RPA in 2024 How Simplified App Development is Reshaping Enterprise Automation
RPA in 2024 How Simplified App Development is Reshaping Enterprise Automation - Low-Code Platforms Fuel RPA Adoption Across Departments
In 2024, low-code platforms are acting as a catalyst for RPA adoption across different departments. Their ability to empower non-programmers to build automation tools is leading to faster development cycles and substantially lower costs. We're seeing a shift towards 'citizen developers', with a wider range of employees taking part in automation efforts. This wider participation makes businesses more adaptable and improves operations. This simpler approach to building automation tools isn't just about making things faster. It also promotes an environment where innovation can thrive. Businesses aren't just automating old ways of working within existing systems; they are using it to rethink their entire processes from start to finish. This combined use of RPA and low-code development tools is significantly altering how companies manage automation, setting the stage for a future where automation is more responsive and adaptable.
It's becoming increasingly clear that low-code platforms are accelerating the adoption of RPA across different parts of organizations. Research suggests that developing RPA solutions using these platforms is considerably faster, potentially up to three times faster than traditional methods, with no-code solutions promising even quicker development for simpler automations. This speed boost translates into tangible cost savings, with reports showing a significant decrease—around 80%—in the costs associated with creating and maintaining automation bots. Examples like the Australian state government's experience, where they saw millions of dollars in savings, illustrate the potential of low-code RPA.
One of the most intriguing aspects is that low-code/no-code tools provided by RPA vendors empower non-technical users to build powerful automation solutions. This means business users can address complex tasks without needing deep coding knowledge. This capability is significant because it allows businesses to reimagine their processes from end-to-end, rather than simply automating tasks within existing systems.
This shift is also impacting organizational structures. Low-code platforms foster a more inclusive approach to automation, encouraging wider employee participation in automation projects. This broader involvement, driven by what we call 'citizen developers', promotes a more agile and responsive approach to automation within companies. It's fascinating to observe how RPA and low-code development complement each other. Together, they contribute to operational efficiency and productivity gains. The increasing adoption of low-code platforms within RPA suggests a trend towards a more democratized approach to automation, with the potential to shift the balance of power from centralized IT to a more distributed, business-driven approach to application development. While the full implications are still unfolding, the rapid growth of this sector is undeniable. There is a sense that by 2024, we may well see low-code approaches become the dominant method for building applications, as organizations look for speed, agility, and adaptability in a rapidly changing landscape.
RPA in 2024 How Simplified App Development is Reshaping Enterprise Automation - AI Integration Enhances RPA Decision-Making Capabilities
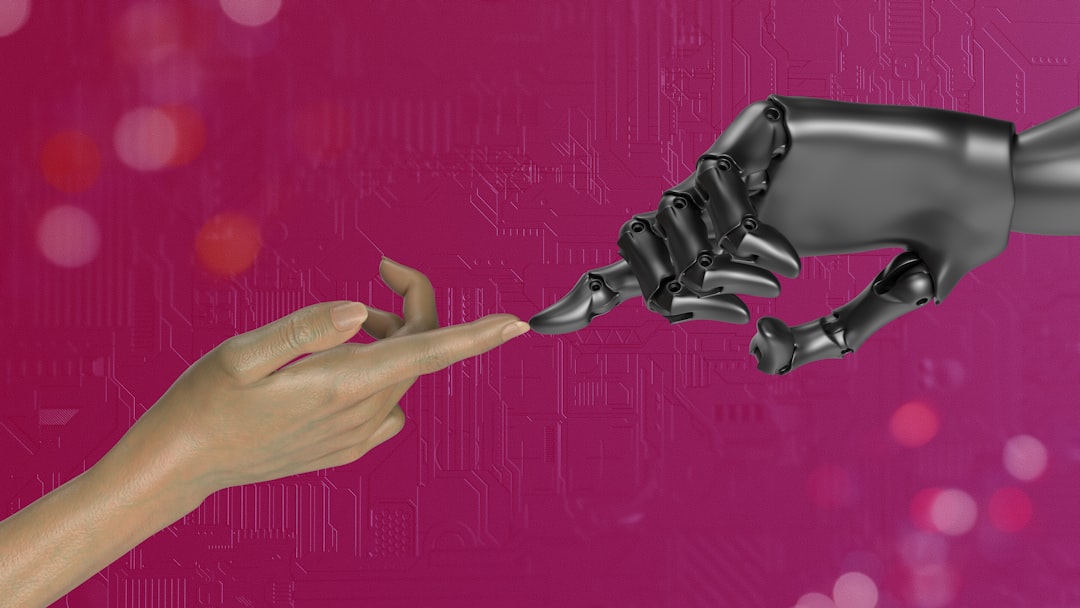
The integration of AI is significantly boosting RPA's ability to make decisions. RPA bots are now capable of handling complex data and using specific business rules to make choices, something that was previously limited. This development is tied to the rise of machine learning automation (MLA), which allows tools to learn from data patterns and make decisions automatically. This is having a noticeable effect in areas like personalized customer experiences in industries like travel.
Looking ahead to the rest of 2024, the convergence of AI and RPA is poised to reshape how businesses automate. We are seeing the development of intelligent systems that can learn and adapt, going beyond basic task automation. This shift makes automation much more dynamic and able to handle a wider range of situations. Businesses can now design automation strategies that are more flexible and resilient to changes in the business landscape, achieving more operational agility and efficiency.
The integration of artificial intelligence (AI) with robotic process automation (RPA) is pushing the boundaries of what automation can achieve, particularly in decision-making. AI, specifically machine learning, allows bots to process information and make choices based on patterns identified in data, going beyond the rigid rules typical of traditional RPA.
This fusion, sometimes referred to as intelligent automation, essentially gives RPA a brain. The bots can learn from the data they process, adapt to changes in business needs, and even personalize user experiences. This learning aspect is pretty interesting, as it means the bots can improve their performance over time, reducing errors and potentially leading to better results than if they were simply following pre-programmed instructions.
It's worth noting that this ability to analyze and learn from data extends to complex decision-making processes, which were previously hard to automate. For example, within travel planning, bots with AI could potentially optimize routes, suggest suitable accommodations, and even predict potential disruptions— all based on learning from past data.
However, just as with any complex system, this integration brings its own set of challenges. Ensuring the AI models are properly trained and maintained requires effort and expertise. If not done well, the accuracy of the decisions made by the bots can be negatively impacted.
It seems that the future of RPA is closely tied to AI. The combination of AI's cognitive abilities and RPA's efficiency creates a potent force for automating even more complex tasks. This integration will likely continue to evolve, with bots becoming more sophisticated in their decision-making. But the need for ongoing human oversight and maintenance of the AI models will likely remain a critical aspect of ensuring their effectiveness. While it’s promising, the complexity of managing these integrated systems is something researchers and businesses should closely consider.
RPA in 2024 How Simplified App Development is Reshaping Enterprise Automation - Process Mining Tools Optimize RPA Implementation
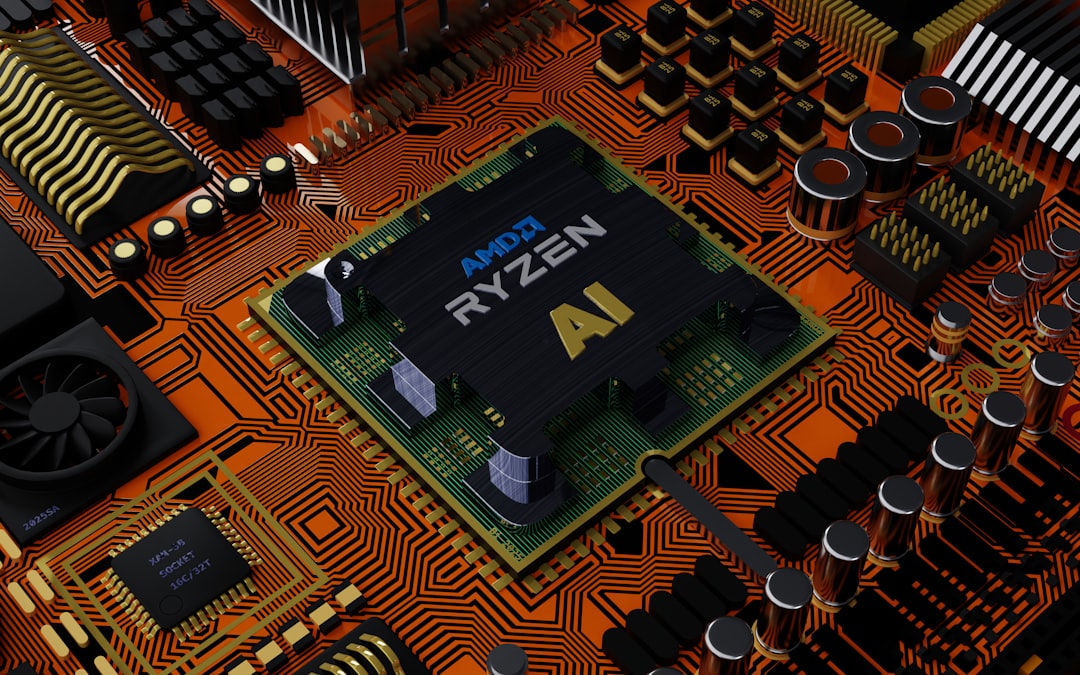
In 2024, the marriage of process mining tools and RPA is becoming increasingly crucial for businesses aiming to optimize their automation efforts. Process mining acts as a powerful lens, offering deep insights into existing workflows. By analyzing these processes, organizations can pinpoint exactly which parts are best suited for automation via RPA. This ensures that RPA investments are focused on areas that deliver the most significant impact, boosting efficiency and reducing wasted effort.
The benefit of this pairing extends beyond just initial implementation. It fosters an environment where continuous improvement is possible. As businesses adapt and evolve, the data from process mining can help tweak and refine RPA deployments. This ensures that automation efforts remain aligned with changing business needs and don't become outdated or ineffective. It's a crucial part of a more agile and responsive approach to automation.
The future likely sees the tighter integration of process mining and RPA leading to more robust and effective automation strategies. By intelligently combining these technologies, businesses are not only automating tasks but also transforming how they understand and manage their operations, paving the way for a more streamlined and efficient future. While challenges exist with implementation, the potential for long-term operational gains through this fusion of techniques appears significant.
Process mining tools are proving increasingly valuable in optimizing how businesses implement robotic process automation (RPA). By examining vast amounts of operational data, these tools offer a clear picture of current workflows, revealing hidden inefficiencies and areas ripe for automation. This data-driven insight helps engineers design and implement RPA strategies that are more targeted and effective.
One of the key benefits is the ability to standardize processes across different departments before RPA deployment. This is vital since automating inconsistent processes can easily lead to unforeseen complexities and higher costs. Moreover, some process mining tools offer real-time monitoring, enabling companies to continuously track and tweak RPA performance, making them more adaptive and resilient to changes.
Traditionally, analyzing business processes could take weeks or even months. Process mining tools, however, can significantly reduce this discovery time, potentially creating process maps within days. This rapid assessment greatly accelerates the entire RPA implementation cycle, enabling faster deployments and refinements of automation solutions. Moreover, they can pinpoint bottlenecks within existing workflows before RPA is introduced. By addressing these issues upfront, businesses can maximize the efficiency of their automation efforts.
Some more advanced process mining tools use machine learning to predict how processes might change in the future and their potential impact on RPA performance. This proactive capability helps organizations stay ahead of the curve, mitigating potential disruptions and ensuring automation stays aligned with evolving business needs.
Understanding user behavior through process mining can also be insightful. By observing how people interact with systems, it becomes easier to identify manual tasks that are ideal candidates for automation. This helps ensure that the designed RPA solutions are tailored to actual business needs, minimizing the risk of ineffective automation.
Further, process mining promotes a better understanding of how different departments and processes are interconnected. By visualizing these cross-departmental workflows, organizations can develop more comprehensive RPA strategies that optimize automation across the entire organization.
Interestingly, process mining tools can also quantify the potential cost savings of RPA implementations. This ability to perform rigorous cost-benefit analyses empowers organizations to make more informed decisions regarding the scale and scope of their automation efforts.
Finally, process mining's transparency can streamline change management during RPA implementation. By clearly showcasing how workflows will evolve after automation, organizations can minimize employee resistance to change and foster acceptance of new processes.
The growing use of process mining tools alongside RPA reflects a shift towards more data-driven and optimized approaches to automation. As businesses strive for improved efficiency, reduced costs, and enhanced customer experiences, these tools offer a powerful approach to achieve these goals in the evolving landscape of 2024.
RPA in 2024 How Simplified App Development is Reshaping Enterprise Automation - Collaborative RPA Breaks Down Enterprise Silos
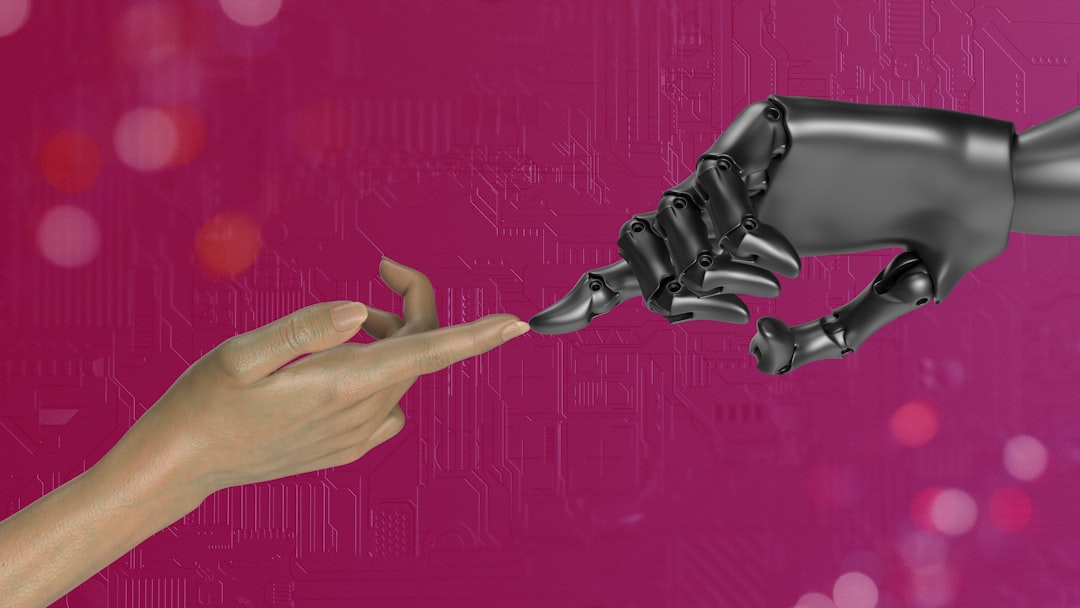
Collaborative RPA is becoming increasingly important for breaking down the barriers between different parts of a company. By involving people from different departments in the design and testing of RPA solutions, companies can create a shared understanding of automation needs and build trust in these new technologies. This collaborative approach demands flexibility in how RPA is implemented, acknowledging the varying preferences and working styles of different teams. This is especially relevant as businesses adjust to the reality of a workforce that might be working in different locations. Moreover, collaborative RPA leads to improved data transparency and better access to information across the organization. This allows companies to make better use of the knowledge and skills of their employees. The ability to connect and collaborate effectively through RPA is a crucial skill as companies work in an increasingly complex business environment.
Collaborative RPA, in essence, is about breaking down the walls that often exist between different parts of a business. It's about getting different teams to work together more effectively using RPA. While the use of low-code platforms has accelerated RPA adoption across departments, fostering a culture of "citizen developers," it can sometimes lead to disconnected automation efforts. If each department is building RPA solutions independently, it can lead to fragmented automations and data inconsistencies. Collaborative RPA tries to avoid this.
One of the ways collaborative RPA tackles this issue is by promoting a shared understanding of what needs automating. When a wider range of users are involved in identifying RPA opportunities, it creates a more holistic view of the company's processes and how they interact. This shared understanding also improves the chances of getting buy-in from the people who will be using the automation. Think of the user acceptance testing that's part of this process. People are more likely to use something they had a hand in developing.
Another interesting angle is how a collaborative approach can deal with the frustration that can arise when teams are implementing RPA in isolation. It's like trying to force together pieces that weren't designed to fit together. Collaborative RPA often involves a more flexible way of deploying RPA, allowing for a "dual mode" approach. This dual mode operation can mean using DevOps principles or just a more adaptable approach to software development.
It's worth noting that the trend of hyperautomation, where we automate more and more things, and the increasing importance of ethical considerations within automation, also play a role in collaborative RPA. When you're automating across departmental boundaries, you need to be careful about the impact on people and data. It’s easy to automate in a way that reinforces the very silos you’re trying to eliminate. For example, if you have an automation command center that's in control of everything, you could end up with a situation where the control is more centralized than before.
There's also the growing role of open-source software and the cloud in all this. It makes sense to me that open standards and cloud-based RPA platforms would contribute to more collaborative efforts. You can imagine how easier it would be to get different teams to work with the same tools, especially if the tools are available to everyone.
This idea of collaboration seems important to me, not just for the sake of technical integration but also for the long-term success of RPA within an organization. When people from different teams have input into the automation projects, they are more likely to accept and understand the changes that are happening. When they are actively involved in the automation processes, the chances of the systems being used and embraced increase.
RPA in 2024 How Simplified App Development is Reshaping Enterprise Automation - Machine Learning Automation Personalizes Customer Interactions
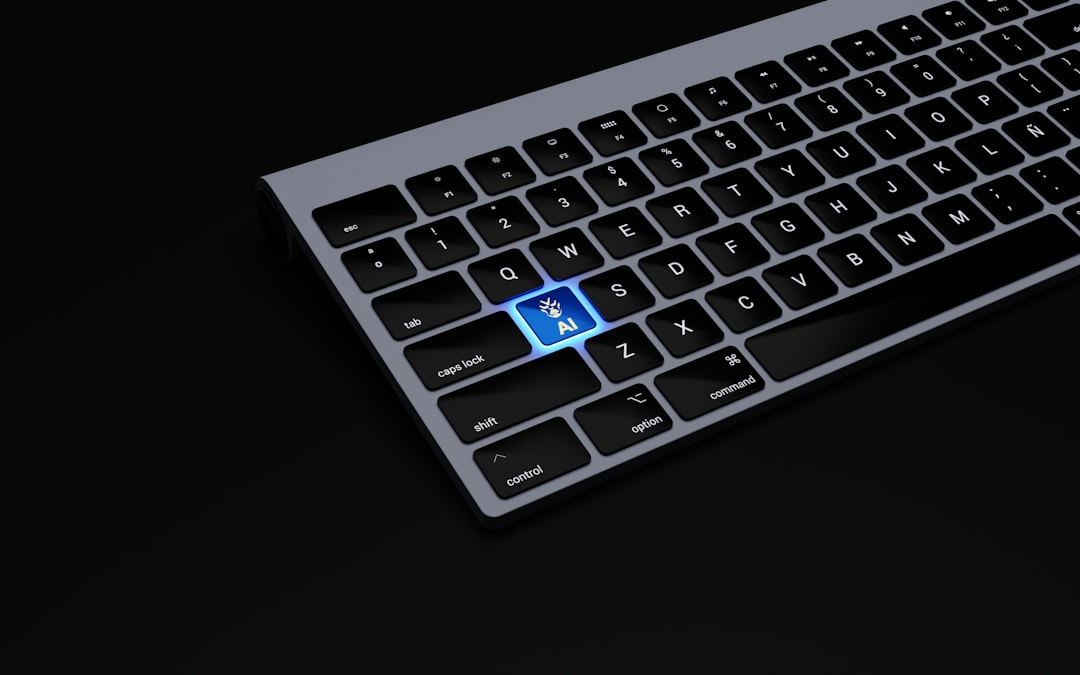
In 2024, the ability of machines to learn from data and automate decisions, known as machine learning automation (MLA), is revolutionizing how businesses interact with customers. Unlike traditional automation which relies on rigid rules, MLA allows systems to analyze customer data and discover patterns, leading to more personalized experiences. Companies can tailor their products, services, and communications to individual customer preferences, creating a more relevant and engaging experience. This type of personalization goes beyond just offering a better experience—it allows businesses to anticipate customer needs and be more proactive in their service delivery.
But there are downsides. The potential for errors due to inaccurate training or poorly maintained models exists, which could lead to a negative impact on customer relationships. MLA needs to be carefully implemented and overseen to be effective. Companies need to be aware that relying solely on automation, without human oversight, carries risks. Balancing the benefits of personalized interactions with the need for human control is crucial as businesses increasingly rely on these evolving technologies.
In the evolving landscape of 2024, machine learning is increasingly being woven into RPA to personalize customer interactions. Machine learning algorithms can sift through massive amounts of customer data, uncovering hidden patterns and preferences that inform more targeted marketing efforts. This capability empowers businesses to craft customized experiences in real-time, which can lead to higher customer engagement.
One intriguing area is how machine learning can enhance interactions through natural language processing. RPA bots can now engage in more conversational exchanges, understanding and responding to customer questions in a way that feels more natural. This is pretty interesting, as it allows for a more human-like experience without needing a human operator, which can improve customer satisfaction.
Beyond just reacting to customer behavior, machine learning models are being used to anticipate future needs. This predictive capability allows businesses to get ahead of the curve, providing solutions or services before customers even realize they need them. This proactive approach can potentially improve customer retention and foster loyalty.
However, the application of machine learning isn't a one-size-fits-all solution. Different sectors have adapted machine learning to address their unique challenges. For example, financial institutions are using it for fraud detection and risk management, while retail uses it to optimize inventory and anticipate trends. This highlights how businesses must carefully consider their specific needs when integrating machine learning into their customer interaction strategies.
We're seeing a trend toward more adaptive and flexible customer interactions powered by machine learning. Businesses can now react to customer feedback in real-time and adjust their approach accordingly. This responsiveness is beneficial, but it raises some interesting questions regarding how businesses handle the rapid shift in customer sentiment. It could lead to more responsive and agile service models, but it also necessitates carefully managing these fast-paced changes.
One area that deserves attention is the ethical considerations surrounding the use of machine learning for personalization. Concerns about data privacy and the need for customer consent are rightfully at the forefront. Companies need to be transparent and respect user privacy while still leveraging the potential benefits of personalization. Finding that balance will be crucial for maintaining trust in the future.
Furthermore, there are the practical hurdles of integrating machine learning into existing systems. Businesses may find themselves needing to allocate more resources to data infrastructure and the development of machine learning solutions. This can be a challenge, as it might necessitate a trade-off between short-term costs and potential long-term benefits.
One notable advantage is the ability of machine learning to combine data from different sources, offering a unified view of customer interactions across various channels. This consolidated perspective enables more informed decision-making, as previously hidden insights can be surfaced.
However, the complexity of machine learning can present a significant barrier to entry for some businesses. Implementing these systems requires a significant investment in skills and knowledge, creating a learning curve that many organizations might struggle to overcome.
Ultimately, ensuring that personalization remains effective requires continuous monitoring and adaptation. As customer behavior changes, the machine learning models need to be retrained and fine-tuned to ensure their continued relevance. It's a continuous process, but the payoff in terms of more effective and engaging customer interactions seems worth the effort.
RPA in 2024 How Simplified App Development is Reshaping Enterprise Automation - Ethics and Transparency Take Center Stage in RPA Deployment
The increasing use of Robotic Process Automation (RPA) has brought a critical focus on ethical considerations and transparent practices. Organizations are now not only aiming for operational improvements but also striving to ensure RPA enhances human roles within the workforce. This has led to the development of ethical guidelines for the industry, a vital step towards ensuring responsible automation. A core aspect of ethical RPA is ensuring fairness and transparency in decision-making processes, especially in sensitive areas like managing human resources. It's crucial that organizations are aware of and actively work to prevent biases that could arise from automated decisions. Organizations implementing RPA need to be mindful of their role in society and ensure their automation initiatives align with both their strategic objectives and broader societal values, creating a positive and inclusive integration of automation. As RPA becomes more sophisticated, ongoing discussions about the ethical ramifications are vital to ensuring its beneficial deployment across various work environments and for the benefit of all stakeholders.
The increasing use of Robotic Process Automation (RPA) in various sectors has brought ethical and transparency concerns to the forefront. As organizations grapple with the societal implications of technology's growing influence on human roles, a need for formal guidelines has emerged. Initiatives like the development of the first code of ethics for RPA, aimed at fostering human augmentation through robotics, represent a crucial step in addressing these concerns. This focus on ethical practices is especially important as RPA systems become more integrated into complex decision-making processes and interact with sensitive data like customer information.
We're seeing a push for transparency and accountability as automation starts to handle decisions that were previously handled by people. One consequence is a growing need for clear protocols that define who is responsible for outcomes arising from automated decisions. In addition, organizations are encountering pressure to secure user consent before employing automated systems for interactions involving personal data, highlighting the growing emphasis on trust and data protection.
However, the increasing sophistication of RPA brings new challenges. For example, research is showing how RPA systems can unintentionally amplify existing biases in data, leading to concerns about fairness and equality in automation. Organizations are becoming more aware that automated decisions, if not carefully overseen, might reinforce inequalities. Furthermore, the complexity of RPA applications calls for a more nuanced approach to compliance risk assessments that goes beyond traditional automated control measures.
In response, some companies are adopting strategies like establishing interdepartmental ethics committees to guide their automation efforts. These committees often play a key role in evaluating the broader organizational impact of RPA, ensuring that automation aligns with organizational values and minimizes potential downsides for employees. Furthermore, as RPA solutions leverage Artificial Intelligence (AI), the choice of algorithms itself is now subject to scrutiny to ensure the systems operate in ways that are not manipulative or exploit vulnerabilities.
Ultimately, ethical concerns regarding RPA are prompting a shift towards continuous monitoring. Organizations are implementing systems that track RPA operations and help them ensure adherence to established ethical guidelines. This continuous evaluation allows companies to quickly adapt to unforeseen consequences or biases detected during automated processes. This highlights how researchers and developers are trying to address ethical challenges in a constantly evolving technological landscape. It’s also leading to more research into the effects of RPA on specific areas, such as its impact on gender dynamics in areas like hiring processes. There is a growing sense that these kinds of investigations are critical to prevent unintended consequences in the future. The future of RPA will depend on carefully balancing automation's benefits with the need to ensure these powerful tools are used responsibly.
More Posts from :