7 Critical Features of ServiceNow's Cloud Management That Transformed Enterprise Operations in 2024
7 Critical Features of ServiceNow's Cloud Management That Transformed Enterprise Operations in 2024 - Automated Resource Distribution System Cuts Cloud Costs by 35%
Automating the way cloud resources are allocated can significantly reduce cloud spending, potentially leading to cost reductions of up to 35%. This automation enhances the ability to monitor and match cloud resources with actual workloads. By optimizing virtual machine sizes and adopting cost management principles like those found in the FinOps framework, businesses can realize considerable savings. While tools exist to manage cloud costs, automating this process is a key step in streamlining expenses as organizations move more of their operations to the cloud. It promotes a more responsible approach to cloud expenditure, making it easier to track and control where money is spent.
It's fascinating how an automated system can potentially optimize cloud spending. The Automated Resource Distribution System (ARDS) seems to be a game-changer, particularly in its ability to predict resource needs based on usage patterns. By essentially anticipating demand and adjusting resource allocation in real-time, it aims to significantly reduce wasted resources and, in turn, cloud expenses. Reportedly, some companies have seen a 35% reduction in costs, which is impressive.
The use of advanced algorithms and machine learning within ARDS is interesting. It suggests that the system learns from historical data and adjusts its resource allocation strategy over time, likely becoming more accurate and efficient in predicting future resource needs. This continuous learning aspect could be crucial for environments with fluctuating workloads, making the system more adaptive and ultimately more cost-effective.
However, I'm a bit cautious about relying solely on predictions. While automated systems can be efficient, there's always the risk of unforeseen events impacting resource needs. It's important to consider the potential for inaccuracies in predictions and ensure that the system's decision-making process is transparent and allows for human intervention when necessary.
From an operational standpoint, the consolidation of resource management offered by ARDS seems promising. It could free up IT teams to focus on more complex tasks rather than manual resource allocation, a desirable outcome. Furthermore, the ability to simulate different resource allocation scenarios and visually present the cost impact is a valuable tool for IT managers, enabling data-driven decision-making regarding cloud optimization.
The system's reported ability to reduce downtime by proactively adjusting resource allocation to match demand is noteworthy. However, the success of this aspect is likely dependent on the accuracy of its predictions and the system's ability to react quickly to sudden changes in workload. Also, the ability to support a multi-cloud environment gives the organization more options and flexibility in choosing cloud providers based on performance and cost.
Ultimately, ARDS appears to be a valuable tool in optimizing cloud resource utilization and costs. However, as with any complex system, it's crucial to thoroughly evaluate its capabilities and limitations before implementing it. The potential benefits are significant, but careful planning and monitoring will likely be needed to maximize the system's efficacy and minimize potential downsides.
7 Critical Features of ServiceNow's Cloud Management That Transformed Enterprise Operations in 2024 - Multi-Cloud Dashboard Integration with AWS and Azure Now Standard
In the evolving landscape of cloud management, the ability to seamlessly integrate and manage resources across different cloud providers has become paramount. It's no longer surprising that multi-cloud dashboard integration with major platforms like AWS and Azure is now considered a standard feature in many tools. This shift signifies a growing need for enterprises to gain a holistic view of their cloud operations, regardless of the underlying platform.
Having a unified dashboard to monitor AWS and Azure services is a huge step forward. It allows businesses to aggregate performance data, track resource utilization, and manage security across a broader cloud footprint. However, the real value comes from the added flexibility this provides. It's about having the choice to utilize the best features of each cloud environment.
Solutions like Azure Arc, designed to extend Azure's management capabilities across other cloud environments and on-premises, are part of this trend. The ability to manage everything from one place is very attractive, especially as the complexity of multi-cloud environments can become overwhelming quickly.
And, as security becomes increasingly important, there are related developments to note. Frameworks like Open Cybersecurity Schema Framework are encouraging better security data sharing across cloud platforms, making it easier to implement consistent security policies and procedures across a multi-cloud architecture. While this standardization helps, the overall security posture of such a diverse system remains a concern.
In the end, the movement toward standard multi-cloud dashboard integration is driving a change in how businesses view cloud management. It's about breaking down silos and creating a more flexible and integrated operating model for a dynamic IT landscape. Yet, whether the promise of a truly simplified multi-cloud experience has been fully achieved remains to be seen.
It's interesting to see how ServiceNow's cloud management capabilities are evolving to handle the complexities of multi-cloud environments. The ability to integrate dashboards from different cloud providers like AWS and Azure is becoming increasingly important. While it used to be a challenge to manage resources and get a clear view of performance and costs across multiple clouds, this new integration, which is now standard, appears to simplify that significantly.
Having a single view of real-time metrics and performance indicators from both AWS and Azure can be very valuable for operational efficiency. Decisions can be made faster with a consolidated view of data. It seems that this sort of unified view is also helping to establish consistent security policies and practices across different clouds. This aspect is critical in a multi-cloud environment where ensuring a standardized security posture can be tough otherwise.
However, one of the main challenges with managing multiple cloud environments is resource sprawl – losing track of what you have and where it's deployed. The ability to monitor resource usage across both AWS and Azure in a single dashboard can address this. It potentially allows teams to identify any underutilized resources and adjust allocation as needed.
Another important aspect is cost visibility. While the idea of cost optimization and control has been discussed before, this integrated dashboard approach appears to provide a much more transparent look at spending across different cloud platforms. I'm curious how this is impacting decision-making regarding resource allocation and potentially revealing previously unknown cost areas.
Besides efficiency, there are also user experience benefits. Imagine not having to switch between multiple consoles to monitor and manage different cloud environments. A streamlined and unified approach makes a lot of sense. Furthermore, if incidents and issues can be tracked and resolved through a single dashboard regardless of where they occur within AWS or Azure, that can be a big win in terms of response time and resolution speed.
The integration also potentially enables the automation of actions based on real-time insights gleaned from the dashboards. Based on specific metrics, a system might be able to automatically scale resources or even send out alerts when thresholds are hit. The overall effect of automation is to likely free up IT personnel to focus on more strategic initiatives.
In addition to internal efficiency, there's a connection to the customer experience. By understanding how both AWS and Azure environments are performing, and how they're impacting customers, companies can make decisions that improve services and create a more responsive and personalized experience.
Finally, the management of data itself seems to benefit from the integration. A unified view of where data is stored and how it moves across cloud platforms should make it easier to ensure compliance with various regulations and data handling requirements. This element is often overlooked but very important in today's compliance-focused environment.
While the overall trend of multi-cloud is interesting, it does require careful consideration. The new standard multi-cloud dashboards are a positive step in simplifying the complexity and enabling better management of these complex environments. But only time will tell how truly impactful these changes will be across various industries and business scenarios.
7 Critical Features of ServiceNow's Cloud Management That Transformed Enterprise Operations in 2024 - Real-Time Security Monitoring Through AI Pattern Detection
Real-time security monitoring, powered by AI's ability to identify patterns, has become a crucial part of managing today's complex cloud environments, especially within ServiceNow's offerings. The use of AI algorithms allows systems to analyze network traffic for anomalies, enabling swift detection of potential security incidents like malware or data breaches. This move towards AI-powered security is significant because it shifts the focus away from tedious manual log analysis, allowing security teams to react faster and more effectively to threats. Furthermore, the ability to gain real-time insights into security vulnerabilities via AI-driven metrics, coupled with consistent updates across security applications, supports a more preventative approach to security.
There are concerns, though. One major issue is the potential for false positives, where AI might flag normal activity as a threat. The accuracy of AI-driven security is paramount, as misinterpreting data can disrupt operations or lead to unnecessary responses. Striking a balance between effective threat detection and minimizing false alarms remains a key challenge in the development and implementation of AI-powered security systems. The need for human oversight in validating AI's findings is likely to continue as a critical part of maintaining a robust cybersecurity posture.
Real-time security monitoring using AI-powered pattern detection is transforming how we approach cybersecurity in 2024. It's fascinating how these systems can sift through massive amounts of data, identifying subtle deviations that might indicate a security threat.
One of the most compelling aspects is the speed at which threats can now be detected. Instead of security teams potentially taking days or weeks to notice an unusual pattern, AI can often detect anomalies within seconds. This rapid identification is critical in preventing a breach from escalating, minimizing potential damage. While promising, this speed raises questions about the possibility of generating an excessive number of false alarms. Luckily, modern AI algorithms combined with larger datasets have helped to improve accuracy, pushing false positive rates down to as low as 1%. This level of precision is crucial; it allows security personnel to focus on the genuine threats, significantly boosting their overall effectiveness.
Another aspect of AI in security is its ability to analyze user and device behavior. By building baseline profiles, the system learns what constitutes normal activity for a particular user or device. Then, any significant deviation from that established norm triggers an alert, suggesting a potential incident. This approach not only highlights anomalies but provides a richer understanding of the entire network environment.
Furthermore, AI-powered security systems are designed to evolve and adapt. They learn from past security incidents, continuously refining their threat detection capabilities. This continuous learning process is vital because the landscape of cybersecurity threats is constantly changing. As new attack vectors emerge, AI systems are able to incorporate this information, helping to keep defenses updated and effective against the latest schemes.
It's also interesting how some AI systems can trigger automated responses. When a threat is detected, the system can initiate actions like isolating an infected system, all within seconds. This level of automation is essential in containing incidents quickly and effectively, often before major damage is done. This contrasts sharply with the slower, often manual, processes of the past.
These AI-driven security features don't necessarily require a complete overhaul of existing security infrastructure. They can often integrate well with established SIEM systems, improving their capabilities without a major disruption. This integration capability is valuable as it allows businesses to leverage their current investments while enhancing security.
While protecting against breaches is paramount, these advanced systems can also assist with enforcing data privacy regulations. By monitoring activity, the AI can detect actions that violate data privacy policies, providing an early warning to security teams. This proactive approach is essential as penalties for non-compliance are becoming more severe.
Beyond threat detection, AI-powered pattern detection can provide deeper insights into security posture, optimizing resource allocation. By analyzing patterns in security-related data, organizations can allocate resources – like budget and personnel – to areas that pose the highest risks. This approach leads to a more strategic and efficient distribution of security resources.
Another benefit is that these systems can simultaneously monitor for a wider range of threats. They're not limited to just external attacks; they can also monitor for internal misuse. This comprehensive monitoring capability is essential in today's complex threat environment where attacks can come from a variety of sources.
Finally, it's worth noting that real-time monitoring and its associated logging greatly enhance an organization's ability to perform audits and maintain compliance with industry standards. Automatic reporting on security events and responses greatly simplifies the compliance process, helping to ensure organizations meet their regulatory obligations.
While AI-powered security solutions are still developing, it's evident that real-time monitoring driven by pattern detection is becoming an indispensable tool in the ongoing battle against cyberattacks. It’s clear that this technology is changing the landscape of cybersecurity, but continued research and responsible development will be crucial in maximizing its benefits while mitigating any potential risks.
7 Critical Features of ServiceNow's Cloud Management That Transformed Enterprise Operations in 2024 - Self-Service Portal Reduces IT Support Tickets by 60%
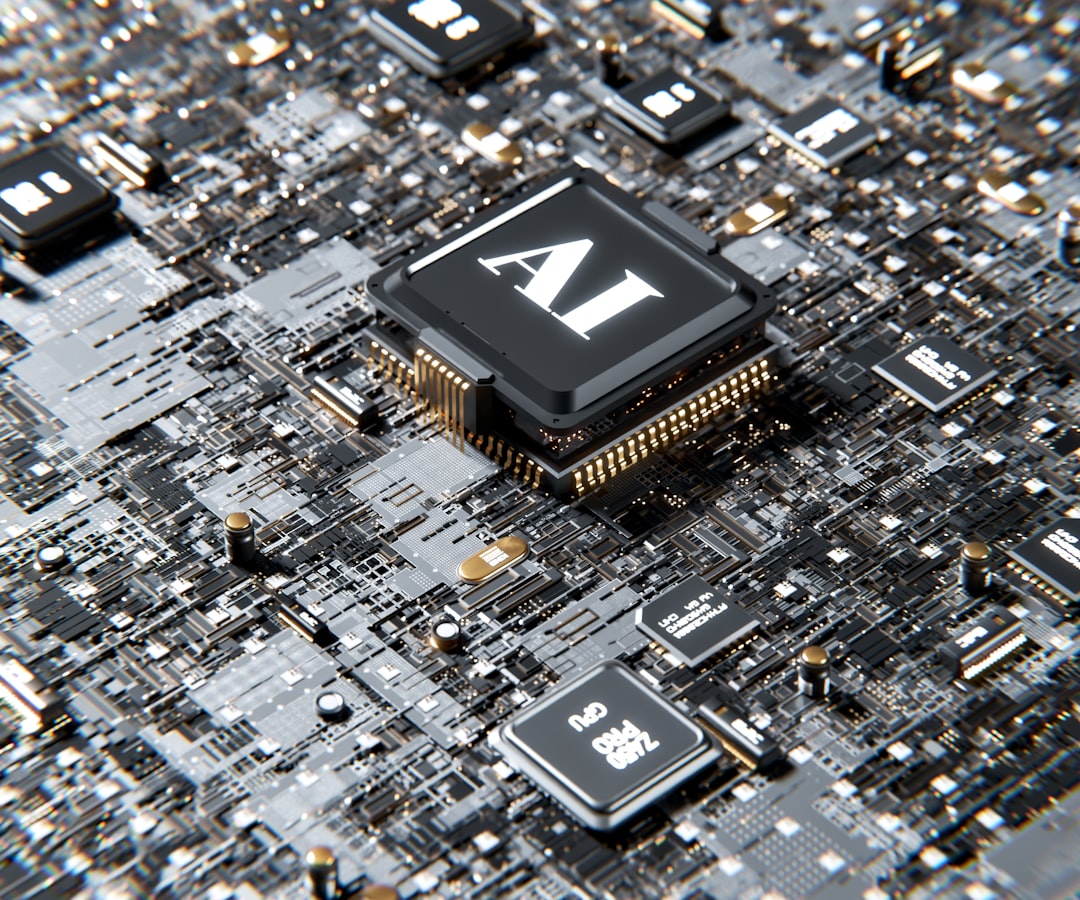
Introducing a self-service portal has proven to be a powerful way to reshape how IT support operates, leading to a significant drop in support tickets—as much as 60% in some cases. This is achieved by enabling users to find solutions and resolve issues on their own. Tools like knowledge bases and the ability to log their own incidents empower users, reducing the strain on support teams and boosting overall IT efficiency. Interestingly, this trend of self-service is being driven by a growing demand from users—over 95% of businesses have reported a significant increase in requests for this type of support. It appears that users are increasingly valuing the speed and convenience of resolving their own issues. Moreover, these portals often contribute to smoother onboarding experiences for new users. They can access needed information quickly and directly, without waiting for a support agent. The benefits of self-service are clear—it streamlines operations and meets modern user expectations for easily accessible tech support. However, it's worth noting that there are potential downsides, like the need for careful portal design and maintenance to ensure that users find the information they need easily and efficiently.
Observing the trend towards self-service portals, it's intriguing how they've been linked to a substantial reduction in IT support tickets. Reports indicate a decrease of up to 60%, which suggests a significant shift in how employees are approaching IT-related issues. Instead of relying on traditional support channels, they seem to be increasingly comfortable seeking solutions themselves, utilizing the information and tools available through these portals.
This self-reliance, fostered by self-service, appears to be empowering users. It's been reported that well-designed portals can yield high user satisfaction rates, reaching 70%. This positive experience likely stems from the ability to find answers quickly without having to wait for IT assistance, a significant improvement in employee experience compared to traditional support workflows.
This shift towards self-service is not only impacting user satisfaction but also impacting support team efficiency. The ability to resolve issues independently naturally reduces the Mean Time to Resolution (MTTR). Studies suggest that MTTR can be reduced by up to 50% when organizations adopt self-service, which translates to faster resolution times and a reduction in disruptions to ongoing operations.
This increase in efficiency can have a major impact on operational costs. Estimates suggest that handling a ticket through a self-service portal can cost companies $5 to $10 less than traditional support methods. This difference can be significant for businesses with high volumes of support tickets. While it might not seem like a lot per ticket, it quickly adds up over time, potentially leading to significant cost savings for organizations.
It's interesting to look at the impact self-service has had on traditional support ticket volumes. Previously, a typical IT help desk might handle 80% of support requests manually. With the adoption of self-service, that proportion can drop to around 40%, freeing up IT personnel to focus on more complex tasks that require their specialized expertise. This shift in workload suggests a better allocation of resources.
However, beyond the initial cost savings and improved user experience, self-service portals provide a wealth of data through user interactions and the types of issues they encounter. By analyzing this information, companies can get insights into trends and frequently asked questions. This allows them to make proactive improvements to their services, potentially reducing the number of repetitive requests that plague traditional support systems.
One of the challenges organizations face as they grow is maintaining high-quality IT support for an expanding workforce. Fortunately, self-service portals have a distinct advantage: they scale easily. This scalability is essential as it allows the support system to adapt to the needs of a growing organization without having to hire additional support staff in the same proportion.
Furthermore, the availability of resources like knowledge bases and tutorials within many self-service platforms is likely improving employee onboarding. The ability to access training materials immediately can significantly accelerate the process of acclimating new employees to company systems.
Finally, it's worth considering the impact self-service has had on the morale of IT staff. A reduced ticket load provides them with more time to tackle more strategic and complex projects instead of being bogged down with routine tasks. This shift in duties could lead to greater job satisfaction, which, in turn, may lead to higher retention rates among skilled IT professionals.
It's evident that successful implementations of self-service portals lead to a cultural shift in how IT service management is perceived within a company. IT transitions from a primarily reactive support function to a more proactive provider of solutions. This change can enhance collaboration and innovation throughout the organization as employees and IT teams work together to solve problems in new and efficient ways.
While there's certainly a shift towards self-service, there are potential areas of concern. The quality of the self-service portal will play a large role in the success rate. If the knowledge bases are out-of-date or unclear, it may lead to further frustration. Also, there is a risk of employees becoming overly reliant on self-service and never seeking the appropriate expertise when a problem requires it. However, from what we have observed, the benefits of self-service have outweighed these concerns.
In conclusion, it seems clear that self-service is a rapidly evolving trend that is having a significant impact on the way IT support is delivered in today's enterprise. It will be interesting to see how these technologies evolve and how organizations continue to optimize their implementation and leverage the various benefits.
7 Critical Features of ServiceNow's Cloud Management That Transformed Enterprise Operations in 2024 - Advanced Workload Optimization with Predictive Scaling
"Advanced Workload Optimization with Predictive Scaling" has become increasingly important in cloud management, especially for businesses wanting to boost efficiency this year. This approach uses smart algorithms and AI to predict how much work a system will handle, which helps automatically adjust resources as needed. While promising, companies have to deal with the fact that workloads can change a lot and that predictions aren't always perfect. The way autoscaling is improving is part of a larger trend where we see efforts to get the most out of resources while keeping service quality high, even when the amount of work changes. With businesses relying more on AI solutions, figuring out the best way to put these systems in place is essential for getting the most out of cloud resources. There are obstacles to overcome, but when handled carefully, predictive scaling can be useful.
Predictive scaling relies on sophisticated algorithms, often time-series forecasting models, that can process vast amounts of data to predict workload changes with surprising accuracy. This ability to anticipate fluctuations is a key differentiator from older scaling methods. It's fascinating how these systems learn from historical performance data, including past usage patterns, to make educated guesses about future needs. They can even take into account seasonal trends or specific historical events, like the usual spike in activity at the end of a month.
Unlike traditional scaling approaches, which can be sluggish in reacting to changes, predictive scaling adjusts resources dynamically. This means that resources can be scaled up or down in real-time as needed, making it especially useful when dealing with sudden spikes or drops in traffic. I wonder how the accuracy of these predictions might vary across different types of workloads. It also presents an opportunity for substantial cost savings, not just from optimization but also because it helps reduce wasted resources. By predicting resource needs instead of over-provisioning, businesses can avoid unnecessary costs, especially in environments with unpredictable workloads.
It's also becoming increasingly common to integrate predictive scaling into CI/CD pipelines. This means that resource allocation can automatically adapt to the anticipated load from new deployments, making releases smoother and reducing disruptions. This integration seems like a smart approach to streamline the deployment process, and I'm curious how the feedback loops from these integrations are being used to further improve the predictive models.
Going beyond just looking at historical patterns, some advanced methods also factor in user behavior. By tracking user interactions, the systems can anticipate how user demand might shift in response to external events, such as promotions or marketing campaigns. However, it's crucial to do this in a way that respects user privacy and doesn't create unintended consequences.
Many organizations are exploring hybrid cloud environments to optimize the benefits of predictive scaling. By balancing workloads between private and public clouds, businesses can improve flexibility and efficiency, which should provide a cost advantage while maintaining performance. It would be interesting to see how the predictive models handle the intricacies of hybrid environments where different resource pricing models are at play.
Predictive models are not only good at predicting typical workload fluctuations but also learn from unusual events. By incorporating feedback mechanisms that examine deviations from the norm, the models become more accurate and resilient over time. This ability to learn from unusual situations adds a robustness that is essential in dynamically changing environments. This adaptive nature seems crucial in environments where operations can shift unexpectedly.
Organizations that have effectively used predictive scaling often report significantly less downtime. By proactively allocating resources in anticipation of demand increases, they are able to maintain service levels and enhance the user experience. It's crucial that predictions are accurate in this context since incorrect assumptions can lead to problems. It's interesting how the systems deal with the uncertainty inherent in real-world conditions.
One valuable aspect of predictive scaling is its ability to provide consolidated insights across different cloud environments. By aggregating data from multiple cloud platforms, it allows for a comprehensive understanding of resource usage, which is extremely useful for companies managing complex multi-cloud strategies. This type of aggregated view can help companies make better decisions regarding the selection and use of various cloud providers.
While there are still areas to research and potential challenges to address, it seems that predictive workload optimization is a powerful tool for businesses navigating the challenges of modern cloud environments. The ability to anticipate future needs, adjust resources dynamically, and minimize downtime makes it an approach worthy of further investigation.
7 Critical Features of ServiceNow's Cloud Management That Transformed Enterprise Operations in 2024 - Cross-Platform Data Management with Zero-Trust Architecture
The concept of "Cross-Platform Data Management with Zero-Trust Architecture" has become increasingly vital as businesses embrace cloud-native environments and operate across multiple cloud platforms. This approach fundamentally redefines data security and access by rejecting the notion of implicit trust. No user or system, regardless of its location or network connection, is automatically granted access to data. This shift creates a new set of challenges as organizations grapple with integrating Zero Trust principles into their existing infrastructure, which often includes both modern and legacy systems. The dynamic nature of cloud environments further complicates this integration process.
Central to this approach is the idea that identity is the new security perimeter. This necessitates robust identity and access management (IAM) solutions, including techniques like multi-factor authentication (MFA) and single sign-on (SSO). These solutions become critical for protecting sensitive data across diverse operational landscapes. By rigorously enforcing these access controls, businesses can potentially strengthen their defenses against data breaches and other security threats. In essence, effectively implementing a Zero Trust architecture becomes crucial for organizations aiming to manage data securely across multiple platforms and in increasingly complex environments. Whether this approach will actually achieve its security goals remains to be seen.
Cross-platform data management is becoming increasingly complex, especially as organizations adopt a mix of cloud environments. Zero-Trust Architecture (ZTA) is emerging as a key approach to handle these complexities while bolstering security. It's not about eliminating trust entirely, but instead about verifying every access request, regardless of network location or user's assumed status. This core principle dramatically reduces the risk associated with implicit trust, which can be a major weakness in many systems.
One of the immediate advantages is the ability to tightly control how data flows between cloud environments. ZTA gives granular control over access rights, allowing you to define who can see which pieces of data, and when. This precision is important, as it helps maintain the integrity and confidentiality of sensitive information.
Interestingly, adopting ZTA often shrinks the overall attack surface of an enterprise. Studies suggest that organizations can see reductions in insider threat incidents by over 60% when implementing a Zero-Trust approach. This is because every access request is meticulously checked, which is a big change from assuming a user has legitimate access just because they're within a particular network segment.
Moving away from static security, ZTA encourages context-aware, dynamic policies. This means security measures adjust based on a variety of factors like the user's current activity, device status, location, and the sensitivity of the data being accessed. This adaptability helps build defenses that are better prepared for the evolving nature of cybersecurity threats.
One cool feature of pairing cross-platform data management with ZTA is the opportunity to use micro-segmentation. This is where the network is sliced into smaller sections, which allows for more precise access controls. Should a breach occur, micro-segmentation reduces the potential damage by limiting the ability of an attacker to move freely within the network.
Another interesting aspect of ZTA is its inherent scalability across different cloud providers. This flexibility allows organizations to react quickly to changes in their business, adopting new cloud services without major hurdles. The adaptability of the security framework keeps up with the business needs, making the system more robust and easier to maintain.
ZTA can help streamline compliance efforts. The increased granularity of access logs and policies makes auditing much easier, which can help simplify the compliance process. Being able to show detailed evidence of access patterns and decisions reduces some of the burden that comes with complying with regulations.
Modern ZTA implementations tend to include AI and automation for continuous monitoring. This real-time approach can pick up on unusual user activity or patterns that indicate a potential breach, providing a more proactive security posture. Rather than just responding to security incidents, the system proactively searches for them, using AI to recognize anomalies in data flows.
The adoption of ZTA often leads to increased trust from customers. By demonstrating that they take data security very seriously and have transparent policies in place, companies build confidence that client data is safe. This can strengthen relationships and boost customer satisfaction, especially as data privacy concerns are top of mind for many users.
What's surprising to some is that ZTA can be integrated into legacy systems. This allows companies to adopt ZTA without a complete overhaul of their existing infrastructure. Careful design and planning are key, but it allows organizations to gradually move to Zero-Trust without disrupting their current operations.
While ZTA has clear benefits, it's not without its own set of challenges. The complexity of managing and maintaining these frameworks is a concern for some teams. However, the advantages of using ZTA across multiple platforms for enhanced data control and security are compelling and may be necessary for organizations that need the highest level of assurance that data is secure and accessible only to authorized users.
7 Critical Features of ServiceNow's Cloud Management That Transformed Enterprise Operations in 2024 - Cloud Performance Analytics with Machine Learning Support
ServiceNow's Cloud Performance Analytics has taken a leap forward in 2024 with the integration of machine learning. This addition allows for a more sophisticated approach to monitoring and managing cloud resources. It now analyzes key performance metrics, like CPU and memory usage, in real-time, giving organizations a much clearer view of how their cloud is operating. This real-time information helps automate and optimize resource allocation, leading to improved efficiency and cost management. The ability to create custom reports and visually engaging dashboards makes it easier for users to understand performance trends, spot issues, and communicate insights across teams. The proactive nature of these analytics, ensuring cloud resources are utilized effectively and costs are kept in check, has become a crucial element in strategic decision-making within many organizations.
However, this shift towards more advanced analytics also highlights a need for cautious consideration. The accuracy of the insights provided by machine learning models is crucial, as relying too heavily on automated recommendations can sometimes lead to unforeseen issues. There's always a potential for inaccurate predictions or biases in the algorithms, which can negatively impact operations if not carefully monitored. So, while the potential benefits of Cloud Performance Analytics with machine learning are undeniably substantial, it's important to maintain a balance between relying on the automated insights and incorporating human oversight and judgment in critical decision-making processes.
Cloud performance analytics has been enhanced by integrating machine learning, offering a fresh approach to managing cloud resources. It's interesting how these systems can analyze things like CPU usage and memory to figure out the best way to use resources. Essentially, these tools use algorithms to make educated guesses on how to optimize resource allocation. While seemingly straightforward, it's intriguing how this optimization can translate into real-world benefits for businesses.
One notable aspect is how these analytics can be used to measure progress towards specific business goals. By tracking performance against established targets, organizations can gain insights into operational efficiency and identify areas that could be improved. It's like having a dashboard that shows you where you're winning and where you need to focus your attention. But the question I find interesting is how accurate are these insights? Can these models truly provide an unbiased view of performance or are there biases built into the system itself?
Furthermore, a key strength of these analytics lies in their ability to provide readily accessible visualizations of key performance indicators (KPIs). The integration of dashboards and real-time reporting provides users with a fast way to understand trends and gain valuable insights without diving deep into complex data sets. This type of visibility is critical for decision-making. I'm curious about the scalability of these dashboarding capabilities. How well can these tools handle a growing volume of data and users without sacrificing performance?
It's also interesting to see how these analytics have been integrated with project management tools. This convergence of data, visualization, and project management can lead to enhanced decision-making capabilities during the project lifecycle. Yet, I'm a little wary about the potential over-reliance on data visualization. It's important that these visualizations are easy to understand and don't obscure critical aspects of projects, especially in situations that involve human judgment.
One of the main reasons to employ cloud performance analytics is the ability to monitor crucial cloud metrics. By closely tracking things like resource availability and costs, businesses can operate their cloud infrastructure efficiently. This proactive approach to resource management can help reduce unexpected downtime and minimize wasteful spending. It seems like a good idea, but it's a constant balancing act between achieving desired performance levels and the actual costs of achieving them.
Real-time access to data empowers faster decision-making. With instantaneous insights into performance, organizations can quickly prioritize tasks and allocate resources to the areas where they will make the biggest impact. This translates to a more agile response to business needs. However, I'm a bit concerned about the potential for bias in how decisions are made in this real-time environment.
Moreover, the wider distribution of performance insights encourages stronger collaboration between teams. Improved communication and data sharing facilitate informed decision-making across various parts of an organization. But, ensuring consistent interpretation of performance metrics across departments is a real challenge and requires careful management.
Overall, the addition of cloud performance analytics with machine learning support enhances data analysis capabilities and strengthens the link between data and strategic decision-making. By providing a more holistic view of operations, these analytics tools empower organizations to make better informed decisions and optimize their cloud resources for improved performance and cost efficiency. However, these are relatively new tools and I believe a lot of further research needs to be done on data reliability and the impact of potential bias on decision-making within these systems.
More Posts from :