The Rise of AI-Driven Predictive Maintenance in Business IT Solutions A 2024 Perspective
The Rise of AI-Driven Predictive Maintenance in Business IT Solutions A 2024 Perspective - AI revolutionizes IT infrastructure monitoring in 2024
2024 is witnessing a profound shift in how businesses manage their IT infrastructure, thanks to the increasing adoption of AI. Instead of reacting to problems after they occur, IT monitoring is becoming increasingly predictive. This change is driven by AI's ability to analyze massive amounts of sensor data in real-time, identifying patterns and anomalies that signal potential issues. Through sophisticated machine learning models, organizations can now anticipate equipment failures and optimize resource allocation, reducing downtime and overall costs. This proactive approach contrasts with older methods, leading to more efficient maintenance and a smoother operational flow.
However, the journey towards fully embracing AI-powered infrastructure management is gradual. While the benefits are clear, many businesses still approach AI with measured steps. This is partly due to the evolving nature of AI technologies themselves, with new tools and approaches emerging constantly. But this cautious progress also reflects the need for organizations to carefully tailor these powerful new tools to their unique infrastructure and operational needs. The landscape is changing rapidly, and we are seeing the birth of new solutions and service offerings specifically designed to support this shift towards AI-driven IT management.
The way IT infrastructure is monitored has fundamentally changed in 2024, thanks to the integration of AI. These systems are capable of sifting through massive volumes of data in real-time, allowing for the identification of infrastructure hiccups much faster than older approaches. Some researchers claim a 50% improvement in issue resolution time.
It's not just about predicting equipment failures anymore. AI's ability to analyze network performance is enabling IT teams to anticipate and mitigate bottlenecks before they impact service delivery. This proactive stance is a significant change from the reactive approaches of the past.
Interestingly, there's a consensus among those working in this field that AI-powered monitoring is significantly reducing downtime, with reports suggesting an average reduction of around 30%. This, in turn, boosts operational efficiency and cuts down on the costly consequences of outages.
Furthermore, the use of AI has refined the process of allocating resources. The systems leverage historical data and current demands to optimize workloads, potentially resulting in a more efficient use of infrastructure. These systems often have self-learning capabilities that enable them to continuously refine their predictions, adapting to new data and shifting circumstances without needing constant human intervention.
Beyond just resource allocation and maintenance, some AI-driven monitoring tools are even providing insights into user behavior. This information can guide IT departments in understanding evolving user demands and responding with necessary infrastructure modifications. However, this area is relatively new and raises questions about privacy and the potential for bias in how AI interprets user actions.
The accuracy of these tools in detecting anomalies is also quite impressive, often exceeding 95%. The improved accuracy helps filter out typical fluctuations and noise, minimizing the disruptive false alarms that were a common problem with earlier monitoring systems.
While the benefits of AI-driven maintenance are being observed, the transition is not without its costs. Several studies suggest that companies using AI to manage maintenance see up to a 25% decrease in overall maintenance costs. This change in approach does require substantial upfront investment for both hardware and specialized expertise to design, implement, and operate these AI-enhanced systems.
Beyond operational efficiencies, AI monitoring systems are being applied to enhance cybersecurity. They are now capable of detecting anomalies that could suggest a security threat, offering an added layer of defense. This is a promising area, though it is still being developed, and the real-world efficacy of these AI-based security measures remains to be seen.
The introduction of AI-based decision-making in infrastructure management has also reduced the burden on human IT staff. Repetitive and routine tasks are now automated, freeing up engineers to tackle higher-level strategic planning and focus on more innovative solutions. However, this automation also creates new challenges as it necessitates a workforce shift to focus on higher-order skills and understanding how to supervise and validate the AI systems.
The Rise of AI-Driven Predictive Maintenance in Business IT Solutions A 2024 Perspective - Machine learning algorithms detect subtle system anomalies
Machine learning algorithms are becoming adept at uncovering subtle system anomalies that might be missed by traditional monitoring approaches. These algorithms sift through vast amounts of operational data, identifying intricate patterns and deviations that can signal potential problems. This capability is significantly improving predictive maintenance practices across different sectors. By recognizing these subtle signs of trouble, organizations can intervene more promptly, leading to reduced downtime and optimized resource use. Not only does this approach improve efficiency, but it also reshapes the way organizations manage their infrastructure, driving a new era of proactive maintenance.
However, the integration of these powerful tools isn't without its complexities. The quality of the data fed into these systems is paramount, and achieving reliable results often requires specialized knowledge and expertise. Businesses need to cautiously navigate these challenges to fully leverage the benefits of AI-driven anomaly detection and fully realize the potential of these technologies in maintaining their IT infrastructure. The ongoing evolution of AI promises further improvements, but careful planning and implementation are crucial for achieving successful outcomes.
Machine learning algorithms are increasingly adept at detecting subtle system anomalies by examining granular details within operational data. Instead of just looking at overall system health, they can pinpoint deviations in individual devices or components, allowing IT teams to identify failing equipment before problems escalate. This ability to process massive datasets reveals irregularities that might escape human notice, leading to a deeper understanding of system behavior and more proactive maintenance strategies.
These algorithms can achieve remarkably fast anomaly detection, often within milliseconds, which is critical for systems needing real-time monitoring to prevent disruptions. Interestingly, the field leverages a mix of supervised and unsupervised machine learning approaches. Supervised learning relies on labeled data to learn patterns, while unsupervised learning can detect anomalies without prior training, allowing for greater flexibility in finding unexpected behavior. However, this flexibility comes with its own set of challenges.
Recent research shows that these algorithms are getting better at filtering out false positives—those irritating and ultimately unhelpful alerts that flood IT teams. By incorporating contextual data, such as operational conditions and historical trends, they can reduce unnecessary alerts by up to 40%. However, as we use increasingly complex neural networks to build our models, we face a new challenge: understanding how these models arrive at their conclusions. It's become vital for engineers to trust and understand the decision-making processes of these increasingly sophisticated systems.
The ability of these systems to adapt is certainly an advantage, as they can adjust to changing data patterns. However, there's also a risk of overfitting, where models become too focused on recent data and lose the ability to accurately predict future anomalies. The rise of the Internet of Things (IoT) and a plethora of new sensors increases the amount of data available to the algorithms, boosting detection capabilities. But this influx of data also poses new challenges in data management, model training, and evaluation.
Further, the capacity of these algorithms to link diverse data sources, such as network metrics, temperature readings, or even user interactions, enables a more holistic view of system health. This offers a more comprehensive picture for maintenance strategies, but it also makes the analysis considerably more complex. The potential financial benefits of using machine learning for anomaly detection can be significant. Organizations often recoup their investment within a year or two due to reduced downtime and improved maintenance procedures, suggesting that early adoption of this technology can provide a strategic advantage. However, like any new technology, it is important to be mindful of potential problems that might arise in this evolving field.
The Rise of AI-Driven Predictive Maintenance in Business IT Solutions A 2024 Perspective - Predictive analytics reduce unplanned downtime by 35%
Predictive analytics are showing impressive results in minimizing unplanned downtime, with some organizations reporting a 35% reduction. These tools use sophisticated algorithms to anticipate potential equipment failures before they impact operations. This proactive approach to maintenance allows for timely interventions, preventing costly outages and enhancing the overall efficiency of operations. By identifying and addressing issues early, the lifespan of vital equipment is extended, leading to a reduction in overall maintenance costs. As businesses increasingly adopt AI-driven predictive maintenance, the accuracy and sophistication of these analytics are expected to improve. However, realizing the full benefits of these systems is not without its challenges. Implementation requires significant investments in both hardware and expertise, demanding careful planning and adaptation to achieve the optimal outcomes.
Studies have shown that using predictive analytics can significantly reduce unplanned downtime, with some reporting a reduction of up to 35%. This is a compelling figure, suggesting a notable improvement in operational reliability. It makes sense, as these systems are essentially forecasting when something might go wrong, allowing for proactive maintenance before a failure occurs. The ability to pinpoint the likely time of failure is also getting better; some systems can even predict the remaining useful life (RUL) of a piece of equipment with surprising precision, often within just a few hours.
However, achieving this level of accuracy requires careful consideration of the data used to train the algorithms. We need to think critically about the types of data we're collecting and how we are modeling these systems. It's not just about looking at internal equipment data – some models include external factors like weather or operational load, which can further enhance prediction reliability. There's still an ongoing need for researchers and engineers to refine these models and make them more robust in different environments and with different types of equipment.
While these improvements are impressive, it's crucial to recognize that a significant portion of downtime is potentially preventable. It's estimated that up to 70% of unplanned downtime stems from failures that could have been predicted and avoided. This emphasizes the potential impact of predictive maintenance strategies and further underscores the importance of ongoing research and development in this field. We need to consider these successes in the context of the potential that still exists. It's not a finished product; it's an ongoing pursuit of better prediction and optimization.
The Rise of AI-Driven Predictive Maintenance in Business IT Solutions A 2024 Perspective - Cloud-based AI platforms streamline maintenance workflows
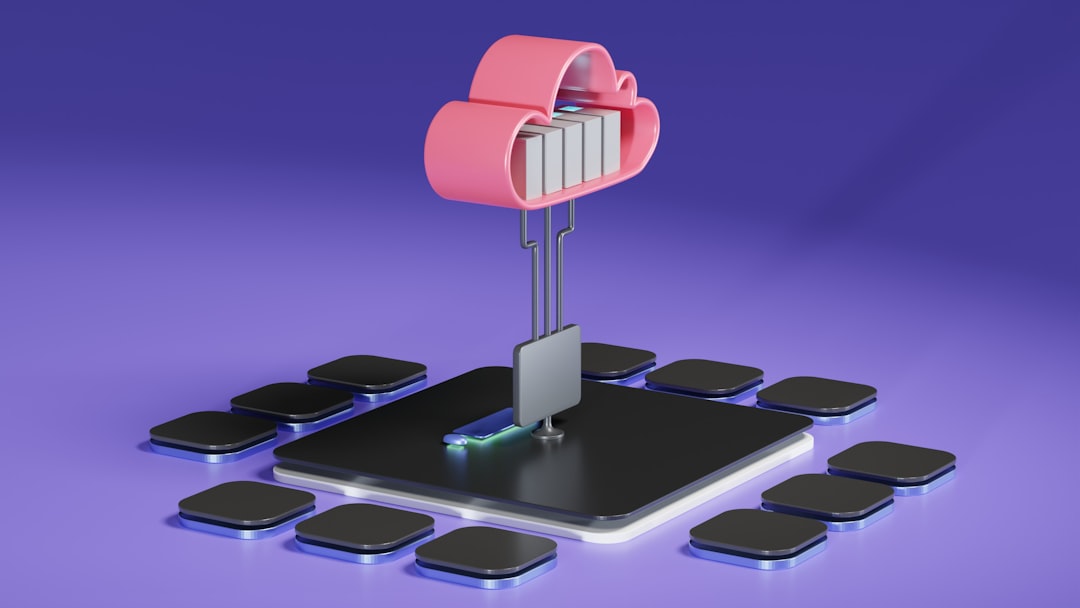
Cloud-based AI platforms are reshaping how maintenance is handled by providing enhanced data analysis and immediate insights. This change pushes businesses to move from a reactive approach to a proactive one when it comes to maintenance. By combining historical and current operational data, these systems can help businesses anticipate equipment breakdowns before they happen, leading to timely interventions that stop costly disruptions. The growing idea of Predictive Maintenance as a Service (PdMaaS) is making these advanced analytical capabilities accessible to companies of all sizes. However, as the integration of these systems evolves, there are hurdles, especially making sure the input data is reliable and adapting operational routines to get the most from the information generated. As 2024 unfolds, the power of cloud-based AI for improving maintenance is evident, but the path forward involves carefully handling both the opportunities and the potential difficulties.
Cloud-based AI platforms are revolutionizing how we approach maintenance, particularly in the realm of predictive maintenance. It's fascinating how they've streamlined the entire process, offering several advantages over traditional methods.
One notable aspect is the ability to visualize data in real-time. These systems can tap into data streams from IoT devices, giving maintenance teams immediate insights into equipment performance. This real-time feedback empowers quicker decisions, enabling more proactive responses to anomalies compared to the old method of scheduled inspections.
Furthermore, cloud platforms excel at managing the increasing amounts of data coming from various sources. Their inherent scalability allows for the seamless integration of data from a growing number of devices. This is a significant advantage because it enables maintenance teams to dynamically adapt resource allocation to meet real-time needs without sacrificing operational efficiency.
The machine learning models employed within these platforms also exhibit adaptability. These AI systems learn from new data continuously, refining their predictive capabilities over time. The more data they process, the better they become at anticipating maintenance requirements, potentially leading to increased accuracy in the future.
Moreover, cloud-based AI platforms foster collaboration across teams. Since data is centralized, various teams—including IT, operations, and engineering—can readily access and utilize the information. This shared access promotes faster problem-solving because different expertise can contribute based on a unified understanding of system health.
It's been interesting to observe how AI-driven predictive maintenance is finding applications in previously unforeseen domains, like agriculture. Farmers are utilizing sensor data to monitor their equipment's condition in real-time, enabling them to proactively address issues before they disrupt the crucial growing seasons. This illustrates how these technologies are adaptable and can be tailored to a variety of contexts.
Many platforms now offer integrated cost-benefit analysis tools. These are valuable for organizations aiming to justify investments in predictive maintenance. With clear ROI figures, obtaining budget approvals for these projects becomes easier, encouraging wider adoption.
Another intriguing development is the integration of external data sources. These AI systems can factor in external variables, such as weather or traffic patterns, into their models. This provides a more comprehensive view of how external conditions might impact equipment reliability, ultimately refining the accuracy of predictions.
There's a clear trend towards creating user-friendly interfaces for these platforms, making them accessible to non-technical personnel. This democratization of technology allows a broader range of employees to interact with maintenance data, contributing to decision-making and improving overall awareness of system health.
Some platforms are going even further by automating the procurement of spare parts based on anticipated needs. The AI system automatically orders necessary components when a potential failure is predicted. This capability can significantly reduce repair times, ensuring operations continue without interruption.
Finally, newer machine learning models are capable of anomaly detection even without relying on large amounts of historical data. This unsupervised learning capability is particularly useful for new installations or when integrating new equipment into a workflow. This signifies a fascinating area of AI research where these systems are increasingly capable of recognizing patterns outside of predefined frameworks.
The ongoing development and integration of AI within cloud platforms will undoubtedly continue to reshape maintenance workflows. It's an exciting time to observe how these innovations are streamlining operations and enabling greater efficiency in managing complex systems.
The Rise of AI-Driven Predictive Maintenance in Business IT Solutions A 2024 Perspective - Cybersecurity benefits from AI-driven threat prediction
In 2024, AI's role in cybersecurity is increasingly focused on predicting threats. As cyberattacks become more sophisticated and data breaches surge, the need for proactive defenses is paramount. AI's ability to analyze massive amounts of data allows cybersecurity systems to spot emerging threats much faster than traditional methods, and can also predict potential future threats based on learned patterns. This predictive capability enables organizations to take preventative action, patching vulnerabilities and strengthening defenses before a successful attack can occur. While this is a promising development, implementing AI-powered threat prediction is not without hurdles. Issues like ensuring the accuracy of AI predictions, concerns about the integrity of the data used to train AI systems, and the need for a skilled workforce to manage and interpret AI-driven security solutions all need to be carefully considered. However, in this era of rapidly evolving cyberattacks, developing strong AI-powered cybersecurity defenses has become vital for any organization seeking to protect its sensitive information and maintain operational integrity.
The integration of AI into cybersecurity is proving to be a game-changer in 2024, particularly when it comes to anticipating and preventing threats. It's fascinating how AI algorithms are now capable of analyzing vast amounts of data from various sources, like network logs and user behavior, to identify patterns that indicate potential security breaches. These systems can achieve remarkable speeds in identifying threats, often in a matter of milliseconds, which is considerably faster than traditional methods and human responses. We're seeing reported accuracies exceeding 95% in identifying anomalies, which suggests that the persistent problem of false alarms—a common hurdle in past security measures—is becoming less significant.
One of the most intriguing aspects is AI's ability to adapt to ever-changing cyber threats. Unlike static cybersecurity solutions, AI systems can learn from new data and refine their threat detection methods. This dynamic approach is essential in an environment where new and sophisticated attack methods are being devised constantly. Furthermore, AI enables a more strategic approach to managing security threats. By analyzing the risks associated with different incidents, AI systems can help security teams prioritize their efforts, ensuring that the most critical threats are addressed first. This is a big change from older approaches, which often reacted to every alert, whether high priority or not.
AI is also allowing us to delve deeper into understanding user behavior as a security factor. These systems can monitor how users interact with networks and applications, identify deviations from normal patterns, and potentially catch insider threats or compromised accounts. This focus on behavior adds another layer of security that traditional methods simply couldn't provide. But, it also raises questions regarding the balance between security and privacy and how we design these systems to avoid biases.
We're also observing that integrating data from various sources—including network logs, applications, and user credentials—allows AI security tools to develop a holistic view of the IT landscape, which enhances the accuracy and reliability of threat assessments. However, this richness of data also increases complexity and makes the validation and interpretation of AI outputs challenging. This integration allows for a much richer understanding of potential threats than before but also necessitates a more cautious approach to implementing and evaluating these solutions.
The adoption of AI-driven security tools also has practical implications for the human element of cybersecurity. With AI taking over a lot of the routine monitoring and anomaly detection, human security staff are free to focus on more complex tasks that demand human expertise, such as strategic planning and tackling unique cybersecurity challenges. It's quite a shift in workload, with the need for IT professionals to adjust their skills to working with and supervising complex AI systems.
Perhaps surprisingly, AI integration into cybersecurity has been reported to reduce costs. Organizations have reported up to a 25% decrease in overall security expenses, which is likely a result of fewer breaches and reduced manual interventions. This cost-benefit aspect could be a significant driver for wider adoption of AI-driven cybersecurity measures. These systems are also relatively scalable, which means they can adapt to the growth of organizations—making them relevant to both large enterprises and smaller businesses.
Looking ahead, it appears AI is not just a tool for responding to threats but is also providing capabilities for "proactive threat hunting." This means AI systems can actively scan for potential vulnerabilities before they are exploited, making IT environments more resistant to attacks. There are still challenges related to the accuracy, explainability, and data availability, but the future of cybersecurity seems to be intrinsically tied to the advancements in AI. This transition, however, demands continuous research and careful consideration, as it’s not just about the technology itself but also the human impact and the ethical considerations around its use.
The Rise of AI-Driven Predictive Maintenance in Business IT Solutions A 2024 Perspective - Challenges in implementing AI-powered maintenance solutions
Implementing AI-powered maintenance solutions presents several obstacles that organizations need to overcome to fully realize the benefits. One major issue is the difficulty in scaling up these solutions. What works well in a small-scale trial can be challenging to implement across a large enterprise, especially with complex legacy systems. Furthermore, the quality of data used to train AI models is crucial for accurate predictions. Poor-quality data can easily result in unreliable outputs and flawed maintenance strategies. There's also a growing need for individuals with specialized skills to understand the AI's output and manage its ongoing development and upkeep. Although the potential of AI-driven maintenance for reducing downtime and operational costs is undeniable, companies must be aware of these difficulties and approach implementation with careful planning and adaptation to achieve their objectives.
Implementing AI-driven maintenance solutions, while promising, faces several hurdles. One key challenge is ensuring high-quality data feeds the AI systems. If the data is flawed or incomplete, the predictions generated will likely be inaccurate, potentially causing more maintenance issues than they solve. This highlights the crucial need for thorough data cleansing and validation processes.
Another major challenge is integrating AI systems into existing operational workflows. Many businesses struggle to connect these sophisticated tools with older systems, leading to operational bottlenecks and frustrations. It's often a complex undertaking to harmonize new technologies with legacy infrastructure.
Furthermore, the adoption of AI-driven maintenance requires specialized skills that many businesses simply don't have readily available. Finding and training staff to manage these systems can be a significant obstacle, impacting the effective deployment and utilization of these advanced technologies.
AI systems, while adaptive, can also be susceptible to a phenomenon called overfitting. If they are only trained on a specific set of data, they might struggle to predict outcomes in new operational settings or when conditions change. This can undermine the reliability of the AI predictions and impact the intended gains.
Moreover, AI systems can unintentionally reinforce biases that might exist in the data they are trained on. If historical data reflects inequalities or discrepancies, the AI might perpetuate these biases in its predictions. This underscores the importance of carefully examining the data sets used and regularly evaluating the models for fairness.
Getting started with AI-driven maintenance also involves a significant initial investment. The upfront costs for hardware, software, and expertise can be a deterrent for many companies, especially smaller ones. This investment also requires time and resources for implementation and continued maintenance.
While advancements in AI have reduced false positives, excessive alerts can still be an issue. Too many alerts can lead to what's known as "alert fatigue", where operators simply tune out the warnings, potentially missing critical signals. Finding a balance between effective alerting and avoiding information overload remains a key challenge.
The field of AI is constantly evolving, and this requires companies to be nimble and adaptable. Predictive maintenance systems need to be continually updated to remain effective. Failure to stay current with new versions and approaches could lead to technological obsolescence.
AI-powered maintenance solutions often heavily rely on real-time data to function effectively. Without a robust infrastructure to capture and transmit data, there will be gaps in the monitoring and analysis processes. Maintaining a continuous and dependable data stream is essential for the successful use of these tools.
Lastly, building trust and transparency in AI systems is critical for acceptance. Maintenance staff might be hesitant to rely on automated recommendations without a clear understanding of the AI's decision-making processes. Explaining how the AI works and the rationale behind its recommendations is essential for building user confidence and ensuring its effective implementation.
More Posts from zdnetinside.com: