The Overlooked Role of Data Governance in Digital Transformation Success
The Overlooked Role of Data Governance in Digital Transformation Success - Data Management Practices Essential for Digital Success
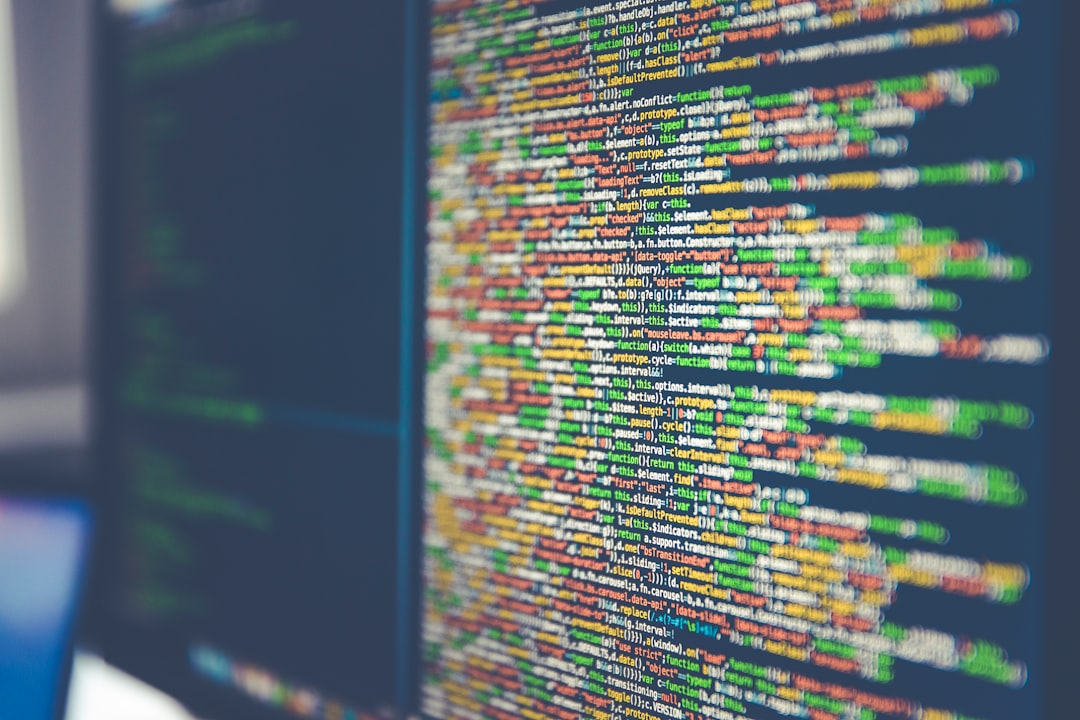
In the realm of digital transformation, effective data management practices are no longer optional – they are foundational for success. Managing the vast and ever-growing volume of data in today's world requires a structured approach, and a robust data governance framework provides exactly that structure. This framework acts as the central nervous system, ensuring that data remains accessible, trustworthy, and compliant with various regulations.
A key component of this framework involves defining clear roles and responsibilities. Establishing who is accountable for data quality and integrity is essential for maintaining data consistency and reliability throughout the organization. Data owners and stewards, empowered through clearly defined roles, become integral to upholding data standards. Moreover, thoughtfully implementing data classification schemes and well-defined data lifecycle management processes – from data creation to eventual deletion – not only enhances operational efficiency but also mitigates risks associated with potential data breaches and security vulnerabilities.
These data management practices ultimately translate into tangible benefits. They empower organizations to make informed decisions based on reliable data, thereby enabling better strategic alignment with business goals. In essence, a strong foundation in data management is a necessary ingredient for maneuvering successfully through the challenging landscape of digital transformation. However, it’s also crucial to acknowledge that data management is an ongoing journey, not a destination. Continual refinement and adaptation are vital to keeping pace with evolving digital landscapes.
Data management practices are fundamental for achieving success in the digital landscape. How organizations manage their data profoundly impacts their ability to leverage it for insights and decision-making. It's not simply about storing data, but about its availability, reliability, and security – aspects overseen by a broader concept known as data governance.
Data governance often necessitates a multi-layered approach that connects business and technical sides of an organization. This usually involves dedicated roles like a Chief Data Officer and the formation of a data governance council. Organizations that are more successful in digital transformation strategically allocate their resources and focus data leadership efforts around the most important data assets that drive business value.
Establishing clear lines of responsibility is critical for effective governance. Defining who owns and maintains the data—data owners and stewards—is foundational. A streamlined, centralized structure helps establish and enforce data quality standards across an organization. This centralization often incorporates robust classification schemes that maintain data integrity and make data management more manageable.
Data has a lifecycle—it’s born, processed, and ultimately retired. Establishing a lifecycle management strategy helps ensure compliance and security at each step of this process. A well-defined governance framework provides a clear pathway for protecting organizational assets from data breaches and ensuring alignment of data management with broader strategic goals.
While data governance is a cornerstone of informed decision-making and maintaining a competitive edge, it's notable that there's often a disconnect in how data is perceived. Many leaders fail to see data as a strategic asset, overlooking the potential for innovation it offers. This lack of understanding can hinder progress in digital transformation. There is strong evidence that data governance initiatives often impact an organization's ability to achieve its goals.
However, the journey towards effective data management is not without its challenges. Many companies struggle to ensure their data across different platforms can work together—data interoperability. The potential benefits of using new technology are enticing—some companies are exploring how machine learning can improve data processing speeds, for example—but the complex nature of data across many systems can create obstacles to realizing these benefits. If these problems aren't addressed, they can impede a company's overall digital transformation initiatives.
The Overlooked Role of Data Governance in Digital Transformation Success - Bridging the Gap Between Data Privacy and Innovation
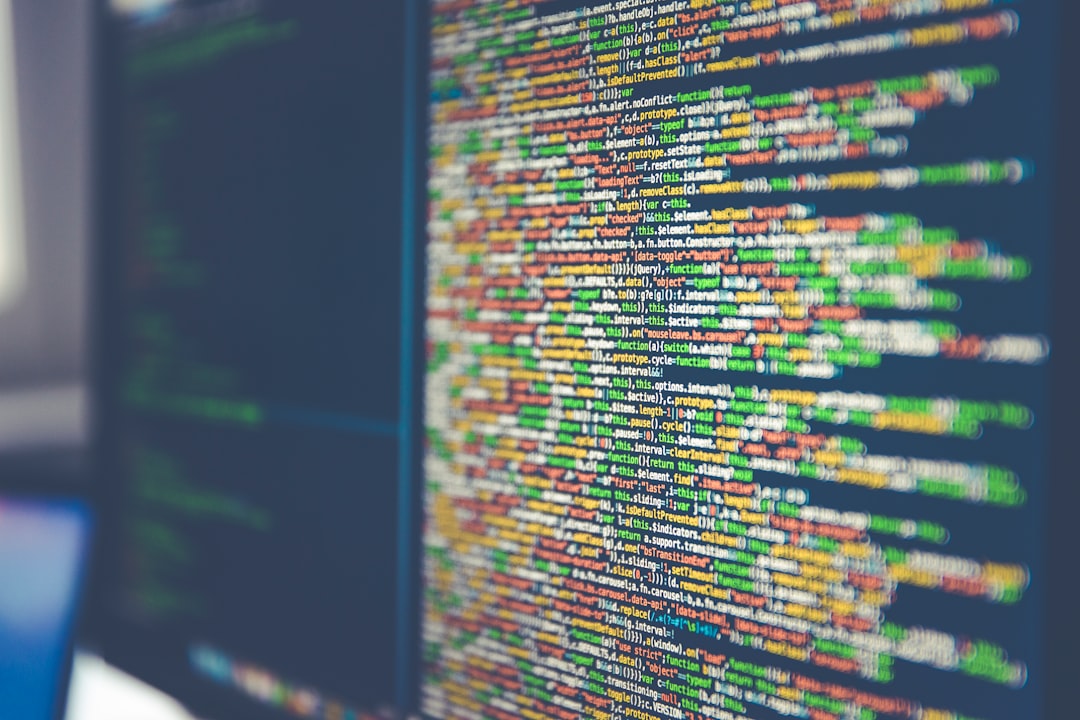
Successfully integrating data privacy concerns into the drive for digital innovation presents a significant hurdle for organizations. The adoption of cutting-edge technologies, including artificial intelligence and large-scale data sets, necessitates a heightened focus on strong data privacy measures. A central element of navigating this complex landscape successfully is the role of data governance. Not only does effective data governance protect consumer data rights and ensure compliance with regulations, but it also cultivates a supportive environment where innovation can flourish. The key lies in achieving a delicate equilibrium between privacy and innovation. When organizations prioritize both, it reinforces consumer trust while simultaneously unlocking the potential of data as a vital resource for driving progress. By building robust data governance structures, businesses can expertly navigate the inherent tensions and unlock new pathways to capitalize on the opportunities present within the evolving digital realm.
The intersection of data privacy and innovation presents a compelling challenge. While we need robust data privacy safeguards to protect individuals, excessive regulation could potentially stifle the very innovation that drives progress. On the other hand, too little focus on privacy risks could lead to data breaches, eroding consumer trust and potentially harming a company's reputation. This delicate balance underscores the need for a nuanced approach.
Evidence suggests that organizations committed to data governance often achieve greater financial success than those that don't. This implies that sound data management practices not only support compliance but can also fuel innovation and growth. However, a significant portion of businesses still haven't fully prepared themselves to meet new privacy standards, making them susceptible to legal action and also potentially limiting their ability to leverage data for innovation.
Interestingly, consumers are often willing to share their data if it translates to a better service or experience. This indicates a potential path forward for businesses to develop creative solutions that utilize data while prioritizing consumer trust and transparency. A deeper look shows that businesses with established data governance frameworks tend to have more streamlined and efficient data management. This suggests that a well-defined structure and clear responsibilities are key to unlocking the potential of data for innovation.
However, the cost of data breaches can be substantial, impacting a company’s financial health and potentially hindering its ability to innovate due to diverted resources and loss of trust. Further complicating matters, a significant portion of breaches appear to stem from human error, rather than sophisticated technical attacks. This highlights the importance of comprehensive employee training and education regarding data privacy.
Unfortunately, data privacy considerations aren't always integrated early into the development cycle of new products and services, suggesting a critical gap between innovation efforts and fundamental data governance principles. This lack of integration may stem from cognitive biases where leadership teams may underestimate the risks of neglecting data governance practices. However, there's evidence to suggest that businesses with formalized data governance policies are more adept at identifying valuable insights from their data. This implies that careful data management not only ensures compliance but also lays the groundwork for innovative discoveries.
The Overlooked Role of Data Governance in Digital Transformation Success - Quality Control Mechanisms in Data-Driven Decision Making
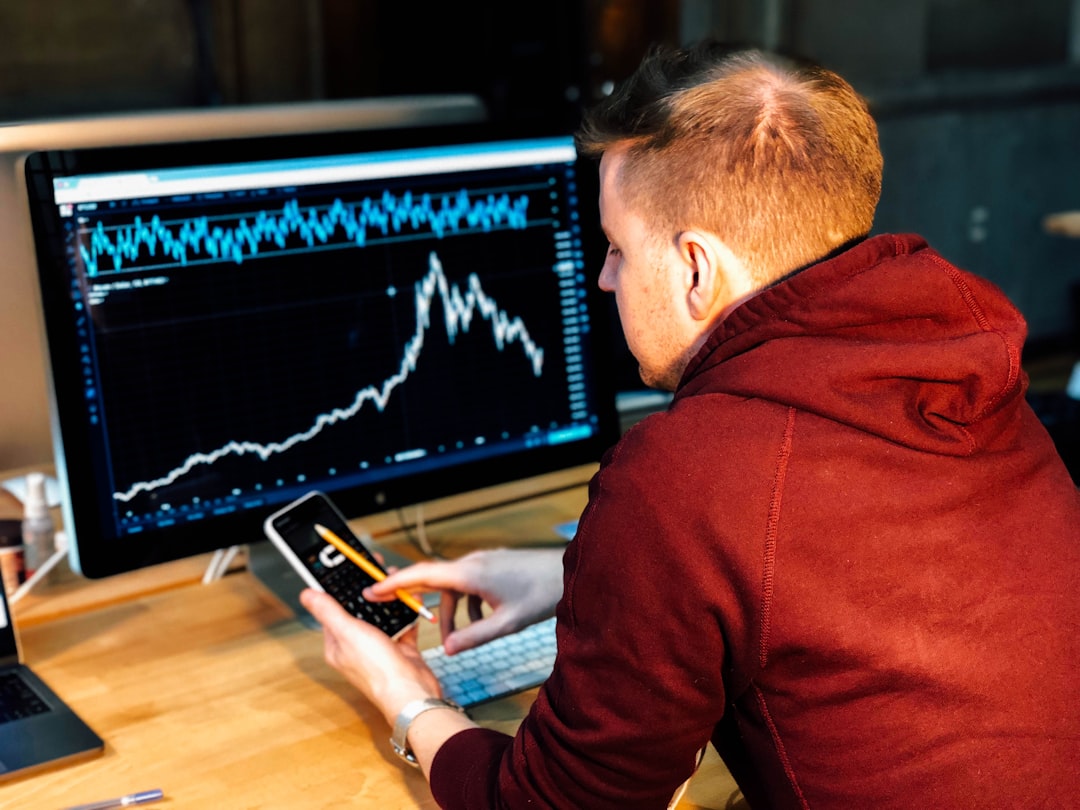
In the pursuit of data-driven decision-making, the quality of the data itself becomes paramount. Organizations need reliable, consistent data to gain accurate insights and make sound choices. This requires implementing robust quality control mechanisms. Regularly assessing data for accuracy, identifying and rectifying errors, and establishing clear processes for data management are all key elements in this effort. Encouraging a data-centric culture, where employees across all levels appreciate and leverage data's insights, also plays a vital role. Despite these efforts, challenges remain. Data silos and the fragmented nature of data across different systems create inconsistencies and complicate efforts to establish strong data governance. Successfully navigating these hurdles and prioritizing quality control helps ensure that organizations derive maximum value from their data while successfully navigating the complexities of digital transformation.
Data governance, as we've explored, forms the bedrock of successful digital transformation by ensuring data's reliability and accessibility. However, within this framework, the specific mechanisms for ensuring data quality—a critical aspect of trustworthy data—deserve more attention. It's not just about having data, but having data that's fit for purpose.
Research suggests that prioritizing data quality can significantly accelerate and enhance decision-making processes. Studies show that organizations with a keen focus on data quality can see a boost in decision-making speed and accuracy, potentially up to 40%. This improvement, stemming from reliable information, directly translates to operational efficiency and agility.
Conversely, neglecting data quality can prove costly. Estimates indicate that poor data quality leads to a substantial loss of revenue, anywhere from 20% to 30% of total revenue, arising from inefficient data handling and the flawed insights it produces. This stark reminder emphasizes the importance of prioritizing data quality throughout its entire lifecycle.
Intriguingly, the root of many data quality issues can be traced to human error. Studies show that a significant portion, potentially as high as 90%, of data quality problems originate from manual data entry or processing mistakes. This finding highlights the need for rigorous training programs and the implementation of automated validation checks to improve data integrity.
The implications of data quality extend beyond internal operational efficiency to regulatory compliance. Regulations like GDPR and HIPAA mandate specific data quality controls, and non-compliance can lead to severe consequences, including hefty fines that can run into millions of dollars. These regulations emphasize the intertwining relationship between data quality and regulatory adherence.
The emergence of machine learning has introduced innovative approaches to data quality control. Organizations using machine learning for data quality find that these AI-powered systems can spot anomalies up to ten times faster than traditional methods. This illustrates the potential for technological advancements to reshape the landscape of data quality control.
Cultivating a culture of data awareness among employees can also play a critical role in enhancing data quality. Businesses that prioritize regular training on data management best practices have observed a noticeable decrease in data-related errors—around 50%. This suggests that encouraging a widespread data-centric mindset among employees can significantly contribute to overall data quality.
In addition to internal efforts, establishing feedback mechanisms where data users can readily report quality concerns can also drive improvements. This approach allows data consumers to flag potential issues, leading to a 20-25% increase in accuracy. Such a participative approach promotes a shared sense of ownership and responsibility in data management, leading to enhanced data reliability.
Integrating data quality checks seamlessly into operational workflows can also streamline the process and lead to better outcomes. Organizations that integrate these checks into existing processes are more likely to achieve their data governance objectives, by roughly 30%. This seamless integration helps ensure ongoing monitoring of data quality without disrupting routine operations.
Furthermore, there's a direct correlation between robust data quality metrics and overall organizational performance. Companies that regularly track and analyze data quality metrics tend to see a higher return on investment—up to 10% more than those who do not. These metrics serve as crucial indicators of data quality, providing insights that drive informed decision-making and strategy development.
Lastly, pursuing a continuous improvement model for data quality provides a path towards sustained, ongoing enhancement. Regularly reviewing and updating quality control mechanisms, adapting to changing circumstances and learning from past experiences, can lead to compounding improvements over time. This approach not only benefits data quality but also enhances the decision-making processes that rely upon this data.
It’s clear that the success of data-driven decision-making hinges not just on the availability of data, but also on its quality. Ensuring data quality is no longer a secondary consideration but a crucial element of a robust data governance framework, essential for organizational success in the age of digital transformation.
The Overlooked Role of Data Governance in Digital Transformation Success - Securing Data Assets in a Rapidly Evolving Digital Landscape
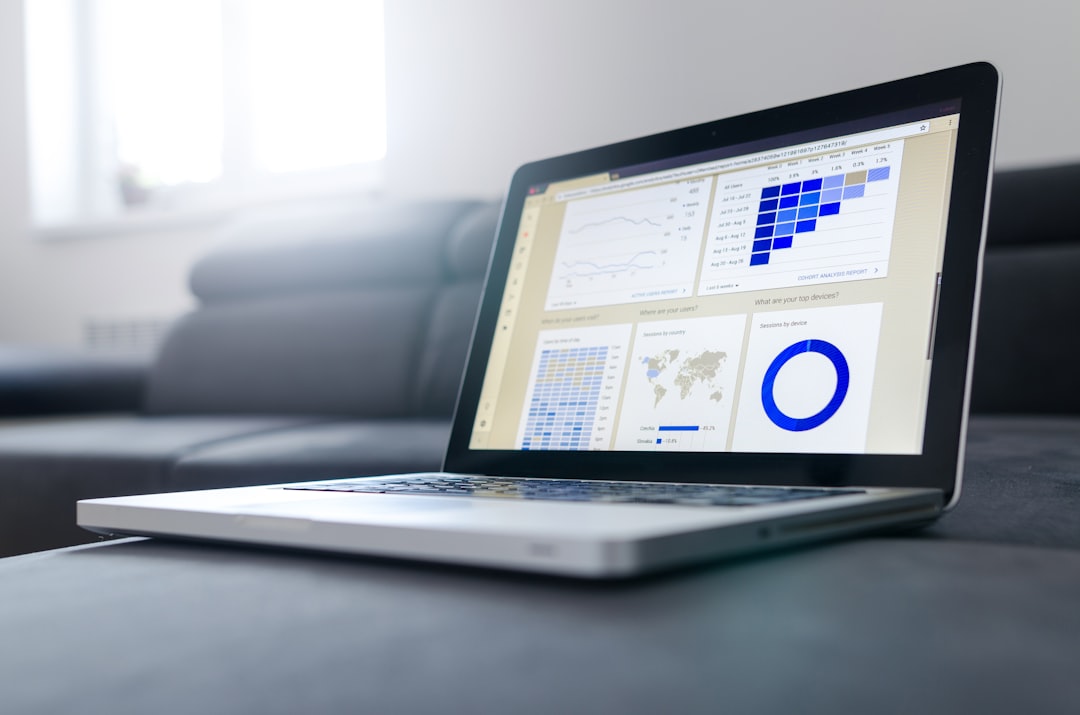
The rapidly changing digital world has made protecting our data a top priority. Businesses are facing more complex cyberattacks and data breaches because of how much they rely on technology in nearly every part of their operations. This requires cybersecurity approaches that adapt to how data and software are used. Data governance, often overlooked, becomes crucial here, giving us the structure we need to manage risks, meet legal rules, and ensure reliable information. It's important for companies to be flexible and include solid data governance as part of their cybersecurity plans. This joined-up approach not only protects sensitive information but also helps with digital transformation goals. Combining data governance and cybersecurity is vital in today's complex data world and helps businesses stay competitive as things change quickly.
The ever-increasing reliance on digital technologies has significantly amplified the importance of safeguarding data assets. It's become evident that traditional cybersecurity approaches are often insufficient in the face of rapidly evolving data usage and software trends. This calls for a shift in how we approach security, constantly adapting to the changing landscape.
Organizations are becoming increasingly vulnerable to cyberattacks and data breaches due to their widespread dependence on IT systems, impacting nearly every operational aspect. This trend highlights the growing need for proactive security measures and robust data management strategies.
While digital transformation initiatives aim for innovation, data governance often gets overlooked. Its critical role in achieving digital transformation success can't be emphasized enough. Having strong data management practices isn't merely advisable, it's essential to build a resilient, compliant, and trustworthy data environment.
Cybersecurity strategies need to evolve in parallel with the digital landscape. The threat environment is constantly changing, meaning that outdated approaches will not provide adequate protection. It's critical that organizations have a thorough understanding of emerging threats to implement effective security controls.
Data protection demands are increasingly driven by financially motivated attacks, such as email account compromises. These types of attacks underscore the fragility of many existing security practices and the significant risks they pose to organizations and their data management processes.
The area of data security and privacy is in constant flux. This dynamic necessitates that organizations take a proactive approach to data governance. They need to be aware of changes in the regulatory landscape and anticipate potential issues proactively to remain compliant and competitive.
Effective data governance frameworks are crucial for minimizing the risk of data security breaches and ensuring compliance. By providing a structured approach to data management, governance frameworks promote consistency in data handling practices and improve a company's overall security posture.
Integrating cybersecurity and data protection measures directly into a business's core strategy is vital to achieving the intended outcomes of digital transformation initiatives. Too often, security is seen as an afterthought rather than an integral part of business operations.
Organizations need to grasp the influences shaping the cybersecurity landscape and adapt accordingly in light of the accelerated pace of digital transformation. Many security leaders are finding it difficult to keep up with the rapid changes in threat landscapes and it's increasingly difficult to find talented cybersecurity professionals with the needed experience.
Ultimately, the path to securing data assets in this rapidly changing environment lies in the convergence of innovative data security practices and strong data governance principles. It requires a shift in mindset from seeing data protection as an afterthought to integrating it into every stage of the digital transformation process.
The Overlooked Role of Data Governance in Digital Transformation Success - Leadership's Role in Championing Data Governance Initiatives
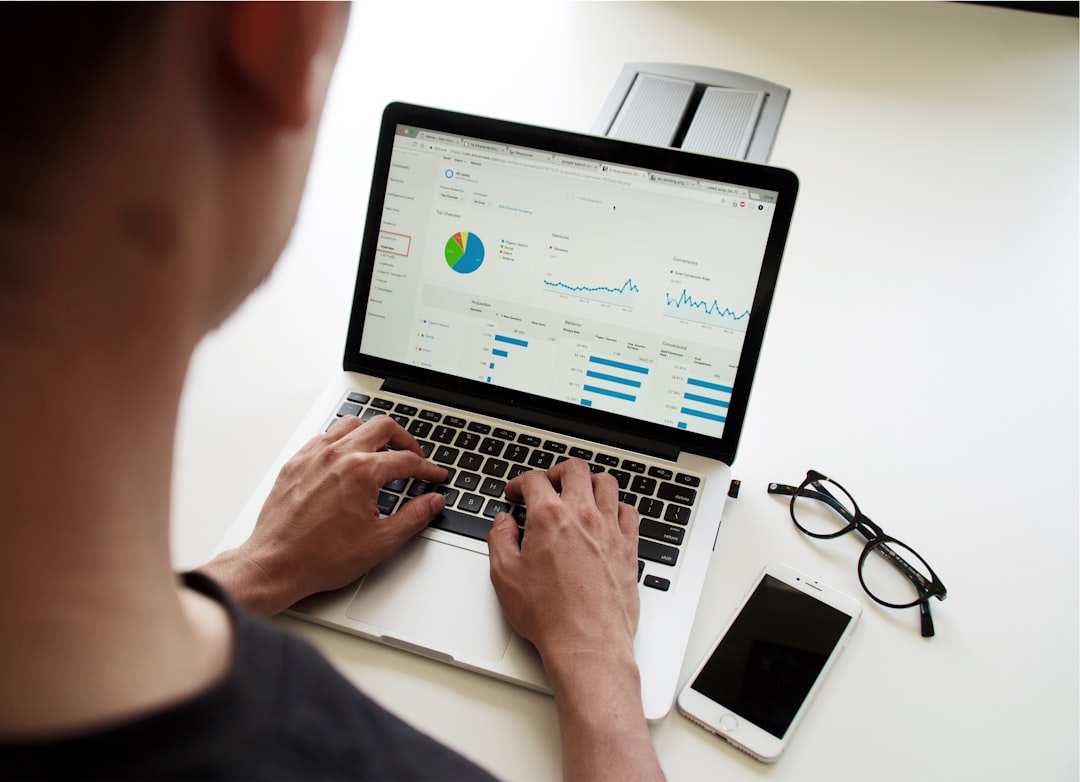
Leadership plays a vital role in driving data governance initiatives. They are, in essence, responsible for establishing a company culture that values data as a critical asset. For data governance programs to succeed, they need strong backing from leadership, especially at the highest levels. Executive leaders must define a clear vision and strategy for data governance, ensuring it's aligned with the organization's overall mission and strategic goals. It's also essential for leaders to proactively engage with the C-suite to understand their specific data challenges and needs. This engagement not only helps solve problems, but it also fosters a sense of responsibility amongst executives to champion data governance. In addition, selecting the right people for crucial data governance roles is critical for maintaining the integrity of the data management process. Leadership's involvement goes beyond simply giving the green light; it involves creating an environment where effective data governance can flourish. This, in turn, supports the organization's broader efforts in digital transformation.
Leadership plays a crucial role in fostering a data-centric culture and driving the success of data governance initiatives. When leaders prioritize data as a key asset, they establish a foundation for improved data quality and compliance. The effectiveness of a data governance program often hinges on the commitment of the person in charge, who is ultimately responsible for ensuring that data management practices align with the organization's strategic goals and risk mitigation strategies.
Securing buy-in from leadership, especially the C-suite, is essential for successful data governance implementation. Engaging with executive leadership to understand their needs and existing data challenges is the first step. Leaders need to clearly articulate a data governance vision and strategy that aligns with the organization's overall mission and goals. This leadership endorsement extends beyond mere budget approvals; it demands active advocacy and promotion of data governance principles.
A well-structured data governance program safeguards organizations from data breaches while supporting the achievement of shared objectives. It establishes trust in the data, guaranteeing reliability and integrity through the identification of trustworthy data sources and robust verification procedures. Given the increasing value of data as an asset in today's digital landscape, effective data governance directly impacts the success of digital transformation efforts.
However, the importance of carefully selecting individuals for key roles within the data governance framework cannot be overstated. Appointing the wrong people can hinder the progress of initiatives, underscoring the need to develop a skilled and experienced team from the very beginning. Effective data governance relies on adherence to best practices, including ensuring data quality, compliance, and security, all of which are critical for realizing organizational objectives. These best practices are instrumental in building a data infrastructure that supports business goals and mitigates potential risks.
While the benefits are clear, organizations often face obstacles in implementation. One concern researchers frequently encounter is the difficulty in integrating data across different platforms. The complexities involved in managing and integrating data from multiple systems, as well as the potential roadblocks in adopting new technologies, such as machine learning, can hinder the successful completion of digital transformation initiatives. Addressing these issues effectively can unlock the full potential of data governance for organizational success.
The Overlooked Role of Data Governance in Digital Transformation Success - Automated Metadata Management Enhancing Organizational Efficiency
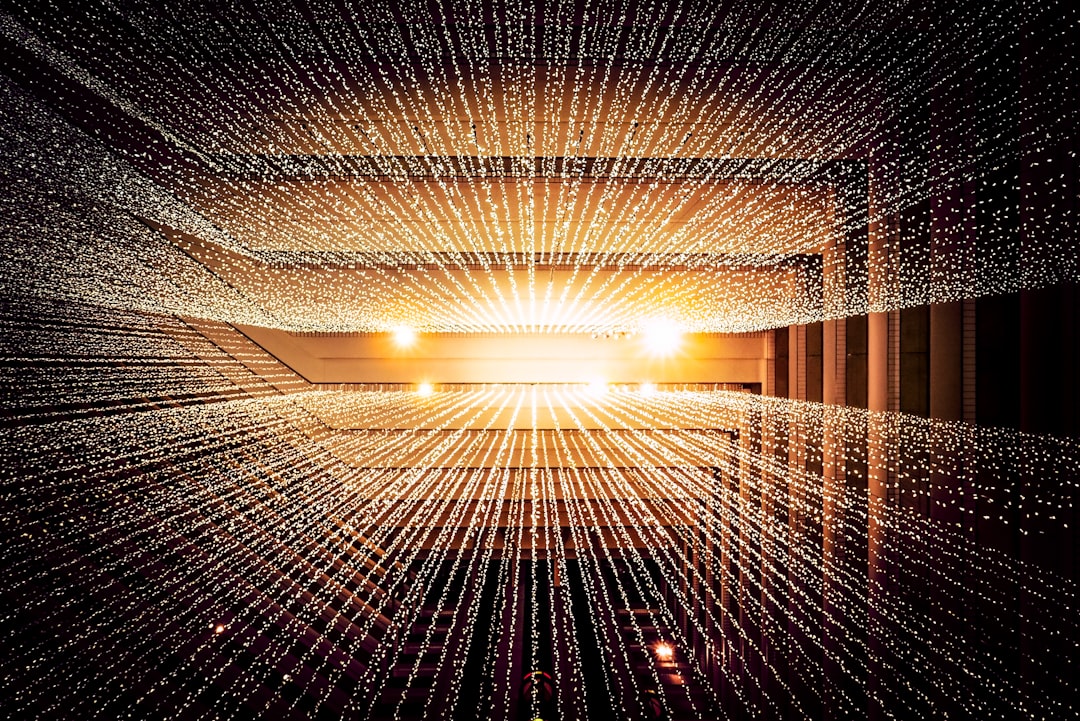
Automated metadata management is changing how organizations manage their data, particularly in the context of digital transformation and the often-overlooked role of data governance. Automating metadata management brings about a level of consistency in how metadata standards are used, which improves data quality and makes sure that data is accurate and reliable. The tools used for automated metadata management allow for real-time updates, ensuring that the metadata accurately reflects the changes that are happening within the data itself. Data governance provides a framework that is essential in this process. It establishes policies and procedures that are crucial for protecting data integrity and maintaining compliance with regulations. The interaction of automated metadata management and a comprehensive data governance strategy is key to ensuring that organizations can effectively leverage their data. This enhanced ability to use data improves decision-making and drives gains in operational efficiency. With the rapid pace of digital transformation, incorporating automated metadata management into an organization's data governance approach is crucial for success. Successfully managing and understanding the complexity of data is a necessary part of being successful in the current technological environment.
Automated metadata management offers a compelling approach to improving how organizations handle their data assets. Automating the management of metadata significantly improves data quality and consistency because it ensures metadata standards are applied uniformly across all data sources within an organization. Automated systems also help maintain data accuracy in real-time by automatically updating metadata whenever the underlying data changes.
It's becoming increasingly clear that a strong data governance model is essential for organizations to effectively manage data. This often requires executive leadership to push these data governance efforts. This connection between data governance and metadata management is crucial for success in today's data-driven environments. The idea behind Big Data Governance and Metadata Management (BDGMM) is to handle the challenges posed by massive amounts of data in diverse datasets. It aims to make data easier to find, use, and reuse.
Data governance policies are enforced through careful metadata management. This enforcement is critical to ensure compliance with regulations and protect the reliability of data. The relationship between metadata management and data governance is foundational to achieving the full potential of an organization's data assets. We see in many organizations, a focus on digital transformation strategies that aim to leverage data in decision-making.
Automated metadata management empowers organizations to improve how they make decisions and makes their operations more efficient. But, as with other tools, there are some notable challenges. One common issue is ensuring that data from different systems can be readily used together—data interoperability. Many organizations struggle with integrating different data platforms or systems due to disparate formats and approaches. It's estimated that as many as 80% of organizations experience significant interoperability difficulties. If automated metadata management systems are unable to address these challenges, the advantages of automation may be diminished. However, with well-designed implementations of automation, we see substantial reductions in time spent on manual tasks, up to 40%. This time saving allows teams to focus on strategic decisions instead of simply moving data around.
It's interesting that metadata itself has become a valuable asset, as studies suggest it contributes to as much as 30% of the overall value derived from data systems. This value can stem from its role in improving decision-making processes. Furthermore, automated metadata management leads to greater efficiency in compliance-related tasks, with some organizations reporting a decrease of up to 60% in the time and effort needed to meet regulatory requirements. This can be quite helpful in managing the growing number of data regulations.
Also, employee productivity has shown to increase with the implementation of automation systems in this area, with studies showing a roughly 25% boost in efficiency. The reason for this improvement seems to be that employees can spend less time handling basic data processes, leaving more time for analysis, problem solving, and other more complex tasks. A key reason for these efficiencies is the reduction of data entry errors. Manual data entry can create errors exceeding 30% of the time. Automating metadata management has the ability to minimize this.
Organizations are looking into how to incorporate AI and machine learning into their metadata management processes. The combination can greatly speed up the processes involved in using AI models, potentially reducing the amount of time to implement these technologies by as much as 50%. This type of benefit could help an organization adapt quickly to market changes. Over time, automated systems also result in significant savings in data storage costs (up to 20%). Furthermore, an organization's data governance maturity is estimated to improve 2 to 3 times faster when they utilize automated metadata management, leading to better data ownership and accountability.
These examples help to demonstrate that these automated approaches can improve organizational efficiency and adaptability. As digital transformation initiatives continue, these methods will become even more important to handling vast quantities of data.
More Posts from :