The Evolution of Internal Knowledge Bases From Data Silos to AI-Powered Collaboration Hubs
The Evolution of Internal Knowledge Bases From Data Silos to AI-Powered Collaboration Hubs - From Fragmented Data to Unified Knowledge Ecosystems
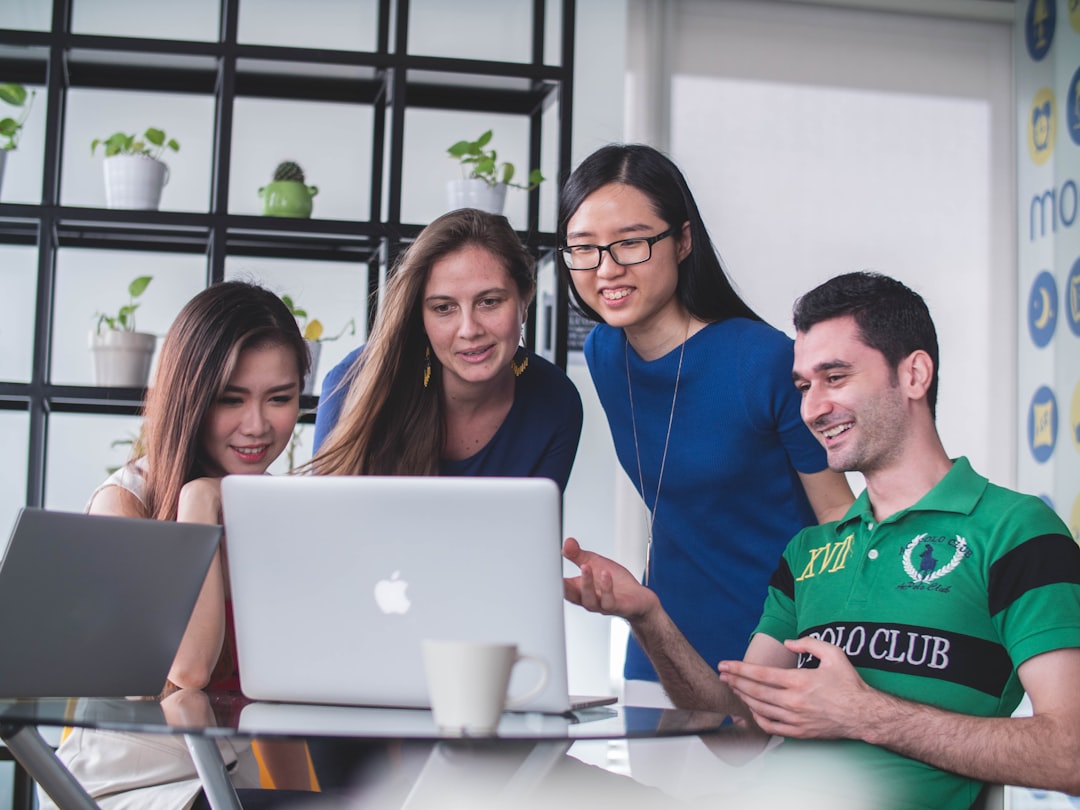
The journey from disparate data pools to cohesive knowledge ecosystems represents a fundamental change in how organizations handle and utilize information. This evolution highlights the need to break down the barriers created by isolated data silos, allowing for enhanced collaboration, improved communication flow, and streamlined workflows across departments. The move towards unified platforms facilitates the creation of central knowledge repositories, improving user experiences and enabling more efficient knowledge dissemination.
This transition is propelled by the intersection of artificial intelligence advancements and increased data interoperability. These combined forces enable organizations to develop a broader understanding of their operations, market trends, and customer interactions. Organizations that strive to enhance innovation and achieve greater operational efficiency will find that building unified knowledge ecosystems is no longer optional, but essential for future success. The integration process can be challenging, requiring careful planning, and it's worth recognizing that simply combining data does not guarantee a seamless ecosystem; careful design and consistent governance are critical to realizing the benefits.
The journey from scattered data repositories to unified knowledge ecosystems signifies a profound shift in how organizations handle information. We've seen how traditional data silos, while perhaps understandable in the past, create serious roadblocks to extracting real value. It's not uncommon for organizations to lose a large percentage of the potential insights embedded within their data due to this fragmented nature. The inability to easily connect data across departments hinders comprehensive analysis and obstructs collaborative efforts.
The impact of these barriers is felt across the organization. It's become clear that many employees struggle to find the information they need to do their work effectively because of disjointed systems. This frustration inevitably leads to reduced productivity and hampers an organization's ability to respond quickly to both market changes and customer demands.
One of the most intriguing developments in this space is the growing interest in and adoption of platforms designed to act as central knowledge hubs. These platforms, like SharePoint Online, show promise in bringing together disparate systems and data sources into a coherent whole. This centralization, however, is not just about consolidation; it's about establishing a foundation that promotes active knowledge sharing. We are only starting to understand the full impact that unified data ecosystems have on the speed of decision-making and responsiveness to change.
However, the challenge of building a genuinely unified knowledge ecosystem is multifaceted. It involves not just the technical integration of data but also the careful consideration of human factors. The traditional mindset of compartmentalized data management needs to evolve into one that embraces broader interconnectedness and collaboration. The next iteration of knowledge management is heavily influenced by AI and advanced data interoperability techniques, represented by tools like Unigraph. We are now in a position where we can imagine creating knowledge bases that learn and adapt over time, becoming even more effective at anticipating user needs.
While the transition to integrated knowledge ecosystems might seem daunting, the potential rewards are significant. Organizations that are successful in breaking down information silos are often able to foster more innovative and collaborative cultures. The goal is to build environments where knowledge is not just stored but shared, used, and constantly refined to drive even better outcomes.
The Evolution of Internal Knowledge Bases From Data Silos to AI-Powered Collaboration Hubs - The Rise of AI-Driven Information Retrieval and Analysis
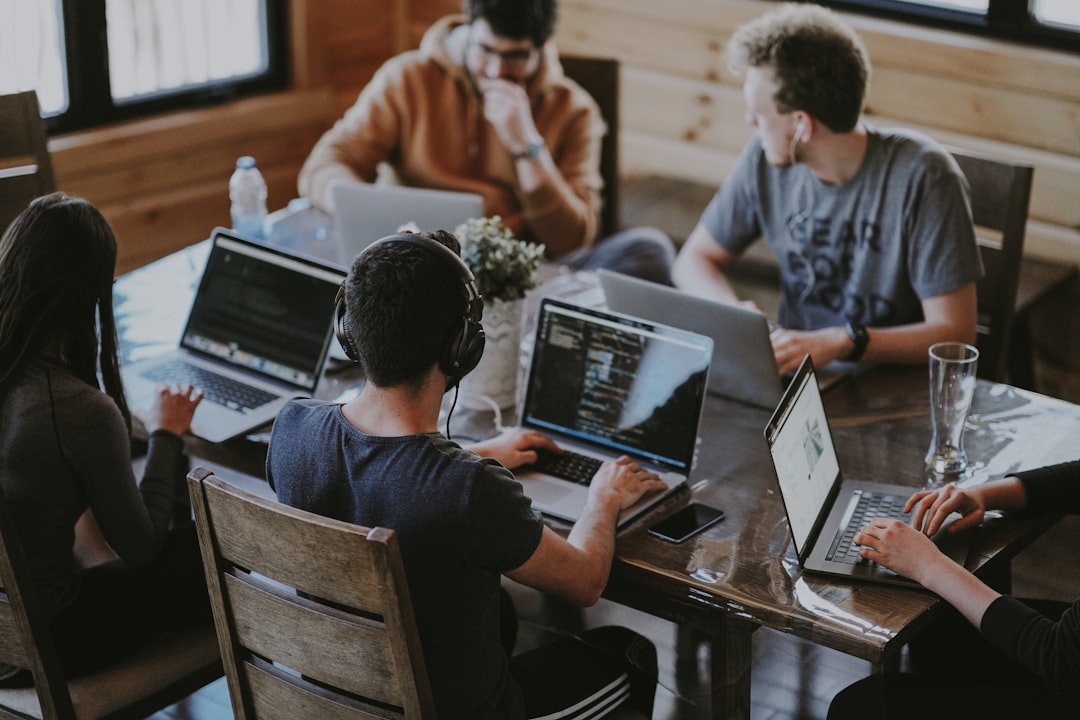
The increasing adoption of AI in information retrieval and analysis signifies a major change in how knowledge is accessed and used within organizations. AI's ability to automate tasks and improve user services is fundamentally altering the role of information professionals. They are transitioning from simply mediating information to directly enabling access to knowledge through AI-powered tools. While AI holds incredible promise in the areas of indexing, analyzing, and retrieving large amounts of data, there's a noticeable lack of preparation and training among many information professionals to fully harness these emerging tools. The shift towards AI-driven knowledge hubs within organizations necessitates the representation of information in a format conducive to machine learning and extensive data analysis. This transformation, however, isn't without hurdles. It requires a fundamental shift in organizational culture, a move towards embracing broader collaboration and interconnectedness. The ultimate aim is to build more agile and responsive knowledge ecosystems that fuel innovation and knowledge creation.
The field of information retrieval is undergoing a profound transformation driven by the increasing capabilities of artificial intelligence. We're seeing a noticeable shift in how people find and utilize information, with AI-powered systems promising to drastically reduce the time it takes to uncover relevant insights. Studies suggest that AI can cut search times by up to 70%, allowing organizations to access crucial data much faster than with traditional methods. This speed is crucial in today's fast-paced environments.
Interestingly, the benefits of AI extend beyond simply analyzing numbers. Qualitative information, like text and conversations, is also being effectively processed and synthesized by AI, enabling us to extract more nuanced understandings from a wider array of data sources. This capability opens doors to richer insights and deeper analysis than was possible before.
Furthermore, AI algorithms are now allowing for more sophisticated searches. Instead of just matching keywords, these systems are beginning to understand the context and intent behind a search query. This context-aware approach significantly enhances the relevance and usefulness of the search results, making the entire information retrieval process more efficient and insightful.
As AI continues to power these systems, we're discovering hidden connections within our data that were previously obscured by the fragmented nature of traditional data silos. By analyzing interactions within knowledge bases, AI can reveal unexpected relationships between seemingly disparate data points, potentially unlocking valuable new understandings about the organization and its environment.
The importance of user experience (UX) in designing these AI-driven systems is becoming more apparent. It's no longer enough for these systems to be fast and efficient; they also need to be intuitive and easy to use. AI-powered systems are being carefully designed to cater to human preferences and behaviors, striving to create a smooth and effortless interaction with knowledge.
A significant trend is the emergence of knowledge bases that are dynamic and self-updating. Many organizations are integrating AI to prioritize real-time updates, ensuring that knowledge bases continually reflect the latest information. This dynamic approach ensures that employees always have access to the most relevant and current data.
There's a growing body of evidence that organizations using AI-enhanced knowledge retrieval systems see improvements in employee satisfaction and engagement. It seems that when employees spend less time searching for information and more time actually doing their work, they tend to be happier and more productive. It makes sense—spending hours hunting for a document is not only frustrating but also unproductive.
While the integration of AI into knowledge bases brings exciting possibilities, it's important to acknowledge the ethical and governance implications. Issues around data privacy and the potential for algorithmic biases need to be addressed carefully. There's a risk of flawed AI systems creating insights based on skewed data, resulting in inaccurate or unfair outcomes.
The financial implications of adopting AI-driven information retrieval systems are substantial. Organizations are reporting impressive returns on investment (ROI), often exceeding 300% within a few years. This positive financial impact is primarily due to reduced operational costs and faster decision-making enabled by quicker access to critical information.
It's exciting to think about the future potential of these evolving systems. With technologies like natural language processing and machine learning continuously developing, we're moving towards intelligent knowledge bases that can anticipate user needs and proactively provide information and solutions. The shift is not only about finding information but also about fostering a culture where knowledge is actively shared and refined. This shift could potentially redefine the traditional role of the employee from being simply a consumer of information to being a contributor and shaper of organizational knowledge.
The Evolution of Internal Knowledge Bases From Data Silos to AI-Powered Collaboration Hubs - Breaking Down Departmental Barriers Through Centralized Knowledge Hubs
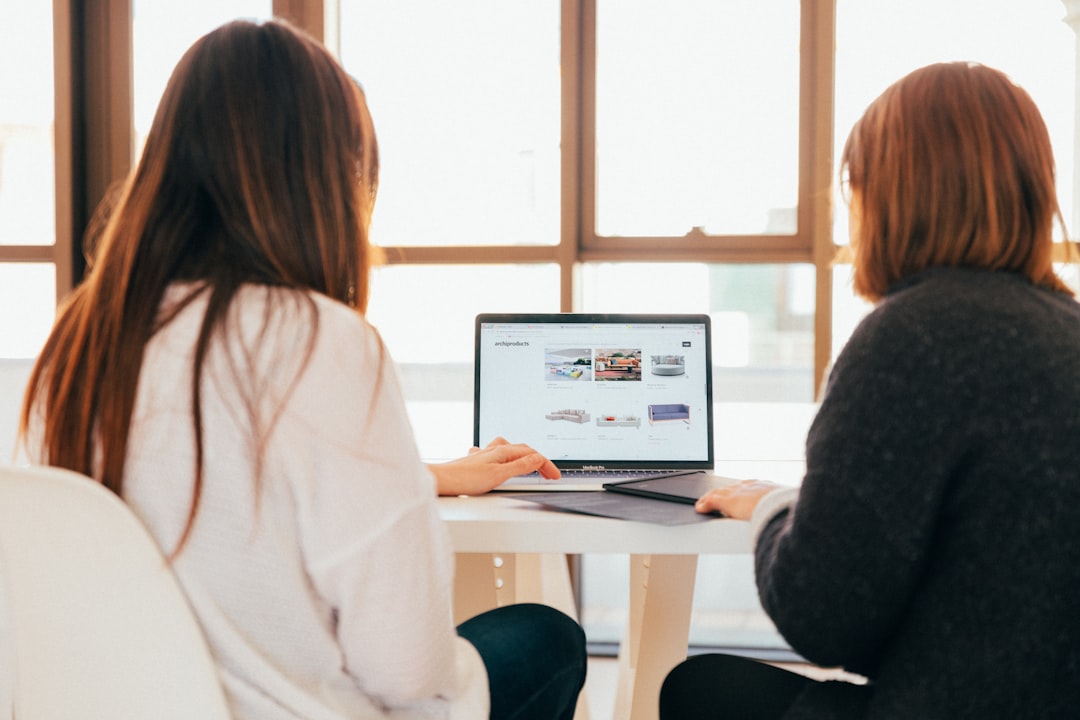
Centralized knowledge hubs are becoming increasingly vital for organizations striving to dismantle the barriers that separate departments. These hubs act as a bridge, allowing for the smooth flow of information between different teams and overcoming the negative consequences of data silos. Data silos hinder communication, slow down processes, and limit overall effectiveness. By prioritizing cross-departmental knowledge sharing and cultivating a culture that values openness, centralized hubs empower employees with easy access to crucial information. This, in turn, bolsters an organization's ability to adapt and respond quickly to changes. However, achieving this collaborative environment requires a comprehensive shift in both how information is managed technologically and how it's perceived culturally. It calls for a collective approach to knowledge, shifting away from the traditional, siloed perspective.
In our increasingly dynamic business landscapes, organizations are realizing that proactively retaining and sharing knowledge is crucial for staying competitive. One of the biggest hurdles is the existence of departmental "silos"—isolated data pools that prevent information from flowing freely. These silos, born from traditional ways of organizing information, often hinder collaboration and efficient workflows.
If we want to break down these barriers and encourage true collaboration, we need to change how we manage information. This means implementing strategies that foster a more integrated and interconnected flow of knowledge. Digital transformation initiatives play a key role here, encouraging the exchange of information across different teams and departments. Tools designed to facilitate cross-departmental collaboration, like platforms dedicated to knowledge sharing and regular inter-team meetings, can help establish a more connected environment.
A centralized knowledge hub, easily accessible to everyone within an organization, offers significant benefits. It serves as a repository for vital information, promoting efficiency and boosting overall communication. Creating a culture of shared knowledge requires active participation, and recognizing and rewarding those who contribute to this central hub can encourage more people to contribute.
The negative impacts of data silos are becoming increasingly clear. They slow down processes, limit effective communication between departments, and ultimately reduce the organization's overall efficiency. Maintaining robust internal communication channels is vital to ensuring that knowledge moves smoothly and readily throughout the organization.
Cultivating an environment of openness and transparency is key to dismantling these silos. Encouraging open communication between teams, facilitating cross-functional collaborations, and promoting a shared sense of purpose across departments are important practices in fostering a more collaborative and informed workforce.
It's clear that the traditional way of managing information isn't sufficient for organizations that seek agility and innovation. A shift towards centralized knowledge hubs is necessary, but it requires careful consideration. Building these hubs is not simply about consolidating data; it's about fostering a collaborative culture where knowledge isn't just stored but actively used and refined. This transition requires a careful balancing act between technical integration and the human element. While the prospect of building fully integrated knowledge ecosystems might seem daunting, the rewards – a more collaborative and innovative workforce – are significant. We're still in the early stages of understanding how best to design these systems to maximize their potential, but the potential for improvement in efficiency and collaboration is compelling.
The Evolution of Internal Knowledge Bases From Data Silos to AI-Powered Collaboration Hubs - Transforming Employee Onboarding and Training with Smart Knowledge Bases
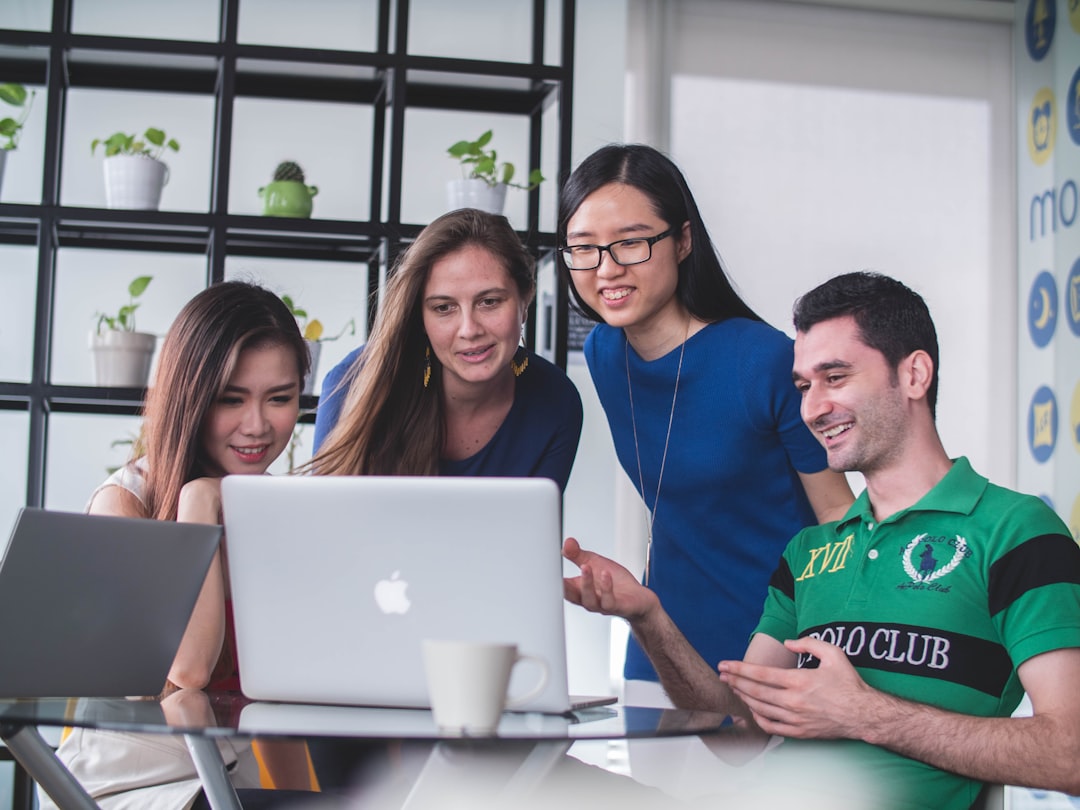
Smart knowledge bases are reshaping how employees are onboarded and trained by introducing AI-powered personalization and automation. This shift allows organizations to tailor learning experiences to individual employee needs, making onboarding more engaging and relevant right from the start. AI can streamline HR processes, automating tasks like scheduling training, monitoring progress, and gathering feedback. This automation frees up HR professionals to focus on more strategic aspects of talent development. The ability to gather and analyze data using AI provides valuable insights that improve decision-making in training and onboarding programs. Smart knowledge bases also help keep training materials current, ensuring that new employees are equipped with the latest information. Despite the benefits, organizations need to be mindful of the potential pitfalls. If not implemented thoughtfully, these AI-powered solutions could lead to onboarding programs that are less effective, potentially resulting in higher employee turnover and reduced retention.
The integration of intelligent knowledge bases is rapidly changing how organizations approach employee onboarding and training. We're seeing the potential for these systems to significantly reduce the time it takes for new hires to become productive members of the team. A well-structured knowledge base, readily accessible to all, offers a centralized source for essential training materials and internal procedures, facilitating a smoother integration process. This, in turn, has the potential to boost employee retention, with studies showing a possible 25% increase in retention rates when onboarding is well-managed through these kinds of systems. It seems intuitive that if new employees have readily available resources to learn and integrate they'll feel more supported and be less likely to leave shortly after joining.
These intelligent systems are not static. They adapt to how people are using them. The training content itself can evolve based on the feedback gathered from employees, automatically adjusting to remain relevant and useful. This dynamic nature of the knowledge base can save considerable time and effort as opposed to constantly updating static materials manually. In the past, finding the right information consumed a significant portion of employees' training time—estimates suggest between 40-60%. AI-powered search features and context-aware suggestions within smart knowledge bases can significantly reduce the time spent searching, allowing employees to spend more time focusing on tasks and learning.
Another interesting trend is the positive impact on employee engagement that we're seeing with centralized knowledge hubs. When workers can easily access and share information, it often fosters a sense of connection and empowerment, which can lead to improvements in engagement. Reports indicate that organizations using these tools see a potential increase in engagement of around 37%, which could have ripple effects across the organization. These hubs can also break down departmental barriers, traditionally known as "silos," by promoting collaboration across different teams. This increased collaboration can spark innovative solutions that might not have surfaced otherwise.
Beyond engagement and innovation, the use of these systems also promotes continuous feedback loops where employees can actively assess the training programs and provide direct input. This ongoing feedback cycle is essential to continuously improve the quality and relevance of the training materials, ensuring they effectively cater to the changing needs of the workforce. It's also a useful tool for compliance training. Using a central hub to track employee completion of mandatory training programs not only improves compliance but can also significantly mitigate the risks associated with non-compliance, potentially reducing them by 50%.
Of course, there are some challenges and ethical considerations with using such systems. Concerns about data privacy and the potential for biases within algorithms need to be thoughtfully considered. However, the overall picture is positive. These AI-powered solutions are showing a clear path to substantial cost savings, with estimates of 20% reductions in onboarding costs. This cost reduction arises from the automation of various administrative tasks associated with training, which in turn frees up valuable resources for more strategic HR activities. As AI and related technologies continue to advance, we can expect these smart knowledge bases to become even more sophisticated, capable of anticipating user needs and proactively delivering information and solutions. It's a transformation that could profoundly reshape the way knowledge is created and shared within organizations, with employees shifting from simply being consumers of knowledge to becoming active participants in shaping and refining the organization's collective understanding.
The Evolution of Internal Knowledge Bases From Data Silos to AI-Powered Collaboration Hubs - Leveraging Machine Learning to Identify and Fill Knowledge Gaps
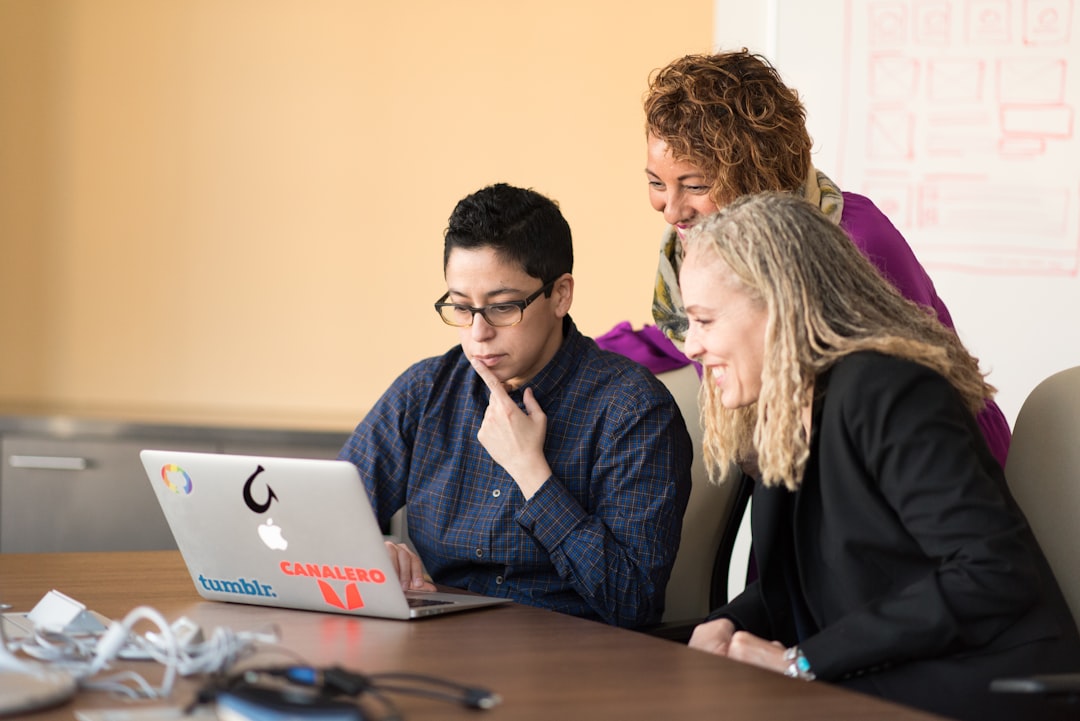
The use of machine learning to uncover and address knowledge gaps within organizations is a significant development in knowledge management. AI tools can analyze large datasets to detect recurring patterns and trends, prompting the timely update of knowledge repositories. This ongoing analysis ensures that knowledge bases remain relevant and reflect the current needs of the organization. This not only streamlines operations but also creates an environment that encourages a collaborative and continuous learning approach to knowledge management. However, successfully integrating AI into knowledge management requires a thorough approach to data handling and a shift in how the organization views knowledge sharing. Ultimately, organizations can move beyond simply storing data towards a more dynamic and proactive knowledge-driven approach by using machine learning to understand and act upon their collective understanding.
Exploring the use of machine learning within internal knowledge bases reveals some interesting ways to identify and bridge knowledge gaps. It's not just about storing data anymore—it's about making that data work for us.
For instance, machine learning algorithms can anticipate potential knowledge gaps before they cause problems. They do this by tracking how people interact with the knowledge base and noticing which topics are searched for often without successful results. This predictive ability is quite powerful—it's like having a built-in early warning system for knowledge gaps.
Another intriguing aspect is how these systems can move beyond simple keyword matching. They're developing a contextual understanding, using natural language processing to grasp the intent behind a user's query. As a result, they can suggest relevant content that users may not have even considered. It's like having a knowledgeable guide within the knowledge base, proactively suggesting helpful information.
Furthermore, these systems are becoming increasingly adaptive. They're designed to learn from user feedback and interactions, constantly refining the knowledge base in real-time. This means that the most up-to-date and relevant information is always at the forefront, which is crucial in today's fast-changing environments.
One of the more unexpected uses of machine learning in this context is its ability to analyze the interconnectedness of information. By mapping how different pieces of knowledge within the base relate to each other, we can uncover hidden relationships and insights that might have otherwise been missed. It's akin to discovering the underlying structure of the knowledge itself.
Additionally, machine learning can excel at spotting anomalies in how people are using the knowledge base. This ability to identify outliers can highlight where knowledge gaps are most prevalent. It's like having a magnifying glass to pinpoint specific areas that need attention, whether it's a topic that needs better documentation or an area with conflicting information.
The personalization aspect is also becoming increasingly important. Machine learning algorithms can now tailor content recommendations to individual users based on their roles and previous interactions. This targeted approach helps users find what they need faster, leading to a more efficient and productive experience.
Interestingly, machine learning can also be used to enhance collaboration across teams. By analyzing how teams interact with the knowledge base, it's possible to identify knowledge gaps that are impacting multiple departments. This can then lead to better-targeted initiatives to facilitate knowledge sharing between teams.
Furthermore, algorithms can assess the comprehensiveness of documents stored within the knowledge base. This allows us to identify content that might need updating or expansion to avoid becoming obsolete. It's like a quality control system for the knowledge itself.
The feedback mechanisms within these knowledge bases can also be improved using machine learning. It helps ensure that user input is effectively integrated to continuously refine the relevance and usability of the content. It's a continuous cycle of improvement, allowing the knowledge base to adapt to the changing needs of its users.
Lastly, machine learning has shown promising results in reducing training time for new employees. By providing tailored training resources that address specific knowledge needs, onboarding can become more efficient and impactful. Instead of wading through generic information, new employees can dive directly into the knowledge most relevant to them, reducing training time by up to 50%. This leads to a smoother transition into the organization and increased productivity right from the start.
While the potential of using machine learning within internal knowledge bases is impressive, there are always challenges to consider. We'll need to be vigilant about data privacy and potential biases within the algorithms. But, it's clear that the ongoing development of AI and related technologies is significantly changing how we approach knowledge management. These systems are not just repositories anymore—they are evolving into intelligent hubs capable of learning, adapting, and helping us bridge knowledge gaps in new and unexpected ways. It's an exciting area of research and development, and it will be interesting to see how it continues to shape the future of organizational knowledge.
The Evolution of Internal Knowledge Bases From Data Silos to AI-Powered Collaboration Hubs - Fostering a Culture of Continuous Learning and Collaboration
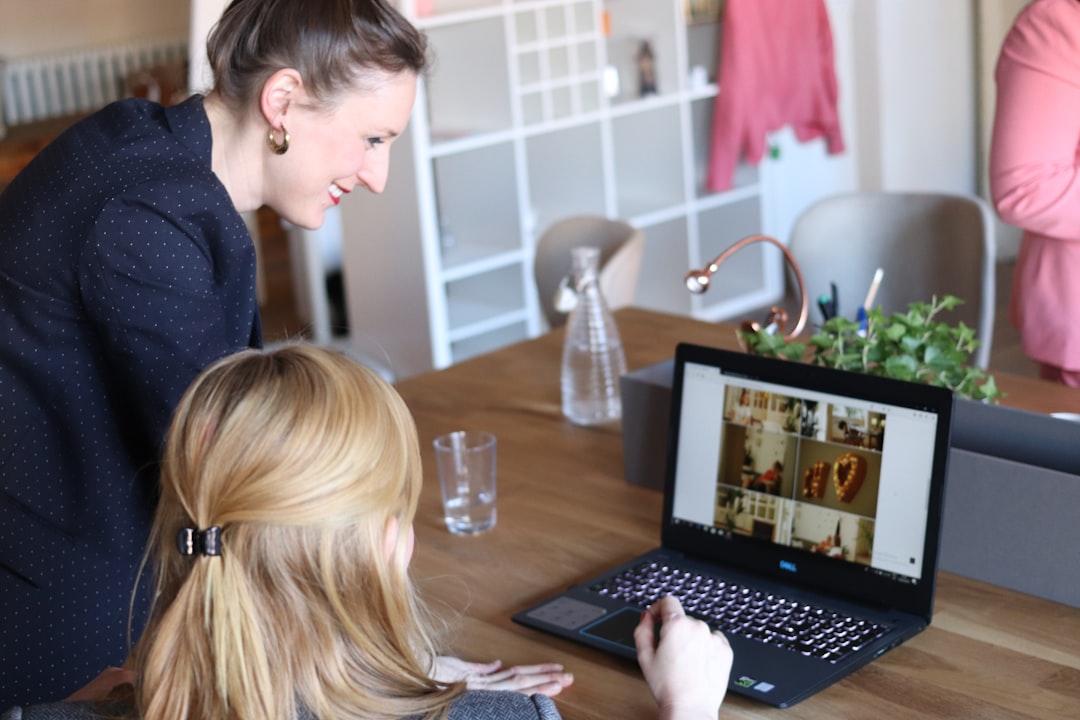
Cultivating a culture that prioritizes continuous learning and collaboration is crucial for organizations navigating today's rapidly changing landscape. This isn't simply about occasional training but rather a deep-seated commitment to ongoing personal and organizational growth. Learning becomes a continuous process where both triumphs and setbacks contribute to the development of knowledge and skills. Central to this approach is the creation of internal knowledge repositories that go beyond basic onboarding. They provide a steady stream of training opportunities and act as platforms for shared learning experiences. This fosters stronger collaboration and increases employee engagement across teams. Further enhancing this environment is the development of feedback mechanisms, which promote openness, help organizations adjust to new realities, and empower employees to actively contribute to innovative ideas and the expansion of shared knowledge. The transition to this learning-focused culture reveals that the true value of information isn't found in its static storage but in its active use and constant refinement within the everyday operations of the organization.
The shift towards AI-powered knowledge hubs isn't just about consolidating data; it also emphasizes the need for cultivating a culture that values ongoing learning and collaboration. We see a strong connection between organizations that foster a continuous learning environment and their capacity for adaptation and innovation. The evidence suggests that individuals learn and retain information more effectively when actively engaged in collaborative settings. This isn't just about enhancing training outcomes; it's about fundamentally changing how knowledge is perceived and utilized within an organization.
It's become increasingly clear that traditional training methods often fall short in their ability to promote lasting knowledge retention. In contrast, when employees are encouraged to share their knowledge and learn from one another, retention rates can increase significantly. The very act of participating in knowledge sharing can strengthen memory and improve understanding. Collaboration tools integrated within knowledge bases have been shown to dramatically increase the frequency of knowledge sharing amongst employees. This increase is tied to the simple convenience of these tools, but it also represents a cultural shift in how people view and interact with information.
These shifts have a noticeable impact on an organization's capacity for innovation. We're seeing a clear correlation between environments that encourage continuous learning and increased rates of innovation. When teams actively share their understanding and insights, the chances of developing new, creative ideas or solutions grow. This continuous learning environment doesn't just impact innovation; it also leads to faster decision-making. It's fascinating how easier access to shared knowledge can bolster confidence in employees' ability to make sound choices.
A further impact is seen on employee engagement. It's apparent that employees are often more engaged and satisfied when they feel a sense of belonging to a learning community. These environments appear to reduce employee turnover and create a more positive and productive workplace. Continuous learning can also help organizations adapt more quickly to evolving circumstances. When information flows freely between departments and employees actively contribute to a shared understanding, organizations can respond more quickly to change.
One of the more interesting aspects of this evolution is how AI can play a role in supporting human collaboration within knowledge management systems. While AI can automate many processes and improve the efficiency of knowledge retrieval, it shouldn't be seen as a replacement for human interaction. AI can be particularly valuable in highlighting overlaps and connections within existing knowledge, encouraging employees to build upon existing understanding. In this sense, it acts as a catalyst for collaboration, providing a foundation for further insights.
There is evidence that when organizations embrace a culture that supports continuous learning, their employees develop necessary skills at a significantly faster pace. Collaboration enhances the speed at which employees can adapt to new information and integrate it into their work. While the integration of AI into knowledge-sharing systems is a promising development, maintaining a human-centered approach is essential to ensuring that these platforms foster meaningful collaboration and support a culture of continuous learning. The human element remains key in leveraging insights from AI, ensuring that individual perspectives and insights are integrated into the collective knowledge base. The future of organizational knowledge management seems increasingly tied to this careful balance of human ingenuity and AI support.
More Posts from :