The Rise of AI-Driven IT Solutions How Businesses Are Adapting in 2024
The Rise of AI-Driven IT Solutions How Businesses Are Adapting in 2024 - AI-driven IT solutions dominate enterprise software market
The enterprise software market is experiencing a rapid reshaping in 2024, with AI-powered solutions taking center stage. The surge in investment in generative AI, particularly among larger companies, highlights the growing understanding of its potential to revolutionize business. A substantial number of larger businesses have integrated AI into their operations, and early adopters are keen to accelerate its deployment across more areas. The arrival of AI systems capable of handling various input formats, like text and images, adds another layer of flexibility. This adaptable nature allows businesses to streamline their processes and improve interactions with customers in new ways. Furthermore, the integration of AI is fueling a broader push toward improved efficiency and the creation of new innovations, signifying a foundational shift in the way enterprise software functions and impacts various sectors. The future trajectory points toward a landscape where AI will increasingly drive the core functionalities of businesses, impacting everything from internal workflows to external engagement.
The enterprise software landscape is undergoing a dramatic shift, with AI-driven solutions rapidly gaining traction. Early indicators suggest a substantial investment in generative AI, with major enterprises allocating a portion of their software budgets to these technologies in 2023. Surveys reveal a growing adoption rate, particularly among larger organizations, with many already leveraging AI and planning to further integrate it into their operations. This is reflected in the rising trend of tailored, in-house AI solutions.
We're also witnessing the emergence of multimodal AI systems, which are capable of understanding and responding to a wider array of user inputs, beyond just text. This adaptability seems to be linked with the expansion of cloud platforms, as evidenced by the notable growth experienced by companies like Microsoft leveraging AI within their cloud infrastructure. It appears that AI is enhancing operational effectiveness and driving innovation across numerous sectors, fundamentally altering how enterprises approach digital transformation.
The industry is evolving towards a more AI-centric approach to managing processes and interacting with customers. Forecasts predict a continued upward trajectory for the artificial intelligence software market, suggesting a substantial growth in its overall size in the coming years. Looking further out, the vision is that businesses will embed data seamlessly into various aspects of their organizations – leading to automated actions and potentially a 'data-ubiquitous' future by 2030. This potential future raises interesting questions about how organizations will adapt and manage data in this environment, especially from a governance and compliance perspective.
While the potential benefits are substantial, the integration process is not without challenges. Maintaining compatibility with existing IT infrastructure can be problematic, leading to delays and potentially hindering the realization of intended gains. Furthermore, data governance issues are becoming more prominent as AI systems require meticulous data handling and careful compliance with evolving regulations to avoid legal consequences or reputational damage. The talent landscape is also shifting, with the demand for AI-related expertise on the rise, highlighting a potential skills gap within IT organizations as traditional roles evolve to accommodate these changes.
The Rise of AI-Driven IT Solutions How Businesses Are Adapting in 2024 - Machine learning transforms business decision-making processes
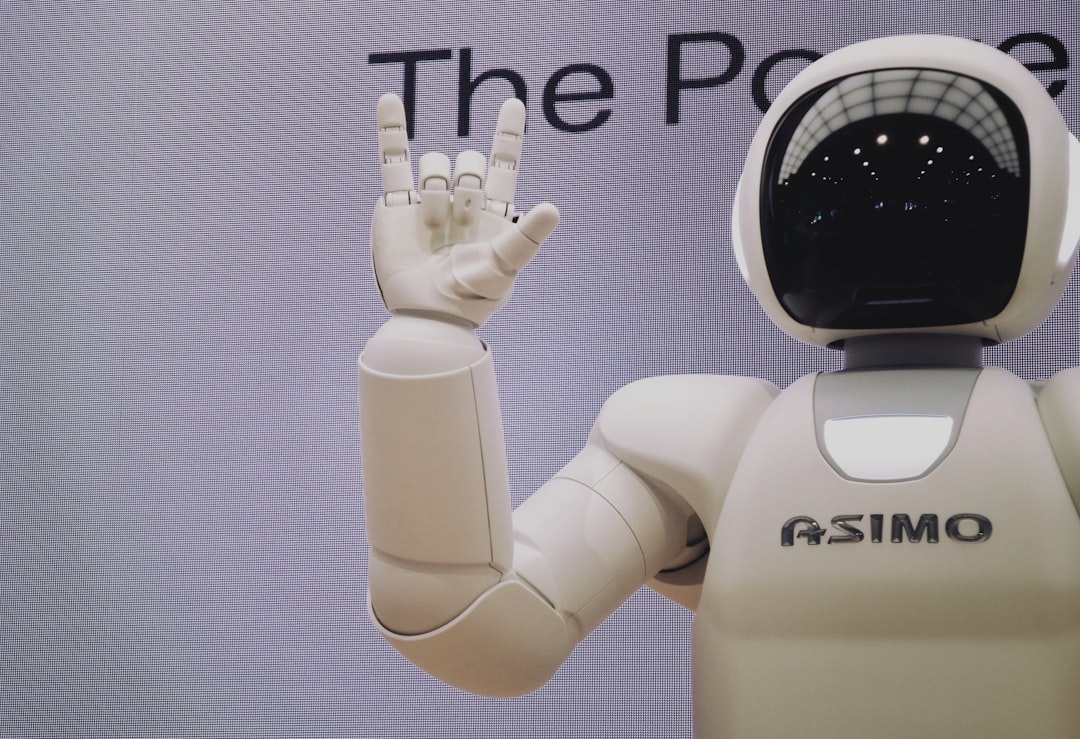
Machine learning is reshaping how businesses make decisions in 2024, offering tools to enhance efficiency and predict future outcomes. While its widespread adoption is still in its early stages, with only a small fraction of businesses fully utilizing AI and machine learning, the potential is undeniable. For example, using AI to forecast demand in supply chains can substantially lower error rates, highlighting the technology's power to improve accuracy and reliability.
The concept of "decision intelligence" is gaining traction, emphasizing the need to use AI to help, not replace, human judgment, especially in crucial decisions. Businesses are pushing for better returns on their investments in AI, and the focus is shifting to smoothly incorporating these technologies into their decision processes. The move toward a world where data is everywhere and decisions are automated offers both exciting possibilities and inherent challenges, notably with data governance and the need for specific skills. There's a tension between relying on AI and maintaining human oversight, especially for high-impact decisions. It remains to be seen how successfully businesses will balance automation with human judgment in their quest for improved decision-making.
The way businesses make decisions is being fundamentally altered by machine learning, a subset of artificial intelligence. It's becoming increasingly apparent that machine learning algorithms can sift through massive amounts of data much quicker than human analysts, enabling decisions to be made more promptly and with greater awareness. This acceleration can shorten the time between collecting data and gaining actionable insights, potentially from weeks to just minutes.
Beyond simple speed, machine learning has a predictive edge over traditional statistical approaches. It has the ability to uncover complex relationships and patterns within data that might escape human notice, allowing businesses to anticipate customer behaviors and market trends with increased accuracy. Research suggests a strong link between leveraging machine learning for decision-making and improved business performance. Some companies report revenue increases of 10% or more, indicating that advanced data analytics can translate directly to better operational outcomes.
The potential applications of machine learning extend to real-time decision-making, enabling companies to react swiftly to dynamic market conditions. This capability to adapt immediately can be a critical competitive advantage, especially in rapidly evolving industries. In addition to potentially improving revenue, machine learning is increasingly being used to reduce costs. By automating routine tasks, organizations free up human employees to focus on higher-level activities, leading to a more effective use of resources.
Looking across various business sectors, it's noteworthy that organizations employing machine learning for decision-making tend to report a higher level of innovation. The culture of insight that develops with these tools seems to encourage experimentation and the creation of new ideas. However, it's important to remember that reliance on machine learning introduces new risks. Inadequately trained models can result in suboptimal decisions, making rigorous validation and constant monitoring of machine learning models essential.
The impact of machine learning is not restricted to customer-facing activities. Back-office operations, like supply chain management and finance, are also seeing significant changes. Optimizing these areas with machine learning can lead to more efficiency and cost savings, contributing to the overall success of an organization. As companies integrate machine learning more deeply into decision-making processes, the need for expertise in both data science and specific business areas grows. Combining data science skills with an understanding of particular business domains is crucial for achieving the full potential of these technologies.
One of the challenges is that the complexity of machine learning interfaces and tools can be daunting for decision-makers who aren't familiar with data science. This implies that organizations need to invest in education and training programs so that all relevant stakeholders can confidently use these advanced tools to their fullest extent. As we move forward, successfully integrating machine learning into decision-making will hinge on building a workforce that can bridge the gap between the technical aspects of AI and the practical application within business settings.
The Rise of AI-Driven IT Solutions How Businesses Are Adapting in 2024 - AI chatbots become standard for customer service operations
AI chatbots are quickly becoming the standard for how businesses interact with customers. They're taking over many of the routine tasks in customer service, allowing human agents to handle more complex problems. A growing number of companies are implementing these chatbots, leading to noticeable cost savings and improved customer experiences in many cases. This trend signifies a significant shift in how businesses provide support, moving towards greater automation. However, this shift also introduces potential concerns. Maintaining a human-centric approach and adapting the technology to provide personalized experiences for customers can be challenging. These concerns need to be considered alongside the benefits as companies continue to integrate AI into their customer service strategies. Ultimately, the growing use of AI chatbots in customer service reflects the broader industry-wide move towards more efficient and AI-driven IT solutions, forcing businesses to adapt and innovate within the evolving digital landscape.
AI chatbots are quickly becoming the standard for handling customer service inquiries. A growing number of businesses are adopting them, with surveys indicating a significant portion of customer service leaders now using chatbot or conversational AI platforms. This shift appears to be driven by consumers' increasing preference for speed and efficiency. Companies are finding that integrating these AI systems can lead to a substantial reduction in operational costs, potentially around 30%, by automating a large portion of routine interactions. Human agents can then focus on more intricate problems.
One of the more interesting aspects of these chatbots is their 24/7 availability. Businesses are now able to provide customer service around the clock, which seems to be improving customer satisfaction metrics. Additionally, these systems can scale to handle a large influx of inquiries, proving valuable during peak periods like holiday shopping seasons when call volumes spike. Unlike traditional rule-based systems, these AI-powered chatbots continuously learn from interactions, allowing them to refine their responses over time. This learning capability can enhance response accuracy and tailor interactions to individual users.
Furthermore, AI-driven customer service holds the potential for personalization. By analyzing past interactions, these systems can offer tailored recommendations and information, contributing to both increased customer satisfaction and potential sales. Some more advanced AI systems even offer multilingual support, expanding a business's reach to a global customer base. This broader access might be a driver for inclusivity. There's also a noticeable improvement in response times compared to traditional methods, as chatbots can typically provide answers within seconds, leading to increased efficiency.
It's worth noting that the interactions with chatbots generate a wealth of customer data. Companies are leveraging this data to gain insights into trends and preferences. This can help refine marketing efforts and inform future product development. However, it's crucial to acknowledge that these systems do have limitations. While they are excellent at handling basic inquiries, complex or emotional situations still benefit from a human touch. Businesses will need to carefully manage the interplay between human and AI-powered interactions to achieve the optimal balance and maintain customer loyalty. Striking this balance, and addressing challenges that may arise, will likely be a key focus for organizations moving forward.
The Rise of AI-Driven IT Solutions How Businesses Are Adapting in 2024 - CFOs leverage generative AI to drive strategic innovation
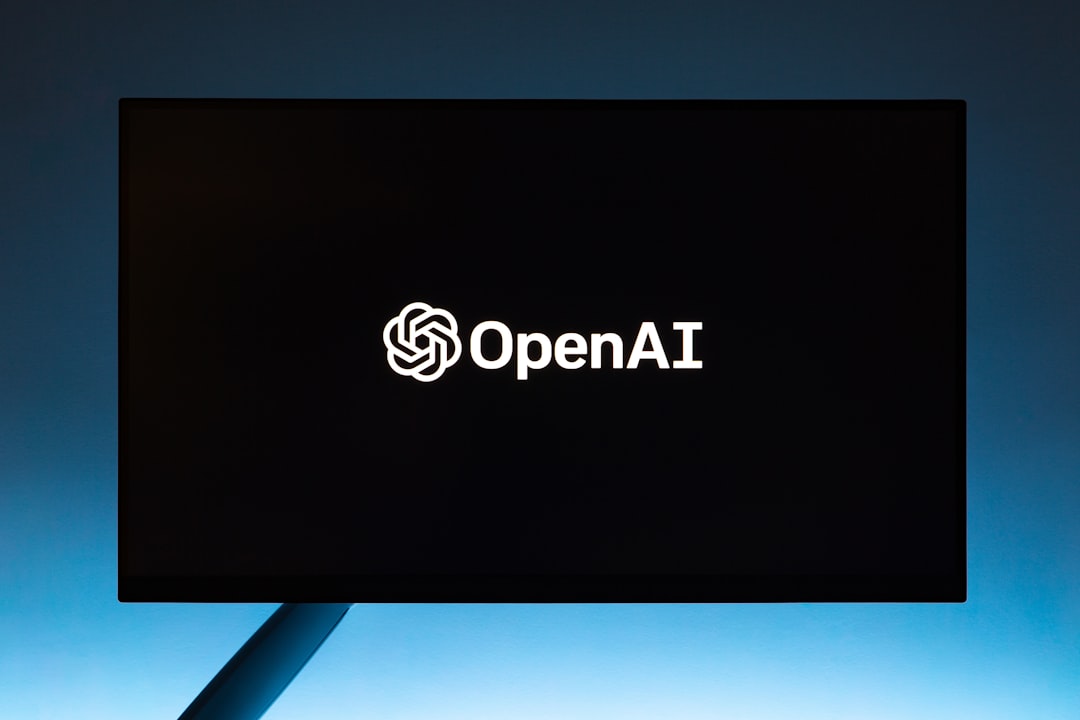
In the rapidly changing landscape of AI-driven IT solutions in 2024, CFOs are increasingly embracing generative AI to drive strategic innovation within their organizations. This powerful technology isn't just about crunching numbers anymore—it's fundamentally altering the role of the CFO, transforming them from a traditional financial guardian to a key architect of company strategy. We see a growing number of CFOs experimenting with and even integrating generative AI into various operational aspects, recognizing its ability to produce useful insights that can provide a competitive edge.
This shift, however, isn't without its obstacles. CFOs face the challenge of understanding and addressing the risks that come with implementing generative AI. They need to ensure their companies can effectively utilize these sophisticated tools, and this often involves establishing appropriate safeguards and processes. In doing so, CFOs are reshaping how financial predictions are made, boosting operational effectiveness, and setting the stage for companies to rapidly respond to the ever-changing demands of the marketplace. The move towards generative AI is forcing CFOs to reassess their role and proactively adapt to this new reality.
Finance leaders, the CFOs, are increasingly using generative AI to change how they do their jobs and make the companies they work for more competitive. A recent study by Deloitte found that nearly half of CFOs are at least experimenting with these tools, with some already incorporating them into their strategies. This suggests that the role of CFO is changing from just watching over the money to becoming a major part of shaping the company's direction in this new AI age.
Generative AI can do more than just look at data; it can also create new content, ideas, and potential solutions. This makes it a really powerful tool for business. It's important that CFOs don't just blindly accept it. They need to be constantly questioning the risks and opportunities that come with these technologies, as they apply them in their company.
There's growing pressure on CFOs to get useful information out of the huge amounts of data they have available. The technology landscape is changing rapidly, and they have to keep up. Generative AI is also creating some new types of risks that CFOs need to figure out and reduce. This underscores their role as a vital part of the risk management process.
As companies move past just trying out generative AI in small projects to using it everywhere, CFOs have to make sure the company uses these advanced technologies in the best way possible. It's very helpful if the way data is organized is standardized, because it allows CFOs to easily see and analyze the data that is scattered throughout different systems. This is really crucial for taking advantage of generative AI.
If you can use generative AI in a smart way, it can improve how accurately you can predict financial things, and that can change the basic business model. Companies can adapt much better to the ever-changing market with this approach. However, while the potential is clear, it's important to consider that as with any new technology, the successful adoption of generative AI requires careful planning and risk management, and may necessitate adapting existing processes and skillsets within the organization. It'll be interesting to see how this unfolds.
The Rise of AI-Driven IT Solutions How Businesses Are Adapting in 2024 - Third-party AI platforms preferred over in-house development
In 2024, as organizations increasingly embrace AI within their IT infrastructure, a trend toward utilizing third-party AI platforms rather than developing in-house solutions has emerged. Many businesses face hurdles in building their own AI systems, encountering lengthy development times and high costs. This can impede their ability to readily incorporate innovative AI features into operations. Turning to established third-party platforms provides a more rapid path to AI implementation and access to sophisticated technologies, without the considerable resource commitment required for internal development. However, this reliance on external platforms introduces its own set of concerns, including managing compliance risks and ensuring responsible AI practices. The growing divide between organizations with strong responsible AI programs and those struggling to implement them emphasizes the need for businesses to actively address the risks and complexities associated with relying on third-party AI solutions to remain competitive.
The trend towards using third-party AI platforms rather than building AI systems in-house is becoming increasingly prominent in 2024. One of the main reasons for this is the speed at which third-party solutions can be implemented. Businesses can get AI up and running in a matter of weeks, as opposed to the months or even years it takes to develop systems from scratch. This rapid deployment is crucial in today's fast-paced market, where companies need to adapt quickly to stay competitive.
Research suggests that companies leveraging third-party AI platforms often see a return on investment (ROI) much faster than those that build their own AI solutions, sometimes as much as 30% faster. This efficiency is especially important for maintaining investor confidence and strong financial performance. Cost also plays a significant role. While in-house AI development can cost millions of dollars, using third-party AI often comes with a more predictable subscription model. This lowers both the initial investment and ongoing expenses, making it a financially attractive option.
Data security is another factor that favors third-party providers. These providers often have more robust security measures in place since they dedicate significant resources to protecting sensitive data. In contrast, many companies may not have the resources or expertise to establish equally robust security for in-house systems. It's also worth noting that many of these platforms rely on a much larger pool of data than what any single company might have. This larger dataset contributes to more refined and effective AI models as the algorithms can learn from a wider variety of data points.
Integration is often simpler with third-party AI solutions. Many platforms are designed with interoperability in mind, allowing businesses to easily integrate AI into existing systems without extensive customization or modifications. Another element that frequently gets overlooked is the current talent gap in AI and machine learning expertise. Using third-party solutions removes the immediate need to hire numerous specialized AI experts, a significant challenge for many companies in a competitive talent market.
Furthermore, compliance with regulations can be a significant obstacle for in-house development. Many established third-party platforms already comply with a variety of regulatory standards, which can help businesses avoid legal complications and expensive redesigns. Third-party AI solutions are typically also updated and optimized regularly, meaning customers always benefit from the newest capabilities. In contrast, in-house solutions can become outdated if regular maintenance and upgrades are not a priority.
Interestingly, there's a growing observation that third-party AI solutions encourage collaboration across departments. Standardized tools help teams share insights and leverage AI across various functions, potentially dismantling siloed approaches that can stifle innovation in organizations with proprietary, in-house systems. It's clear that while the benefits of in-house development can be tempting, the complexities and challenges often outweigh the advantages in 2024.
The Rise of AI-Driven IT Solutions How Businesses Are Adapting in 2024 - Dynamic AI pricing reshapes market strategies and efficiency
Dynamic AI pricing is transforming how businesses approach pricing, using sophisticated algorithms to automatically adjust prices based on current market conditions and customer behavior. This agility has helped companies significantly increase revenue and gain market share compared to more static pricing approaches. Businesses that embrace these AI-driven pricing strategies are gaining an edge over competitors still relying on older, established pricing methods, as the majority of US retailers now utilize and plan to expand their AI-based pricing strategies. However, successfully implementing dynamic pricing requires several key components, including high-quality data, a clear organizational vision, and a thoughtful approach to innovation while ensuring customers maintain trust in the business. This ongoing transition highlights the opportunities and challenges companies face as they integrate AI into their pricing strategies, searching for improved efficiency and stronger market positions.
The integration of artificial intelligence (AI) into pricing strategies is reshaping market dynamics and operational efficiency in 2024, particularly within the retail sector. We're seeing a significant shift towards dynamic pricing, where algorithms adjust prices in real-time based on various factors like demand, competitor pricing, and inventory levels. A substantial portion of larger retailers, about 60%, are already using this approach.
The results have been notable. Studies indicate that dynamic pricing can lead to a noticeable 15% increase in sales compared to static pricing models, suggesting that adapting prices can incentivize quicker purchasing decisions. It's fascinating how AI can leverage machine learning to analyze vast datasets and predict optimal pricing points. These predictive models continually adapt based on past sales data and emerging market trends, which makes them more effective than conventional pricing methods.
One of the most interesting aspects is the ability to respond quickly to changing market conditions, particularly in fast-paced sectors like e-commerce. Companies using dynamic pricing can adapt to consumer demand fluctuations within minutes. This swift adaptability is a powerful competitive advantage, especially in environments where prices frequently change. Furthermore, it's been observed that this approach can increase profit margins, in some cases up to 20%, by optimizing pricing and better understanding what customers are willing to pay.
The application of dynamic pricing extends beyond retail. Travel, hospitality, and entertainment industries are also implementing these strategies, highlighting its adaptability across different sectors. It's interesting to see that it enables businesses to potentially legally segment customers based on detailed analysis, charging different prices to different segments to optimize revenue. Interestingly, many AI dynamic pricing systems also include real-time competitor monitoring features, some claiming to integrate with over 100 competitors, allowing businesses to immediately adjust their prices to maintain a competitive edge.
However, it's crucial to acknowledge that while AI-driven pricing is efficient, it's not without its challenges. One concern is that fluctuating prices on the same product might lead to customer mistrust, especially if the approach lacks transparency. Furthermore, regulatory bodies are beginning to take notice of the potential for unfair pricing practices. As this technology continues to evolve, businesses can expect increased scrutiny of their pricing models and their adherence to consumer protection regulations. It's a delicate balance between maximizing revenue and fostering customer trust, a crucial area for researchers and engineers to study further. The evolution of AI-powered dynamic pricing raises questions about the role of algorithms in setting prices, and the need to balance innovation with consumer fairness in the marketplace. It's clear that this is an area that will require continuous attention from businesses, policymakers, and researchers alike.
More Posts from :