How Data Analytics is Revolutionizing Customer Relations in 2024 A Deep Dive into ROI Metrics
How Data Analytics is Revolutionizing Customer Relations in 2024 A Deep Dive into ROI Metrics - Predictive Analytics Shifts from Generic to Individual Customer Focus in Digital Banking
Digital banking is undergoing a significant shift, moving away from broad, generalized strategies in predictive analytics towards a much more individualized approach. Banks are now capable of leveraging extensive data sets to understand specific customer needs and behaviors, allowing them to anticipate and respond to these individual preferences in a way that generic solutions simply cannot.
This change necessitates a closer integration of tools like machine learning and AI into predictive analytics. This enables banks to craft services tailored to individual customer profiles, taking into account their transaction history and online behavior. Banks that successfully implement this approach see benefits beyond improved customer satisfaction. It's a way to establish a stronger position in a market where competition is fierce.
We are also witnessing a decentralized trend within the application of predictive analytics in banking. Teams across various departments now have the ability to develop their own analytical capabilities, allowing them to personalize and refine the customer experience in ways that were previously unimaginable. This shift further empowers banks to offer a more nuanced and individual approach, benefiting both the customers and the bank's competitive position.
Digital banking's predictive analytics journey has shifted from broad, generalized approaches towards a laser focus on individual customers. This means sophisticated algorithms can now examine hundreds of unique data points per person, going beyond simple demographics and creating incredibly specific financial services aligned with individual spending patterns.
The impact of this shift is tangible, with some banks reporting significant jumps in customer retention—up to 20%—simply by personalizing their service offerings. This heightened personalization is being achieved in part through behavioral biometrics. Banks now monitor subtle user behaviors like typing speed and mouse movements for enhanced security, but also to personalize the entire user experience.
Interestingly, this individualized focus isn't just about providing a better experience; it's also revealing potential needs before they even surface. Real-time analytics, for instance, can anticipate a customer's requirement for a loan or investment opportunity months in advance, enabling proactive engagement. This level of foresight has also yielded substantial cost savings, reducing marketing expenditures by roughly 30% due to the effectiveness of targeted campaigns compared to traditional, broad-based methods.
Moreover, these predictive models are becoming more nuanced. They can now integrate external data like social media interactions to generate risk profiles that go beyond traditional credit scoring systems. Similarly, the incorporation of natural language processing lets banks understand the context and emotions in customer interactions, leading to better estimations of needs and satisfaction.
This isn't limited to just predicting customer wants, it's also about preventing loss. Predictive analytics can flag individuals at risk of churning, allowing banks to step in with solutions and retain about 15-25% of customers who might otherwise leave. However, this amplified emphasis on individual customer data raises important questions surrounding privacy. We're seeing banks invest heavily in robust security measures to ensure they're compliant with regulations and maintain customer trust as they delve into personal data for analytics.
This increased focus on individual customer experiences also suggests the increasing integration of predictive analytics with newer technologies like blockchain. This potential synergy promises greater transparency and control over personal financial history for customers, further fostering trust and offering more personalized financial management in real-time. It will be fascinating to see how this evolution of predictive analytics continues to shape the digital banking landscape.
How Data Analytics is Revolutionizing Customer Relations in 2024 A Deep Dive into ROI Metrics - Real Time Customer Data Integration Shows 32% Higher Response Rates
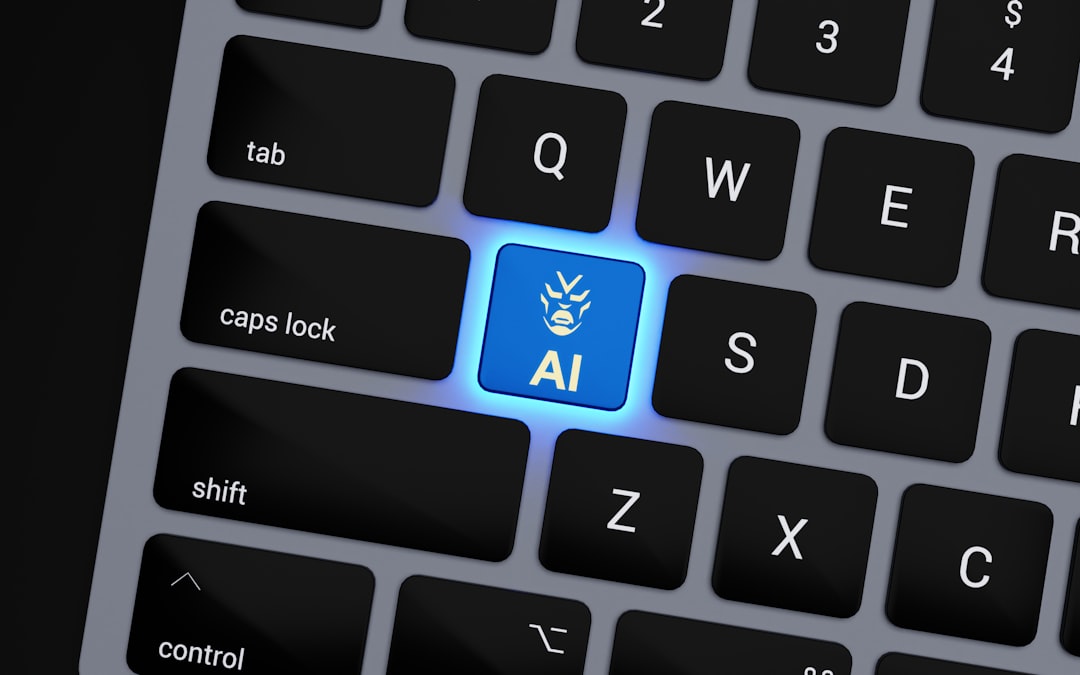
The ability to integrate real-time customer data is proving increasingly vital in today's business environment, leading to demonstrably better results in customer outreach. Studies show that incorporating real-time customer data into marketing efforts can boost response rates by a significant 32%. This underscores a wider trend towards highly personalized customer experiences. Businesses are realizing that understanding customers' immediate needs and preferences is critical in a climate of heightened expectations for service.
The use of real-time analytics isn't just about boosting engagement—it's about making marketing more efficient. By getting a clearer picture of customer behaviors in the present moment, companies can more effectively anticipate needs and tailor their strategies. This allows them to move away from broad, generic outreach and adopt a more responsive and tailored approach, maximizing their return on marketing investments. As competition intensifies and customers expect faster and more individualized responses, the capacity to utilize real-time customer data offers a distinct competitive advantage in 2024 and beyond. It allows organizations to meet the changing demands of a customer base that's become accustomed to instant gratification and highly personalized experiences.
The ability to integrate customer data in real-time has been shown to significantly boost response rates in marketing initiatives, with some studies suggesting a 32% increase. This suggests that being able to rapidly analyze customer interactions and preferences is becoming increasingly important for connecting with customers.
It's intriguing that this isn't just about the speed of delivery, but also the ability to adapt quickly to the ever-changing customer sentiment. When you can analyze data in real time, you're not just sending out generic marketing messages, but rather are attempting to align your messaging with the evolving preferences of each customer segment. This can create a sense of responsiveness that isn't achievable with delayed or batch processing of data.
This rapid processing can help businesses identify trends and changing needs much sooner. This responsiveness can be crucial, especially when it comes to product releases or targeted promotions. If a business notices a sudden uptick in requests for a certain product feature, they can act fast to either improve current offerings or create new ones —potentially gaining a competitive edge.
However, this requires some pretty sophisticated technology. We're talking about algorithms and machine learning models designed to process hundreds of data points per user in real-time. This level of processing power not only boosts response rates, but can also enhance personalization and tailor communications down to the individual user level, in a way that was impossible before.
Some organizations report that this immediate access to customer data has also contributed to lower customer churn. Presumably, this is because they're able to react more efficiently to customers' needs and resolve problems or frustrations before they escalate. If a customer feels heard and understood, they're less likely to shop around.
The speed with which organizations are able to analyze data has also dramatically changed product development cycles. Now it's possible to integrate real-time customer feedback and make iterative improvements to products at a much quicker pace. It's like a continual testing ground, always optimizing products based on real-world customer experiences.
Interestingly, while it's intuitive that this type of data integration could improve marketing, it can also translate to a reduction in overall spending. By being laser-focused on only the most relevant marketing initiatives and leveraging customer data, businesses can potentially trim down their advertising expenses because they're getting a much higher ROI compared to old methods.
However, the improved response rate isn't just driven by better offers. These analytical systems are able to glean insight into customer emotion, allowing for a more empathetic communication style. Knowing whether a customer is frustrated or excited can be incredibly valuable in calibrating your messaging.
It's important to note that with these benefits come significant responsibilities regarding data privacy. As customers become more aware of how their data is used, they are also becoming more vigilant. Organizations leveraging real-time data integration need to be completely transparent about their data handling practices and have strong security measures in place.
Finally, it's worth considering that this type of system could potentially positively impact the workforce as well. Employees who feel empowered to use data to adapt and solve customer issues are likely to have higher job satisfaction and engagement. If employees are aware they are making a difference through data-driven decision making, it can have a beneficial ripple effect on the entire organization, not just customer satisfaction.
How Data Analytics is Revolutionizing Customer Relations in 2024 A Deep Dive into ROI Metrics - Machine Learning Models Cut Customer Service Response Times by 47%
In 2024, the use of machine learning models in customer service has resulted in a significant 47% decrease in response times. This improvement highlights a clear trend towards integrating AI into customer interactions, as organizations increasingly utilize AI tools to manage customer inquiries. A majority of customer interactions are now being successfully handled through digital channels, further solidifying this trend. This adoption is yielding benefits for businesses in terms of faster responses and simpler management of customer needs. While these AI-powered solutions offer benefits in terms of operational efficiency, they also enable more customized and compelling customer interactions, part of the broader trend of using data analytics to enhance customer relationships. It's important, however, to be aware of the need to address data privacy concerns and ethical considerations as companies expand the role of AI in their customer service strategy.
Machine learning models are increasingly being used to analyze past customer service interactions, helping to spot patterns and predict the types of questions that customers might ask. By preparing for these common inquiries beforehand, companies can significantly decrease the time it takes to provide a response. It's fascinating how this proactive approach can have such a direct impact on response times.
Early implementations of machine learning in customer service have shown some pretty impressive results. Models trained on just a few months of data can noticeably improve the efficiency of responses, often within just a few weeks of being put into use. This is a stark contrast to more traditional customer service systems, which typically need a huge amount of historical data to produce useful outcomes.
We're also seeing a shift in customer preferences, with research suggesting that roughly 70% of individuals favor self-service options these days. Machine learning plays a critical role in this, providing the foundation for chatbots and virtual assistants that can quickly resolve simple questions. This reduction in the demand on human agents allows them to focus on more complicated issues, further enhancing overall efficiency.
A 47% decrease in response times isn't simply a product of faster technology. It's also a consequence of machine learning's ability to categorize customer questions by their complexity and urgency. Simpler issues are handled rapidly, while more intricate cases are efficiently routed to a human agent. It's this intelligent triage system that helps optimize response time across the board.
Machine learning models possess a unique characteristic: they continuously learn and improve. As new customer interaction data becomes available, the models can refine their responses and suggestions. This adaptive capability ensures that service quality and efficiency continue to improve over time without constant human intervention. It's intriguing how these systems can evolve and improve without a constant need for human oversight.
Some studies suggest that machine learning tools can cut the need for human intervention by up to 60% when it comes to handling standard customer inquiries. This enables companies to redirect human resources towards more complex tasks, potentially optimizing both staffing levels and operational costs. It's interesting to think about how this shift could influence workforce allocation and efficiency.
Integrating machine learning not only speeds up responses, but also improves the overall quality of the interaction. Because it's possible to personalize solutions based on a customer's history, it can lead to a more positive experience, as the service provided aligns with individual needs and preferences. This individualized approach seems to be a significant factor in improving customer satisfaction.
The shift toward using machine learning is having a positive effect on employee satisfaction. Fewer routine inquiries means human customer service reps get to work on more stimulating and engaging issues, which could ultimately boost overall productivity. It's logical to think that a more engaging work environment would have a positive effect on employee morale and motivation.
Transparency in machine learning models could further enhance the customer service experience. When models can explain their reasoning behind certain responses or suggestions, customers may feel more connected to the entire process. This greater understanding can potentially lead to a more positive perception of the brand.
Despite all these benefits, it's crucial to remain aware of the potential for biases within machine learning models. If these biases are not addressed, they can negatively impact customer interactions. This emphasizes the importance of regularly assessing and fine-tuning the models to ensure that customer service is fair and unbiased across the board.
How Data Analytics is Revolutionizing Customer Relations in 2024 A Deep Dive into ROI Metrics - Privacy Compliant Data Collection Methods Double Opt In Rates
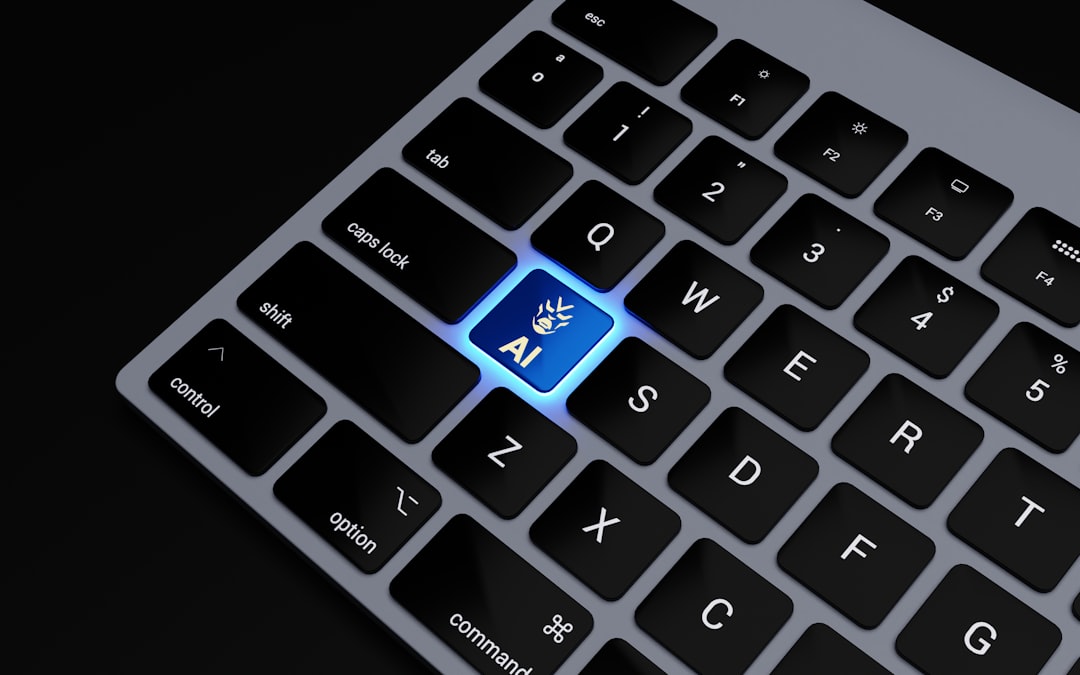
In the current landscape of 2024, where data analytics is transforming customer interactions, achieving compliance with privacy regulations is increasingly important. One key method for demonstrating this commitment is the double opt-in process. By requiring explicit confirmation from users before they are added to a mailing list or similar service, companies demonstrate a respect for data privacy. This two-step verification process not only fulfills legal obligations, but also shows customers that their data is handled carefully and responsibly, boosting their trust and confidence. The rate of double opt-ins becomes a significant indicator of a company's dedication to ethical practices, a crucial factor in building strong customer relationships.
While leveraging data to personalize the customer journey is becoming more common, it's essential to do so in a way that respects individual privacy. The double opt-in approach is a clear demonstration of this balance. As organizations rely more on data-driven strategies to optimize customer interactions, maintaining a culture of transparency about data handling is becoming vital. This is particularly true in a digital world where users are more aware than ever of how their data is collected and utilized. By placing a premium on consent, businesses can cultivate a stronger relationship with their audience, fostering deeper engagement and loyalty. The implications of neglecting these privacy-centric strategies can be significant, potentially harming customer trust and eroding the hard-earned value of a strong customer base.
When we talk about data privacy and its connection to building better customer relationships, double opt-in processes stand out as a significant element. These methods, where a customer confirms their initial opt-in with a second, separate action (like clicking a link in an email), are gaining importance, particularly with increased focus on data privacy regulations.
Organizations are realizing that the simple act of requiring this double verification step can have a surprising influence on their ability to build trust. From the perspective of a data researcher, this double check offers a chance to cultivate a stronger connection between a company and its customers. It is often suggested that when people go through this added step, they are essentially signaling that they want to receive information from the company, not just passively tolerate it.
This heightened level of intent has demonstrable benefits. The increase in engagement is, from my perspective, one of the most interesting outcomes. When a customer actively confirms their desire to receive communications, it seems logical that they would be more engaged with those communications. Studies have shown that this can result in a significant boost to overall engagement levels, sometimes even as high as a 50% increase.
Furthermore, the impact isn't limited to just engagement. It appears that this higher level of commitment often translates into a better conversion rate. A customer who's taken the time to double opt-in is more likely to go through with a purchase, subscription, or other desired action. Some research shows an increase in conversion rates by 20-30% for companies who implement double opt-in compared to those that don't.
It’s worth mentioning that the increased focus on privacy has other beneficial implications. It appears that when customers believe their data is being handled with care, they tend to be less likely to switch brands. This 'stickiness' effect has been measured in the reduction of customer churn. Companies who emphasize privacy through measures like double opt-in sometimes report seeing churn rates fall by as much as 15%.
Interestingly, the trust fostered through double opt-in methods appears to extend to a customer’s willingness to recommend a company. When a customer feels respected and valued, they're more likely to share their positive experiences with others. Studies have shown that this can translate to an increase in referral rates of about 25%.
This greater degree of consent offers more than just a tick-box compliance exercise, it offers a richer insight into individual preferences. When a user takes the effort to confirm their interest, the information provided is often more reliable and allows for more effective behavioral segmentation. This increased understanding of customer behavior, in turn, can improve the effectiveness of marketing communications and potentially reduce the amount of wasted effort on less-engaged leads.
In this current climate of stricter data privacy expectations, particularly among younger demographics, businesses who want to succeed need to be attuned to these signals of intent. The shift towards more careful and considered engagement is a significant development in customer relations, especially as the understanding of ethical data practices continues to develop.
How Data Analytics is Revolutionizing Customer Relations in 2024 A Deep Dive into ROI Metrics - Automated Sentiment Analysis Tools Detect Customer Issues 4 Hours Earlier
Automated sentiment analysis tools are changing how businesses handle customer service by allowing them to spot potential issues up to four hours sooner than with older methods. This early detection lets companies respond proactively, leading to much better customer interaction and overall satisfaction. These tools analyze customer feedback in real-time, offering deeper insights than traditional surveys and giving companies a clearer view of how to adapt their offerings to changing customer preferences. Using AI in sentiment analysis also helps build loyalty by ensuring the services provided are more closely matched to what customers expect. As these technologies continue to grow, they are likely to have a big impact on how customer relationships are managed, showing that being able to respond promptly and accurately is vital for businesses in 2024. While offering benefits, it is important to consider the ethical implications surrounding the use of customer data.
In the realm of customer relations, the integration of automated sentiment analysis tools is emerging as a game-changer, especially in 2024. These tools are capable of sifting through vast amounts of customer feedback from various sources, offering a nuanced understanding of customer sentiment that goes beyond simple keywords. It's fascinating how they can detect emerging issues up to four hours earlier than conventional methods, like manual reviews. This capability allows companies to address problems proactively before they snowball into full-blown crises, enhancing the overall responsiveness and effectiveness of their customer service efforts.
The speed at which these tools analyze data is remarkable. They can dissect thousands of interactions each second, identifying crucial feedback that might slip through the cracks of a human-only review process. This is particularly useful for spotting emerging concerns about product faults or service hiccups. Moreover, these tools go beyond simply recognizing words. Using advanced natural language processing, they can interpret the emotions behind the words—whether a customer is happy, frustrated, or furious. This allows companies to craft more tailored and effective responses, ensuring customer interactions feel more personalized and considerate.
Furthermore, the ability to recognize sentiment trends over time is quite powerful. Businesses can utilize this capability to objectively gauge the impact of their customer service adjustments or new product launches. This link between sentiment analysis and tangible results allows them to understand how well their strategies are resonating with their customer base, providing a direct connection between data analytics and ROI.
Another interesting aspect of automated sentiment analysis is its ability to reduce human error. While human analysis is invaluable, it's prone to biases and inconsistency when dealing with vast amounts of data. Automated systems, on the other hand, offer a consistent and accurate evaluation of sentiment across different sources, diminishing the potential for mistakes that manual processes can produce.
There are tangible benefits to these systems. Some businesses have noted a remarkable increase in customer retention rates, up to 20%, due to their implementation. This suggests that customers feel valued and appreciated when their problems are addressed promptly and effectively. Moreover, the ability to foresee and avert customer issues leads to notable cost reductions. It's been reported that businesses can see savings of 15-30% due to fewer escalations of issues to major crisis levels, lessening the burden on the brand and operational budgets.
Beyond immediate issue resolution, automated sentiment analysis can be integrated into a continuous feedback loop. The insights gathered from these tools can be fed back into product development and marketing strategies, creating a cycle of improvement based on customer preferences. This constant evolution of products and services can contribute to a more loyal and engaged customer base.
The versatility of these systems also extends to data aggregation. Automated sentiment analysis tools can combine insights from multiple platforms—social media, email, chat—creating a more comprehensive understanding of the customer experience across various touchpoints. This holistic view can be incredibly insightful for tailoring approaches across the customer journey.
Finally, the potential synergy between sentiment analysis and predictive models is incredibly promising. By analyzing sentiment trends, companies can anticipate potential customer churn before it occurs, allowing them to intervene and retain more customers. This combined approach strengthens customer relationship strategies, highlighting the power of integrating data analytics into customer retention efforts.
While still a relatively new development in the customer relations space, it's clear that automated sentiment analysis tools have the potential to reshape how businesses interact with their customers in 2024. Their ability to quickly identify issues, tailor responses to individual sentiment, and connect customer feedback to business outcomes makes them a valuable tool for any organization striving to achieve better customer relations and ROI. However, as we delve deeper into this domain, we must also remain cognizant of the need to address any potential biases within the algorithms and ensure that the use of such systems complies with privacy standards.
How Data Analytics is Revolutionizing Customer Relations in 2024 A Deep Dive into ROI Metrics - Cross Platform Analytics Reveal 28% More Customer Touch Points
Across various digital platforms, the use of analytics has revealed that customers interact with businesses through 28% more touchpoints than previously recognized. This means companies now have a more complete picture of how their customers engage with them, from websites to social media to mobile apps. This expanded view of customer behavior allows businesses to develop more comprehensive strategies, tailor their offerings to each individual's preferences, and ultimately improve the overall user experience across all platforms. A key element of making the most of this increased visibility is consolidating the data from various sources into a single, integrated system. This allows organizations to respond more rapidly to customer needs and tailor interactions to different individuals. Such swift responses build a stronger bond with customers by fulfilling their expectations in a timely way. However, this broader view of customer interaction also brings up questions regarding the privacy of that information. As we move further into 2024, striking a balance between maximizing the benefits of cross-platform analytics and responsibly managing customer data will become increasingly vital to building and retaining trust.
Examining customer interactions across multiple platforms, like websites, apps, and social media, reveals a surprising amount of previously unseen interaction points. In fact, using cross-platform analytics consistently shows that businesses can uncover about 28% more of these touch points than traditional methods. It's a bit like looking at a puzzle with many more pieces than you initially thought—it reveals a more intricate picture of how people interact with brands in today's digital world.
This enhanced view of customer interactions is invaluable for building a cohesive understanding of customer behavior. It allows companies to create more robust customer profiles, moving beyond simple demographic information to include a wide range of behaviors across different channels. This unified perspective is essential for building more effective marketing strategies. For example, it helps companies pinpoint specific customer segments with greater accuracy, potentially allowing for hyper-targeted promotions or product recommendations that resonate more effectively.
One of the most useful aspects of cross-platform analytics is their ability to support real-time adjustments. It's no longer necessary to wait for large batches of data to be processed before making changes. Instead, businesses can analyze customer interactions as they occur, adapt their approaches in real-time, and deliver a much more responsive experience. This agility is becoming increasingly critical as customers demand faster, more personalized service.
Looking deeper, we find that cross-platform analytics don't just track current actions, but also offer insights into the likelihood of future customer behavior. It's intriguing that they can predict, with increasing accuracy, what customers may need or want next. This predictive capability enables businesses to proactively address customer needs and prepare for anticipated demands, streamlining operations and potentially getting ahead of future trends.
The sheer volume of data that cross-platform analytics handle can be overwhelming. However, a key aspect of their functionality is the ability to sift through the noise and identify truly valuable insights. They can help businesses focus on the most significant interaction points, those that truly drive engagement and help build loyalty. This kind of intelligent filtering can lead to more impactful and efficient strategies.
Moreover, these tools offer a unique lens through which businesses can compare the effectiveness of different channels. Companies can readily see which channels yield the highest return on investment, empowering them to make smarter allocation decisions. It's like a head-to-head competition between marketing channels, but instead of guesses, the data provides a clear view of each channel's performance, allowing companies to prioritize their efforts based on actual results.
By identifying previously hidden touchpoints, cross-platform analytics can help businesses streamline the customer journey. It's a bit like optimizing a complex network, removing any roadblocks that may impede a customer's experience. This leads to a smoother, more intuitive customer journey across all channels, boosting overall satisfaction and potentially increasing engagement.
Furthermore, a natural consequence of improved channel understanding and more accurate customer segmentation is increased efficiency. Businesses can reduce wasted marketing dollars by targeting specific segments more accurately, leading to better ROI and potentially a reduction in overall marketing costs.
The insights generated by cross-platform analytics aren't static; they feed into a continuous loop of improvement. As businesses gather more data, they can refine their marketing strategies, adjust their operations, and optimize for a better customer experience. It's like a self-improving system, always learning and adapting to provide the best possible customer interaction.
Finally, cross-platform analytics naturally foster collaboration between different teams. Marketing, sales, and customer support can leverage these insights to create a more unified, customer-centric approach. Instead of working in silos, teams gain a common language through data, which leads to more effective strategies and a more cohesive customer experience. This collaborative spirit highlights the transformative potential of data-driven insights in the ever-evolving landscape of customer relations.
More Posts from zdnetinside.com: