The Evolution of Knowledge Bases From 1950s Card Catalogs to Modern AI-Driven Repositories
The Evolution of Knowledge Bases From 1950s Card Catalogs to Modern AI-Driven Repositories - Card Catalogs Transform Library Science 1950s Manual Filing System Modernizes
During the 1950s, card catalogs significantly reshaped library science by evolving from a basic manual filing method to a more structured system for organizing information. Initially, they were primarily tools for library staff, but they blossomed into a comprehensive method for cataloging the entire library collection. However, the advancement of technology eventually challenged the traditional card catalog. The adoption of MARC standards in the 1970s ushered in a new era of computer-based cataloging, underscoring the growing need for streamlined and accessible library services. While many people hold fond memories of the traditional card catalog, its eventual replacement with digital systems irrevocably altered how users interacted with library materials. Libraries today rely on AI-driven repositories, symbolizing a new era in knowledge management that values both efficiency and the user experience. This transition reflects a fundamental shift in how we organize and access knowledge within libraries.
In the mid-20th century, the landscape of library science was still largely defined by the card catalog. While functional, this manual system was a behemoth—requiring vast storage and a significant human effort for upkeep and retrieval. The inherent nature of manually organized cards made errors and misplacement more frequent.
This cumbersome approach became increasingly inefficient as libraries expanded their holdings. Fortunately, the 1950s and 60s witnessed a confluence of advancements, including novel indexing approaches and the burgeoning field of computing. This set the stage for a shift towards faster and more scalable information retrieval, helping libraries manage increasingly large collections.
The path towards digital libraries took shape in the late 1960s and early 1970s with early automated systems using rudimentary technologies like punched cards and magnetic tapes. While pioneering, these early systems presented new challenges—particularly in the need to train library staff in unfamiliar technologies. Many librarians were initially unfamiliar with the nuances of managing computerized systems.
Interestingly, the structure of early card catalogs subtly influenced the foundational design of today's digital databases. Hierarchical, network, and relational database models, which became dominant later, were, in part, responses to the organizational problems solved by the card catalog method.
A pivotal shift occurred in 1971 with the introduction of Cataloging-in-Publication (CIP). This initiative transferred much of the bibliographic record creation responsibility from libraries to publishers, enabling libraries to prepare their catalogs prior to receiving physical books.
The 1970s marked a critical stage as libraries began migrating to digital systems and developed the first user interfaces for catalogs. These rudimentary systems, known as public access catalogs, empowered patrons to independently search library collections.
The transition from analog to digital ushered in an era of change, prompting concerns about the preservation of historical materials. Libraries were forced to confront the complexities of digitization and rethink their archiving strategies to ensure their collections endure.
By the late 1980s, the field had matured and online public access catalogs (OPACs) gained widespread use, dramatically changing how patrons interacted with resources. OPACs made it possible for individuals to search collections from anywhere with an internet connection.
The movement from physical catalogs to electronic databases is more than a technological upgrade. It’s a philosophical shift in how we conceptualize access to information. The traditional focus on the physical organization of knowledge has been replaced by an emphasis on efficient retrieval and user-centric services.
The Evolution of Knowledge Bases From 1950s Card Catalogs to Modern AI-Driven Repositories - Digital Revolution 1980s Libraries Launch First Electronic Database Systems
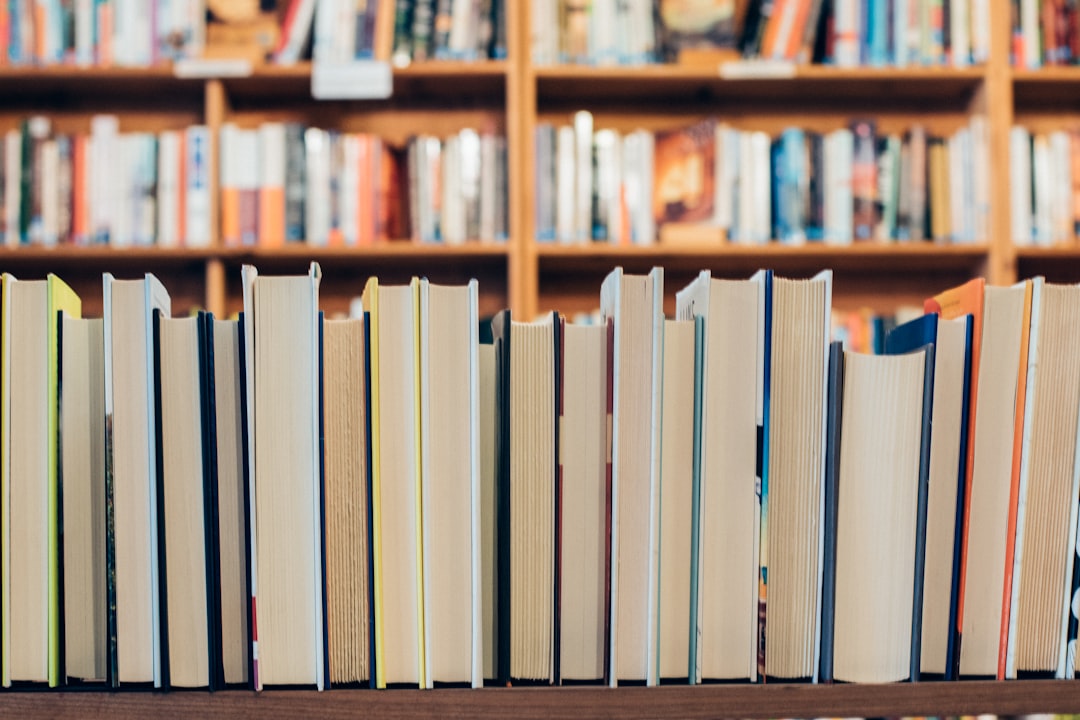
The 1980s witnessed a pivotal moment in library history with the introduction of the first electronic database systems. This marked a significant shift away from the traditional card catalog, ushering in the digital revolution within libraries. These early electronic systems were more than just digital replacements for card catalogs; they fostered new ways of discovering information. Libraries moved beyond basic electronic repositories, embracing multimedia and interactive features that enriched the user experience.
However, the rapid growth of global publishing and the increasing volume of information presented challenges. No single library could realistically aim to comprehensively capture and manage the expanding world of knowledge. This necessitated a change in approach, pushing libraries to collaborate and share resources more effectively.
The changing landscape of libraries also brought about a shift in the types of professionals needed. Beyond traditional roles, libraries began to incorporate staff with expertise in areas like data analysis and database management. This reflected a broader transformation of libraries from primarily being repositories of physical books to becoming active participants in managing and disseminating digital information. This period laid the groundwork for the convenient, accessible, and user-focused digital libraries that are commonplace today, highlighting a dramatic transformation in how we interact with knowledge.
The 1980s marked a pivotal moment in library history with the introduction of the first electronic database systems. This shift from traditional card catalogs represented a significant step toward a future where knowledge access would be fundamentally different. Early electronic systems were, in many ways, a glimpse into the future of AI-driven information retrieval. They utilized intricate algorithms, foreshadowing the more advanced AI systems we see today. Systems like MEDLINE, developed by the National Library of Medicine, demonstrated the power of these early systems to efficiently process and retrieve information, especially within specific fields like medicine, which helped set the stage for specialized databases across various disciplines.
However, the transition to digital systems wasn't without its hurdles. Libraries faced considerable financial challenges in the 1980s, with the initial implementation costs of these systems often reaching hundreds of thousands of dollars. This economic barrier restricted the adoption of these systems by smaller libraries, highlighting an issue that persists in various aspects of digital transformation. The user experience itself was quite different from today's interfaces. Early systems were often text-based and required familiarity with command line interactions, a stark contrast to the visually intuitive interfaces that are commonplace today. The need to adapt to the new technology also created challenges for both librarians and library patrons, prompting a significant effort in user training and the development of materials to help navigate the new systems.
Beyond operational aspects, this transition brought about new concerns regarding data security and the safeguarding of sensitive information. In a pre-cybersecurity era, the shift to storing information electronically created a fresh set of vulnerabilities. Furthermore, the reliance on mainframe computers for these systems also highlighted a central processing approach, a far cry from the distributed cloud-based systems of today.
This period also saw the development of data exchange protocols like Z39.50. These standards allowed different library systems to interact and share data, creating a foundation for the interconnected library networks we use today. While many initially believed that digital systems would streamline operations, they actually created new layers of complexity related to data maintenance, software updates, and training. These are challenges that continue to shape library operations even today.
The advent of electronic databases had a profound impact on the role of librarians, shifting their responsibilities from being primarily focused on cataloging physical materials to also incorporating user instruction and technology management. It's fascinating to see how these early digital systems, while crude compared to what we experience today, were a fundamental step in the broader evolution of how knowledge is accessed, managed, and shared. While the transition was far from seamless, it fundamentally reshaped libraries, laying the groundwork for the sophisticated digital libraries and AI-driven repositories that exist today.
The Evolution of Knowledge Bases From 1950s Card Catalogs to Modern AI-Driven Repositories - MARC Format 1965 Standardizes Machine Readable Library Records
In 1965, the Library of Congress introduced the MARC (Machine-Readable Cataloging) format, a pivotal development aimed at creating a standardized way to represent library records in a format computers could understand. This initiative started with a test project called MARC I, exploring the practicality of converting catalog information into a machine-readable form. The successful outcome of MARC I led to the development of MARC II, with the Library of Congress taking on the role of distributing these standardized cataloging records. This standardization greatly simplified the process of sharing and accessing library data across institutions. The MARC format facilitated the move from hand-written library records to computerized catalog systems. This was a crucial step toward the smooth and efficient access to bibliographic data that defines modern digital libraries and the current generation of AI-powered information hubs.
The MARC format, introduced in 1965 by the Library of Congress, represented a pioneering attempt to standardize how bibliographic information was encoded. This was a dramatic departure from the traditional, manually-driven card catalog system, which prioritized physical organization over a format that machines could easily read and process. It was a bold step towards creating a standardized system that could potentially facilitate the sharing of catalog records between institutions, a concept that had the potential to make interlibrary cooperation and resource sharing more seamless.
However, the initial adoption of MARC wasn't universally accepted. Many librarians were hesitant, fearing it would add unnecessary complexities to their work and potentially diminish their role in the cataloging process. They were understandably concerned about the introduction of a new technology that could upend their well-established workflows.
MARC's structure is based on fields and subfields, allowing for quite a bit of detail in capturing bibliographic information. This flexibility proved important as libraries faced the growing need to adapt to the evolving world of digital information and resources, helping to facilitate the transition into new digital formats.
Though MARC facilitated substantial advancements in library cataloging, it has also attracted criticism for its perceived complexity. Many new librarians entering the field have struggled to understand its intricacies, sometimes resulting in a noticeable gap in knowledge amongst library professionals. It's a situation where a powerful tool hasn't always been fully adopted or utilized because of its challenging nature.
The design of MARC has been influential, shaping metadata standards across various areas and paving the way for future information retrieval systems. Yet, its inherent complexity has, to some degree, slowed the adoption of the standard in certain digital library efforts. It remains a question if the challenges posed by MARC are outweighing the benefits it provides to certain libraries.
One interesting element of MARC is the use of "indicators," two-character fields used to add context to data. This allows for more precise indexing than simple categories might offer, but it adds another layer of complexity to the system.
It's important to remember that the foundation of MARC stems from the 1960s, a time when the world of information was primarily based on print materials. Consequently, the standard has encountered limitations when it comes to handling modern digital and multimedia resources that have become commonplace in libraries. One might wonder if the system has aged and requires a rethinking of its core foundations.
The MARC format has evolved over time, with revisions like MARC21 attempting to stay current with technology and user demands. This demonstrates a degree of adaptability, but it also highlights the continuous evolution needed to remain relevant.
It's fascinating that, despite being designed for improved machine readability, many libraries continue to rely on MARC, even as they integrate newer systems. This demonstrates the inherent resistance to change sometimes found within organizations, even when faced with more modern, potentially more effective alternatives. It's a reminder that technological adoption, particularly in established institutions, can sometimes be a slow and complex process.
The Evolution of Knowledge Bases From 1950s Card Catalogs to Modern AI-Driven Repositories - Online Public Access Catalogs 1995 Replace Physical Card Systems
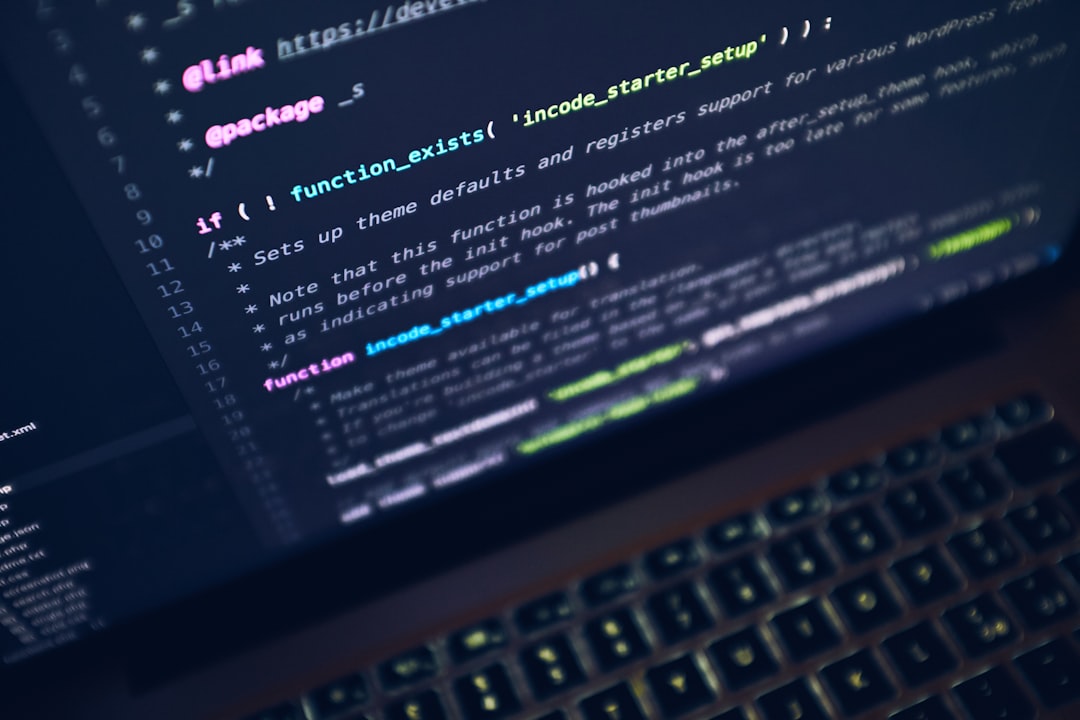
By the mid-1990s, the familiar landscape of libraries began to change with the widespread adoption of Online Public Access Catalogs (OPACs). These digital systems replaced the cumbersome card catalog systems that had been the mainstay of libraries for decades. This shift was fueled by advances in computer technology and increased internet access, which made it possible to create searchable databases of library resources. OPACs, in their early forms, retained the structure of the card catalogs they superseded, offering users familiar search methods based on pre-defined indexes. However, they also incorporated features that enhanced the user experience, like readily available tables of contents and expanded search capabilities.
Though some library patrons may hold a sentimental attachment to the old card catalog systems, the efficiency and accessibility offered by OPACs proved compelling. This transition reflects a larger movement towards digitizing information and streamlining access to it. Libraries, in adopting these new technologies, clearly prioritized improved user experience and easier access to their collections. This move towards digital systems highlights a fundamental shift in how we organize and share knowledge, showcasing libraries' ongoing effort to adapt to the evolving needs of their communities in an increasingly digital age.
By the mid-1990s, online public access catalogs (OPACs) were starting to replace the traditional physical card catalogs in libraries. This transition represented a significant shift towards making library resources more readily available to a wider audience. The move was fueled by the technological advancements of the 1980s, including better computers and easier network access.
Interestingly, the early OPAC systems kept a structure much like the card catalogs they were replacing. Users could search using pre-defined indexing methods, just as they had before. One of the first libraries to venture into this new realm was Ohio State University, which launched its OPAC in 1975, followed by the Dallas Public Library in 1978. These early adopters essentially took all the information from the card catalog and put it into a computer, adding extra features like showing tables of contents for books.
The groundwork for this change was laid with the introduction of the MARC (Machine-Readable Cataloging) format back in 1968. MARC's goal was to make it easier to share catalog information and served as the basis for building these computerized systems. By the early 2000s, libraries of all sizes were moving towards OPACs, reflecting a broader trend of making information more digitally accessible.
OPACs and similar information retrieval systems illustrate how our methods of accessing knowledge have evolved. They've gone from relying on librarians' mental lists to heavily automated systems. While some folks miss the old card catalogs, fewer people seem to miss the even older technologies that OPACs replaced. The whole process of changing library catalogs from physical cards to online databases really shows how libraries have fundamentally changed their approach to organizing and sharing knowledge.
It's interesting to ponder the implications of such a radical change. OPACs have allowed for remote access to information but have also introduced new challenges like the need for digital literacy skills and managing the sheer volume of data. The shift in library user behavior from reliance on librarians to independent search has also created new challenges and considerations for libraries and library staff. It's clear that libraries have not only adapted to the technological landscape but also transformed the role of librarians and how people engage with knowledge.
The Evolution of Knowledge Bases From 1950s Card Catalogs to Modern AI-Driven Repositories - Natural Language Processing 2015 Makes Knowledge Base Search More Intuitive
In 2015, Natural Language Processing (NLP) began to significantly impact how we interact with knowledge bases. The incorporation of large language models (LLMs) has allowed for more intuitive searches within these systems. Essentially, users can ask questions in a more natural way, rather than relying on strict keyword searches. This development doesn't replace the traditional structured data found in databases and knowledge graphs, but rather adds a layer of enhanced accessibility.
Interestingly, LLMs have demonstrated the ability to handle relational knowledge – information that links different concepts or entities. This makes it easier to retrieve complex, multi-faceted information from knowledge bases. The adoption of NLP has also opened new paths for innovation, particularly in leveraging open sources of information. Organizations are exploring these opportunities to build more advanced search experiences with a stronger understanding of the user's intent.
Ultimately, as knowledge bases continue to evolve, the integration of NLP appears to be a crucial element in making these valuable resources more accessible and user-friendly. This shift towards more natural, conversational search methods hints at a future where the retrieval of information from complex knowledge repositories becomes more seamless and intuitive. However, there are questions regarding how NLP will deal with bias, ethical use of data and misinformation in complex systems. While the advancements are undeniable, it's important to be aware of the potential downsides.
In 2015, the field of Natural Language Processing (NLP) took a significant leap forward, particularly in how we interact with knowledge bases. Google's introduction of Knowledge Graphs was a prime example, showing how NLP could be used to understand the nuances of a user's search query rather than just relying on basic keyword matching. The goal was to build a system that could connect related concepts, leading to more accurate and insightful results. This development suggested a shift away from the rather rigid structures of traditional databases towards a more intuitive and human-centered approach to accessing information.
This period saw a major focus on improving the contextual understanding of user input. NLP models were getting better at figuring out what a person really meant when they asked a question. This resulted in search engines delivering more relevant results and making the overall search experience much smoother. It was a movement towards truly "semantic search", where the focus is on the meaning of words and concepts rather than simply matching keywords. This change was crucial for enhancing knowledge base interactions, moving beyond basic retrieval to a level where the system could truly understand the user's intent.
The role of machine learning also increased considerably in 2015, within the NLP landscape. Machine learning algorithms proved adept at classifying and retrieving knowledge base entries. A key aspect was the ability for these algorithms to learn from user behavior, which meant they could improve accuracy and efficiency over time, adapting to user needs.
These advancements made it much easier for people to interact with knowledge bases. NLP allowed users to phrase their searches in a natural way, akin to how they would speak. This was a huge benefit for users who weren't necessarily tech-savvy, as it reduced the need to learn complex search syntax. The result was a more inclusive and user-friendly approach to knowledge retrieval.
Another interesting facet was the increase in multilingual search capabilities. The NLP improvements allowed knowledge bases to reach a broader audience by supporting searches in various languages. It also improved the accuracy of language interpretation, which is important for global access to information.
Information extraction, a process of automatically extracting key details from large datasets, saw significant gains. These improvements greatly streamlined the use of knowledge bases. Instead of users having to comb through vast amounts of data, the system could quickly identify and present pertinent facts and details.
Interestingly, this period saw a rise in the use of chatbots within knowledge base interactions. Many organizations adopted intelligent assistants capable of interpreting user queries and delivering relevant information instantly. These systems greatly improved user satisfaction and offered a more streamlined approach to accessing knowledge.
NLP also helped to standardize data across different knowledge bases. This was crucial for reducing inconsistencies and redundancy, making it easier to retrieve relevant information across different sources. By 2015, NLP systems were getting better at understanding synonyms and misspellings, ensuring users could find what they needed regardless of how they phrased their query.
However, the rapid evolution of NLP also raised concerns about privacy and ethics. The ability of these systems to dynamically interpret queries prompted questions about how user data was being collected and utilized. It became important to have discussions about the ethical implications of knowledge management, ensuring that user privacy was protected and data was used responsibly.
Overall, 2015 was a transformative year for how we interact with knowledge bases. The advancements in NLP not only improved search accuracy and efficiency but also made these systems more intuitive and accessible to a wider audience. While the potential benefits of NLP are exciting, it's crucial that the field continually engages in discussions around ethics and privacy, ensuring that these powerful technologies are developed and used responsibly.
The Evolution of Knowledge Bases From 1950s Card Catalogs to Modern AI-Driven Repositories - Graph Databases 2024 Enable Complex Relationship Mapping Across Data Sources
In 2024, graph databases are emerging as a crucial tool for navigating the intricate relationships within modern datasets, especially when those datasets are scattered across multiple sources. Unlike traditional databases with their rigid table structures, graph databases offer a more flexible approach. They use a network of nodes (representing data points) and edges (representing relationships between them). This makes it easier to understand and analyze complex connections within information. This capability is particularly useful for dealing with situations where data is interconnected in various ways and user requests can be quite complex, needing a fast and sophisticated search system. Graph databases integrated with AI can also interpret natural language queries more easily, opening them up to a broader range of users. As organizations strive to gain deeper insights from the massive amount of data available to them, the use of graph databases is expected to expand, likely leading to new ways of interpreting and using the relationships inherent in digital data. There are some potential drawbacks, like a concern that reliance on this type of database could lead to further entrenchment of systems of control over knowledge, which is already a significant concern. Nonetheless, the flexibility and adaptive power of these newer systems represent a potentially impactful advancement in how organizations work with information.
Graph databases are becoming increasingly prominent in 2024, particularly for situations where mapping intricate relationships across various data sources is crucial. Unlike traditional relational databases, which organize information in rows and columns, graph databases represent data as nodes (entities) and edges (relationships). This structure makes it much easier to visualize and query the interconnectedness of information, which can be invaluable in complex scenarios.
One of the intriguing aspects of graph databases is their ability to provide real-time analytics. Instead of relying on batch processing methods common in relational databases, graph databases can analyze the relationships within data immediately, allowing organizations to make timely decisions. Moreover, the inherent flexibility of graph databases, with their dynamic schemas, allows for the seamless integration of new data types and relationships without requiring extensive system reconfigurations or downtime. This adaptability is a major advantage for organizations constantly dealing with evolving data environments.
The trend toward leveraging diverse data sources is driving the adoption of graph databases. Their design simplifies the aggregation of data from different sources, including unstructured data, making them an attractive option for organizations relying on heterogeneous datasets. This is especially true for businesses seeking to improve data integration and create a unified view of their data assets. It's interesting to note how graph databases have also become quite effective in powering recommendation systems. By meticulously analyzing real-time user behavior, they can provide much more accurate recommendations of products or content.
Another noteworthy trait of graph databases is their scalability. They can efficiently handle enormous amounts of data by scaling horizontally across multiple nodes, making them suitable for large organizations and applications that require distributed data processing. Furthermore, the clear visual representation of relationships in a graph format makes it significantly easier for users to understand and interact with the data. This improved user experience reduces the learning curve, allowing more people to access and analyze complex data sets.
Graph databases are also a good fit for integrating with graph algorithms, leading to unique capabilities. They can easily perform tasks like identifying communities within a dataset, discovering the most efficient path between two points (think optimized route planning or resource allocation), or applying sophisticated algorithms designed to solve complex problems that might be difficult with relational databases. Interestingly, in the long run, implementing graph databases can lead to reduced costs compared to traditional relational database systems, primarily due to faster query performance and the ability to optimize data retrieval. Additionally, the structure of a graph database lends itself to efficiently generating audit trails of data modifications, which is essential for organizations dealing with strict compliance requirements, where maintaining the lineage of data modifications is necessary.
While some challenges might remain regarding certain aspects of performance or complex query optimization for specific tasks, the capabilities of graph databases continue to expand, and they appear poised to play an increasingly vital role in data management and analysis. It remains to be seen if the promises they offer will lead to widespread replacement of relational databases for all purposes, but it's certainly an area worth monitoring.
More Posts from :