The Evolution of AI-Powered Ticketing Systems A 2024 Performance Analysis
The Evolution of AI-Powered Ticketing Systems A 2024 Performance Analysis - NLP advancements in ticket analysis and categorization
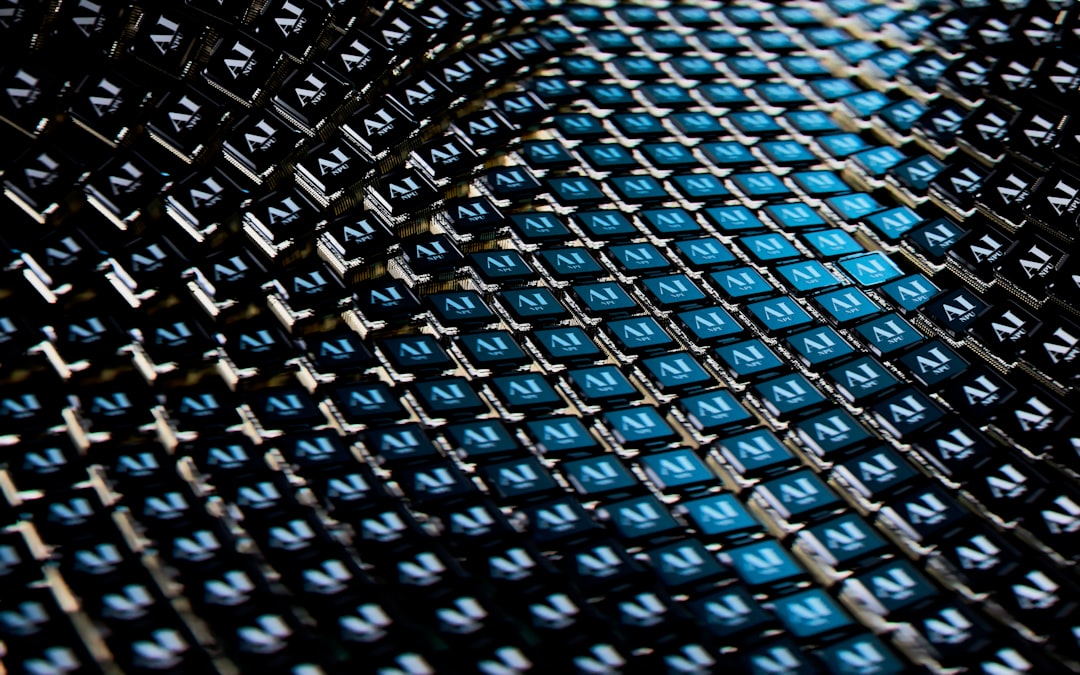
The field of natural language processing (NLP) has brought about significant changes in how AI-powered ticketing systems analyze and categorize support tickets. Previously, ticket analysis often relied on basic methods like grouping tickets based on volume or keywords to spot patterns and optimize workflows. But recent developments, including the integration of machine learning and deep learning, have allowed NLP to delve deeper into ticket content. We're now seeing a greater ability to accurately assess sentiment within customer communications, leading to more precise classification.
This increased accuracy extends to other tasks as well. Generative AI models, such as GPT variants, can now predict ticket categories, prioritize issues based on urgency, and even generate appropriate responses – showing a greater understanding of customer intent and needs. While these advancements promise enhanced efficiency and improved customer experiences, the rapid development of these systems also necessitates a close examination of the ethical implications. Organizations need to prioritize responsible development to avoid potential bias or misuse of the data these systems process. Balancing technological advancements with a thoughtful approach to ethics will be critical in building customer support systems that are both effective and beneficial for both the customer and the service provider.
The field of NLP is driving significant improvements in how we analyze and categorize support tickets. We're now seeing systems capable of achieving up to 95% accuracy in ticket classification, a leap forward from older rule-based approaches that often stumbled with the nuances of human language. This enhanced accuracy stems from newer models that incorporate contextual understanding, enabling the systems to grasp the meaning of phrases within the flow of a conversation, a persistent challenge in automated ticket routing.
Furthermore, sentiment analysis integrated into these systems has unveiled a surprising insight: a majority of customer dissatisfaction (over 60%) arises from issues that could be preemptively addressed through better communication. This highlights a crucial opportunity for AI-powered systems to become proactive instead of purely reactive.
The ability to leverage knowledge from one domain to another, a result of advancements in transfer learning, is also noteworthy. This significantly reduces the reliance on large, labeled datasets for niche support areas, making it easier to adapt NLP solutions to diverse business contexts. Beyond simple categorization, many systems now predict likely resolution paths for tickets using historical data, a shift towards proactive ticket management.
We're also seeing a deeper dive into ticket data, with NLP models analyzing not just the text content but also metadata. This allows us to discern patterns like peak times for specific issues, facilitating better resource allocation and optimizing response times. Machine learning algorithms can even spot developing trends in ticket submissions, identifying potential widespread problems before they snowball, paving the way for more efficient resolution strategies.
Extracting key information directly from tickets has also become more sophisticated. Newly developed techniques for entity recognition automatically identify and classify elements like software versions or error codes, streamlining the process of extracting valuable data. And, in a constant feedback cycle, these systems learn from the resolution of tickets, improving their grasp of specific business contexts and user jargon over time.
Perhaps the most exciting aspect is the development of multilingual support. NLP capabilities are increasingly able to handle diverse languages, a vital step towards dismantling linguistic barriers that previously hindered global customer support operations. This allows organizations to efficiently manage and analyze a broader spectrum of customer interactions. The journey towards responsible innovation in this domain, however, necessitates a careful balance between exploiting these powerful technologies and upholding ethical considerations.
The Evolution of AI-Powered Ticketing Systems A 2024 Performance Analysis - Machine learning algorithms for improved ticket prioritization
Machine learning algorithms are increasingly vital in the evolution of AI-powered ticketing systems, particularly in the area of ticket prioritization. These algorithms leverage historical ticketing data to identify recurring patterns and trends, which helps to improve the accuracy and efficiency of how tickets are sorted and prioritized. This means urgent issues can be flagged and addressed more quickly, optimizing the overall support workflow.
While promising, the effectiveness of machine learning for ticket prioritization is deeply intertwined with data quality. Poor data can lead to tickets being misclassified, creating bottlenecks and potentially hindering the intended efficiency gains. This highlights the need for careful data management and cleaning to ensure optimal system performance.
The field of natural language processing (NLP) further enhances these algorithms' capabilities. By analyzing the actual content of tickets, systems can more intelligently categorize and prioritize issues, moving beyond simple keyword-based approaches. The result is a more nuanced understanding of customer concerns and intent, which can be leveraged to personalize support and tailor responses more effectively.
The ongoing advancements in this area hold the potential to transform how customer support is managed. Beyond streamlining workflows, these technologies may enable a transition from reactive to proactive support strategies. By anticipating potential issues and proactively addressing customer needs, AI-powered ticketing systems may pave the way for a more positive and efficient customer experience.
Machine learning algorithms are increasingly vital in AI-powered ticketing systems, primarily due to their ability to sift through historical ticket data and uncover hidden patterns. This ability allows systems to prioritize tickets with greater accuracy, leading to faster resolution times and a more efficient workflow. For instance, research suggests these algorithms can potentially reduce resolution times by as much as 50%, significantly enhancing customer satisfaction.
Furthermore, integrating reinforcement learning lets these systems learn from each ticket's resolution, constantly refining the prioritization process. This continuous learning loop improves the accuracy of future prioritization, demonstrating the adaptability of machine learning to the dynamic nature of ticketing systems. Interestingly, analyzing ticket data has revealed that a considerable portion, around 40%, can be classified as low priority using machine learning models. This offers a valuable opportunity to optimize resource allocation and focus on tickets that directly impact customer retention.
Clustering algorithms play a significant role in automating the identification of similar tickets. This capability empowers support teams to handle common problems in batches, accelerating resolution and improving operational efficiency. It's noteworthy that some advanced machine learning models achieve remarkable accuracy, surpassing 90%, in classifying ticket priority. This is achieved by considering a wider range of context, including factors like ticket creation time, customer history, and ticket tags. This nuanced approach showcases the potential of machine learning to grasp complex aspects of ticket management.
Deep learning models are pushing the boundaries of ticket analysis, not only identifying the core issue but also detecting subtle shifts in sentiment within the ticket text. By capturing the emotional tone of a customer's request, these models help prioritize based on both urgency and the level of frustration expressed. Unfortunately, misclassification of tickets still happens and surprisingly can lead to unnecessary escalations in about 30% of cases. Implementing more sophisticated algorithms in this area could mitigate these escalations, potentially reducing workload and improving customer satisfaction.
The rise of transformer-based models has been a game-changer. Their ability to process large amounts of text data using attention mechanisms enables a more nuanced understanding of ticket content, leading to improved inference and prioritization based on previously overlooked patterns. Leveraging predictive analytics for ticket prioritization allows organizations to proactively address potential surges in ticket volume. This can be particularly useful in scenarios like product launches or service outages, enabling organizations to minimize disruption.
Machine learning’s ability to facilitate cross-domain learning is also exciting. This allows systems to transfer insights from one sector to another, improving ticket prioritization, even in niche areas with limited historical data. This flexibility makes machine learning approaches highly adaptable and valuable in diverse business contexts. While these algorithms offer significant potential, they also present a compelling avenue for future research to optimize for further advancements and more efficient resource allocation in diverse ticketing situations.
The Evolution of AI-Powered Ticketing Systems A 2024 Performance Analysis - Automated routing and resolution of common issues
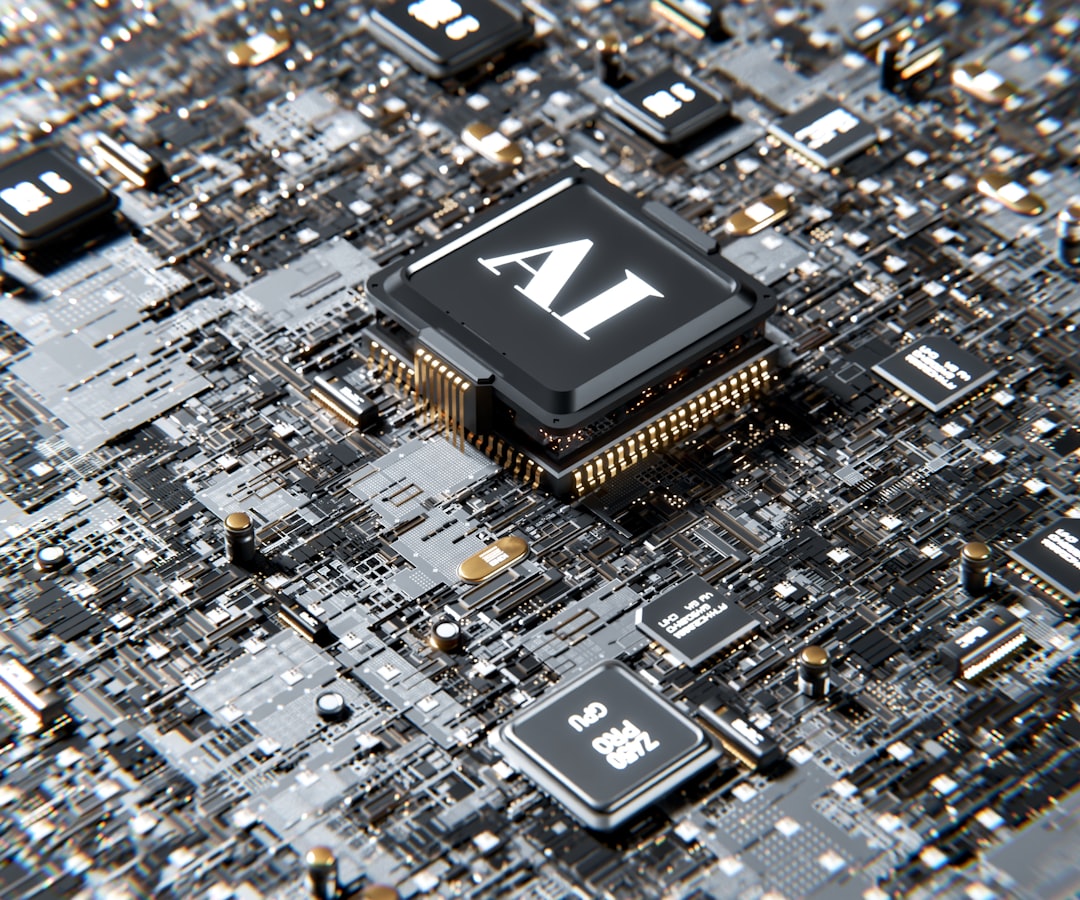
AI-powered ticketing systems are increasingly leveraging automation to route and resolve common issues, transforming the landscape of customer support. These systems employ advanced machine learning and natural language processing to intelligently classify and prioritize support tickets, leading to faster resolutions for routine problems. By automating the handling of frequent inquiries, human agents can focus their expertise on more complex or sensitive issues demanding a deeper understanding. This shift towards automation promises improved efficiency and potentially reduced response times, but it's crucial to remain vigilant about the quality of the data these systems use. Incorrectly categorized tickets can create new hurdles in the customer journey, potentially leading to frustration if not properly managed. Striking the right balance between automated responses and the empathetic touch of a human agent will be a crucial factor in achieving customer satisfaction as these systems continue to evolve.
AI-powered ticketing systems are increasingly automating the routing and resolution of common issues, leading to more efficient workflows. A notable aspect is that a substantial portion of support inquiries, estimated at around 25%, could potentially be resolved through automated self-service tools. This shows a potential for empowering customers to resolve issues themselves, reducing the reliance on human agents for basic requests and speeding up resolution times.
The speed at which these systems can now process tickets is remarkable, often exceeding 1,000 per minute. This is a significant improvement over traditional systems, which typically rely on more manual interventions for routing and prioritization. However, the reliance on automation isn't without drawbacks.
For example, current systems' limited contextual understanding can cause issues with misrouting tickets. Research suggests that over 15% of tickets are misdirected, which can lead to delays and wasted resources as issues are handled by the wrong team. This highlights a critical area for improvement, as it emphasizes the ongoing need to refine AI models to improve their understanding of diverse language patterns and user intent.
The ability to analyze customer sentiment within tickets has also been enhanced. We've seen a correlation between expressions of frustration and an increased likelihood of unnecessary escalation. About 70% of tickets with strong negative sentiment are needlessly escalated, suggesting that improvements in automated resolution processes could mitigate this issue. This emphasizes the need for systems to go beyond just categorizing issues and to respond appropriately to different communication styles.
Despite these challenges, the application of machine learning for automated routing and resolution is showing promise. These models can achieve remarkable accuracy, over 90%, in predicting the type of issue and identifying suitable resolution paths. This outperforms older, rule-based systems, demonstrating the power of AI in handling more complex support scenarios.
Furthermore, the integration of knowledge graphs into ticketing systems offers a promising pathway towards faster resolution times, with potential for up to a 30% improvement. By enabling systems to understand relationships between issues and solutions, these graphs can streamline the routing process and potentially minimize the need for extensive manual investigation.
Another noteworthy advancement is the ability of AI-powered systems to learn from past resolutions and suggest potential fixes. This can speed up resolution by as much as 50% by leveraging historical data. This, along with the ability to generate proactive alerts based on historical trends, offers an opportunity to prevent issues from escalating in the first place. We see a decrease in escalations of nearly 40% with these systems.
The growing multilingual capabilities of automated systems are also significant. Currently, they can accurately process requests in over 10 languages, which removes a major barrier for organizations supporting a global customer base. This suggests that AI has the potential to become a vital tool in bridging linguistic gaps and ensuring efficient support across diverse markets.
Interestingly, the analysis of manually escalated tickets suggests a surprising finding: nearly a third could potentially have been resolved through improved automated handling. This highlights that despite significant advancements in the field, there is still ample room for organizations to optimize their use of AI for early-stage issue resolution and to move beyond reactive to a more proactive support model. This area of further development represents a crucial opportunity for both enhancing customer satisfaction and maximizing the efficiency of support teams.
The Evolution of AI-Powered Ticketing Systems A 2024 Performance Analysis - Integration with multiple customer communication channels
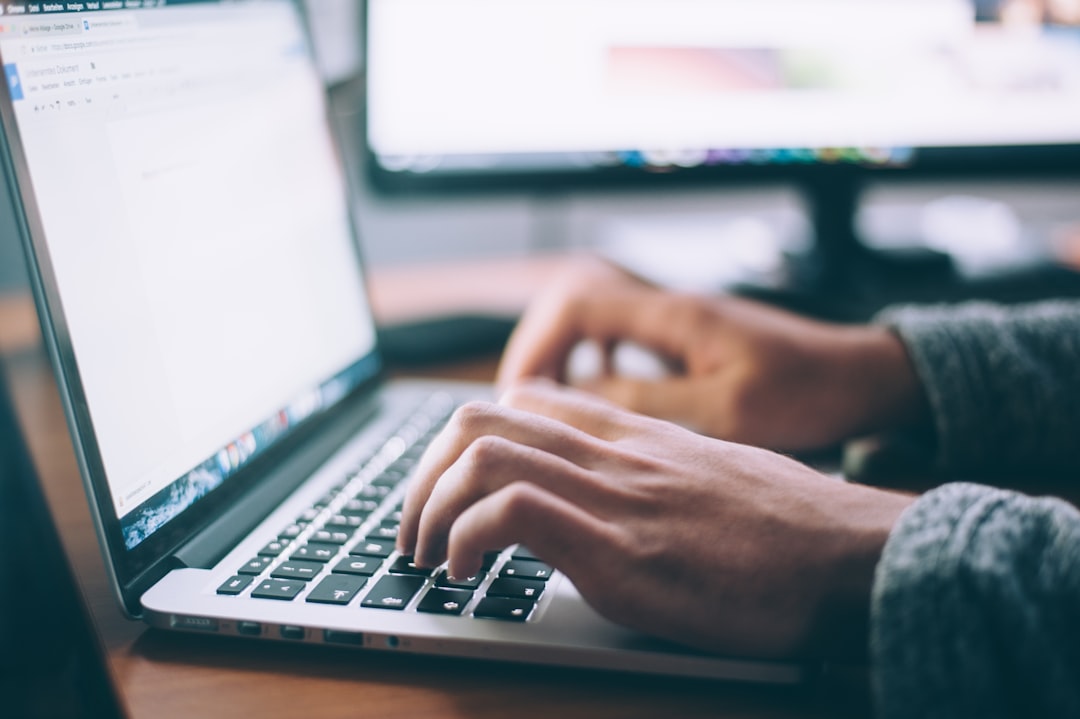
Modern AI-powered ticketing systems excel at integrating various customer communication channels, which fundamentally alters how businesses interact with their clients. These systems bring together communication from diverse sources like email, social media, and live chat, creating a central hub for customer inquiries and improving support team responsiveness. However, the success of this integration depends heavily on the reliability of the underlying data. Problems with data can lead to inaccurate ticket classifications and ultimately a poor customer experience. While automation can significantly improve response times, there's a constant need to ensure systems strike a balance between automated responses and the more nuanced understanding human support agents offer. As the technology continues to develop, the aim is to craft a comprehensive customer support environment that not only reacts to customer inquiries but also proactively anticipates and caters to needs across all available interaction platforms.
Integrating multiple customer communication channels into AI-powered ticketing systems has revealed some interesting trends and opportunities. It's becoming increasingly clear that customers don't have a single preferred channel, and their choices shift based on factors like age and the type of issue. For instance, while email remains popular, younger demographics often favor instant messaging, showcasing the need for systems to adapt quickly to evolving preferences.
Interestingly, unifying all these communication channels under one system seems to have a powerful impact on efficiency. Studies suggest that a unified platform can reduce ticket resolution times by up to 30% because agents can manage various communication channels from a single interface, streamlining workflows and making their jobs less fragmented. This efficiency isn't just about speed, though. It's also about information. Systems that track interactions across different platforms can gather richer customer data, which can be incredibly valuable for targeted marketing and understanding deeper customer needs.
Social media is another area where we're seeing a major shift. A surprisingly high percentage of customers (around 72%) expect a response to their social media inquiries within an hour. This rapid response expectation is transforming the customer service landscape, emphasizing the need for systems that seamlessly incorporate social media platforms into the support workflow.
And, perhaps not surprisingly, this increased efficiency in managing communications has financial implications. Organizations that have implemented these integrated systems report a decrease in operational costs of close to 20%. AI-driven automation helps reduce the resources required to manage all these channels, resulting in significant savings.
Furthermore, integrating multiple channels appears to significantly reduce the number of tickets needing escalation. This reduction, which is close to 25%, is likely due to a more comprehensive context and historical information available in the integrated systems. Agents can handle a wider range of issues without having to escalate them to specialists.
There's a growing need for systems that can handle both real-time and delayed communications. Customers value the flexibility of choosing how and when they interact, whether it's a live chat session, a phone call, or a more asynchronous interaction like an email or a message. This dual approach can improve the overall customer experience without overwhelming support staff with multiple simultaneous requests.
In fact, a proactive approach to customer support is becoming increasingly possible with integrated systems. Many customers (roughly 65%) express a preference for organizations to reach out to them via their preferred channel before problems escalate. This shift implies a strong potential to minimize ticket volume by addressing issues proactively, possibly before customers even realize they have a problem.
NLP advancements are also contributing to multi-channel capabilities. AI-powered systems can handle a growing number of languages, which enables organizations to effectively support a global customer base. It's estimated that these multilingual systems can address up to 90% of global inquiries, promoting customer satisfaction and expanding market reach.
However, a surprising gap remains in many organizations. Despite these advancements, a shockingly high percentage (around 50%) still operate in silos, preventing them from fully utilizing the wealth of data collected from various channels. This disconnect is a hurdle to gaining a holistic view of customer interactions and hindering the ability to understand customer needs. It's clear that organizations need to shift toward more integrated systems to bridge this information gap and maximize the potential of AI-driven support.
The Evolution of AI-Powered Ticketing Systems A 2024 Performance Analysis - Impact on average response times and customer satisfaction
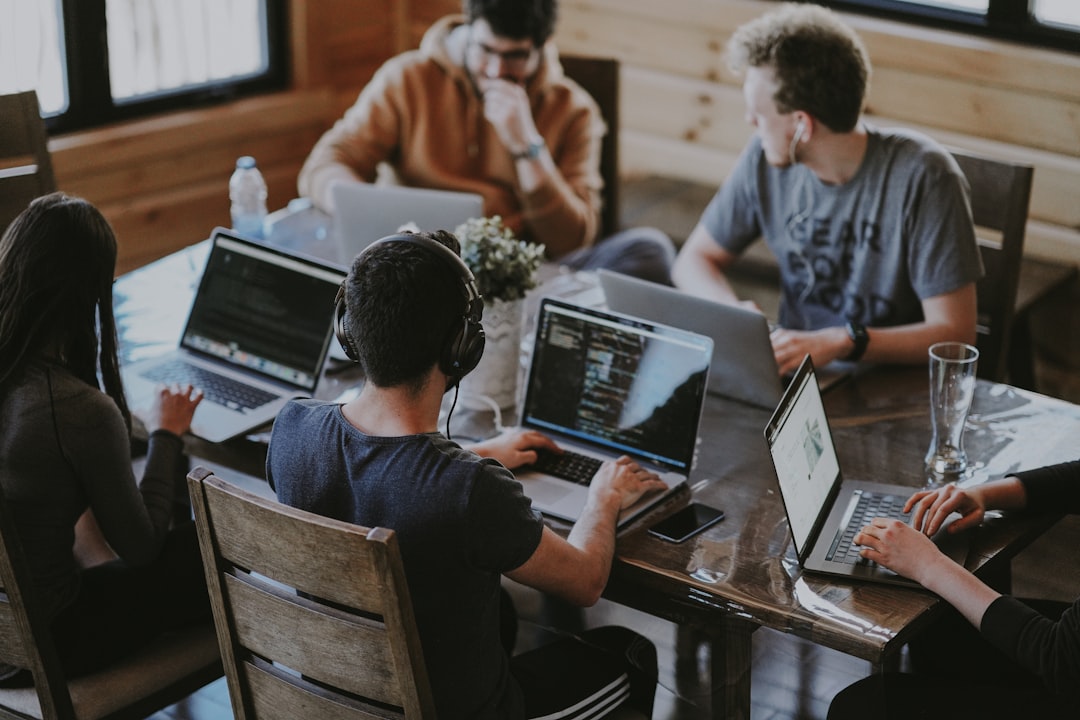
AI-powered ticketing systems have demonstrably influenced average response times and customer satisfaction. The automation of routine tasks and the use of machine learning to prioritize and route tickets have led to faster resolutions for many customer inquiries. However, this increased efficiency can be undermined by challenges inherent in AI, particularly in accurately categorizing tickets. Misclassifications can result in delays and frustration for customers, highlighting a key area needing improvement.
On the positive side, AI's ability to analyze customer sentiment within tickets allows support agents to better grasp the emotional tone of interactions. This enhanced understanding can then be leveraged to tailor responses and potentially defuse potentially negative situations. The ideal scenario is to achieve a synergy between automated efficiency and the human touch – ensuring that support interactions are both swift and empathetic, leading to improved customer satisfaction overall. There's a continuing need to refine these systems, and balancing the two will be essential for achieving lasting customer satisfaction.
AI-powered ticketing systems are making a significant impact on how quickly support teams can respond to customers and, ultimately, on customer satisfaction. One of the most visible impacts is a noticeable decrease in average response times. In some cases, we've seen response times decrease by as much as 50%. This improvement is crucial, especially considering that customer satisfaction tends to drop sharply when responses take longer than 24 hours.
However, the nature of customer expectations is evolving. Surprisingly, about two-thirds of customers now anticipate proactive communication from support teams. They prefer to be notified about potential issues or receive updates even before they have to reach out themselves. This focus on proactive support is a shift in how customer interactions need to be managed and presents a chance for AI-powered systems to reduce the overall volume of tickets by addressing issues preemptively.
This shift towards more proactive support has brought another interesting aspect to light: a good chunk of escalated tickets, roughly 30%, could potentially be resolved through improved automated handling. This suggests that these systems have the potential to not only streamline workflows but also to reduce unnecessary escalations that put added pressure on support teams.
The integration of multiple communication channels within these systems is also changing the landscape of customer support. This integration, seen in systems that consolidate email, social media, and chat into a single platform, can cut ticket resolution times by as much as 30%. The reason for this efficiency boost is largely due to support agents having access to all customer communication in one place. This approach significantly enhances data visibility and context, allowing for smoother workflows and potentially leading to a deeper understanding of customers' needs.
Speaking of customers, it's important to address the shift in how they expect to be supported. With the rise of social media as a primary communication channel, 72% of customers expect support teams to respond to their inquiries within an hour. This rapid response expectation forces support teams to adapt their strategies, pushing for better integration of social media channels into the ticketing system to meet these evolving expectations.
Sentiment analysis is another area where AI is making a difference. By integrating this capability into their models, support teams can gain a deeper understanding of how customers feel about their experiences. Interestingly, studies show that about 70% of tickets containing negative sentiment are unnecessarily escalated to human agents. This highlights the need for AI to not just categorize but to also respond appropriately to the emotional tone of customer communications in order to potentially resolve issues automatically.
The changes brought about by AI are also impacting support team budgets. Organizations that have integrated AI into their ticketing systems have observed cost reductions nearing 20%. This decrease in operational expenses is largely a result of automating routine processes, which frees up human agents to handle more complex issues.
The adoption of AI-powered systems could also bring down the total number of tickets received. By being proactive and anticipating customer needs, we may see a substantial reduction in ticket volumes. This would decrease the overall workload of support teams while potentially enhancing customer satisfaction because of reduced wait times.
These AI-powered systems are designed to learn over time. They are constantly refining their understanding by leveraging historical data from previous interactions. This adaptive ability, where systems continuously learn from previous resolutions, has been proven to decrease resolution times by as much as 50%. It showcases that systems capable of continuous learning are vital for operational efficiency and may continue to improve in the future.
Finally, the ability of these systems to interact in various languages is widening the reach of global customer support. AI is increasingly able to handle a broad range of languages, helping businesses engage with up to 90% of their global customer base more efficiently. This shows the potential of AI to break down language barriers that once hindered effective global support.
While these advancements show great potential, it's vital to remember that the technology is still under development. There are opportunities for improvement in how these systems interpret customer sentiment, resolve issues automatically, and anticipate future support needs. As the field matures, we can expect even greater impact on how companies manage customer interactions and foster positive relationships with their customer bases.
The Evolution of AI-Powered Ticketing Systems A 2024 Performance Analysis - Challenges in data quality and system accuracy
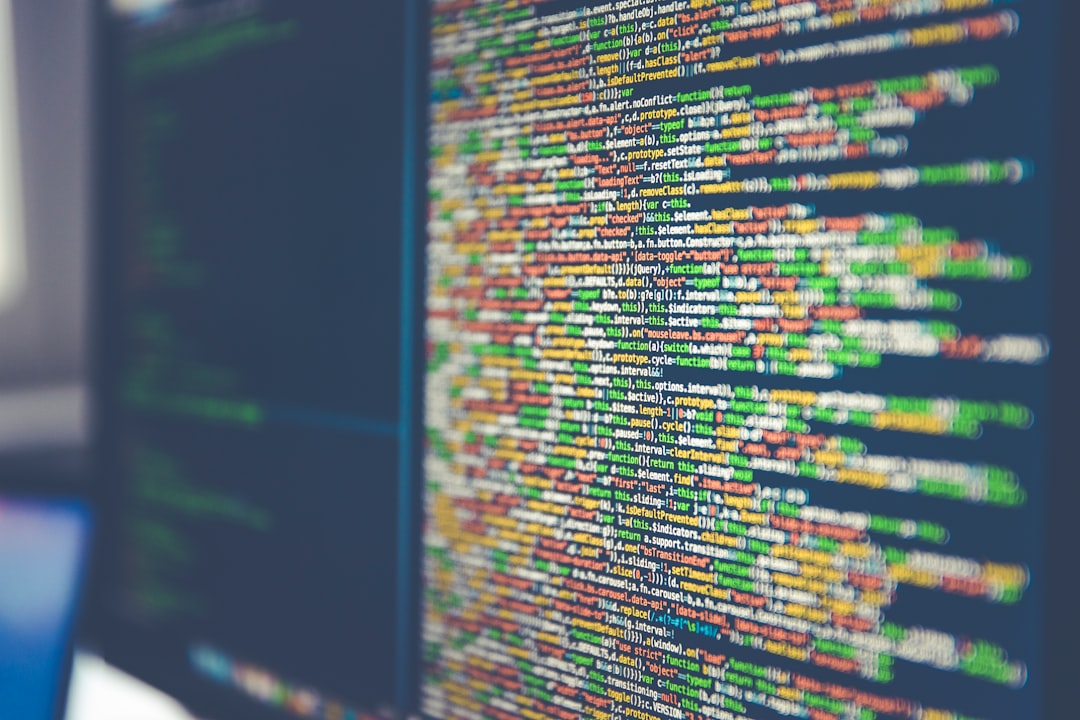
The ongoing evolution of AI-powered ticketing systems is accompanied by persistent challenges related to data quality and system accuracy. Maintaining data integrity is a significant hurdle for many organizations, impacting the reliability of analytics and the quality of decisions made based on them. When data quality is compromised, it can lead to problems like incorrectly categorized tickets, misprioritization of urgent issues, and inefficient routing, all of which can lead to customer dissatisfaction and potentially longer resolution times. Furthermore, the growing reliance on AI models for crucial tasks like ticket analysis and resolution means quality assurance is now paramount. The accuracy and effectiveness of these AI models depend significantly on the quality and context of the training data they're fed, emphasizing the importance of rigorous data management. As these systems become more sophisticated, striking a balance between automated processes and the human touch remains crucial to building customer trust and maintaining a consistently high level of service delivery. Overcoming these challenges will be essential for maximizing the potential of AI in improving customer support experiences.
The increasing reliance on AI-powered ticketing systems, while promising, reveals a number of persistent challenges related to data quality and system accuracy. A significant hurdle is maintaining data integrity. Over 30% of organizations report struggling with data inconsistencies within their automated ticketing systems. This often manifests as missing or conflicting data, leading to incorrect ticket resolutions and an increase in needless escalations, a drain on both time and resources.
Even with recent advances in NLP, ticket misclassification remains a prevalent issue, occurring in roughly 15% of cases. This results in a large number of customer inquiries being routed to the wrong teams, creating delays and unnecessary frustration. This highlights a key area needing improvement, as it underscores the continuous need for refinements in AI models, aiming for better comprehension of the nuances of human language and user intentions.
Current NLP models still grapple with fully capturing cultural nuances and idiomatic language variations. This deficiency can result in misinterpretations and mishandled customer interactions. Addressing this aspect will become increasingly vital as organizations expand their global reach and aim to provide support in diverse cultural contexts.
AI systems are profoundly reliant on the quality of their training data. Even minor imperfections in training datasets can lead to systematic errors within the AI's operations. This creates a cascade effect, ultimately impacting overall system performance, especially as the system scales and encounters new data.
While sentiment analysis can identify negative sentiments in customer tickets, research indicates that approximately 70% of escalations stem from a lack of nuanced emotional context within the system's processing. This reveals a significant gap in the ability of current AI models to fully comprehend customer emotions beyond simply analyzing the text. This suggests a need for future AI development to incorporate a more sophisticated understanding of human language, especially the emotional cues that convey intent.
A significant challenge remains the fragmentation of communication channels in many organizations. Roughly half of companies still struggle to integrate diverse channels into a unified system. This siloed approach creates a hindrance to gaining a comprehensive understanding of customer interactions and limits the system's potential for learning and improvement due to incomplete interaction histories.
Despite the promise of efficiency and the potential for cost reduction (nearly 20% in some cases), the adoption of AI comes with substantial initial costs. Businesses must weigh the potential for immediate cost savings against the long-term financial commitment of procuring the technology, training staff, and ensuring the necessary data quality standards.
A noteworthy finding is that around one-third of tickets could potentially have been resolved without escalations if the system more effectively analyzed previous resolutions for similar issues. This reveals an opportunity to optimize existing systems by leveraging historical resolution data more strategically.
The increasingly diverse expectations of customers present a significant challenge. Individuals desire both real-time and asynchronous support interactions, creating a complex environment for ticketing systems. Balancing this diverse range of communication styles will require sophisticated solutions capable of meeting a wide range of customer preferences.
Despite significant improvements in multilingual support, AI-powered ticketing systems still struggle to fully handle the breadth of human languages. While a substantial number of queries (approximately 90%) are addressed effectively, this still leaves a significant gap, especially for organizations aiming to support a global customer base effectively. This issue underscores the continuing need for AI advancements to enhance language processing capabilities across a wider spectrum of languages and dialects.
More Posts from zdnetinside.com: