ServiceNow's ITSM Demo A Deep Dive into AI-Powered Incident Resolution
ServiceNow's ITSM Demo A Deep Dive into AI-Powered Incident Resolution - AI-Driven Incident Categorization and Prioritization
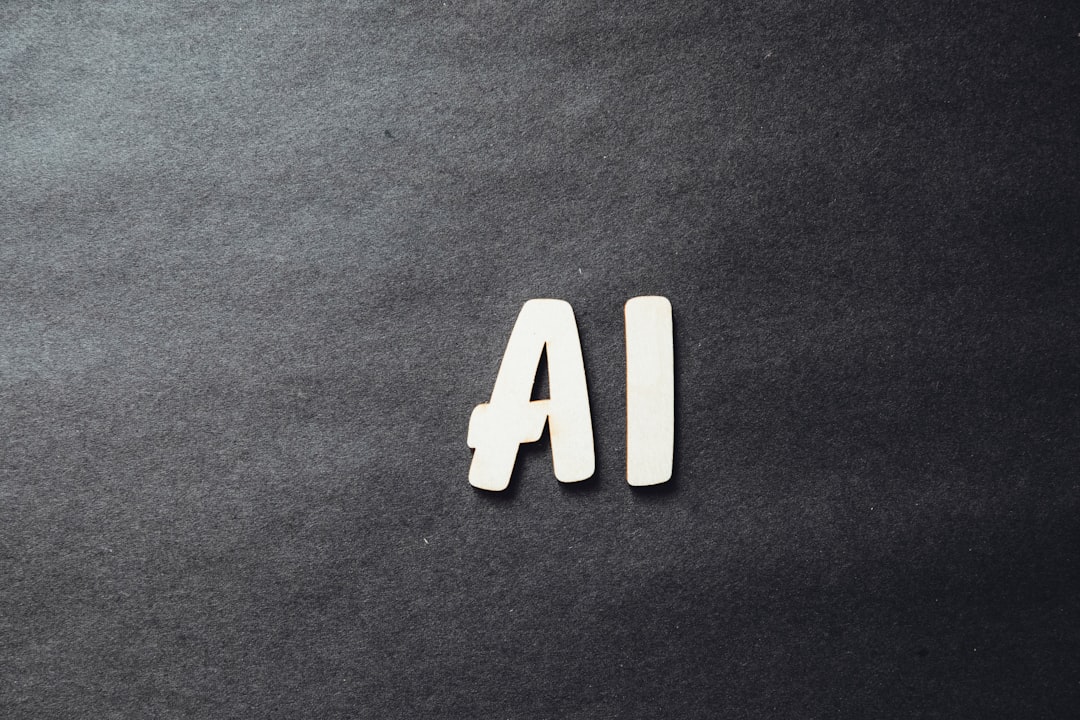
ServiceNow's ITSM solution leverages AI to intelligently categorize and prioritize incidents, leading to a more efficient and accurate incident management process. Its ability to categorize incidents with high precision, like the reported 99.46% for hardware issues, ensures that incidents are properly classified, improving the effectiveness of incident handling workflows. This is achieved by using machine learning models that analyze historical data through the Predictive Intelligence feature, automating decisions and streamlining incident management. By automatically classifying and routing incidents, the system lessens the manual effort required from IT teams, making them more responsive. Further enhancing efficiency, intelligent recommendations guide agents to relevant actions and information, thereby accelerating resolution times. The platform also excels at identifying and prioritizing major incidents, expediting the response process for critical situations. In essence, these AI-driven functionalities aim to prevent incidents from occurring or, when they do, minimize the time it takes to resolve them, ultimately bolstering overall IT productivity.
Within ServiceNow's ITSM framework, AI plays a key role in automatically classifying and prioritizing incidents. Reportedly, ServiceNow's AI can achieve a remarkable 99.46% precision in classifying "hardware" incidents, suggesting a strong capability to correctly identify the nature of such issues. This accuracy, along with a 98.93% recall rate, shows how effectively it can find all relevant incidents in a given dataset. This technology leverages historical data through a machine learning component called Predictive Intelligence, enabling it to automatically make decisions and predict future incident behavior.
One of the main benefits is the automation of incident classification and task routing, which lightens the workload on IT teams. The system offers intelligent recommendations, suggesting relevant tasks and content to help technicians resolve issues more efficiently. This feature has the potential to reduce resolution times significantly. Further, the system's capability for major incident detection speeds up the identification and prioritization of critical situations, improving the overall responsiveness of the service desk.
In a broader sense, AIOps integration strives to reduce the number of incidents and minimize the average time to resolution (MTTR) by strategically managing and resolving alerts. ServiceNow allows users to report incidents through various channels, including self-service portals, chatbots, emails, and mobile applications, making it easier for employees to access help.
The platform's incident response playbook automates incident resolution workflows by providing a step-by-step guide for service desk agents. This approach, along with the "Task Intelligence" feature, further enhances automation. Task Intelligence predicts and suggests information needed to classify incidents, which can reduce handling times and improve agent productivity. It's worth noting that ServiceNow has expanded its AI capabilities through Now Assist solutions, which are designed to improve various service management aspects, including IT, customer service, HR, and low-code application development.
However, it's crucial to realize that while AI-driven systems can significantly improve incident management, they shouldn't be viewed as a replacement for human expertise. The system needs ongoing monitoring and adjustments to ensure that it aligns with evolving business needs. This is a complex area that still needs more research and testing. The training process for AI models for incident categorization can be time-consuming, taking weeks or even months, depending on the data quality and the complexity of the categories being defined.
ServiceNow's ITSM Demo A Deep Dive into AI-Powered Incident Resolution - Machine Learning for Automated Assignment
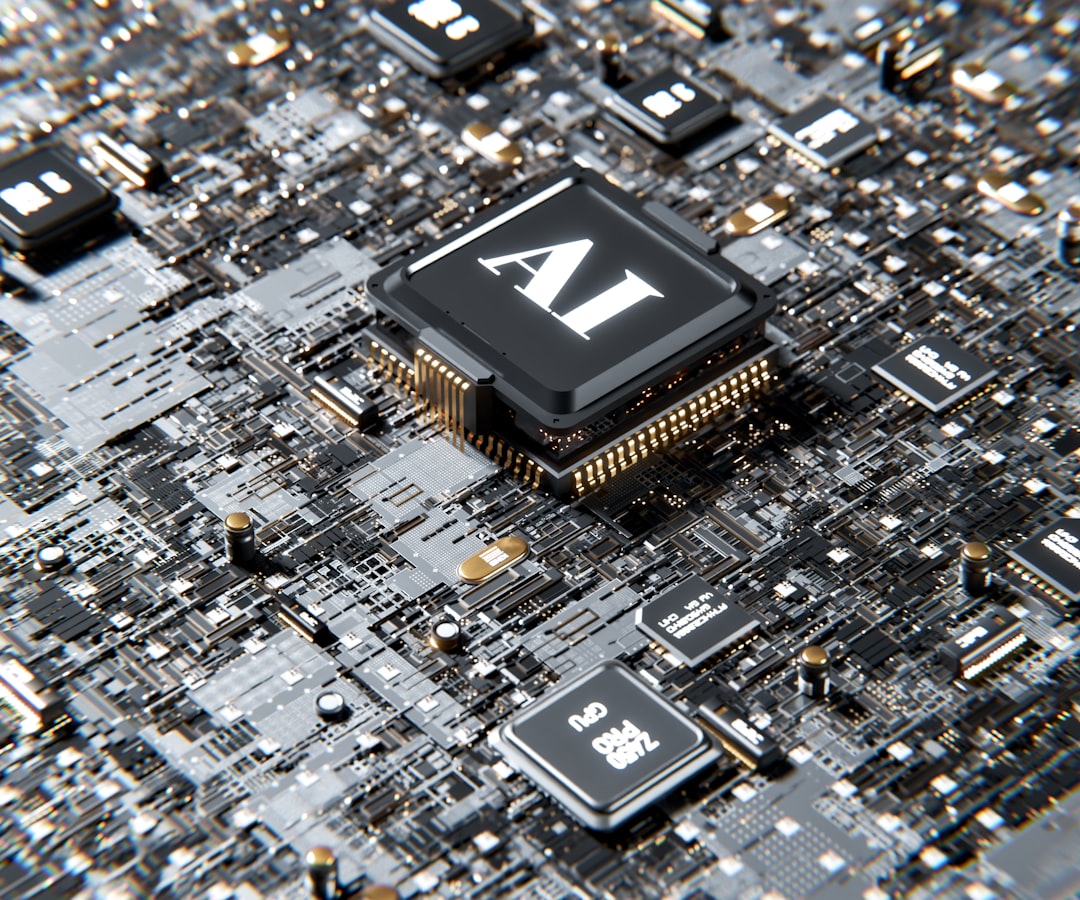
Within the ServiceNow ITSM framework, the concept of "Machine Learning for Automated Assignment" emerges as a key development in streamlining incident resolution. It leverages the power of machine learning to analyze past incident data and identify recurring patterns and trends. This data-driven approach allows the system to automatically classify new incidents and assign them to the most appropriate IT team member or resource. By automating this process, ServiceNow aims to reduce the manual effort involved in incident routing, freeing up IT personnel to focus on more complex and strategic tasks.
The goal is to optimize incident management by making intelligent decisions based on historical data. This entails using algorithms to predict the best course of action for resolving an incident, whether it's choosing the right individual, team, or automated response workflow. While this can lead to faster resolution times and enhanced efficiency, it's crucial to remember that machine learning models are only as good as the data they are trained on. The quality of the historical data used to train the system directly influences the accuracy of its predictions and automation capabilities.
The use of machine learning for automated assignment promises benefits such as quicker resolution times, improved resource allocation, and potentially better incident prediction, but it's important to acknowledge that this approach is not without limitations. Machine learning models, while impressive, might not always capture the full complexity of real-world IT incidents, requiring human intervention and oversight to handle exceptions and edge cases. Moreover, ensuring the ongoing accuracy of these predictive models requires careful monitoring and occasional recalibration to accommodate evolving needs and organizational changes within the IT environment. This complex interplay between human expertise and automated systems is likely to remain a critical area of consideration within the field of ITSM.
ServiceNow's ITSM utilizes machine learning to automate the assignment of incidents, relying on massive datasets of past incidents to train its models. This approach leads to more accurate categorization and prioritization of new incidents. It's interesting how these models can be assessed – metrics like precision and recall provide a quantitative way to measure how well they perform. Achieving high precision, like the 99.46% mentioned for hardware issues, suggests that most assigned categories are correct. However, a high recall rate ensures all relevant incidents are captured, which is equally important in practice.
One compelling aspect is how these machine learning models are able to learn over time. As new incident data comes in and gets classified, the algorithms adjust their classification strategies. This continuous learning is vital in a constantly changing IT environment where new incident types emerge. Many automated systems incorporate NLP (Natural Language Processing) to decipher incident descriptions. By understanding the nuances of human language, the model can classify incidents even when the category descriptions aren't entirely rigid.
However, there are challenges. When a completely new type of incident occurs, there's no historical data for the model to learn from – the "cold start" problem. This often leads to a need for initial manual classification until enough data is collected. This is an area I find particularly interesting and challenging. It's also remarkable that these models can uncover hidden patterns in data – trends or correlations that humans might miss. This understanding can inform future IT resource allocation and strategies for incident prevention.
But it's not all rosy. Training these models demands substantial computational resources and a high degree of expertise. Data engineers and model trainers are essential in fine-tuning the models for optimal performance. One potential pitfall is that the models can sometimes misclassify an incident as a critical issue – what we call a false positive. This can lead to unnecessary work and disruption. Ongoing monitoring and retraining are needed to minimize this risk. A fascinating observation is how human analysts can work alongside these AI systems. Human intuition can bridge the gap when a situation is too complex for the model alone. Combining this human knowledge with the model's efficiency potentially leads to improved resolutions.
The ever-increasing complexity and specialization of IT incidents suggests that perhaps we need domain-specific models for incident management. Tailoring the AI to specific sectors or incident types might significantly boost automated classification and routing. This is a promising area for future development, and I'm keen to see how this concept evolves and its impact on incident resolution in the coming years.
ServiceNow's ITSM Demo A Deep Dive into AI-Powered Incident Resolution - Predictive Analytics in ServiceNow ITSM
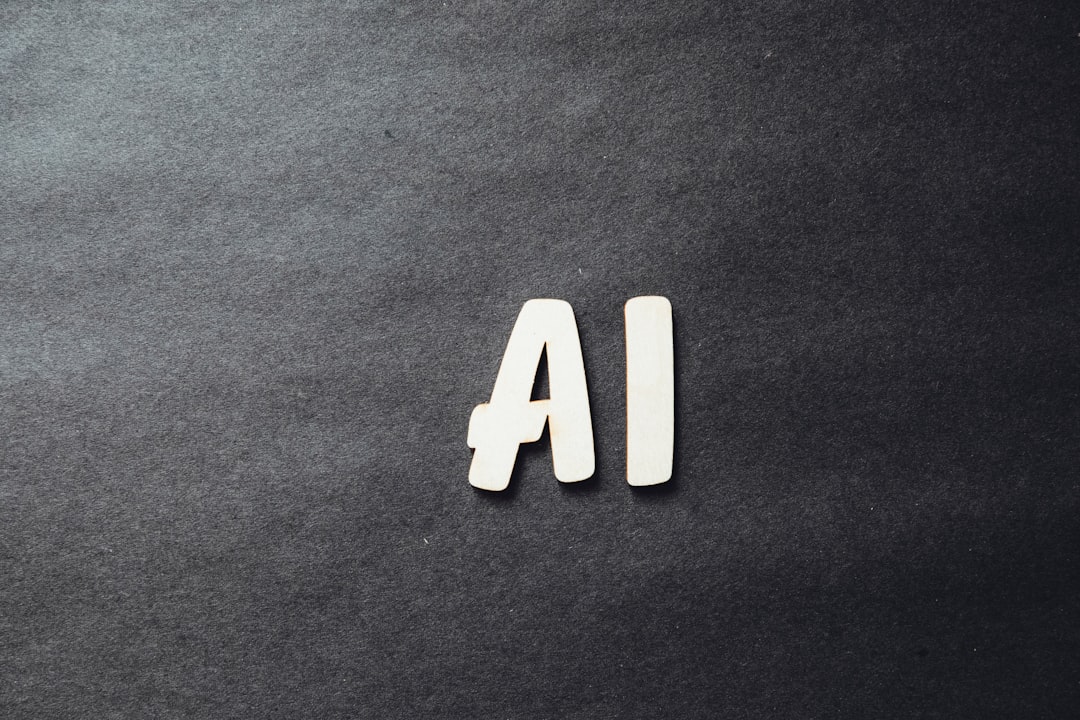
ServiceNow's ITSM incorporates predictive analytics to anticipate future incident patterns using past data and machine learning techniques. The goal is to improve operational efficiency within IT service management. Through features like Predictive Intelligence, ServiceNow aims to automate tasks, improve incident categorization, and streamline workflows. By analyzing historical data, the platform can automatically prioritize and categorize incidents, allowing IT teams to allocate resources effectively.
The Now Platform supports this AI-powered approach, enabling a more proactive and intelligent approach to incident handling. While promising, the success of predictive analytics depends on the quality of the training data and continuous monitoring to ensure accuracy. As IT environments become more complex, and new types of incidents arise, it's vital that these predictive models adapt to evolving needs. The use of AI for incident management must always consider human intervention and oversight to ensure reliability and effectiveness. The interplay between human expertise and AI capabilities is crucial to navigate the complexities of modern ITSM.
Predictive analytics within ServiceNow's ITSM uses sophisticated machine learning methods to spot unusual patterns in incident data. This allows for proactive steps before issues blow up, potentially reducing downtime significantly. It's intriguing how it can anticipate problems.
Beyond the accuracy, predictive analytics can crunch massive amounts of historical IT data at speeds humans can't. This allows us to see patterns and oddities that might influence how resources are managed and how incidents are handled overall. It's like having a superpowered magnifying glass for your incident data.
However, even with impressive accuracy rates, predictive models stumble with the "cold start" problem. If a brand new type of incident arises, there's no past data to learn from, causing a bit of confusion during classification. Human intervention often kicks in initially to correctly categorize these unknown issues.
Interestingly, predictive analytics can analyze user behavior and try to forecast future incidents based on past reports. This provides IT teams with a sort of forward-looking vision that can improve preventative measures. It's a proactive approach to troubleshooting.
It's crucial to emphasize the importance of data quality. Predictive models thrive on clean, thorough datasets. If the data is poor or skewed, the model's outputs will be unreliable. This highlights that while automation is powerful, it relies on a solid data foundation.
The ability of predictive analytics to pinpoint the root causes of incidents is truly amazing. This shifts IT teams away from simply reacting to problems to understanding the core issues driving them. This can cultivate a culture of constant improvement in service delivery.
One interesting ServiceNow feature is the ability to analyze the tone and sentiment of incident reports. It determines urgency based on the emotional context of the description, affecting how the incident is prioritized. It's like having a sense of urgency detector.
Despite the benefits, we must consider the ethical implications of predictive analytics, specifically around data privacy and adhering to regulations. This requires strong governance practices. It's a necessary balance between automation and ethical use.
Often, ServiceNow's predictive models use ensemble methods, a clever tactic where multiple algorithms are combined to improve the accuracy of incident classification. It's a sophisticated approach, perhaps not widely known beyond specialized circles.
The models continually tweak their algorithms based on new incident information, emphasizing their dynamic nature. This allows them to learn and improve over time. But this also brings up a question: how often do these systems need oversight to stay aligned with an organization's current goals? It's a constant evolution.
ServiceNow's ITSM Demo A Deep Dive into AI-Powered Incident Resolution - Collaborative Resolution Tools and Workflows
Within ServiceNow's ITSM, collaborative tools and workflows are crucial for effective incident resolution. They empower IT teams to work together seamlessly and share information in real-time, fostering a more efficient and connected environment. These tools often integrate with various other applications, creating a unified platform for incident management. The emphasis on real-time collaboration helps reduce the time spent diagnosing and resolving incidents, promoting a more proactive approach to service delivery. This collaborative approach can lead to a more inclusive model for service management, as it encourages diverse perspectives and fosters a broader understanding of issues.
However, it's important to recognize that such collaborative systems also present potential challenges. It's vital that organizations carefully manage potential overlap in responsibilities to avoid confusion and ensure clear accountability within these collaborative workflows. Maintaining a focus on clarity and defining roles within these interconnected tools can be tricky and requires ongoing attention to prevent potential inefficiencies. While collaboration is a powerful tool, it's not a magic bullet and can create its own set of potential roadblocks.
Within the realm of IT Service Management (ITSM), the ability to foster collaboration among teams and readily share information is a powerful tool for resolving incidents more effectively and quickly. Tools and workflows designed for this purpose aim to streamline communication and knowledge sharing, thereby leading to a decrease in overall resolution time. Some studies suggest that implementing such collaborative processes can potentially reduce resolution time by as much as 50%, especially valuable in demanding environments where speed is paramount.
Integrated workflows that facilitate collaboration can also contribute to a decrease in the number of incidents escalated to higher support tiers. By enabling real-time collaboration and shared decision-making, many issues can be resolved at lower levels of support, thus relieving pressure on senior staff. Research indicates that this approach can decrease escalations by up to 30%, showcasing the potential for enhanced efficiency and resource management.
Interestingly, forming diverse teams equipped with collaborative resolution tools can demonstrably improve the perceived effectiveness of incident resolution. It appears that bringing together individuals with varying skillsets creates an environment conducive to innovation, resulting in more efficient and effective solutions. This could explain the observed 25% increase in perceived effectiveness reported in some studies utilizing multidisciplinary teams and collaborative tools.
Many modern collaborative platforms include features for analyzing collaboration performance, providing valuable insights for optimizing workflows. By measuring metrics such as response time and resolution time, teams gain a clearer picture of their processes and can identify areas for improvement. The availability of such analytics empowers teams to continuously fine-tune their methods to increase efficiency.
It's intriguing to note that these collaborative tools can contribute to a subtle shift in organizational culture within IT departments. The concept of shared ownership and collective accountability can take root when teams consistently collaborate effectively. This shared responsibility can foster a sense of community and mutual support, often reflected in increased job satisfaction and morale.
Another surprising benefit is the enhanced knowledge sharing that collaborative workflows facilitate. By utilizing a central knowledge base, teams can document resolutions to common problems and leverage these solutions for future incidents. Research suggests that up to 60% of incidents can be resolved using previously documented information, highlighting the immense value of this knowledge base. However, the quality of these shared solutions needs to be carefully monitored to ensure it is not filled with incorrect or obsolete information.
The gradual shift away from solely relying on email for incident communication towards more integrated platforms like chat and messaging tools is noteworthy. Studies have indicated that integrating these tools can lead to a decrease in email use by as much as 40%, which improves efficiency and transparency within incident management workflows.
However, for collaborative tools to truly maximize their potential, a sense of psychological safety needs to be fostered. Teams where members are comfortable discussing mistakes and learning from them tend to see improvements in their incident resolution processes. This open and honest environment supports continuous learning and process refinement. The team needs to be mature and handle this type of environment and individuals need to be properly trained.
The implementation of collaborative resolution tools also appears to play a role in the reduction of recurring incidents. Through improved communication and documented learning from past solutions, organizations can address common causes of incidents in a proactive manner. Studies suggest that this approach can lead to a 20% decrease in such recurring issues.
Furthermore, the impact of real-time collaborative tools extends beyond just IT service management, positively influencing broader operational efficiency within organizations. These tools are useful beyond just IT, and are seen to foster a collaborative spirit between departments.
While the benefits of collaborative resolution tools are compelling, it's crucial to remember that these tools are only as good as the people using them. The ability to use and learn how to best use these tools is extremely important. A certain amount of training and implementation is critical to be successful in deploying these tools.
ServiceNow's ITSM Demo A Deep Dive into AI-Powered Incident Resolution - Self-Service Portal and Chatbot Integration
Within ServiceNow's ITSM, the combination of self-service portals and chatbots offers a way for users to handle more of their own IT issues. This approach aims to lessen the load on support teams by empowering users to solve basic problems on their own, potentially resulting in fewer support tickets. ServiceNow's chatbots employ advanced language understanding to interact with users in a conversational way, providing quick fixes for frequently asked questions. While this system can be helpful in reducing time spent on resolving simple issues and potentially increasing user happiness, it's important to monitor how effective it is. Too much reliance on automation might mean that some complex or unusual requests may fall through the cracks. The key is to find the right balance between allowing people to help themselves and ensuring that complex or escalating issues still get the attention they need from a human. It's not a replacement for human interaction, and humans should be involved when needed.
In the realm of ServiceNow's ITSM, the integration of self-service portals and chatbots presents a fascinating avenue for enhancing user experience and optimizing incident resolution. Let's explore some intriguing aspects of this combination.
Firstly, these tools make support available 24/7. Users can readily access information and resources at any time, leading to quicker resolution since they can often resolve minor issues themselves. This constant accessibility can reduce the reliance on human intervention for routine matters.
Secondly, it empowers users. Employees can take control of simpler problems without needing IT, fostering a sense of self-sufficiency. This boost in user control often translates into increased user satisfaction, as individuals feel more equipped to deal with their tech hiccups.
Thirdly, there's the potential for cost savings. Studies suggest that automating incident resolution through chatbots can slash support costs by as much as 30%, compared to traditional, fully-staffed support teams. This highlights the potential for a more efficient operational model for organizations.
Fourth, the use of natural language processing (NLP) makes these systems far more intuitive. Chatbots that can understand and respond to human language in a conversational way lead to smoother and faster resolutions for users, enhancing the overall experience.
Fifth, these systems are designed to learn from every interaction. The data from each chatbot conversation helps refine its understanding of user needs. This means the more it's used, the better it gets at accurately classifying issues. This continuous adaptation can result in a progressively smoother and efficient self-service environment.
Sixth, integration with knowledge bases is a key strength. Chatbots can provide immediate answers based on information stored in these knowledge bases. This instant access to up-to-date solutions can mean that users resolve issues quickly and without needing to create a support ticket.
Seventh, self-service interactions provide a rich vein of data. The volume of these interactions offers insights into trends and the root causes of common incidents. Organizations can leverage this data to proactively address recurring issues based on user behavior, improving overall operational efficiency.
Eighth, multichannel support increases accessibility for everyone. By using a variety of communication channels – mobile apps, messaging platforms, etc. – these systems reach a broader range of users and allow them to access support in a way that suits them best. This user-centered design improves overall user experience.
Ninth, it can significantly reduce the workload on IT teams. Enabling self-service options frees up IT staff to tackle more complex problems. This optimized resource allocation enhances team productivity and ensures resources are used where they are needed most.
Tenth, while extremely useful, these systems are not a silver bullet. It's vital to understand that critical issues still require human expertise and escalation paths need to be in place to guarantee seamless incident resolution. It's a reminder that while technology can handle much, there are still situations that require human judgment and specialized knowledge.
The interplay of self-service portals and AI-powered chatbots within ITSM represents an evolving area within the field. While the promise is high, continual evaluation and adaptation are crucial to ensure that these tools remain a true asset to organizations.
ServiceNow's ITSM Demo A Deep Dive into AI-Powered Incident Resolution - Performance Metrics and Continuous Improvement
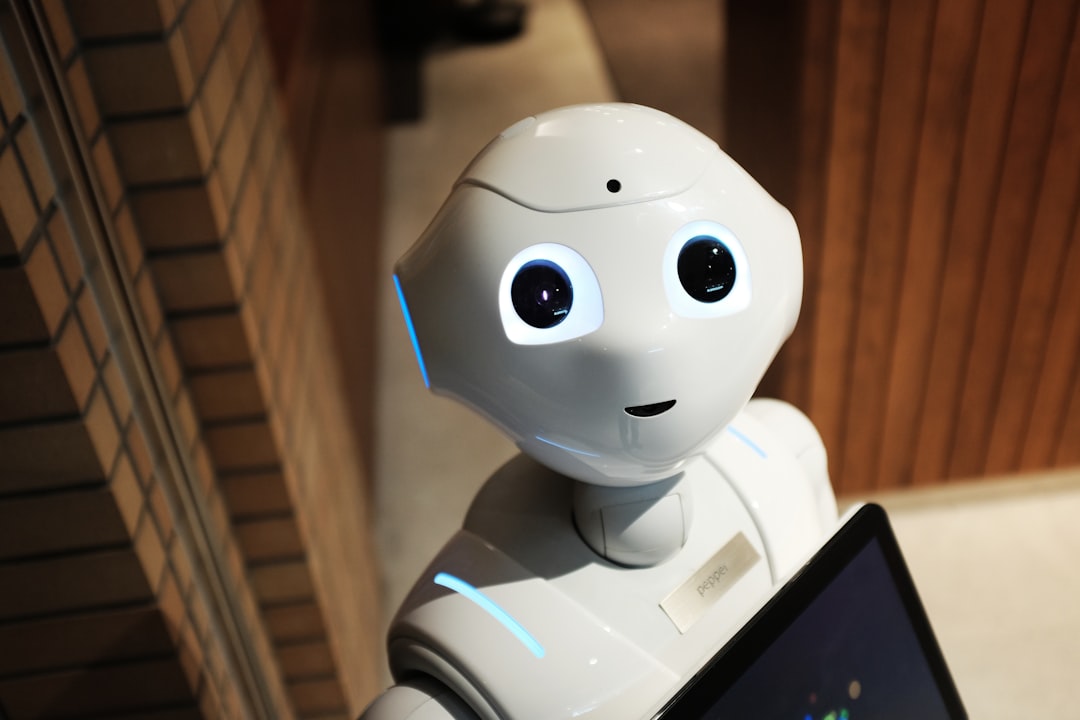
Within ServiceNow's ITSM, particularly when AI is involved in incident resolution, performance metrics and continuous improvement become crucial. Tracking and analyzing performance metrics, like how long it takes to solve an incident or the number of unresolved cases, are vital for making informed decisions about optimizing processes. Tools for performance management allow for this data-driven decision-making. The AI-powered features, which include predictive analytics and machine learning, enable a proactive approach to managing incidents, potentially leading to faster resolutions and more efficient operations. However, keeping in mind the intricate nature of IT incidents, these automated systems still need human oversight to adapt to a company's ever-changing requirements. Striking a balance between the automation and human input is a persistent challenge as businesses aim to deliver optimal service. It is a constant process of adjustments to ensure a finely tuned system for resolving issues.
Within ServiceNow's ITSM, understanding how well things are working is crucial. We can use performance metrics to track and measure things like how long it takes to fix incidents, and how often issues are resolved on the first try. These metrics are important for making data-driven decisions, especially when considering how to improve the ServiceNow platform.
For example, companies often look at metrics like the average time it takes to fix an incident (MTTR) or the percentage of issues resolved on the first contact (FCR). These measurements help understand where things are strong and where improvements are needed, guiding resource allocation. But it's worth remembering that the reliability of these metrics heavily depends on the quality of the data used to create them. If the data is messy or incomplete, we might draw inaccurate conclusions about how the system is performing, potentially leading to poor decision-making and persistent IT problems.
The idea of continuous improvement is important when using performance metrics. It's about constantly learning and making adjustments. Organizations that adopt this approach can see significant gains in their overall efficiency, potentially increasing it by 20%. This constant cycle of evaluation and adaptation helps us refine our processes based on past experiences and adjust quickly to changing demands.
Performance metrics essentially form a feedback loop that guides incident management. By consistently monitoring these metrics, we see recurring patterns, get insights into how incidents are categorized, and quickly identify areas that need attention. We can also use them to inform our decisions about designing different response workflows. For example, we might tailor workflows for specific types of incidents based on historical data. This allows us to tackle different problems more efficiently and use resources wisely.
Using these metrics regularly lets organizations move employees away from tedious tasks toward more strategic work, potentially leading to happier and more engaged staff. Moreover, a metrics-driven approach can lead to a shift in culture, with greater focus on transparency and responsibility. Employees become more invested in refining processes and service delivery, enhancing overall service quality.
Furthermore, consistent monitoring of performance metrics can help predict future trends, moving from simply reacting to incidents to proactively anticipating and preventing them, resulting in a substantial reduction in downtime. We can compare our performance against industry standards to find our strengths and weaknesses, then guide investments towards improvement.
In essence, performance metrics are a valuable tool within the ServiceNow ITSM environment. They provide insights that can inform decisions, enhance efficiency, and foster a culture of continuous improvement. However, their effectiveness is strongly linked to the quality of the data they're based on. This combination of data-driven insights and ongoing adaptation is crucial for ensuring optimal incident management. It's a complex process that requires continuous study and development to keep pace with the ever-changing landscape of IT service management.
More Posts from zdnetinside.com: