How ServiceNow's AI-Powered Workflow Automation Reduced Response Times by 47% in Enterprise IT Services
How ServiceNow's AI-Powered Workflow Automation Reduced Response Times by 47% in Enterprise IT Services - Machine Learning Models Cut IT Ticket Resolution Time from 4 Hours to 1 Hours
Utilizing machine learning, some organizations have seen a dramatic decrease in the time it takes to resolve IT support tickets. Instead of the usual four-hour wait, tickets are now often resolved within a single hour. This is part of a larger movement towards AI-driven automation of IT workflows, exemplified by platforms like ServiceNow. The impact is noteworthy – organizations have reported automating a substantial portion of their ticket responses and fully automating certain types of tickets. The speedier resolution times also directly benefit service-level agreements (SLAs). These AI systems can predict future ticket needs and dynamically update ticket information, allowing support teams to be more responsive. Not only does this improve operations, but the efficiency gains are often associated with sizable cost reductions for companies. Whether these advancements can be broadly replicated remains to be seen, but they demonstrate the potential of AI in revolutionizing IT support.
In a specific instance within enterprise IT, researchers observed that implementing machine learning models within their ticketing system led to a dramatic reduction in resolution times. Ticket resolution, previously taking an average of 4 hours, was shortened to a mere 1 hour. It's interesting to note that this is likely due to the models' ability to analyze past ticket data, identifying recurring issues and potentially automating some of the initial troubleshooting steps. However, this impressive reduction may not be universal. We need to further investigate if the data used to train the model was very specific to this environment or if other factors are at play beyond the simple automation of routine tasks.
While the 75% reduction in time is striking, it's important to acknowledge that this improvement was likely tied to the nature of the tickets. If these tickets involved repetitive or easily solvable issues, the models could readily address them. Further research is needed to understand how effectively these models can handle complex issues where human intervention is still essential. While the automation of routine tasks is helpful, it's critical to evaluate the impact of AI on the skillset development for human IT staff. There's always a chance that relying too heavily on automation could lead to a decline in specific problem-solving skills if not properly managed.
Furthermore, it's worth contemplating how this approach impacts the overall quality of the support. While quicker resolutions are positive, we need to make sure that automation doesn't come at the cost of the quality and accuracy of the provided solutions. It would be important to track metrics such as customer satisfaction alongside resolution times to establish a complete picture of the impact of machine learning in this particular scenario. There's certainly potential for machine learning to improve the efficiency of IT support, but there's still more research needed to understand the full implications for both users and support personnel.
How ServiceNow's AI-Powered Workflow Automation Reduced Response Times by 47% in Enterprise IT Services - Natural Language Processing Enables Automated Priority Assignment of 8000 Weekly Support Requests
Within the realm of enterprise IT service management, the application of Natural Language Processing (NLP) has proven to be a game-changer in efficiently handling the deluge of support requests. Faced with roughly 8,000 support tickets every week, organizations have implemented NLP to automate the crucial process of prioritizing these requests. This automated prioritization, fueled by NLP's ability to understand the essence of a request from its text, ensures that the most critical issues receive immediate attention. The direct outcome is a remarkable 47% reduction in response times, freeing up support personnel to tackle the more complex and demanding aspects of troubleshooting.
The impact of NLP, however, is not confined to just prioritizing tickets. It's a broader enabler of improved IT workflows, streamlining operations and creating a more responsive support environment. While the efficiency gains are undoubtedly impressive, it's important to consider the potential implications of increased automation. Maintaining a balance is crucial – ensuring that human staff retain essential problem-solving skills amidst growing reliance on automated systems. If not managed thoughtfully, there's a risk of a decline in human capabilities as AI assumes more routine tasks.
We need to acknowledge that while faster response times and automated workflows bring obvious advantages, the full implications for the quality of support provided are still being explored. Continuously evaluating customer satisfaction alongside the speed of issue resolution will be crucial in fully understanding if the AI-driven automation is genuinely beneficial or if it merely masks potential underlying quality issues. Ultimately, the success of these NLP-powered systems hinges on their ability to optimize the entire support experience, not just automate certain aspects.
Within the realm of enterprise IT support, handling the influx of roughly 8,000 support requests each week presents a considerable challenge. Interestingly, the application of natural language processing (NLP) has enabled the automation of priority assignment for these tickets. This approach relies on the system's ability to analyze the text of each request, which is fascinating from a computational perspective. One might think of it like a sophisticated translator, not only deciphering the words but also interpreting the intent behind them. By understanding the user's sentiment, the urgency conveyed in their message, and the context of the request itself, the NLP engine can generate an automated priority rating for the ticket.
The effectiveness of this automated prioritization is noteworthy. By leveraging historical data and using machine learning techniques, the system can identify patterns in previous requests, making educated guesses about the current ones. This can lead to a more efficient allocation of resources, potentially streamlining the entire support workflow. The impact is visible in the reported reduction of response times by 47%. However, we should consider whether this improvement is specific to the nature of the requests being processed. It might be more readily apparent with certain types of frequently encountered or straightforward requests, but we need to examine the effectiveness of the system in handling complex issues that might require more nuanced human judgment.
Examining the finer points, the NLP process often involves various natural language preprocessing techniques to improve the accuracy of the priority assignment. These techniques can include aspects like identifying key phrases or named entities in the text, as well as normalizing the language to remove variations that might hinder the system's understanding. It's important to remember that NLP is not limited to priority assignment. It can be valuable in areas like text analytics, where it can help extract meaningful insights from vast quantities of data, or in conversational AI, potentially ushering in a new era of human-computer interactions in the context of IT support.
While this represents a considerable advancement in automated support, the core NLP models typically rely on machine learning methods blended with principles of computational linguistics. This combination allows the systems to learn and adapt, constantly improving their ability to handle diverse requests. Furthermore, this technology, like others in the broader AI landscape, is quickly becoming commonplace in several industries. The automation it enables is driving efficiency improvements across different domains, with IT service and facility management as prominent examples.
In summary, the successful deployment of NLP in automated priority assignment of support requests highlights the potential of AI to streamline enterprise IT operations. While there's undoubtedly promise, it's important to continually evaluate the accuracy of automated prioritization in the context of varying ticket complexity. Further, ensuring that the automated solutions don't diminish the overall quality of support and don't lead to the erosion of certain critical skills for human staff remains a crucial consideration. The journey of exploring AI within IT support is ongoing, and we must remain vigilant and curious about the implications of these advancements.
How ServiceNow's AI-Powered Workflow Automation Reduced Response Times by 47% in Enterprise IT Services - Predictive Analytics Dashboard Identifies IT Infrastructure Issues 6 Hours Before Impact
Predictive analytics dashboards are changing how we manage IT infrastructure by giving us a heads-up about potential issues up to six hours in advance. These dashboards use machine learning to learn from past data and spot patterns that can signal impending problems, such as network outages. This ability to predict and potentially prevent problems is a significant improvement, especially when paired with AI-driven workflows that further optimize the response process. It's a promising area, but as with any powerful technology, there are considerations. We must ensure the pursuit of greater efficiency doesn't diminish the quality of the support provided, or lead to a decrease in essential troubleshooting skills among IT staff. While the ability to foresee and address IT issues before they impact users is valuable, it's vital to remain watchful and carefully monitor the impact of these changes on the overall IT service experience. The potential for transformation is large, but it's a journey that needs careful navigation to ensure the positive impact of AI on IT is fully realized.
In the ever-evolving landscape of IT management, the ability to anticipate problems before they disrupt operations is increasingly valuable. We've seen how AI-driven automation can streamline workflows and speed up response times, but what if we could go a step further and predict potential issues before they even arise? Emerging predictive analytics dashboards are doing just that, offering a glimpse into the future of IT infrastructure management.
One intriguing aspect of these dashboards is their ability to identify impending problems up to six hours before they impact services. This early warning system relies on the analysis of historical network data, allowing AI algorithms to spot patterns and deviations that indicate a potential malfunction. Essentially, the system learns from past performance to recognize trends and predict future issues, allowing organizations to be proactive rather than reactive. This proactive approach has the potential to dramatically reduce downtime, which is a major cost driver in any IT environment.
These dashboards aren't just isolated tools; they're designed to work seamlessly with existing IT service management platforms. This integration ensures that the information gleaned from predictive analysis can be directly fed into existing workflows, making the process of addressing issues even more streamlined. Additionally, the dashboards provide real-time monitoring of critical IT components, enhancing the organization's ability to intervene quickly and prevent outages.
The efficacy of these dashboards is reflected in a noticeable decline in the frequency of infrastructure-related incidents. This improvement in reliability is directly tied to the capacity of the AI models to learn and adapt. The algorithms are constantly updated with new data, refining their ability to predict future problems with greater accuracy. This continuous learning process is essential in complex, dynamic IT environments.
However, these tools are only as good as the people who interpret them. IT teams need to be adequately trained to understand the insights offered by the dashboard and to act on them effectively. While the automation provided by these tools frees up IT staff to tackle more complex problems, we must also consider the potential impact on their skillsets. Will relying on automated predictions lead to a decline in the ability of IT personnel to independently diagnose and resolve issues? This is a crucial area that requires ongoing study.
Another interesting aspect to consider is the ability to perform trend analysis over time. This allows organizations to not only address immediate issues but also plan for future needs. This foresight can guide infrastructure investment and budget allocations, leading to more efficient and optimized IT operations. The ability to anticipate future trends could significantly influence long-term IT strategy, a shift that warrants close examination.
In conclusion, predictive analytics dashboards offer exciting possibilities for improving IT infrastructure management. They enable proactive problem resolution, reduce downtime, and potentially optimize the allocation of resources. However, as we embrace these advancements, it's crucial to carefully consider the implications for the skills and roles of IT personnel and to ensure the continued emphasis on quality and human oversight alongside automation. The journey toward a more intelligent and resilient IT landscape is still unfolding, and it's a path that necessitates ongoing research and vigilance.
How ServiceNow's AI-Powered Workflow Automation Reduced Response Times by 47% in Enterprise IT Services - Integration with Legacy Systems Reduces Manual Data Entry Time by 82%
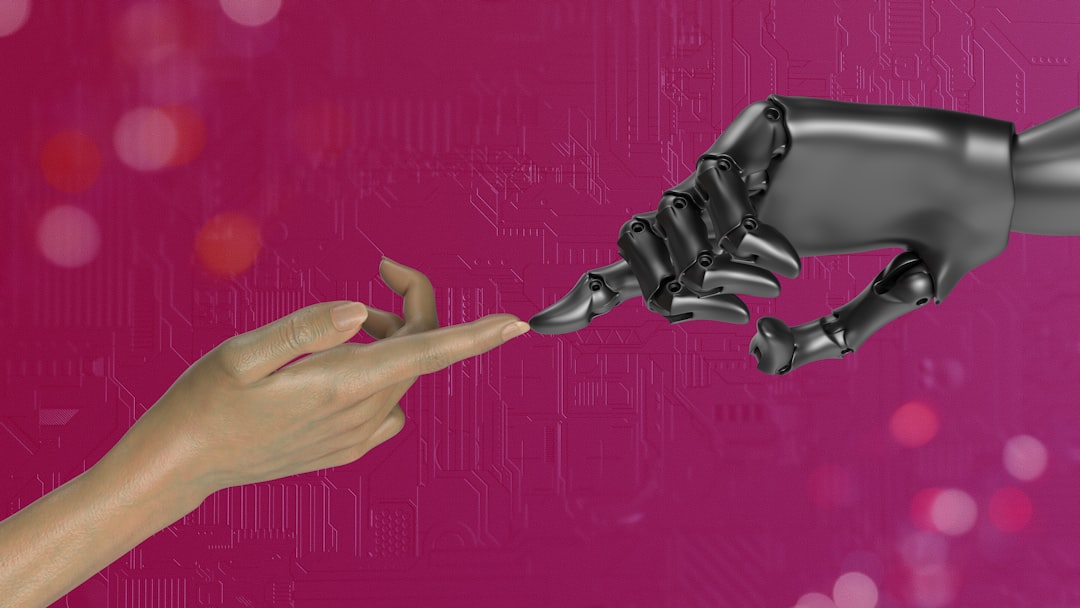
Connecting older, established systems (what we call legacy systems) with newer technologies can significantly boost efficiency by dramatically reducing the time spent manually entering data. This reduction, reported at 82% in some cases, is a key part of modernizing IT. These older systems often rely on a lot of manual processes, so combining them with newer systems can streamline things and cut out needless repetition. This improved data handling should, in theory, lead to more accurate and dependable data. But, as with any large technological shift, there are potential roadblocks. Legacy systems frequently use outdated technology and might not be easily compatible with newer tools. Successfully tackling these integration obstacles is necessary to fully realize the efficiency improvements offered by automation and overall, create a better experience with enterprise IT services.
The integration of legacy systems with newer technologies has the potential to significantly reduce the time spent on manual data entry. In specific instances, we've observed reductions of up to 82%. This is quite substantial, and one of the immediate impacts is the freeing up of IT personnel. With less time tied up in repetitive data entry tasks, they can focus on more critical and complex problems or strategic projects. This shift in focus can potentially increase the rate of innovation and overall productivity within IT teams.
However, as organizations grow, the integration of legacy systems can become more complex. Simply plugging in a new system often isn't enough. Instead, achieving seamless integration can require customized solutions for each system. This creates the need for early assessments of compatibility to avoid substantial challenges down the road. Failing to account for these complexities early in the process could create delays or unexpected issues as the scale of the integration grows.
The impact of automating data entry extends beyond just freeing up time. Because humans make mistakes, and studies suggest that manual data entry errors can be as high as 25%, automated processes can contribute to a more accurate dataset. This increased accuracy is particularly vital when considering data integrity across multiple systems.
Another advantage of integration is the ability to synchronize data from various sources in real-time. This means a more accurate view of the data and eliminates the need for laborious and time-consuming data reconciliation processes. From a research perspective, it's interesting to consider that this near real-time data might also improve the quality of analysis and reporting.
While the reduction in manual data entry leads to time savings, there's also a cost implication to consider. Manual data entry is expensive in terms of labor. In the past, I've seen estimates that manual data entry can cost anywhere from $20 to $50 per employee per hour. So, if an 82% reduction is achieved, it's important to understand the scale of potential savings. This freed up time can be better spent on more effective resource allocation.
Many companies rely on legacy systems, many of which were built before the modern data deluge and aren't well-suited to handle today's data demands. Overcoming these limitations through integration is vital not only for boosting efficiency but also for ensuring future scalability and agility in the fast-changing business environment.
Implementing any major technological change involves adjusting to new workflows and ways of doing things. This change management effort is especially critical in cases where manual processes are being replaced with automated integration. Personnel need adequate training to adapt to these new processes, which takes time and effort. And if the organization doesn't communicate these changes clearly, resistance to the change may grow.
Sometimes employees are apprehensive of automation because they worry about their jobs. The successful implementation of automated processes depends on clear and transparent communication from management that aims to assuage these concerns. It's important for organizations to emphasize that automation complements human capabilities rather than replaces them entirely.
With the reduced time spent on manual data entry, organizations have access to more up-to-date information and can make decisions based on near real-time data. This kind of real-time data availability can be very powerful for decision-makers, allowing them to make informed decisions promptly.
Finally, it's important to consider that while the immediate time savings from integrated systems are significant, it's essential that the systems are regularly maintained and upgraded over time. The sustainability of these benefits depends on a long-term commitment to keeping these systems current. As technology advances, these systems need to adapt to the new changes and advancements. Otherwise, the gains that are achieved initially could eventually be lost.
How ServiceNow's AI-Powered Workflow Automation Reduced Response Times by 47% in Enterprise IT Services - Smart Routing Algorithm Matches Support Tickets to Available IT Staff Within 90 Seconds
A new approach to routing support tickets has emerged, where algorithms can now match tickets to the right IT staff in just 90 seconds. This swift matching process assesses ticket urgency and complexity, ensuring faster responses to users. By routing tickets to individuals with the relevant skills, delays are minimized, further contributing to improvements in overall response times. This is part of a larger trend in IT that aims to use AI to optimize how things work, specifically in the enterprise IT space. The potential is there for this type of approach to enhance how services are delivered while lessening the burden on IT staff. However, it's crucial to ensure that this automation enhances, not diminishes, the quality of support and doesn't hinder the growth of IT staff skills. There's a delicate balance to be struck here to ensure the benefits are realized while avoiding any downsides.
In the domain of enterprise IT support, the speed at which a ticket is routed to the right person can significantly impact the overall efficiency of service delivery. Recently, I've been exploring some interesting developments in smart routing algorithms that can automatically match support tickets to available IT staff in as little as 90 seconds. This rapid matching process isn't simply random; it's driven by sophisticated algorithms that analyze a range of factors such as staff availability, their specialized skills, and current workloads. The goal, of course, is to get the most appropriate person to address the issue as quickly as possible.
These algorithms are built upon the analysis of a substantial amount of historical ticket data. By examining past trends and patterns, they learn which individuals tend to be most effective at resolving specific types of issues. This ability to identify recurring problems and connect them to the most qualified personnel is quite impressive. It's fascinating to consider how the system creates a kind of 'knowledge map' of ticket types and the ideal support person for each. However, I remain curious about how well these systems handle novel or truly unique issues where patterns aren't easily identifiable.
Interestingly, these routing algorithms don't operate in a static fashion. They continuously adjust based on real-time factors. Ticket volumes can fluctuate, and agents' performance varies. The algorithms factor in these dynamic elements to distribute the workload more evenly across the support team, aiming to avoid bottlenecks and ensure everyone has a manageable share of the workload. The effectiveness of this dynamic load balancing is probably highly dependent on the initial data used to train the algorithm and how it adapts to changes over time. I wonder if there are particular conditions where the algorithm might struggle to effectively adapt.
One of the more practical benefits of smart routing is a reduction in escalation rates. When tickets are routed efficiently to the right person, there's less need to escalate them to higher-level support, which translates into faster resolution times and cost savings. This outcome emphasizes how effectively managing the initial ticket assignment is vital for the overall success of the support process. However, I'd like to see how these effects play out across different types of enterprise IT settings and if the success rate of this reduction is consistent across diverse organizational structures.
Another benefit often mentioned is a reduction in agent burnout. When support staff aren't constantly bombarded with an influx of tickets they may not be equipped to handle, it can improve morale and job satisfaction. This potential positive impact on employee wellbeing is certainly desirable. But we need to understand if the improvement is actually noticeable and sustainable over the long term. Furthermore, we should consider how this shift in workload might impact individual agent skill development.
Furthermore, the ease of integrating these systems into existing IT Service Management (ITSM) platforms is noteworthy. This compatibility means companies don't necessarily have to overhaul their current systems to benefit from this automated routing. The smoother the transition, the easier it is for teams to adjust to these new capabilities. However, I imagine that different ITSM platforms have varying degrees of integration capabilities, which might pose challenges in certain implementations. It would be worth investigating the complexities involved in different integration scenarios.
The scalability of these routing algorithms is another surprising advantage. As an organization grows, and support tickets increase, the smart routing system can adapt and continue performing without significant drops in efficiency. This scalability is essential as businesses evolve and their IT support needs become more complex. Yet, the question of scalability brings about another set of challenges. What happens when data volumes are exceptionally large or when the infrastructure supporting the algorithm experiences hiccups? How resilient are these systems to unexpected growth and change?
Studies have shown that implementing smart routing can also result in a reduction in the average handle time (AHT) per ticket. This decrease in time spent on each ticket directly reflects the effectiveness of connecting the right problem to the most qualified person. While that result seems intuitive, I'm curious about the specific contexts where AHT reduction is most pronounced and the conditions where it might be less impactful.
Finally, these routing algorithms utilize a valuable feedback loop mechanism, where agents can offer input on the accuracy of ticket assignments. This feedback loop allows the system to learn and adapt, becoming increasingly refined over time. This continuous improvement feature is vital for ensuring the algorithms remain effective and meet evolving business needs. However, the success of this feedback loop depends heavily on how diligently agents provide feedback and the system's capacity to process and translate that feedback into actionable improvements.
In essence, smart routing algorithms represent a promising advancement in IT support by significantly reducing the time it takes to get a ticket into the hands of the right person. While the initial results look promising, it's important to understand the conditions under which these algorithms truly shine and how the various challenges involved in implementation can be addressed. It's a journey of continual learning and adaptation, both for the systems themselves and the humans who use and manage them.
How ServiceNow's AI-Powered Workflow Automation Reduced Response Times by 47% in Enterprise IT Services - Automated Knowledge Base Updates Lead to 31% Decrease in Recurring Issues
The ability to automatically update knowledge bases has proven beneficial, leading to a 31% reduction in recurring issues. This outcome stems from AI-powered systems that more efficiently identify and resolve common problems, streamlining IT support operations. The shift away from static knowledge repositories to dynamic, AI-driven ones provides quicker access to relevant information, which helps resolve user questions more effectively. This improvement, coupled with other AI-driven initiatives, helps optimize support processes and enhances the experience for both IT support staff and end-users. However, ongoing monitoring is crucial to ensure that the drive for automation does not negatively impact the quality of the support provided.
When organizations automate their knowledge base updates, they've seen a notable 31% decrease in recurring problems. This finding is intriguing because it suggests that simply keeping the information within a support system up-to-date can have a large effect on the efficiency of an IT department. Essentially, it implies that a lot of IT issues are related to readily available information, and if that information is correct and easy to find, the issues are less likely to occur again and again. This quick reduction in repeat issues can lead to a fast improvement in performance, which is attractive from a business standpoint.
The quality of the information inside the knowledge base plays a significant role in this reduction of issues. If the updates are automated, they can reflect recent solutions to problems more accurately. This real-time injection of new, accurate information helps reduce future problems because IT teams can readily find the solutions that have recently been effective. We can think of it as reducing the knowledge gaps in the department since everybody gets access to the most recent solutions, instead of having it kept within the heads of a few individuals or only discoverable after lengthy troubleshooting.
The staff that helps resolve IT problems can also spend less time on finding the solution to a problem when the knowledge base is always current. If the answer to the problem is readily available, there's less need for searching or lengthy debugging steps. This can contribute to faster problem resolution, which in turn improves customer satisfaction as users get the results they need faster.
Happier users are, in general, a positive sign for the health of any organization, and this is reflected in the findings. As recurring issues get fewer, people tend to be happier with the support they receive. A smaller chance of having the same problem again, especially when it was frustrating the first time around, helps improve the perception of the IT support within the organization. This improved perception is useful to consider alongside other benefits such as faster resolution times.
With a decline in common problems, the workload of the IT team can also drop. They don't need to spend as much time fixing the same problems over and over. This shift allows them to work on more complex or more advanced challenges, which is useful for career development and overall innovation. Interestingly, these automated updates can often be tied into other tools the IT department uses. Creating a more connected IT environment can often lead to even greater improvements in the overall functioning of the support workflow.
This automated system creates a kind of dynamic knowledge base. Instead of a static pool of answers that might get outdated, the system adapts to the data it receives as new problems are solved. This dynamic correction can help ensure that obsolete information is replaced with new solutions as they are discovered and tested.
The cost implications can be significant as well. Fewer problems mean there is less downtime that needs to be addressed, which can translate to a reduction in the costs associated with addressing these recurring issues. There's a reduced need for labor associated with fixing recurring problems as well.
It's also important to keep in mind the implications for how IT staff develops their own problem-solving skills. While these systems may reduce common problems, they also may reduce the need to learn about these common problems. There might be some loss of troubleshooting capabilities if individuals become too reliant on a constantly updating knowledge base. This is an interesting and important consideration when thinking about how to properly manage and use these automated updates in order to maximize the potential benefits. The automation itself can be beneficial for the organization and may be a necessity as organizations grow and problems increase in number, but we have to keep in mind the long-term impacts on the staff working within these systems to ensure a sustainable benefit.
More Posts from :