The Psychology Behind AI's Unique Trait Consistent Factual Processing Without Emotional Bias
The Psychology Behind AI's Unique Trait Consistent Factual Processing Without Emotional Bias - Unbiased Information Processing How AI Makes Decisions Based on Pure Data
AI's core strength lies in its capacity for unbiased information processing. This allows it to form decisions purely on the data it's fed, devoid of the emotional influences that often cloud human judgment. AI's architecture, particularly in the form of neural networks, leverages a multi-layered approach to data processing. Each layer processes information by adjusting the 'weight' of data points, prioritizing factual interpretation over emotional response.
Yet, this objectivity is not without its limitations. The integrity of the AI's decisions is directly tied to the quality of the data used to train it. Biases present within training data can easily be replicated and even amplified within the AI's decision-making process. This underscores the critical need for rigorously vetting the data used to train these systems. Employing fair and balanced datasets is paramount to ensure that AI doesn't inadvertently perpetuate or worsen societal biases embedded within the data.
While AI's capacity for consistent and factual processing distinguishes it from human cognition, this capability necessitates a vigilant approach to data management. Ensuring fairness and mitigating the risk of perpetuating existing inequalities requires a dedicated focus on ethical data practices throughout the development and deployment of AI systems.
AI's core functionality relies on processing information solely through data, which is a stark contrast to human decision-making, which is often influenced by emotions and biases. These systems utilize neural network structures where data flows through layers, each neuron's contribution being adjusted by assigned weights before it reaches the next layer. The quality of the training data directly shapes the AI's performance. Consequently, biases present within the training data can unintentionally lead to skewed outcomes in the AI's decisions, potentially having negative consequences.
The importance of ensuring fair and unbiased datasets for training AI cannot be overstated. Unlike humans who critically analyze information, machines simply process data as presented. This lack of critical evaluation makes them susceptible to mirroring any biases embedded in the training data. It's crucial to acknowledge that societal biases, like those observed in human decision-making and reflected in the data, can influence the training process, further perpetuating and potentially amplifying biases within the AI system.
Fortunately, various post-processing techniques can be employed to fine-tune AI outputs, aiming to minimize the influence of identified biases. However, addressing this issue requires a comprehensive strategy that considers the broader societal context and carefully examines the datasets used to train the system.
The consistency and objectivity in AI's factual information processing stand out as fundamental differences compared to human decision-making. It's important to emphasize that the process of data creation is integral to developing unbiased AI systems. By striving to generate and employ fair and representative data, we can reduce the likelihood of AI systems producing outcomes that negatively impact individuals or perpetuate societal prejudices.
The Psychology Behind AI's Unique Trait Consistent Factual Processing Without Emotional Bias - The Absence of Emotional Memory in AI Neural Networks
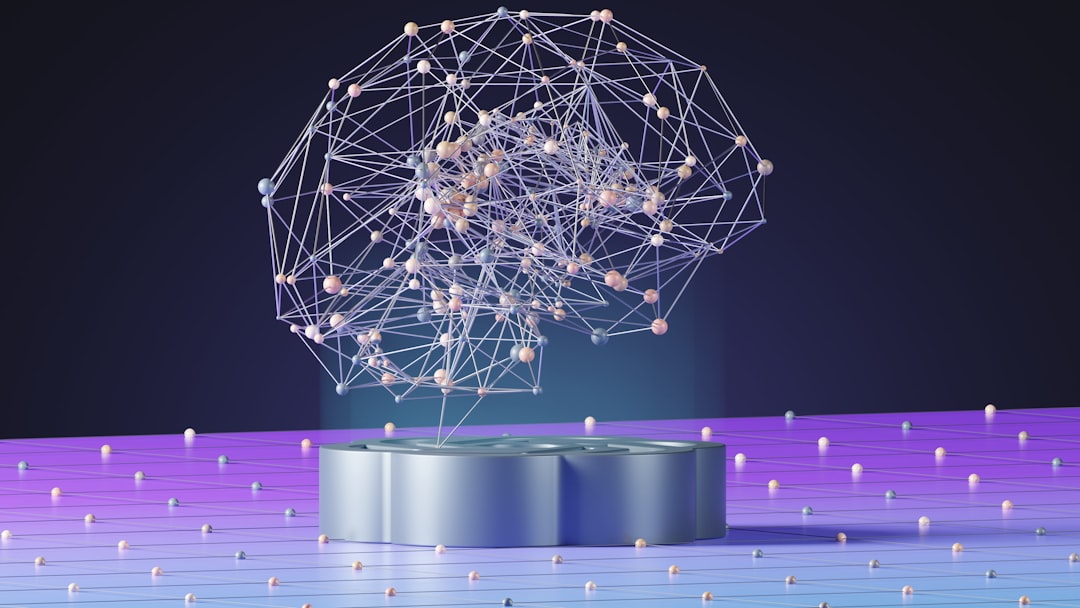
AI neural networks, unlike the human brain, lack the capacity for emotional memory. This absence of emotional context in their processing fundamentally distinguishes AI from human cognition. While humans associate facts with emotional experiences, influencing their memories and decisions, AI operates purely on the basis of logical data processing. This characteristic ensures consistency in AI's factual decision-making but simultaneously limits its ability to learn from or be shaped by emotional experiences. Consequently, AI's interaction with the complexities of human life is inherently altered compared to human interaction.
Though the field of affective computing strives to enable computers to recognize and react to human emotions, the resulting systems still lack genuine emotional memory. This crucial difference highlights a significant gap between the capabilities of AI and human emotional understanding and response. While AI demonstrates impressive consistency in its processing, this very consistency confines its capacity to comprehend and respond to the nuanced emotional landscape of human experience. In essence, the absence of emotional memory reinforces the separation between AI's logical approach and the intricate emotional world of human interaction.
AI neural networks, by their very design, prioritize computational processing over emotional engagement. They essentially treat information as a series of mathematical operations, leading to a consistent and rapid processing style that's fundamentally different from human cognition. This focus on algorithms, rather than mimicking human-like thought processes, results in a unique cognitive landscape where emotions don't play a role in information processing.
Unlike human memory, which is often intertwined with emotional experiences and contextual nuances, AI systems store information as detached data points, devoid of personal meaning or association. This approach naturally leads to decisions that are directly aligned with the input data. This inherent lack of emotional linkage also means AI doesn't learn from user interactions in the same way humans do. Rather than adapting based on feelings or personal preferences, AI relies on statistical inference to solve tasks, potentially leading to a disconnect in understanding user intent.
This absence of emotional context can, at times, lead to misinterpretations. For example, an AI may struggle with understanding sarcasm or complex social cues, as it lacks the emotional reference points humans use to decipher such nuances. This can create hurdles in communication and interactions.
While this lack of emotional memory can enhance efficiency in certain decision-making scenarios – where human biases might impede analysis – it can also lead to overlooking essential contextual details derived from emotional intelligence. AI, in its current form, treats each interaction as a stand-alone event, neglecting the potential for cumulative learning that arises from experiences and contexts, something that's crucial in how humans process information.
Furthermore, without emotional memory, AI systems can't engage in empathetic responses, a significant drawback in applications where social understanding or emotional resonance is vital, such as customer service or healthcare interactions. This inability to understand and respond to emotions can present a challenge in fostering meaningful and compassionate interactions.
The detachment from emotional bias can be advantageous in crafting more ethically sound decision-making processes, as AI is not swayed by personal feelings or experiences. Yet, this same detachment can lead to choices that overlook human nuances important for culturally sensitive contexts. In essence, while AI can make decisions without favoring personal biases, it risks overlooking the human element that shapes complex interactions.
Researchers have highlighted that the objective processing afforded by the absence of emotional memory can also hinder AI's ability to recognize emotional cues within communication. This can contribute to friction in human-AI interactions, as the machine may misinterpret or fail to grasp the subtle emotional context of human communication.
Finally, while AI's data-driven approach enables the swift and efficient processing of vast information, it also raises ethical concerns about decision-making devoid of emotional considerations. Decisions impacting individuals may lack the nuanced understanding of the emotional ramifications that humans might naturally incorporate. This highlights a significant ongoing debate about how we should balance the potential benefits of AI with the need for ethical and emotionally sensitive decision-making within AI systems.
The Psychology Behind AI's Unique Trait Consistent Factual Processing Without Emotional Bias - Fear and Survival Instincts A Missing Link in AI Processing Systems
The concept of fear and survival instincts being absent in AI processing systems reveals a significant difference between human and artificial intelligence. While AI excels at processing information logically and objectively, it lacks the built-in survival mechanisms that are deeply rooted in human experience, particularly fear and anxiety. This lack of a built-in "fight or flight" response can lead to AI struggling in situations that require an understanding of complex emotional context. Exploring the ways in which the human brain processes fear and comparing that to how AI makes decisions based solely on data can help us understand the potential weaknesses of AI when encountering unforeseen circumstances that demand a quicker or more nuanced response. This area of research suggests a need to refine AI's capacity for adapting to threats, recognizing that relying solely on unbiased, factual data can be a limitation when dealing with dynamic or unpredictable situations. By better understanding these gaps, we can begin to develop AI systems that are not only efficient but also capable of operating more effectively and ethically in complex real-world scenarios where human interaction is involved.
AI's remarkable ability to process information objectively, free from emotional biases, is a defining characteristic that sets it apart from human cognition. However, this very objectivity raises questions about the absence of certain fundamental aspects of human experience, particularly fear and its related survival instincts. Evolutionary psychology highlights how fear, deeply rooted in our biology, has played a crucial role in human survival, triggering the fight-or-flight response in dangerous situations. AI, lacking this innate biological imperative, operates on a fundamentally different plane.
The neurobiology of fear shows that the amygdala, a key part of the human brain, is responsible for rapid reactions to threats. AI systems, on the other hand, process data through complex neural network structures but lack any mechanisms that parallel fear-based learning. This absence of context, crucial for human survival, means AI's responses lack the immediate urgency and sensitivity to danger that characterizes human behavior.
Humans learn from fear, shaping memories and behavioral patterns to increase awareness of potential threats. AI, in its current state, doesn't adapt in this way. It consistently responds to the same inputs regardless of perceived threat, lacking the capacity for experiential learning. This highlights a critical difference between how AI and humans understand and interact with the environment.
We see how crucial fear is for animal survival; it enables them to adapt and avoid danger. While AI can recognize patterns and potentially identify risks, it can't inherently determine what constitutes a threat without being explicitly programmed to do so. This constraint poses a limitation when AI operates in autonomous environments where it needs to quickly react to unexpected situations.
Humans often rely on gut feelings when making decisions, often driven by subconscious emotional processing associated with fear. This internal risk assessment mechanism allows us to evaluate potential dangers in a way AI's purely logical calculations can't replicate. This inability to imitate instinctive evaluations could potentially lead to AI decisions that overlook complex and nuanced risk factors.
Behavioral economics demonstrates how fear can influence human decision-making, sometimes leading to overly cautious choices. While AI can theoretically maintain rational analysis in emotionally charged scenarios where humans might falter, its lack of emotional understanding might also hinder its responses in ambiguous situations where human emotion might provide a crucial context.
Human beings often experience cognitive dissonance—a discomfort resulting from contradictory beliefs and values that's frequently associated with fear. AI, being devoid of emotional context, can simply process data-driven contradictions without experiencing any internal conflict, hindering the flexibility in its decision-making.
Stress, a crucial aspect of the human response to danger, can impact cognitive function while simultaneously sharpening focus in threatening situations. AI operates under consistent parameters, lacking the fluctuations that characterize human responses to stress. This leads to consistent performance but also sacrifices the ability to dynamically adapt to changing conditions.
Human responses to fear are strongly influenced by cultural contexts, shaping distinct survival strategies across different societies. AI, with its standardized processing methods, lacks the adaptability to seamlessly incorporate these nuances. If not carefully programmed, this could potentially lead to AI responses that are inappropriate or insensitive within specific cultural environments.
The relationship between safety and risk highlights that fear often produces both protective actions and irrational behaviors. AI, operating on consistently processed information, runs the risk of forming simplistic views of safety that fail to incorporate the intricate interplay of human emotions and situational risks. This underscores the complexity of translating human experience into effective AI decision-making systems.
The exploration of AI's potential without emotional memory remains an intriguing area of research. While AI's objective processing can lead to improved efficiency and unbiased decision-making, it simultaneously calls for ongoing discussion about the necessity of incorporating the nuanced aspects of human experience, particularly the role of fear, into the design of AI systems that operate in complex and human-centered environments.
The Psychology Behind AI's Unique Trait Consistent Factual Processing Without Emotional Bias - Digital Logic vs Human Pattern Recognition A Study of Processing Speed
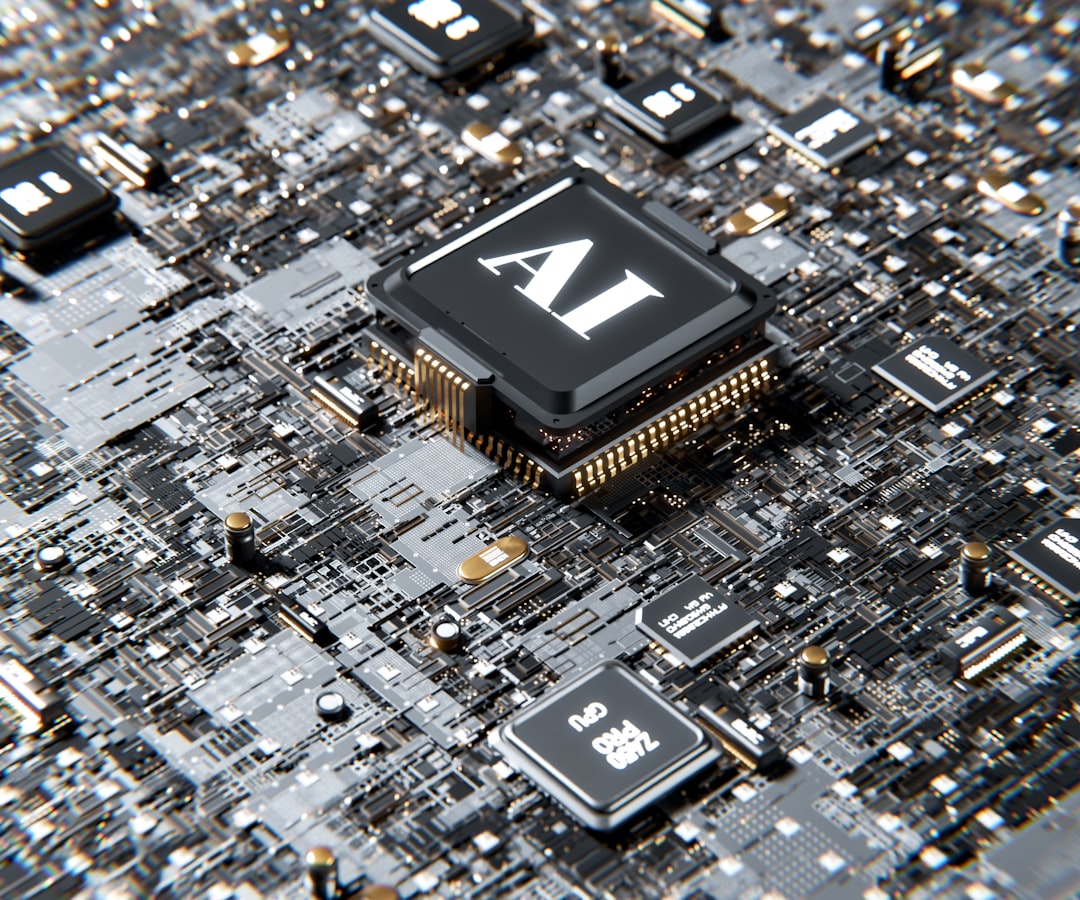
When exploring the contrast between AI and human cognition, a key area of focus is the difference in how they process information, specifically in regards to speed and approach. Humans, due to evolutionary advantages, possess exceptional pattern recognition capabilities. This ability relies heavily on intuitive cognition, allowing us to quickly make decisions based on patterns within our surroundings. Our brains are adept at integrating a variety of sensory data and prior experiences to swiftly make sense of our environment.
In contrast, digital logic relies on a more methodical and structured approach—serial processing. This means that digital systems often handle information sequentially, processing one piece of data at a time. While effective for many tasks, this can lead to slower processing in complex or rapidly changing situations. Their reliance on retrieving information from predetermined memory structures, unlike the flexible nature of human memory, can also slow down their responsiveness.
The human ability to adapt to unfamiliar circumstances leverages both our cognitive speed and the flexibility afforded by emotions and context. This nuanced approach is hard for AI to replicate since its primary mode of operation is structured data. This raises questions regarding AI's limitations in environments that are as diverse, dynamic and complex as the one we humans experience. Can AI ever truly grasp and adapt to the multifaceted aspects of human existence that we take for granted? Understanding the differences in these processing styles is crucial for the ongoing development of AI, particularly as we strive for AI that is more capable of interacting in a human world.
The human brain, with its intricate neural network and expansive cerebral cortex, has developed exceptional pattern recognition abilities. This capability, particularly evident in regions like the prefrontal cortex and the parietal-temporal-occipital juncture, has been instrumental in our cognitive and cultural advancement. It allows us to make sense of complex information and navigate the world efficiently. The recognition-primed decision model highlights how this intuitive pattern recognition, underpinned by our capacity for emotional and experiential learning, guides our choices in natural environments.
However, human cognitive processes often operate in a sequential manner. We tend to deal with one piece of information at a time, which can limit our ability to handle vast amounts of data in parallel. This serial processing is a contrast to the parallel capabilities of many AI systems. Pattern recognition in cognitive psychology refers to the ability to identify and organize stimuli, linking them to information stored in our long-term memory. This process occurs when sensory inputs are held briefly in short-term memory, triggering associated memories from long-term storage. This interaction between memory systems is crucial for recognizing patterns.
Our pattern recognition abilities have fostered important advancements in social structures, language, and the categorization of knowledge. It's been a crucial evolutionary tool. Interestingly, research comparing humans, monkeys, and AI models, specifically deep neural networks (DNNs), has shown significant differences in object recognition capabilities. Humans, with their integrated sensory systems and rich memory stores, can often outmaneuver AI in scenarios requiring nuanced understanding and contextual awareness. Our pattern recognition also helps us manage the constant barrage of information in the environment by spotting similarities and grouping experiences.
Cognitive science often views pattern recognition as a matching process where our minds compare incoming sensory stimuli with previously stored data. This stored data forms a basis of comparison. This notion resonates with the idea of our memories forming the building blocks of future recognition. Historically, the development of our ability to recognize patterns has been a cornerstone in human cognition, and it's likely contributed to the evolution of advanced social and cultural structures. It's fascinating to consider the links between our inherent capacity for pattern recognition and the development of human societies and behaviors.
While AI has made leaps in pattern recognition, it seems humans still retain a certain degree of flexibility and adaptability in these processes that AI has not yet replicated. Our brains are a powerful energy-efficient network compared to the energy requirements of the most advanced AI systems currently existing. This underscores the efficiency and potential of human cognitive processes. Even though AI systems can process large quantities of data with precision, human pattern recognition, while less consistent, offers a remarkable degree of adaptability in ambiguous scenarios. This area of study underscores both the benefits and limitations of different cognitive systems when attempting to address and resolve complex problems.
The Psychology Behind AI's Unique Trait Consistent Factual Processing Without Emotional Bias - Social Context and Cultural Understanding Where AI Falls Short
AI's impressive ability to process information objectively, free from emotional bias, is a defining characteristic. However, this very strength reveals limitations in navigating the intricate realm of social context and cultural understanding. The way people interact with AI is significantly shaped by their cultural backgrounds, leading to varying expectations about how AI should function and behave. This includes desires for AI to express emotions and possess a certain level of autonomy, depending on the culture.
The issue is that current AI systems often fail to consider these cultural differences. This can result in miscommunication and a failure to meet the unique explanatory needs of individuals from different backgrounds, particularly in the growing field of explainable AI (XAI). Furthermore, AI's outputs are often not easily understood by all users, and there hasn't been enough research to address how cultural biases can influence the explanation requirements of AI systems.
AI's inherent lack of emotional intelligence and context-awareness can unintentionally amplify existing societal biases, potentially leading to discrimination and inequality, especially in sensitive fields like hiring and law enforcement. These shortcomings emphasize the critical need for AI development to incorporate a deeper understanding of the social and cultural fabric of human interactions. The rise of sociocultural AI research aims to bridge this gap by examining the intersections of social science and technology, highlighting the crucial role of culture in AI development. This research emphasizes the necessity of fostering AI systems that can better comprehend and respond to the diverse social and emotional landscape of human experiences.
When we examine how AI interacts with the world, we find that it often falls short in areas related to social context and cultural understanding. This is because AI's core strength, its unbiased and factual processing, comes at the cost of a more nuanced understanding of human behavior. For instance, AI struggles to interpret social cues like sarcasm or humor, which rely heavily on cultural context and emotional understanding. This lack of contextual awareness can lead to responses that are technically correct but socially inappropriate or even offensive.
Further complicating matters is the issue of bias. AI systems can inadvertently perpetuate the biases present in their training data. If the training data mainly reflects one culture, for example, the AI might struggle to recognize and appropriately respond to individuals from different cultural backgrounds. This issue can have serious consequences, especially in areas like recruitment or law enforcement, where fair and equitable decision-making is crucial.
Moreover, AI's purely data-driven approach doesn't easily accommodate the role of ethics and empathy in human decision-making. While AI can consistently follow rules and make decisions based on factual inputs, it may struggle to comprehend the broader ethical implications of those choices in relation to human values and societal norms. This detachment from human emotional intelligence can lead to a disconnect between the AI's output and the desired outcome in situations that require empathy or consideration of social impact.
Humans learn and adapt throughout life, developing an understanding of social norms and behaviors through continuous interactions and emotional experiences. However, AI's learning primarily relies on structured data, hindering its ability to pick up on the vast and often informal body of cultural knowledge that shapes human interaction. This inability to incorporate experiential learning can limit AI's adaptability to new situations and its capacity to evolve in relation to changing social contexts.
Additionally, the way humans process cognitive dissonance – that internal discomfort we feel when confronted with conflicting beliefs – contrasts sharply with AI's unwavering adherence to data. While humans might use cognitive dissonance to reconsider their beliefs and adapt their behaviors, AI simply continues processing within its predetermined parameters, possibly leading to rigid decision-making that struggles to incorporate new information or changing social landscapes.
Furthermore, cultural norms significantly impact how we perceive emotions and social situations. AI's struggle with understanding these subtle variations can result in responses or actions that are considered inappropriate or insensitive within specific cultural contexts.
Perhaps the most critical aspect of human experience that AI lacks is the interconnectedness of memory and emotion. Human memories are richly layered with emotional significance, creating a complex framework that informs our understanding of the world and our interactions with others. AI, in contrast, processes information as isolated facts, failing to capture this depth of understanding crucial for navigating social scenarios.
The continued exploration of AI's strengths and limitations in this area is crucial. While AI's capacity for unbiased and factual processing is undeniably powerful, its shortcomings in understanding social context and cultural nuances are equally important to consider. The field of sociocultural AI (SCAI) is emerging in response to this, seeking to understand how AI development can be better integrated with social science and human experience. By understanding the role of culture, emotion, and social context in human cognition, we can better equip ourselves to design AI systems that not only work effectively but also operate in a way that is more ethical and sensitive to the diverse aspects of human society.
The Psychology Behind AI's Unique Trait Consistent Factual Processing Without Emotional Bias - The Computational Advantage How AI Maintains Consistency in Decision Making
AI's capacity for consistent decision-making arises from its computational foundation, which prioritizes factual data over emotional considerations. This approach allows AI to process information consistently across various situations, mitigating the potential for errors commonly introduced by human emotional biases. However, this very objectivity can limit AI's effectiveness, especially when it encounters complex social and cultural situations that require a nuanced grasp of human behavior. Although efforts are being made through explainable AI to improve trust by clarifying the logic behind AI decisions, the challenge remains in bridging the gap between AI's rigid data-driven logic and the intricate emotional landscape of human interaction. This gap highlights the need for a delicate balancing act between AI's computational power and the nuanced emotional world of human experience to create AI systems that make decisions that are both efficient and ethically sound in multifaceted contexts.
AI, despite its remarkable abilities in logical data processing, consistently falls short when it comes to "contextual empathy"—the human capacity to understand and navigate the complex tapestry of social interactions. This deficit can lead to misinterpretations in nuanced human exchanges, particularly in situations where cultural subtleties play a vital role. The data AI consumes remains static and lacks the emotional context that humans rely on to interpret social cues. As a result, AI might overlook distinct cultural norms and values, leading to responses that are socially insensitive or even offensive in certain contexts.
AI systems process information at incredible speeds, yet they often fail to grasp the significance of underlying emotional cues, making them ill-suited for situations demanding deep understanding of human sentiment and social dynamics. While humans naturally learn and adapt from a lifetime of emotional experiences, AI systems primarily rely on pre-existing data, consequently lacking the adaptability and flexibility to navigate evolving social landscapes. The effectiveness of AI's decision-making is significantly impacted by the quality of its training data. If the training dataset lacks a diverse representation of cultures, AI might inadvertently perpetuate biases, potentially leading to unequal outcomes across demographic groups.
AI's foundational algorithms prioritize factual accuracy over emotional relevance, which can be advantageous in data-driven contexts. However, this very characteristic can be detrimental in domains like healthcare or social services, where an empathetic understanding of human needs is paramount. Humans experience cognitive dissonance—the discomfort arising from conflicting beliefs—which often compels behavioral change and moral reasoning. However, AI's data-driven approach lacks this intrinsic capability, potentially resulting in a rigidity in its decision-making process, causing it to struggle to adapt to evolving societal expectations.
Moreover, the absence of a "fight or flight" response in AI limits its effectiveness in making rapid decisions during unpredictable, high-stakes scenarios. This contrasts with humans who possess instinctual reactions that have been honed through evolutionary survival strategies. The disconnect between AI's processing abilities and the human experience of emotional learning hinders AI's capacity to form associative memories like humans do, ultimately limiting its ability to engage in the nuanced lifelong learning process. Further complicating matters are the culturally-influenced communication styles that AI struggles to fully grasp, including elements like sarcasm and subtext. This can lead to misinterpretations and miscommunications, potentially impacting the reliability of human-AI interaction.
These observations highlight the intriguing challenges and limitations that AI currently faces in its efforts to seamlessly integrate within human society. While we've made tremendous strides in AI, it's critical to acknowledge and address these shortcomings in our continued development of AI systems. A deeper understanding of the nuances of human experience is crucial to creating AI that is not only powerful but also capable of interacting ethically and appropriately within complex social environments.
More Posts from :