to deploy AI solutions for various business problems. These pre-built models can be fine-tuned for specific needs with minimal technical intervention. This has the knock-on effect of fostering a more collaborative environment. Business units can now work more seamlessly with data science teams, leading to better product development and improved marketing strategies informed by data.
It's not just about technical accessibility, though. The ease of use is driving a culture shift towards experimentation. Employees without a traditional data science background are more likely to explore AI's possibilities, generating new ideas and driving innovation within their departments. This increased experimentation is potentially leading to a greater understanding of data analytics across organizations. As non-technical users dive into this field, they often gain a better grasp of data principles, making for a more data-literate workforce, crucial for data-driven decision-making.
The user-friendliness of these tools also extends to results interpretation. Platforms are developing features that communicate AI outputs in a readily understandable way, eliminating the need for deep technical expertise. This enhances the overall decision-making process, making it more inclusive and democratizing the benefits of AI.
While initially the need for specialized AI personnel may have been a constraint, the current trend allows businesses to reduce their dependence on external AI experts. This can lead to significant cost savings over time, especially for smaller organizations. By empowering more individuals within an organization, AI adoption barriers are also being lowered. The fear of complexity, which can often hinder AI implementation, is reduced as more people understand how these tools operate.
The continuous interaction with AI through these user-friendly platforms is also fostering the development of new skillsets. Users are naturally exposed to basic machine learning and data science principles, expanding their professional capabilities beyond their traditional domains. It's a positive trend that creates a more versatile and AI-ready workforce. However, it will be interesting to see how this trend continues to evolve as AI tools become even more advanced, and what impact it has on the data science job market in the years to come.
More Posts from :