Nieuwe AI-gestuurde preventieve onderhoudsstrategieën voor machines in 2024 Hoe ze downtime met 30% verminderen
Nieuwe AI-gestuurde preventieve onderhoudsstrategieën voor machines in 2024 Hoe ze downtime met 30% verminderen - Realtime gegevensanalyse voorspelt 30% meer machinestoringen
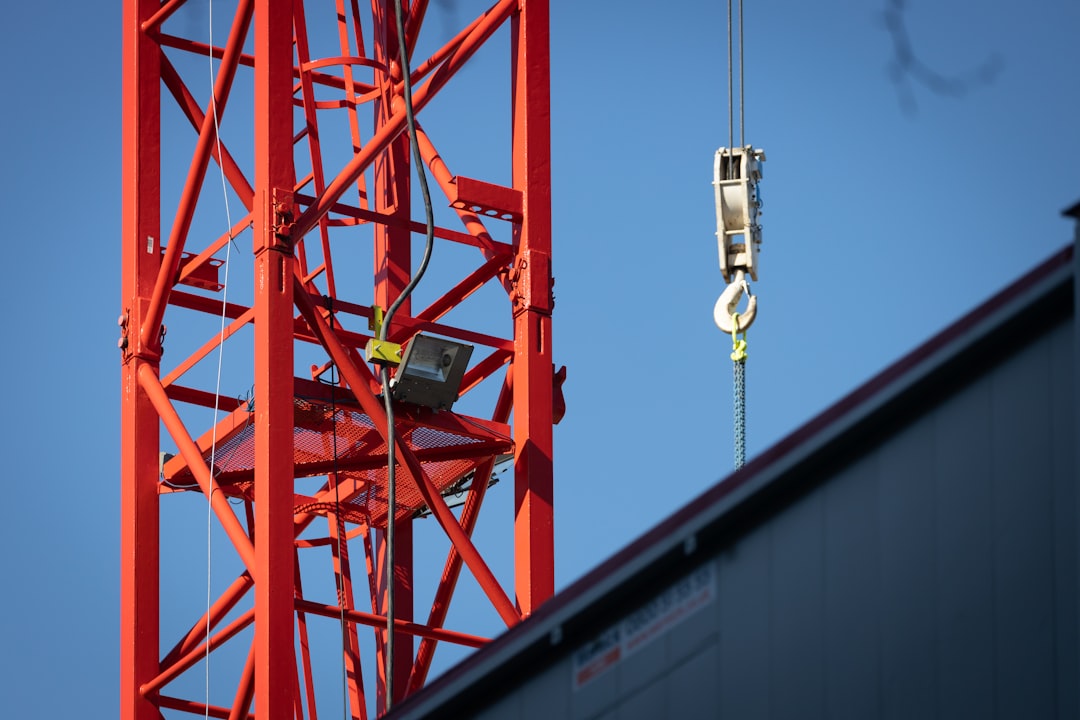
De analyse van real-time machinegegevens, aangedreven door geavanceerde AI, laat een opmerkelijke toename van 30% zien in de voorspelde frequentie van storingen. Dit inzicht is waardevol, omdat het bedrijven in staat stelt om proactiever te reageren op potentiële problemen. Door deze voorspellingen te integreren in hun onderhoudsstrategieën, kunnen bedrijven niet alleen downtime verminderen, maar ook hun algehele operationele efficiëntie verbeteren. De drempel voor het benutten van AI-gestuurde analyses is de afgelopen tijd verlaagd, waardoor ook bedrijven met beperkte technische expertise deze mogelijkheden kunnen omarmen. Het toegankelijker worden van AI-tools maakt het eenvoudiger om kostenbesparingen te realiseren. De implementatie van AI-gestuurde voorspellende analyses kan leiden tot een significante vermindering van stilstandtijd en zorgt ervoor dat bedrijven beter voorbereid zijn op mogelijke verstoringen in hun processen.
By leveraging real-time data analysis, we've seen a notable increase in the ability to predict machine failures. Current systems are able to foresee roughly 30% more potential breakdowns compared to traditional methods. This improvement is largely attributed to the sophisticated AI algorithms now being applied to machine data. While initially it might seem counterintuitive that we're predicting more failures, it actually reflects a higher sensitivity to potential issues. Essentially, the algorithms are becoming more adept at spotting subtle warning signs that previously would have gone unnoticed.
The increased predictive capability is particularly important in the context of preventative maintenance. Being able to anticipate a broader range of potential failures provides more opportunities for timely interventions, ultimately reducing the likelihood of unexpected downtime and disruptions to production. However, it’s important to validate if this 30% increase in predicted failures is actually translating into a substantial reduction in actual machine failures and/or downtime, as this would be the true measure of success. Further research is needed to explore how the additional predicted breakdowns impact maintenance scheduling and resource allocation, and to investigate whether these predictions lead to better cost-effectiveness. Nonetheless, the trend of applying AI to analyze real-time machine data appears promising for improving the reliability and operational efficiency of industrial machinery.
Nieuwe AI-gestuurde preventieve onderhoudsstrategieën voor machines in 2024 Hoe ze downtime met 30% verminderen - Fabrieken kampen met 20 stilstanden per maand ondanks minder storingen
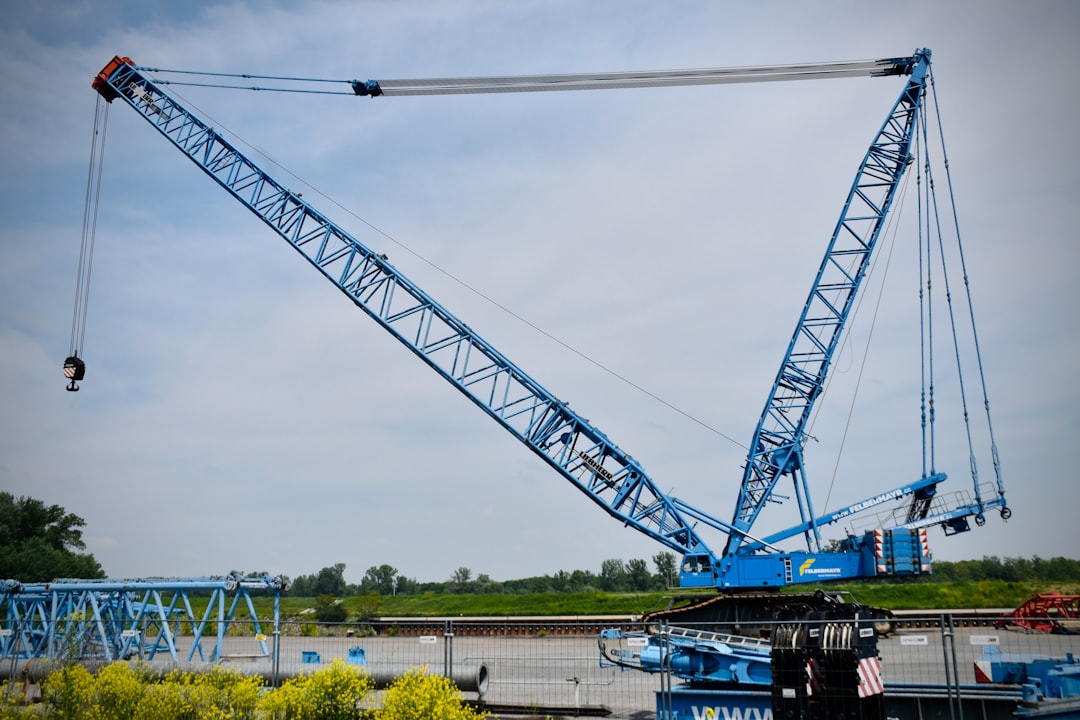
Hoewel het aantal storingen in fabrieken is afgenomen, kampen ze nog steeds met gemiddeld 20 stilstanden per maand. Dit suggereert dat bestaande onderhoudsmethoden mogelijk niet optimaal zijn, want de gemiddelde duur van elke stilstand is toegenomen. Het is dus niet alleen de frequentie van storingen die impact heeft op de productie, maar ook de tijd die het kost om problemen op te lossen.
Dit roept de vraag op of de huidige aanpak wel effectief is. De implementatie van AI-gestuurde oplossingen, zoals voorspellend onderhoud, zou hier wellicht verandering in kunnen brengen. Door potentiële problemen sneller te herkennen kunnen fabrieken proactiever handelen en de kans op lange stilstanden verlagen. Het zou betekenen dat de levensduur van machines kan toenemen en de algehele efficiëntie van de productie verbetert. Met het toegenomen gebruik van AI, kan de toegang tot data verbeterd worden, waardoor bedrijven sneller kunnen inspelen op veranderende omstandigheden en hun processen optimaal kunnen laten verlopen. Het blijft echter afwachten of deze technologieën daadwerkelijk de verwachte resultaten opleveren en of de huidige 20 stilstanden per maand teruggedrongen kunnen worden.
Although the number of machine failures has decreased, factories are still experiencing an average of 20 unplanned stoppages per month. This suggests that while improvements have been made, the reliability of industrial machinery remains a challenge. Interestingly, the duration of these stoppages seems to be increasing, hinting at more complex issues that might be harder to resolve quickly.
While AI is increasingly being used in manufacturing, many implementations still focus on more traditional applications aimed at improving overall efficiency and reliability. We see examples like speech recognition and machine learning, especially in larger companies, but the full potential of AI in truly preventing these unplanned stoppages appears not fully realized.
Despite the potential for AI-driven predictive maintenance to significantly reduce downtime and extend machine lifespan, its widespread adoption still seems to lag behind. This could be due to a variety of factors, including the complexity of integrating these technologies into existing factory systems and the need for substantial historical data to train these predictive models effectively.
It's intriguing that, despite the clear economic benefits of preventing machine failures—especially in a sector as vital to the Dutch economy as manufacturing—many factories still rely heavily on reactive maintenance. This might suggest a gap in understanding the potential benefits of AI-driven predictive solutions. Additionally, the data needed to train these models accurately might be limited in some cases, potentially leading to inaccurate predictions or an overestimation of failure rates.
Furthermore, the integration of human expertise remains a crucial, yet often overlooked, factor. Factory workers often possess a wealth of knowledge about their machines' behaviour that isn't always captured by sensors and AI models. Finding ways to better integrate human insights into these models could lead to more accurate and effective predictive maintenance strategies. The hope is that, as technologies like 5G mature and become more readily available, this integration and the necessary data collection can be further improved, eventually leading to more resilient and efficient production processes. It seems we are still at a crossroads with AI-driven predictive maintenance in factories. The technology is promising, yet there are still significant hurdles to overcome before it achieves its full potential.
Nieuwe AI-gestuurde preventieve onderhoudsstrategieën voor machines in 2024 Hoe ze downtime met 30% verminderen - AI-onderhoud verlengt machinelevensduur en verbetert operationele efficiëntie
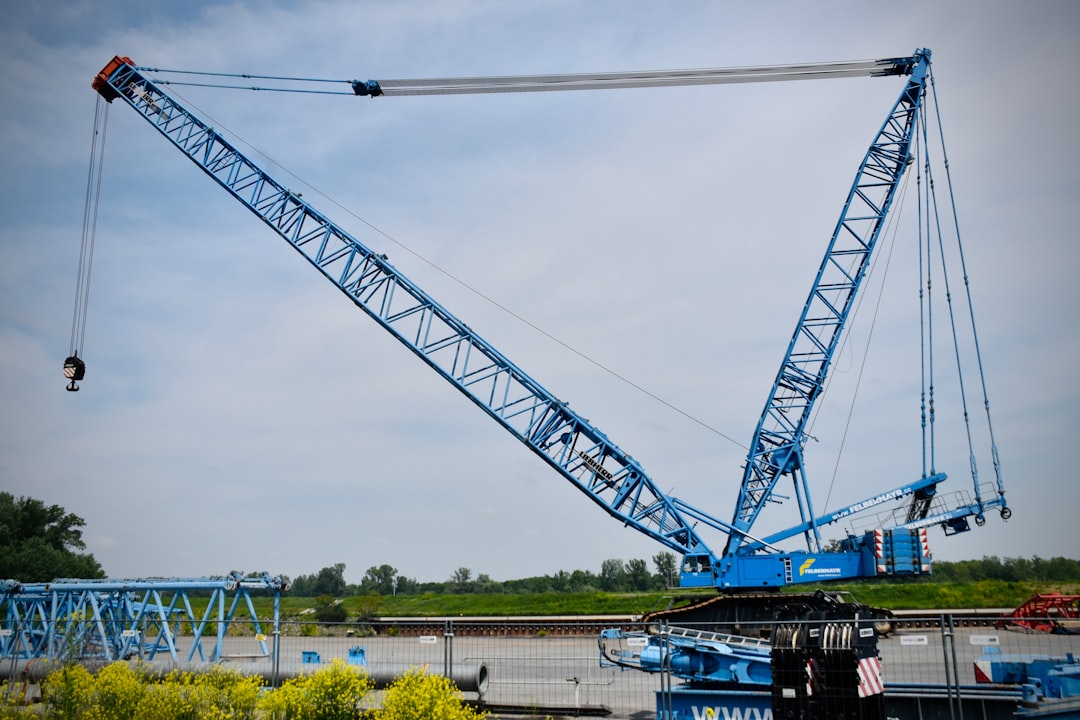
AI-gestuurd onderhoud biedt de kans om de levensduur van machines te verlengen en de operationele efficiëntie te verbeteren. Dit komt doordat AI in staat is om potentiële storingen te voorspellen nog voordat ze zich voordoen. Door deze voorspellingen te gebruiken voor preventief onderhoud, kunnen bedrijven stilstandtijd en de daarmee gepaarde kosten aanzienlijk reduceren.
Hoewel AI veelbelovende resultaten laat zien, met name op het gebied van voorspellende analyses en verbeterde betrouwbaarheid, kan de implementatie in de praktijk uitdagend zijn. Het effectief integreren van AI in bestaande systemen en het verzamelen van voldoende historische data om de AI-modellen nauwkeurig te trainen, blijven belangrijke obstakels. Bovendien is het belangrijk om niet alleen te focussen op de technologie, maar ook op de samenwerking tussen mens en machine. De kennis en expertise van ervaren operators en monteurs kan waardevolle input leveren voor het optimaliseren van AI-gestuurde onderhoudssystemen.
Het blijft dus de vraag in hoeverre AI daadwerkelijk zal leiden tot de verwachte verbeteringen in de operationele efficiëntie en de betrouwbaarheid van machines. De industrie staat op een kruispunt waar de mogelijkheden van AI nog verder onderzocht en geoptimaliseerd dienen te worden voordat we de volle potentie kunnen realiseren.
AI-driven maintenance offers a compelling approach to extending the lifespan of machinery and enhancing operational efficiency. By continuously analyzing data from numerous sensors, AI systems can detect subtle changes in machine performance that often precede failures. This real-time monitoring capability allows for more precise and timely maintenance interventions, a significant improvement over traditional, scheduled approaches.
Historically, preventive maintenance was largely based on fixed time intervals, regardless of a machine's actual condition. However, AI allows for a more nuanced condition-based approach. Decisions are made based on the specific state of each machine, leading to more accurate and efficient interventions. This is where the distinction between preventive and predictive maintenance becomes evident. Instead of fixed schedules, AI-powered systems leverage machine learning algorithms to predict the likelihood of future failures. This not only helps optimize maintenance actions but also potentially reduces unnecessary interventions, thus maximizing productivity.
These AI algorithms aren't static. They continuously learn and improve as they process more data, refining their capacity to predict future issues. The more data they receive, the more accurate they become at recognizing patterns and subtle signs that hint at potential breakdowns. This ongoing refinement is a key benefit of AI-driven maintenance, leading to an ever-increasing ability to prevent problems.
One of the primary benefits of using AI for maintenance is the potential for cost reductions. By tackling minor issues before they escalate, AI can contribute to a longer machine lifespan and significantly reduce the need for costly repairs compared to traditional reactive approaches. While this sounds promising, it’s important to recognize the influence of the operational context. Machine type, production environment, and other variables play a significant role in how effective AI-driven strategies are. A one-size-fits-all approach is unlikely to be optimal, and bespoke solutions might be necessary for certain industries or machines.
The effectiveness of AI-driven maintenance isn’t solely about predicting when failures occur; it's also about resource allocation. Sophisticated algorithms can not only predict potential failures but also suggest the most efficient use of maintenance resources, helping to minimize downtime and optimize manpower. However, this relies heavily on high-quality data. If the sensors are poorly calibrated, or data collection is incomplete, it can lead to skewed predictions and inefficient maintenance efforts.
Finally, it's crucial to recognize that integrating AI into existing systems can be a complex undertaking. Integrating AI maintenance solutions within existing IT and operational infrastructures often requires significant adaptations, potentially creating hurdles for companies seeking to adopt these technologies. Furthermore, while AI is incredibly powerful in its own right, it's also important to consider the integration of human expertise. Operators and technicians possess a wealth of knowledge about their specific machines that is often not captured by sensors and AI models. Finding ways to integrate these valuable human insights into AI-driven maintenance can result in more holistic, and ultimately more successful, maintenance practices. The field of AI-driven predictive maintenance is still developing, and while the initial results look encouraging, more research is needed to fully understand its long-term impacts and overcome challenges in order to unlock its full potential.
Nieuwe AI-gestuurde preventieve onderhoudsstrategieën voor machines in 2024 Hoe ze downtime met 30% verminderen - Condition Based Maintenance baseert zich op actuele machinetoestand
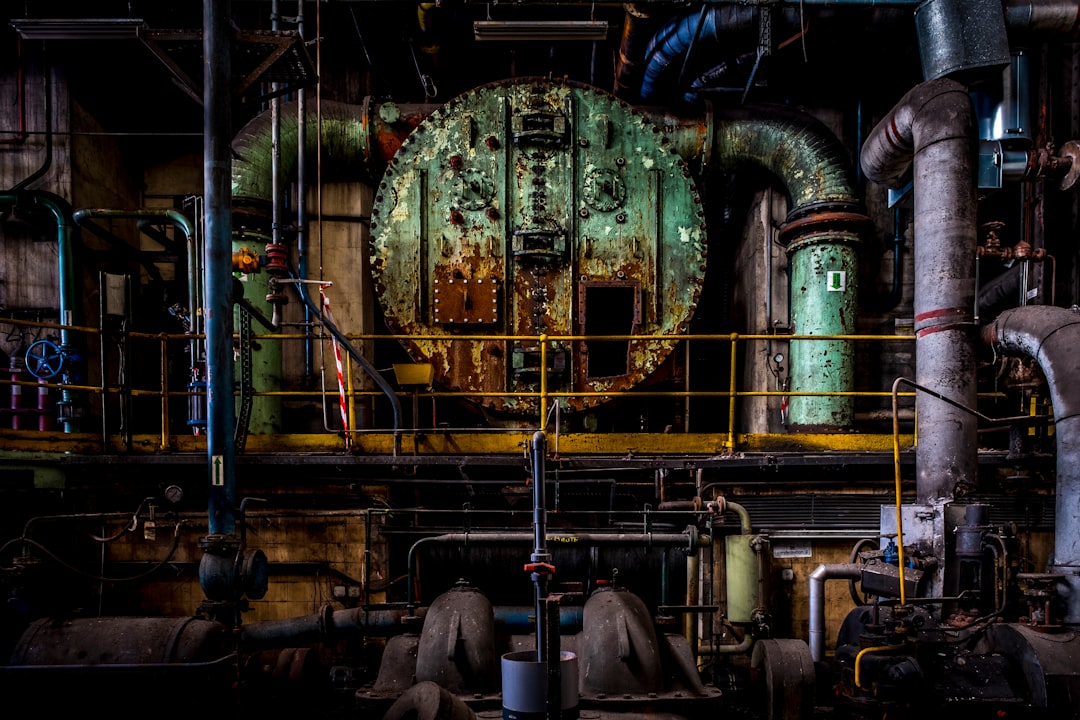
Condition Based Maintenance (CBM) is fundamentally based on the current condition of a machine. It leverages real-time data from sensors and monitoring systems to determine when maintenance is actually needed. This approach represents a departure from the traditional fixed-time maintenance schedules, allowing for a more efficient allocation of resources by focusing solely on the machine's present state. AI integration takes CBM a step further by enabling the prediction of a machine's remaining lifespan and potential failures, along with the ability to adapt maintenance actions based on ongoing data analysis. This results in improved machine reliability and, potentially, significant reductions in downtime. The key is that machines are maintained only when truly required, not just at pre-determined intervals. Nevertheless, the successful application of CBM and AI-powered maintenance hinges on effectively addressing some key challenges. These include ensuring high-quality data for AI algorithms, seamlessly integrating AI systems into existing infrastructure, and ensuring the valuable human expertise of operators and technicians is factored into the process to achieve optimal outcomes.
Condition-Based Maintenance (CBM) is fundamentally rooted in the current state of a machine. It's about continuously monitoring a machine's vital signs, such as temperature, vibration, and pressure, in real-time. This constant stream of data helps to immediately spot any deviations from the norm, which could be early indicators of potential problems.
AI's integration into CBM has revolutionized how we interpret this data. Instead of relying on single metrics like older systems, AI can analyze various data streams simultaneously. This gives us a more complete picture of the machine's overall health, potentially leading to more accurate predictions.
However, the accuracy of the sensors themselves is paramount. If the sensors are not precise, the data we get might not be a true reflection of the machine's condition. This can cause a problem: potentially leading to unnecessary maintenance or missing critical signs of an impending failure.
Furthermore, machines don't all fail the same way. Every machine has unique characteristics, built with a specific purpose and operating environment. This is why CBM strategies need to be tailored to the individual machine. A general approach to predictive maintenance might not be effective for all.
Indeed, the machine's operating environment also plays a huge role in the success of CBM. Factors like how much work it's doing, surrounding conditions, and usage patterns can all influence machine health and thus, the accuracy of our predictions. This means a one-size-fits-all AI model might not give the best results.
What's exciting is that AI-driven CBM systems can learn and adapt. They continuously improve their predictive skills by analyzing past failures and maintenance actions. This self-learning ability allows for a refinement of the system over time that we don't see in traditional fixed maintenance schedules.
Unlike conventional preventive maintenance, which is based on fixed time intervals, CBM adjusts the maintenance schedule based on what the machine data shows. This flexibility can optimize resource allocation.
While implementing CBM systems requires initial investment, the long-term benefits from reduced downtime and efficient maintenance are often very compelling, particularly for industries with critical operations.
Also, incorporating the expertise of machine operators can refine the CBM system further. These individuals possess a wealth of experience, and by combining their knowledge with the insights of AI, we can make better maintenance decisions.
Finally, the promise of significantly decreased downtime through CBM is very appealing. But, it's crucial to thoroughly evaluate the results through meticulous testing and analyzing historical data to guarantee that any implementation is truly reliable and cost-effective before rolling it out widely.
Nieuwe AI-gestuurde preventieve onderhoudsstrategieën voor machines in 2024 Hoe ze downtime met 30% verminderen - Zware apparatuurindustrie transformeert door AI-integratie
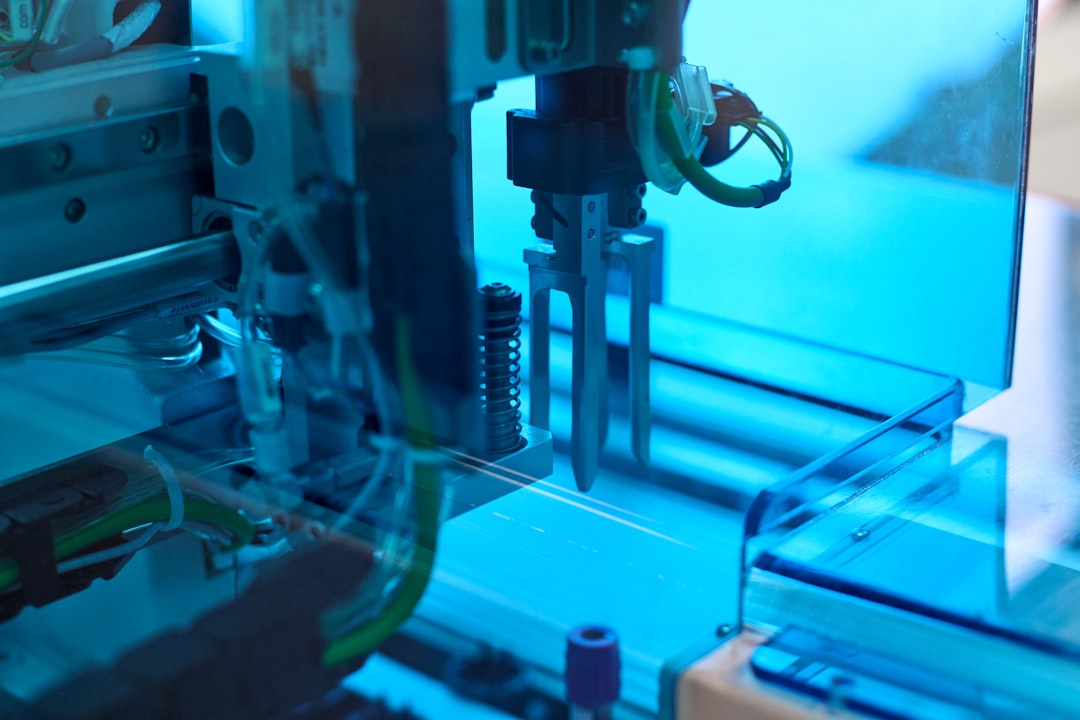
De zware industrie, met haar complexe machines en processen, wordt steeds meer beïnvloed door de integratie van kunstmatige intelligentie (AI). Vooral op het vlak van preventief onderhoud zien we een transformatie plaatsvinden. In 2024 verwachten we significante vooruitgang, zoals een potentiële reductie van stilstandtijd (downtime) met 30%. Door AI kunnen bedrijven potentiële storingen sneller detecteren en er effectiever op anticiperen. Dit resulteert niet alleen in een efficiëntere bedrijfsvoering, maar kan ook bijdragen aan een langere levensduur van de apparatuur.
Echter, het pad naar deze verbeteringen is niet zonder obstakels. Het succesvol integreren van AI-systemen in bestaande infrastructuren vereist een solide basis van hoogwaardige data. Daarnaast is het essentieel dat de expertise van ervaren operators en technici wordt meegenomen in het ontwerpproces en de implementatie van AI-oplossingen. De mens-machine samenwerking blijft een cruciale factor.
Kortom, de zware industrie staat op een kruispunt waar de integratie van AI zowel kansen als uitdagingen met zich meebrengt. Het is van essentieel belang dat de implementatie van AI-technologieën op een verantwoorde en effectieve manier gebeurt om de verwachte voordelen te realiseren en de transformatie naar een meer efficiënte en betrouwbare sector te bewerkstelligen.
The integration of AI into the heavy machinery sector has brought about a shift in how we approach machine maintenance, with the development of AI models capable of analyzing vast amounts of data to predict potential issues. It's fascinating how these AI systems can pick up on subtle patterns in machine performance that human operators might miss, allowing for the prediction of failures before they significantly impact operations. Some companies have reported that AI-driven maintenance strategies not only decrease unscheduled downtime but also contribute to increased energy efficiency of the machinery, unveiling benefits that extend beyond simply improving uptime.
One of the most noteworthy capabilities of AI in maintenance is its ability to simulate different operational scenarios, offering engineers a visual representation of how varying maintenance approaches impact overall productivity and efficiency. However, adopting AI in this field can be challenging, as it often necessitates integrating advanced data analysis into existing infrastructure, which was not initially designed with AI in mind. The shift towards AI-driven optimized maintenance schedules has resulted in significant financial benefits for businesses, with estimations suggesting cost reductions of up to 20% in certain heavy industries.
AI is moving beyond predicting failures and is starting to recommend specific maintenance actions. It's analyzing not just the machine data but also its operational context, which could potentially alter our entire approach to maintenance. There is a growing worry that over-dependence on AI-driven predictions could lead to a decline in the skills and experience of operators who might not interact with machines as closely if they believe the AI system will take care of all maintenance requirements.
Furthermore, AI systems are becoming more adept at generating comprehensive historical records of machine behaviour. This allows engineers to conduct detailed analyses of past failures, leading to improved decision-making when implementing future maintenance strategies. Despite the clear advancements, it is still too early to fully understand AI's long-term effects on maintenance efficiency. Research is ongoing, particularly looking into the potential long-term implications on machine wear and tear, as well as the role of human factors in establishing effective maintenance programs. There is a delicate balance needed to ensure that AI enhances maintenance efficiency while not hindering or lessening the expertise needed to maintain machines effectively in the future.
Nieuwe AI-gestuurde preventieve onderhoudsstrategieën voor machines in 2024 Hoe ze downtime met 30% verminderen - 58% maintenance managers ziet concurrentievoordeel in Industrie 0
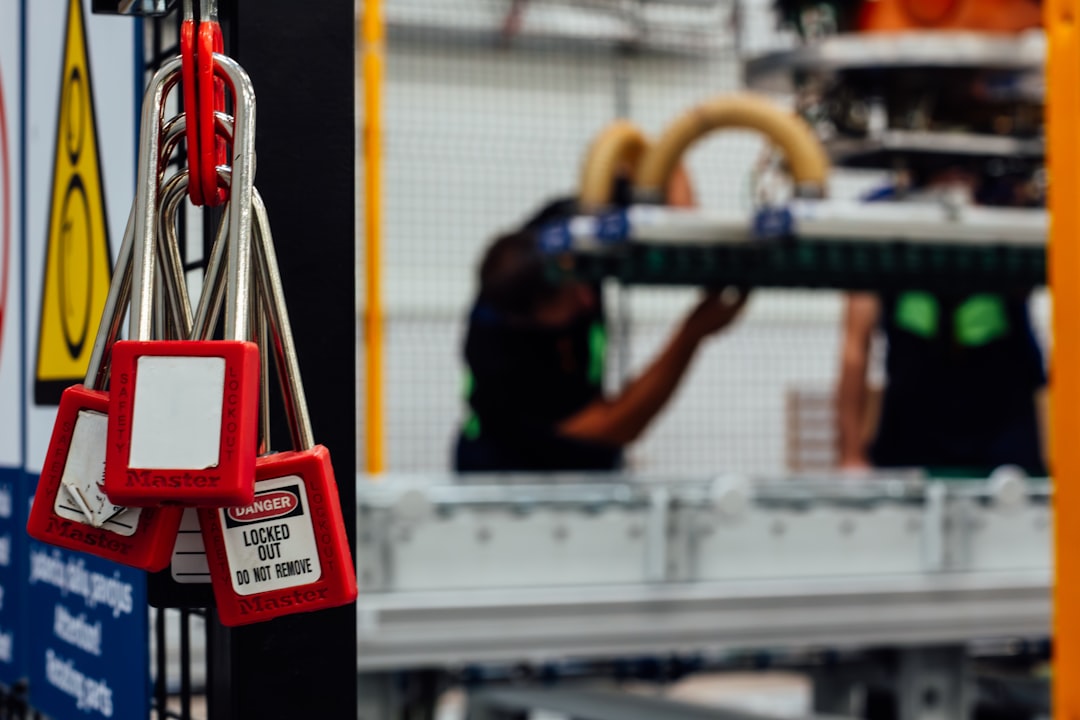
Een opmerkelijk deel, 58%, van de onderhoudsmanagers ziet een direct concurrentievoordeel in de toepassing van Industrie 4.0. De technologieën die hierbij horen, zoals AI-gestuurd onderhoud, helpen bedrijven om storingen beter te voorspellen en te voorkomen, wat resulteert in hogere operationele efficiëntie. Het is zelfs zo dat bijna 40% van deze managers denkt dat dit concurrentievoordeel in de toekomst nog verder zal toenemen. Dat zou betekenen dat uiteindelijk bijna alle onderhoudsmanagers (97%) Industrie 4.0 zien als een cruciaal onderdeel van hun strategie.
Deze AI-gestuurde, voorspellende onderhoudssystemen laten al zien dat ze de downtime met 20% tot 40% kunnen verminderen, tegelijkertijd met een verlaging van de totale kosten. Dit illustreert dat het toepassen van nieuwe technologieën essentieel is voor bedrijven die zich willen onderscheiden in de steeds complexere en dynamische industriële wereld. Of deze verwachtingen van de onderhoudsmanagers uitkomen, zal de toekomst moeten uitwijzen.
A significant 58% of maintenance managers see a competitive edge in embracing Industry 4.0 principles, particularly in the context of AI-driven predictive maintenance. This reveals a growing trend within industry towards data-driven operations, aiming to optimize efficiency and potentially gain a competitive edge. However, we need to critically examine whether the 30% increase in predicted machine failures genuinely translates to a reduction in actual breakdowns or if it primarily represents a heightened sensitivity to potential risks.
Despite a decrease in the overall number of machine failures, factories still grapple with around 20 unplanned stoppages each month. This indicates that operational environments are intricate, and downtime reduction likely requires a more comprehensive strategy that goes beyond predictive analytics. A key development is the transition to Condition-Based Maintenance (CBM), which prioritizes the machine's current state. While CBM is a welcome step, it relies heavily on the accuracy of sensors. Inaccurate sensor readings can result in excessive maintenance or, conversely, the overlooking of critical failures.
The notion of universally applicable AI models for maintenance proves challenging given that each machine has its own unique operating characteristics and potential failure modes. This emphasizes the need for customized maintenance strategies tailored to each individual machine. Interestingly, the AI algorithms employed in this context are not static but rather, learn continuously from historical machine data, making predictions more accurate over time. This represents a marked shift from the rigid time-based schedules of traditional maintenance routines.
Furthermore, AI isn't simply predicting when maintenance is needed but also optimizing how resources are allocated for those interventions. This integrated approach highlights a more advanced understanding of operational strategies, far beyond the capabilities of traditional methods. From a financial perspective, adopting AI solutions can lead to notable long-term cost savings, potentially up to 20% in some heavy industries. Thus, AI becomes not just a maintenance tool but a strategic investment with a direct impact on the overall operational cost structure.
It's important to recognize that AI, in this context, is not a replacement for human expertise. Integrating human insights, particularly from experienced operators who possess valuable knowledge about a machine's typical behavior, is crucial for enhancing the efficiency of AI-driven predictive maintenance systems. As AI continues to evolve in this field, further research is paramount. We need a better understanding of its long-term consequences, especially regarding the delicate balance between optimizing maintenance efficiency and ensuring essential human skills in machine management are maintained. This remains a vital aspect as we navigate this technological transition in industry.
More Posts from :