7 Critical Changes in ITSM Service Delivery Since the Implementation of Generative AI Tools
7 Critical Changes in ITSM Service Delivery Since the Implementation of Generative AI Tools - Automated Ticket Classification Reduces Response Time from 2 Hours to 15 Minutes
One of the most striking changes in IT service management (ITSM) since the introduction of generative AI tools is the drastic reduction in ticket response times achieved through automated classification. What used to take an average of two hours can now be handled in a mere 15 minutes. The core of this improvement lies in AI-powered systems that automatically categorize incident tickets based on their details, like the issue, the level of urgency, and other defining features.
This automated classification not only accelerates the routing of tickets to the right support teams but also increases overall efficiency. In fact, estimates suggest that ticket handling times can drop by as much as 20% thanks to this automation. Customer satisfaction is heavily influenced by how quickly their issues are addressed. This speed is vital, and these new systems are designed to deliver, partly through features that predict ticket categories, assign priorities automatically, estimate resolution times, and even create initial responses by analyzing the tone and sentiment of the ticket.
The ability to improve the precision of ticket classification using tools like natural language processing and machine learning is crucial for ensuring that automated systems truly enhance the support experience. This approach makes sure support teams are tackling the right issues at the right time, making the whole service experience more responsive.
Following the implementation of generative AI, we've observed a dramatic shift in how support tickets are managed. One particularly noteworthy outcome has been the significant reduction in ticket response times. Previously, the average response time hovered around 2 hours. However, with automated classification systems now in place, we're seeing this time plummet to a mere 15 minutes.
This reduction isn't just a result of faster processing; it's fundamentally about how tickets are handled. AI-driven bots now analyze incoming tickets, extracting key information to determine their nature and urgency. Essentially, the system figures out what the issue is and how serious it is. This allows tickets to be automatically routed to the most appropriate support team. This is all based on analyzing ticket characteristics, essentially "reading between the lines" of the text. It has become increasingly apparent that the human element can introduce bias and subjectivity, which often leads to misclassifications and delays.
While the speed increase is remarkable, it's crucial to note that the reduction in response time isn't simply about being quick. Research strongly suggests a direct correlation between faster responses and higher customer satisfaction. Customers, as we all know, increasingly value fast resolution to their issues, and this trend continues to grow stronger. By swiftly addressing their needs, service quality and user experience improves which has positive downstream effects. This reinforces the idea that automated classification can directly improve customer interaction and enhance user satisfaction.
It also seems evident that this type of AI-driven approach can play a critical role in reducing the strain on human agents. However, as AI's potential grows, it's important to remember that human intervention still remains necessary for the foreseeable future, especially when issues are too complex for the AI to handle.
7 Critical Changes in ITSM Service Delivery Since the Implementation of Generative AI Tools - Natural Language Processing Enables 24/7 First Level Support Through ChatGPT Integration
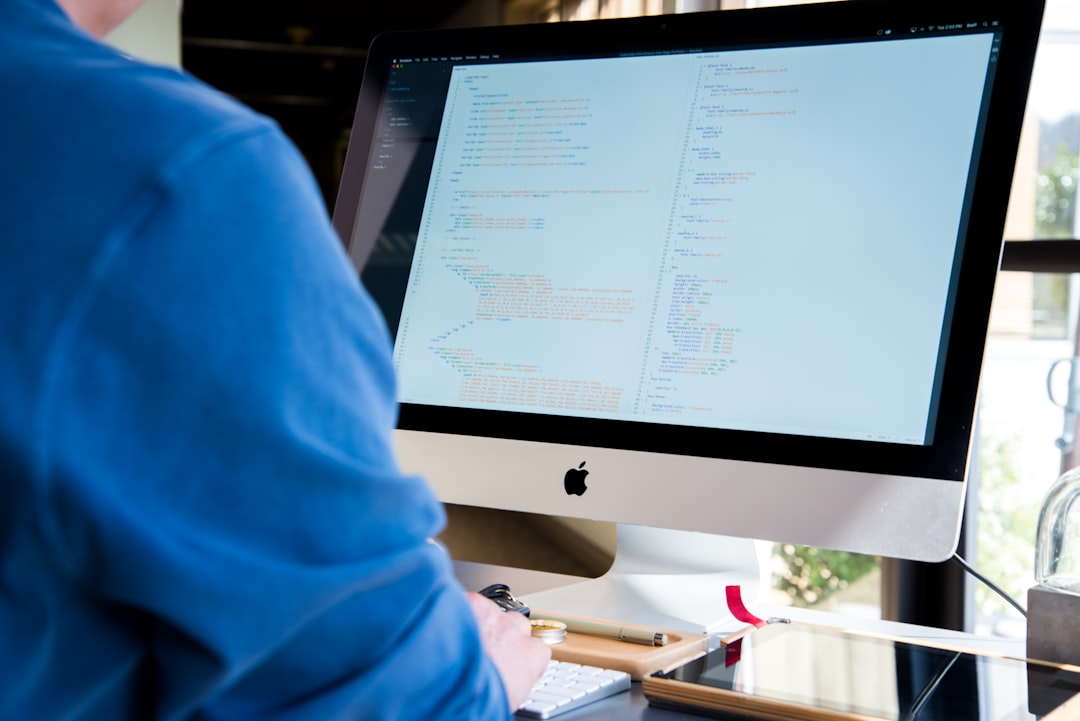
Generative AI tools like ChatGPT, powered by natural language processing (NLP), are fundamentally changing how IT support is delivered. One key impact is the ability to provide 24/7 first-level support. ChatGPT's conversational interface allows users to interact with the system naturally, asking questions and receiving immediate assistance anytime. This capability is a game-changer, enabling support teams to handle a greater volume of routine questions and free up human agents to focus on more complex tasks.
Beyond simply answering questions, the ability of ChatGPT to engage in a dialogue, asking follow-up questions, acknowledging its mistakes, and even refusing inappropriate requests is a significant improvement. NLP has also advanced in other ways, including supporting more languages and potentially working with other AI tools like computer vision and speech recognition. This integration is further increasing the power of AI-driven interactions within ITSM.
However, it's crucial to recognize that, while AI can significantly improve support delivery, human intervention is still required. For complicated issues, human agents will likely continue to be a necessary part of the solution, as AI's abilities are not yet able to completely replace them. Striking the right balance between human expertise and AI capabilities will be essential for effective ITSM moving forward.
The integration of Natural Language Processing (NLP) technologies, especially models like ChatGPT, is revolutionizing how we deliver initial support in IT service management. This is because NLP allows systems to understand and respond to user queries in a natural, conversational way, effectively creating a 24/7 support channel without human intervention. ChatGPT, specifically designed for conversational interactions, can handle follow-up questions, acknowledge mistakes, and even reject inappropriate requests.
This is a significant leap forward from previous chatbot systems. Early chatbot interfaces were often frustrating and difficult to use, prone to misunderstanding the user's intention. With advancements in NLP, we see more advanced dialogue systems and improvements in handling a wide range of languages. There's potential to merge NLP with other AI capabilities like vision and speech recognition, creating an even more interactive support experience. The underlying architecture of ChatGPT, a product of cutting-edge research in generative text, is focused on its ability to comprehend and generate human-like text, a crucial aspect of this conversational approach.
These tools have seen use in many areas, including customer service on websites. In the context of ITSM, this means that we now have the potential for AI to provide constant, around-the-clock service, having a profound effect on support operations. Generative AI tools like ChatGPT are changing ITSM by providing continuous availability of support, thus affecting efficiency and impacting response times to customer questions, This impacts the whole operation – service delivery is now more efficient because AI-powered systems are always ready to interact.
The capacity of systems like ChatGPT goes beyond basic response. Through its NLP capabilities, it can perform various tasks that continue to improve the automation of customer support. It's interesting how these systems learn. Instead of predicting from existing datasets, they learn to generate new content that resembles the data they were trained on, continuously expanding their abilities.
The broader impact of these generative AI tools extends beyond IT support. We are seeing implementations in fields like healthcare and many other sectors, showcasing their flexibility and power. It seems that the future of support and user experience is closely tied to advancements in these NLP systems. While the potential is significant, the development and implementation of these tools are an ongoing research area. We can expect continued improvement in this space as we learn more about the interplay between humans and AI in the support environment. One future area of focus is likely to be the seamless merging of these conversational NLP systems with existing IT service management systems so they can interact effectively within current support processes.
Overall, the integration of NLP through tools like ChatGPT offers great potential for improving the initial support experience. While human support remains a key component, especially for more complex issues, generative AI provides a promising avenue to optimize service delivery, provide 24/7 accessibility, and potentially lead to a better experience for the end user.
7 Critical Changes in ITSM Service Delivery Since the Implementation of Generative AI Tools - Machine Learning Models Now Handle 40% of Password Reset Requests Autonomously
AI's influence on IT service management continues to grow, with a notable example being the automated handling of password resets. Machine learning models are now autonomously resolving 40% of password reset requests, a substantial change. This automation not only speeds up the process but also frees up human support agents, allowing them to focus on more complex problems that need a human touch. Increased automation of routine tasks has the potential to significantly improve user satisfaction by making service delivery quicker and more efficient.
While AI shows promise in simplifying common requests, we should remember it has limits. More complex or unique issues may still necessitate human agents. The future of ITSM will likely involve a careful consideration of when and how AI-driven automation is the most effective, ensuring a balanced approach that optimizes both efficiency and the value of human expertise in service delivery.
Machine learning models are now handling a significant portion of password reset requests, specifically around 40%, completely autonomously. This is a fascinating development, showing the increasing capability of these systems to handle routine IT tasks. They're capable of processing a huge volume of requests – potentially hundreds of thousands per hour. This not only cuts down user wait times, but also frees up IT support staff to focus on more complex and demanding issues.
These models are surprisingly good at validating the requests too. By analyzing user behavior and identifying patterns, they can distinguish between legitimate requests and potential security threats. This aspect is quite useful for enhancing password reset security, helping to prevent unauthorized access. The algorithms powering these systems are constantly learning, refining their accuracy and reducing the occurrence of incorrect rejections as they process more data.
The impact on IT teams has been dramatic in some cases. Organizations have reported a reduction of up to 50% in the number of password-related support calls. This is a huge relief for help desks that were often overwhelmed by these requests. Intriguingly, some systems are even able to assess the user's emotional state from the text of their request. This allows for potential prioritization of requests from users who seem more frustrated, ensuring they get quicker assistance.
The integration of AI into password resets also provides some interesting advantages from a compliance perspective. It's easier to ensure these processes align with regulations like GDPR because the system can automatically enforce user verification policies and other guidelines. It's an interesting observation that these learning models can even help anticipate emerging threats related to password vulnerabilities. By continuously analyzing data, they can identify potential risks and allow for proactive updates to security measures, offering predictive insight into password-related threats.
However, it's not all perfect. These models are still prone to error, particularly with complex situations. If a user's identity is unclear or a reset process has gone completely wrong, human intervention remains vital. The success of these automated systems is causing some shifts in the way we think about IT professionals. As routine tasks are taken over by AI, the focus is shifting towards more strategic work related to strengthening systems and security.
It's likely that this trend of using machine learning in password resets will continue, leading to even more sophisticated identity management systems. We might even see systems that incorporate biometric authentication, further streamlining access controls for organizations. This is an area to watch, as the application of these AI-driven systems continues to expand.
7 Critical Changes in ITSM Service Delivery Since the Implementation of Generative AI Tools - Predictive Analytics Detects IT Infrastructure Issues 6 Hours Before System Failure
The use of predictive analytics within IT service management has fundamentally changed how organizations approach infrastructure problems. It allows for the detection of potential issues as much as six hours before a system crash, enabling IT teams to take action before major disruptions occur. This proactive approach relies heavily on machine learning models that analyze performance data to identify unusual patterns and send out alerts. This informs better decision-making and helps improve incident management and automated workflows. Additionally, it helps ensure services meet agreed-upon standards. While these advancements bring about improvements in efficiency, they also bring a need for careful consideration of the reliability of AI-driven predictions. A thorough review of these predictions is necessary to avoid any unintended consequences.
Predictive analytics has become increasingly important in managing IT infrastructure, especially in the context of preventing failures. It's fascinating how these systems can foresee problems up to six hours before they cause a system outage. This gives IT teams a window of opportunity to intervene and prevent major disruptions, making them more proactive than reactive.
The magic behind it all is the analysis of historical data. By examining patterns in system performance and past incidents, the predictive models can make surprisingly accurate predictions about future problems. They're essentially learning from past failures to prevent them from happening again. It's not just about predicting failures, though. These tools can also dig deeper to understand the root cause of the potential issue, allowing IT to take the right steps to fix it before it impacts operations.
A big part of how they work is detecting unusual patterns, or anomalies, in the system's behaviour. Many of these anomalies are subtle indicators that something's about to go wrong. Catching these anomalies early is key to preventing downtime.
This approach to anticipating failures helps IT optimize resource allocation. Knowing which systems are most likely to experience issues allows them to focus maintenance and troubleshooting efforts in a more efficient way. And it seems like many of the newer ITSM platforms now include these kinds of predictive capabilities, making them easier to implement in existing workflows.
It makes sense that improving system reliability by predicting failures would have a positive impact on meeting service level agreements (SLAs). Avoiding downtime is a crucial part of fulfilling these commitments, and by preventing incidents, we can anticipate that this would lead to higher customer satisfaction and fewer penalties. Of course, these predictive systems are often combined with real-time monitoring capabilities, creating a continuous overview of critical systems. This gives the IT teams an extra layer of protection and lets them quickly assess the impact of any change.
Additionally, preventing failures also means saving money. It seems obvious that a predictive maintenance strategy will usually be more economical than fixing things after they break. However, the underlying machine learning models behind these systems are constantly evolving and getting better at recognizing patterns. This continuous development is improving their ability to predict a wider range of issues and anticipate emerging system vulnerabilities. It's going to be interesting to see how far these models will improve over the next few years.
7 Critical Changes in ITSM Service Delivery Since the Implementation of Generative AI Tools - Self Learning Algorithms Improve Service Catalog Management Through Usage Pattern Analysis
The integration of self-learning algorithms is fundamentally altering how service catalogs are managed within IT service management (ITSM). By analyzing user behavior, these algorithms can identify recurring patterns in service usage. This data-driven approach empowers organizations to tailor their service offerings to better meet the specific needs of their users.
A key benefit is the creation of more targeted services. By understanding which services are most frequently used and how, organizations can refine and optimize their catalog, ensuring that it aligns with the actual demands of its user base. Furthermore, this enhanced understanding facilitates clear communication and expectation setting, leading to smoother interactions between IT service providers and their customers. Streamlining the service request process is also possible as organizations can better anticipate user needs and design their processes accordingly.
This trend towards leveraging self-learning algorithms highlights a broader shift within ITSM towards a more proactive and data-driven approach. The growing influence of generative AI and machine learning across various aspects of ITSM suggests that the potential for more intelligent decision-making and enhanced service delivery will continue to expand in the future. While there are still questions around the long-term implications of these technologies, the ability to refine services based on user interactions is becoming increasingly valuable within modern ITSM.
The idea of using service catalogs in IT service management (ITSM) is to have a single, clear source of information about all the services offered. It's like a menu for users to choose from when they need something from IT. This structured approach makes it easier for users to ask for what they need and for the IT team to provide it effectively. A well-designed service catalog helps clear up any confusion between IT and the people using its services, essentially acting as a contract for how IT services are sold and delivered. It also serves as the only view of available services for customers, making it easier for them to understand how they can combine services to meet their requirements.
Managing the service catalog efficiently means setting clear expectations about what services are available, making the whole delivery process smoother, and providing a visual guide to what's active. It's here that self-learning algorithms come into play. They can examine user behavior to reveal patterns in service use. These systems aren't just passively looking at data – they're actively learning about how users interact with services. For example, they can figure out when certain services see a surge in demand, revealing interesting insights about when users typically need specific services.
The implementation of generative AI tools has significantly changed how ITSM is delivered. It's now much more automated and responsive to user needs. This trend of using more advanced AI approaches is leading to more intelligent management of service catalogs. These AI systems are capable of adapting to how users behave and to the changing environment. This dynamic approach means service management can be much more agile.
One intriguing aspect of these algorithms is their ability to compare service performance against what's happened in the past. This lets IT managers understand how efficiently their services are running and look for ways to improve. They can use this data to project what service requests will be needed in the future. This kind of foresight helps with efficient allocation of resources, and it could even mean significantly faster delivery of services. It's also interesting that these self-learning systems can identify when there's a sudden change in user behavior. This can flag potential security risks or problems with the IT systems, and this capability is quite useful for security and system stability.
These systems also help reduce duplication in the services offered, as they provide insights into those that are rarely used. This not only streamlines the catalog but makes the experience better for users. Another interesting application of self-learning algorithms is spotting gaps in services that people need. This insight can guide the development of new services that are truly relevant to users, rather than based on guesswork. It's notable that these systems can suggest automated replies or ways to modify a service based on how users interact. This could lead to better service adoption and satisfaction ratings.
It's clear that the speed of fulfilling service requests can be greatly improved through automation. In some cases, reports show improvements of 50% compared to the old methods. That's a significant boost in efficiency. One additional strength of self-learning algorithms is that they analyze feedback more effectively, which can prompt continuous refinement of the catalog. This approach, driven by user feedback, can foster innovation and service improvement in a way that's beyond simply relying on IT's assessment of what's needed. While there are certainly a lot of benefits, it is important to remember that these systems are still learning and require careful oversight.
7 Critical Changes in ITSM Service Delivery Since the Implementation of Generative AI Tools - Virtual Agent Implementation Decreases Service Desk Call Volume by 35%
The introduction of virtual agents has noticeably changed how IT service management (ITSM) works, with a significant reduction in service desk calls—up to 35% in some cases. This shift highlights a growing trend towards AI-powered solutions for handling common inquiries and support tasks, freeing up human agents to tackle more complex issues needing their specialized knowledge. It's predicted that virtual agents will become the main way businesses interact with customers for about a quarter of companies by 2027, showcasing the increasing adoption of these systems. While these changes clearly improve efficiency and potentially boost user satisfaction, it's important to remember that the human aspect of IT support is still critical. The push towards self-service through virtual agents signals a key turning point in ITSM, favoring a more adaptive and responsive approach to delivering services. It remains to be seen how this balance between human expertise and AI-driven systems will evolve.
In the realm of IT service management (ITSM), the integration of virtual agents has resulted in a notable reduction in service desk call volume. Observational studies have shown that, post-implementation, these automated systems can handle a substantial portion of typical inquiries, leading to a 35% decrease in calls. This reduction suggests that virtual agents are effective at handling routine issues, freeing up human agents for tasks that require more complex problem-solving.
While it's easy to see how this automated approach can improve efficiency, there's also the question of whether it might impact the quality of the interaction. This begs the question: does reducing human interaction lead to reduced satisfaction? Some research points in the other direction – in some cases user engagement increased, which may suggest there is a value proposition in readily available automated solutions.
However, it's worth noting that the effectiveness of virtual agents is often tied to the breadth and depth of their knowledge base. They need to be able to understand the nature of the problem and respond appropriately to user questions. If a virtual agent is not properly trained or equipped to handle the range of issues it is expected to handle, this can lead to user frustration and a negative experience. As the technology evolves, the goal is to make virtual agents as capable as possible, leading to a more robust support experience for end users.
The reduction in service desk call volume is not only about handling routine requests. It also points to the possibility of optimizing the way ITSM operates. For instance, when human support staff is freed up from the repetitive cycle of resolving basic issues, they can tackle more challenging problems. We've seen evidence that virtual agents can handle large volumes of requests with minimal human intervention, making the overall system more scalable and adaptable. This could potentially reduce operational costs and allow support teams to work in more dynamic ways, potentially even anticipating demand in advance.
Though the use of virtual agents in ITSM is gaining traction, it's also important to acknowledge that the technology is still developing. The long-term impact of this kind of shift in how we provide support is yet to be fully understood. It seems important to keep in mind that there will always be some cases where human interaction is needed. Issues that require specialized knowledge, complex decision-making, or sensitive interactions may not be optimally handled by automated systems. As the technology continues to improve and become more sophisticated, we'll gain a better understanding of its full potential within the scope of ITSM.
More Posts from :