ServiceNow Washington DC Release 7 Key AI-Powered Workflow Improvements for 2024
ServiceNow Washington DC Release 7 Key AI-Powered Workflow Improvements for 2024 - Text Analysis Bot Improves ServiceNow Search Through Document Processing
ServiceNow's Washington DC release introduces a "Text Analysis Bot" designed to improve how users find and process information within the platform. The bot utilizes text analytics to automatically extract meaningful data from various document formats, like PDFs and scanned images. This capability is intended to make searching more effective by finding relevant information within knowledge bases more quickly.
The bot's user interface is built to be easy to use, meaning users without a lot of coding experience can still define what information to extract from documents and even train AI models to improve the bot's abilities. This ability to customize the bot could lead to faster document processing, potentially lowering the time spent manually reviewing and entering data. Overall, the inclusion of this bot shows ServiceNow's ongoing efforts to apply generative AI to solve real-world problems within the platform. However, it is important to note that the full potential of some of the features, such as text-to-code, may not be readily available to all users in the initial release.
ServiceNow's latest release has introduced a Text Analysis Bot that aims to drastically improve the way users interact with its knowledge base. Instead of relying solely on keyword matching, the bot utilizes natural language processing to understand the context behind a search query, which is a significant leap forward for more nuanced information retrieval.
The bot learns over time, using machine learning to refine its search capabilities based on user interactions. This means search accuracy becomes more precise with usage, adapting to individual preferences and the evolution of information within the platform. Interestingly, it's not limited to one language, which has great potential for businesses operating across various global markets.
Beyond just identifying relevant documents, the bot can analyze the sentiment expressed in text. This enables it to prioritize urgent matters based on the emotional tone of a document, allowing for potentially faster responses to critical issues. Moreover, the ability to process unstructured data like PDFs and scanned images, transforming them into searchable formats, automates a process that can be incredibly time consuming when done manually.
Looking ahead, it appears they're focusing on a predictive element as well. The bot can anticipate user needs by suggesting relevant search results even before a query is fully typed, which could lead to a massive increase in workflow efficiency. Further, its capacity for "entity recognition" means it can automatically pull key data like names, dates, and locations from documents – this automated data extraction could play a crucial role in the automated creation of tasks and follow-ups.
The system's design includes user feedback loops, allowing users to rate the relevance of search results. This user input is critical for ongoing refinement of the bot's algorithms, fostering continuous improvement in performance. Additionally, the bot can tap into external data sources, enhancing the scope of searches and allowing users to access relevant data from multiple platforms. Of course, with access to sensitive information, there's a need for security protocols. From what I understand, the bot is built to comply with strict standards, which is paramount in many of the industries that might utilize this type of platform.
ServiceNow Washington DC Release 7 Key AI-Powered Workflow Improvements for 2024 - Natural Language Interface Adds Context Aware Responses To Service Desk
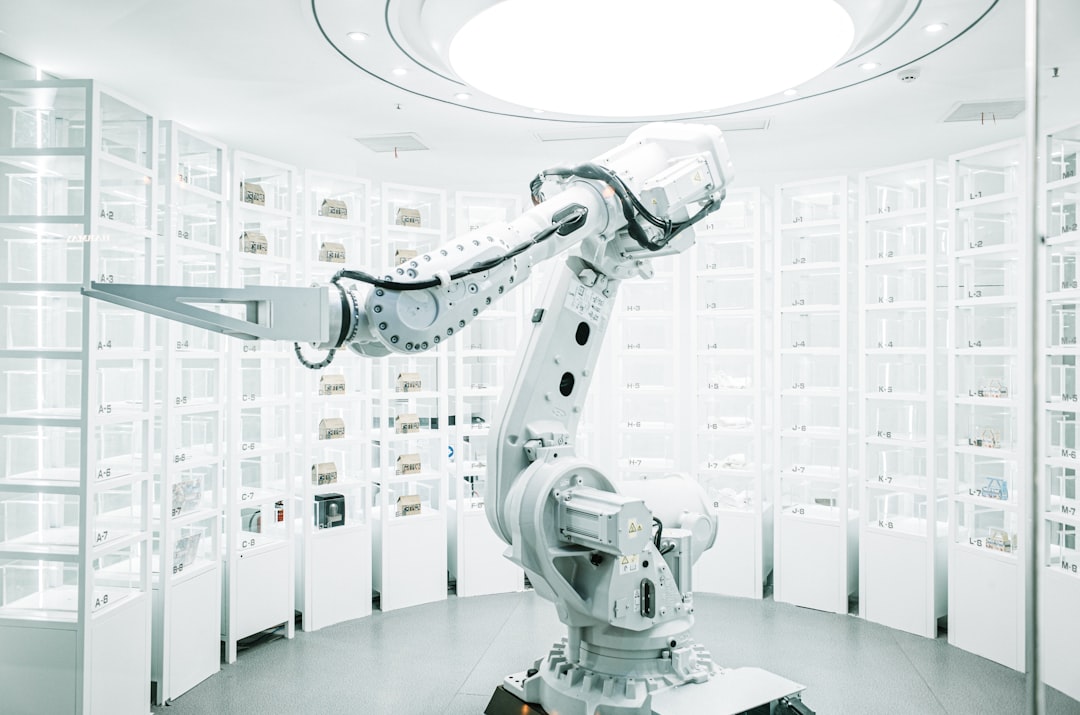
ServiceNow's Washington DC release brings a boost to the service desk by enhancing its natural language interface. This means the system is now better at understanding the context of user requests, allowing it to provide more intelligent and relevant answers. This improvement is driven by advances in Natural Language Understanding (NLU) which helps the system decipher what users really want when they ask questions. The Virtual Agent, a core part of the service desk, now benefits from these NLU improvements, offering 24/7 support for users needing help with common issues or wanting to complete certain tasks.
This is all part of ServiceNow's larger effort to incorporate more advanced AI features into their platform. The idea is to make the overall experience more intuitive and productive for users, especially when interacting with the service desk for troubleshooting or completing actions. However, it remains to be seen how successful these AI enhancements will be in practice and if they can consistently meet user expectations for a truly contextual and useful interaction.
The ServiceNow Washington DC release brings a significant enhancement to their service desk with a new natural language interface. This interface aims to improve how the system understands and responds to user requests by incorporating context awareness. It's essentially a step towards bridging the gap between human language and machine understanding, which is a complex and fascinating area of AI research.
They're using machine learning to make the interface smarter over time. The system is designed to adapt and learn from past interactions, allowing it to better understand user intent based on the context of their inquiries. This is a very useful approach, especially for service desks that handle a wide variety of requests and need to provide fast and accurate responses.
One interesting aspect is its ability to handle multiple turns in a conversation. This means the system can keep track of the topic at hand, even as a user asks follow-up questions or clarifies their initial request. This 'conversational memory' has the potential to make interactions with the service desk more seamless and efficient.
The underlying technology uses advanced natural language processing models that are capable of interpreting a range of wording styles and syntax. This means a user doesn't have to worry about phrasing a query in a specific way for the system to understand it. This adaptability can significantly improve the user experience and eliminate some of the frustration associated with interacting with less-intelligent systems.
There's a clear push towards integration with other enterprise applications. This can create a unified service desk platform, potentially streamlining workflows by pulling together different sources of information within a single interface. While this has the potential to be beneficial, integrating disparate systems can sometimes introduce new challenges.
A rather important aspect of this interface is its ability to identify and prioritize tasks based on the emotional tone within a user's request. In stressful situations, quick identification of a critical issue can be important for directing resources to solve problems promptly. It's certainly a novel concept that might have interesting applications beyond the service desk.
The system also seems geared towards automating responses to common requests, freeing up human agents to tackle more complex tasks. While this shift towards automation can help improve efficiency, it's crucial that the system’s responses are accurate and helpful to avoid creating more problems than it solves.
Of course, in any system that handles sensitive data, security is a major concern. The team has apparently built-in robust security measures to protect the information exchanged through natural language interactions. Adherence to data privacy standards like GDPR is a necessity, especially for organizations that handle personal information.
Beyond simply answering queries, the interface is designed to be proactive. It can suggest relevant topics or follow up on past interactions, aiming to provide a more dynamic and personalized service experience. This is a great step towards building a genuinely helpful and intuitive system.
Lastly, user feedback plays a crucial role in the continuous improvement of the interface. The designers have built in mechanisms to collect user feedback, allowing them to refine the accuracy and relevance of responses over time. This commitment to ongoing improvement is an important sign that the system will continue to evolve to meet the needs of its users.
Overall, the new natural language interface shows some promise for improving user experience and streamlining service desk operations. As with any technology, there will likely be both benefits and challenges as it's implemented and used within the larger ServiceNow environment. It will be interesting to see how it develops and if it can achieve its full potential in practice.
ServiceNow Washington DC Release 7 Key AI-Powered Workflow Improvements for 2024 - Automated Policy Summaries Replace Manual Document Reviews
ServiceNow's Washington DC release introduces a new way to handle policy documents by automating the creation of summaries. This change aims to replace the tedious process of manually reviewing documents, which can save time and improve compliance efforts. This release also boosts overall workflow automation with tools like Workflow Studio and Decision Builder, allowing for better control over approvals. This focus on automation, fueled by artificial intelligence, is intended to speed up various business operations.
While using AI to make policy management more efficient is certainly a positive development, it's important to consider if these automated summaries are truly effective in dealing with the complex rules and regulations organizations face. The impact on efficiency and overall compliance still needs to be seen in real-world applications. Still, this initiative is part of a larger drive to use technology to transform businesses and improve how work gets done, so it's a significant step in that direction.
The ServiceNow Washington DC release brings in automated policy summaries, a change aimed at streamlining compliance by doing away with the manual review process. This shift suggests a potential for significant efficiency gains, though it's worth considering if it will truly meet the complexities of nuanced policy documents. One aspect I find interesting is that this feature, theoretically, can cut down the time spent on policy reviews substantially. This could be a boon for organizations dealing with large volumes of policy documentation or those with tight deadlines.
However, the accuracy of these automatically generated summaries is a concern. While claims of reduced error rates are common in the field of AI, it's essential to understand how these systems handle edge cases and ambiguities that are common in complex policies. Perhaps, we can anticipate better accuracy with future improvements in the natural language processing that drives these features.
One point they highlight is scalability. This is sensible, as manually reviewing large numbers of policies is a major bottleneck. This automated approach, if successful, could handle surges in policy-related work without needing a major ramp-up in staff. This is certainly attractive from a cost and resource perspective.
These automated systems boast the ability to digest and summarize many different document types. I imagine it would be handy if it can truly handle a wide range of file formats, making it easier to manage documents from diverse sources. This uniformity could lead to better consistency in how summaries are generated, but whether the output will be truly helpful across a diverse set of document styles remains to be seen.
Another aspect is the use of Natural Language Processing (NLP). NLP is designed to help these automated summaries go beyond simple keyword extraction and understand the context and main points of documents. This nuance is crucial for policy documents, as they often involve complex language and interconnected clauses that can't be simply paraphrased. While this is a promising direction, I wonder if the current state of NLP technology is advanced enough to fully grasp the subtleties of legal and regulatory language.
These summaries, according to the documentation, can integrate with existing document management systems, hopefully without requiring an extensive overhaul. However, I believe it's important to analyze the real-world impacts of integration with various enterprise systems. Often, compatibility and data transfer can lead to unexpected complexities and require careful planning and development.
User customization is another aspect that seems promising. It can potentially lead to more useful summaries if users can control how things are summarized. However, providing user controls in a way that is both flexible and intuitive can be a challenge.
Regarding audit trails, this is certainly crucial for any system handling sensitive information like policies. The ability to track changes to summaries and how they were generated can be very beneficial for compliance with regulations and internal controls. I believe this will also be crucial for building trust in the technology.
There's also a mention of learning capabilities, which would mean that over time, the system would learn and refine how it summarizes documents. This is a key area in AI development, and if they get this right, the summaries could become more accurate and useful.
Lastly, the documentation points out the ability to support many languages, which could potentially improve global operations. This is particularly relevant for businesses operating in diverse markets and needing to standardize policies across language barriers. However, there can be considerable challenges in achieving accurate translation and handling linguistic nuances across different languages and cultural contexts.
The promise of these automated policy summaries is certainly appealing, but it's crucial to understand the challenges that likely exist. How well these systems will adapt to different policy formats and language styles remains to be seen. There are significant questions about the reliability and accuracy of AI-generated policy summaries, which will be crucial for organizations to understand as they evaluate if this is a suitable replacement for manual processes. Nonetheless, the future of policy management potentially lies in this direction, so understanding these early releases is a crucial step for those in the field.
ServiceNow Washington DC Release 7 Key AI-Powered Workflow Improvements for 2024 - Process Mining Tool Maps And Optimizes Enterprise Workflows
ServiceNow's new Process Mining tool is a significant addition to their workflow optimization arsenal. It essentially maps out how work is done within an organization by creating visual representations of workflows, built from existing data. This clear visualization allows users to spot problems like bottlenecks and inefficiencies that might not be obvious otherwise. It's designed to help organizations make swift adjustments to improve their operations, relying on AI to analyze patterns and provide insights that lead to improvements.
This tool used to be called "Process Optimization", but the renaming to "Process Mining" highlights that it's become more capable and versatile. It's promoted as a solution that scales across multiple ServiceNow workflows—like the ones used in IT, customer service, HR, and custom applications built on the platform. While the promise of a streamlined, optimized workflow is appealing, the actual effectiveness in diverse and complex business environments remains to be seen. There's a possibility that the tool's insights might not be as useful or as universally applicable as the initial marketing suggests. Still, its ability to potentially automate aspects of process improvement could lead to more efficiency and less time spent manually trying to identify and fix workflow problems.
ServiceNow's Process Mining tool, previously known as Process Optimization, helps businesses understand how their work actually gets done by analyzing data from their systems. It essentially creates a map of a business process flow by examining data like timestamps and task completions. This approach, rooted in data mining and process management, lets you see how processes really work, not just how they're supposed to work.
This approach can highlight unexpected issues – maybe a certain task is always delayed due to an approval step nobody thought about. Or, it can show that a workflow is not behaving as designed and needs refinement. Some of the newer tools can even provide near real-time insights, letting you react to performance issues as they happen.
Process Mining goes beyond simply visualizing workflows. It considers various aspects of the process like time, cost, and resources used. This holistic view makes it easy to see how different factors influence a workflow's efficiency. It can help find the balance between resources and results. And, some of the more advanced versions can even use this historical data to predict future workflow issues and bottlenecks.
What's nice is that the visualization side of this is often user-friendly. So, whether you're an executive or a worker, understanding the workflow visually makes participation in optimization efforts easier. Furthermore, these tools can connect with other systems like CRM and ERP platforms. That lets you leverage your existing technology investments to build a more complete picture of your workflows. You can even benchmark how well you're doing compared to other businesses in the industry.
The really cool part is that Process Mining can be used for compliance monitoring. By automatically mapping out how processes are completed, you can easily demonstrate that you're following regulations. This can make audits easier and reduce the chances of penalties. Although it's not typically the primary focus of the technology, it is a rather nice side-effect of being able to visualize complex workflows in such a fashion.
It's interesting to think about the true cost of inefficiencies. While most companies might realize there are problems, they might not realize how expensive they are in the long run. Research suggests even seemingly small delays in workflows can turn into big problems over time. The takeaway for me is that the ROI on investing in a Process Mining tool can be pretty good for those looking to optimize the work they do. It really provides a novel way to see and fix problems which, from a research perspective, seems quite intriguing.
ServiceNow Washington DC Release 7 Key AI-Powered Workflow Improvements for 2024 - AI Security Controls Monitor Machine Learning Model Performance
The ServiceNow Washington DC Release 7 introduces enhancements designed to monitor how well AI models are performing, specifically focusing on security aspects. This is a critical step as more and more AI-powered tools become part of business processes. Essentially, these security controls act like a watchdog for the AI, making sure it operates reliably and doesn't run into problems with data security or biases within the model itself. The goal is to give organizations a greater degree of control over how AI is used, especially in areas where they handle sensitive information.
This new monitoring approach is meant to improve compliance, which is important for organizations needing to adhere to certain regulations. It also helps strengthen the overall reliability of the platform’s AI features. While this is a promising development, it remains to be seen how effectively these security measures will play out in practice. As organizations become increasingly reliant on AI, they'll be looking for ways to ensure that the benefits of AI come without compromising security or data privacy. It's a balance that needs to be carefully managed. There are sure to be challenges and opportunities as this technology matures.
ServiceNow's Washington DC release brings a focus on AI security within the context of machine learning models. This means that beyond just ensuring the models are accurate, we're also looking at how they behave in real-world settings. It's not enough for a model to be accurate if it's susceptible to unforeseen problems like "data drift". In simpler terms, data drift occurs when the kind of data a model is trained on changes over time, which could lead to its performance degrading.
The new tools allow for a closer watch on how models are performing in real-time. We're talking about collecting and analyzing various performance metrics – think of this like having a constant stream of information about the model's health. If something goes wrong, like unexpected outputs, the systems can be configured to immediately flag it for review. It's an efficient way to stay on top of potential issues in a fast-changing environment.
Another interesting development is the automated identification of strange patterns. If a model starts doing things it shouldn't, like consistently giving out odd predictions, this can trigger an alert. This level of automatic detection can be useful in keeping the model running smoothly.
However, there are some challenges. The complexity of these security features can vary greatly depending on the specific industry and compliance standards. For example, a financial institution has different requirements than a healthcare provider, so ensuring the monitoring setup follows those regulations is a key part of this.
Further, there is a growing focus on the quality of data being used to train the model. It's essential to prevent scenarios where the input data is flawed or biased. Models are only as good as the data they are fed, so tools that validate and clean data are crucial.
Scaling these monitoring systems across multiple environments can be tricky as well. There's a need for uniformity in how different models are assessed and tracked, and if we want to monitor performance across various data sources, that can be a challenge. This likely requires collaboration between data scientists and cybersecurity teams – a strong communication loop between these groups can be essential in identifying and fixing problems effectively.
This whole space is evolving quickly with greater regulatory pressures around data privacy and AI accountability. Tools are needing to be more comprehensive, not only tracking performance but also compliance. It's an extra layer of complexity for the system.
Interestingly, we're now seeing a move towards more sophisticated analytics within monitoring systems. For instance, some systems are starting to not only track changes in performance but also attempt to identify the underlying reason behind them. Understanding *why* a model is behaving in a particular way is an exciting area of development that could pave the way for even more robust monitoring capabilities in the future.
It's still early days for many of these AI-powered security controls, but they hold the potential to enhance our confidence in how machine learning is used. The key is to ensure that these controls can adapt to the evolving landscape of AI applications and regulations. As the field progresses, it will be fascinating to see how these monitoring capabilities improve and become integral to ensuring AI applications remain both beneficial and trustworthy.
ServiceNow Washington DC Release 7 Key AI-Powered Workflow Improvements for 2024 - Predictive Analytics Dashboard Forecasts IT Service Disruptions
The ServiceNow Washington DC release introduces a Predictive Analytics Dashboard designed to anticipate potential problems with IT services. This new feature uses historical data, along with techniques like machine learning, to try and predict when disruptions might occur. The idea is that by seeing these potential disruptions coming, organizations can be ready to respond more quickly and avoid bigger issues.
This dashboard builds on ServiceNow's push towards using AI to automate tasks and improve workflows. It allows users to identify patterns in data and even train AI models to get better at predicting problems. This could potentially improve how work is routed to the right teams, leading to faster resolution times.
With the growing reliance on technology for all aspects of business, the ability to predict potential IT service hiccups is becoming more crucial. Being able to foresee disruptions is a big help in keeping everything running smoothly. However, the practical success of these predictive features still needs to be proven. It remains to be seen how well it can handle the complexity of real-world IT systems and the accuracy of its forecasts.
The ServiceNow Washington DC release brings in a new predictive analytics dashboard that aims to forecast potential problems with IT services before they happen. It does this by analyzing historical data, using clever statistical methods and machine learning to spot patterns that indicate future issues. This approach is meant to give a clearer picture of what might go wrong and when, helping to maintain smooth operations and prevent outages.
Having data-driven insights is a big improvement over relying solely on hunches or intuition. With the predictive capabilities, ServiceNow users can make decisions about how to best allocate resources and improve service delivery based on concrete evidence rather than guesswork. The hope is that this will translate into better service performance.
The platform can also be set up to recognize unusual behavior within IT systems in real-time. If the system observes anything out of the ordinary that could be a precursor to a problem, it flags it instantly. This allows for immediate fixes and helps to keep interruptions to a minimum. This aspect has implications for reducing downtime, which is obviously a positive in many settings.
Beyond simply detecting potential service disruptions, ServiceNow has begun to incorporate data on individual user behavior to make the experience more personalized. By understanding user patterns, the platform can offer custom notifications or preventive actions based on a user's history with the platform. This has the potential to increase user satisfaction, though I wonder about the privacy concerns that might accompany such a feature.
This whole approach integrates well with established ITIL (Information Technology Infrastructure Library) practices, particularly when it comes to proactive incident management. Organizations can anticipate potential problems and respond in a more coordinated and efficient way, thereby adhering to IT service best practices.
One of the advantages of this is the ability to look across multiple IT systems and find relationships between them. This could be valuable in a complex setting where different components interact and an issue in one place could ripple through others. For instance, perhaps a network bottleneck impacts server performance, and this feature could help find those relationships.
The beauty of the dashboard is that it’s adaptable to a variety of settings. Whether your IT infrastructure is all in the cloud, on your own hardware, or a hybrid mix, the analytics engine is designed to work with it. This means that diverse organizations can use it without having to change a lot about how they manage their IT systems. It's more adaptable and can potentially pull data from different systems, which is a plus for its usefulness.
In theory, using predictive analytics to head off problems should mean reduced costs. Organizations should spend less on dealing with surprise issues or needing to do urgent repairs. If it is truly effective, the operational efficiency and cost savings could be significant.
By looking at the historical patterns within IT service operations, organizations can get a much better idea of what’s likely to happen in the future. The platform can help users see repeating problems or seasonal trends and can then adjust their operational strategies to be prepared for them. How well it does in this regard remains to be seen.
The designers of the dashboard have focused on making the information it presents easy to understand. It uses lots of visuals to show the data, which should help everyone from system admins to executives easily see what the risks are and make better decisions based on them. The visual presentation is quite important if the insights are going to be useful to a broader audience, so hopefully, it's been designed well.
While it’s relatively new, the predictive analytics dashboard has the potential to significantly improve how IT services are managed. It seems like a powerful tool for enhancing both reliability and cost-effectiveness. However, as with many AI-powered tools, the true benefits and challenges are likely to become clearer as more organizations start to implement them in the real world.
ServiceNow Washington DC Release 7 Key AI-Powered Workflow Improvements for 2024 - Service Catalog Assistant Personalizes Employee Technology Requests
The latest ServiceNow release, Washington DC Release 7, includes a new feature called the Service Catalog Assistant designed to make requesting technology resources more personalized. This feature uses AI to analyze employee requests, understand their needs, and offer suggestions tailored to each individual. This means, instead of a generic catalog, the assistant can anticipate common requests and help users more quickly find what they need. Another change for administrators is the ability to directly link users to their corresponding consumer records using a lookup list. This makes managing user accounts within the system much easier. Further enhancing the employee experience, the Service Catalog Assistant now includes a conversational interface. This interface allows for a more natural back-and-forth, with the assistant explaining complex policies or helping guide users through complex requests. These changes are intended to make the Service Catalog a more flexible and responsive resource for employees seeking technology solutions. Whether it truly accomplishes that goal will depend on how well the system adapts to the different needs of its users, and if there are unforeseen issues introduced with these changes.
The ServiceNow Washington DC release introduces the Service Catalog Assistant, a feature that aims to personalize technology requests for employees. It does this by learning from the patterns in how people ask for things, like what type of equipment they typically request and how often. The idea is to make suggestions that are more likely to be relevant and useful, hopefully reducing the time employees spend searching through a menu of options. It seems they're building up a profile of user behavior, which could lead to a smoother experience.
This also extends to potentially predicting future needs. By looking at past trends, like spikes in requests around certain times of year, the system could anticipate when resource demands might increase. This is interesting as it moves away from the more traditional reactive model where IT has to respond to surges in requests on the fly. It could help avoid potential issues with shortages or bottlenecks.
Furthermore, the Service Catalog Assistant can attempt to sort requests based on how important they are. They accomplish this by looking at historical data from tickets and service-level agreements (SLAs) to figure out which requests are truly urgent. This prioritization could be a very handy feature to help IT direct their attention to the highest-priority issues.
One notable feature is the ability to process requests written in natural language. Employees don't need to use specific technical jargon or terms to ask for what they need; they can just write a clear, straightforward sentence. This avoids some of the errors that occur when people interpret technical forms incorrectly, which is good for user experience and could potentially reduce the number of incorrectly submitted requests.
The system includes a feedback loop as well. After getting a suggestion, employees can provide feedback on how relevant the suggestion was. This kind of ongoing interaction is important, as it allows the system to improve and adjust its suggestions based on what people find useful. If the feedback loop is implemented well, it could mean the assistant gets smarter and better over time.
The ability to integrate with other systems, like enterprise resource planning (ERP) platforms, is also significant. This could create a seamless flow of data for the system, avoiding a lot of manual re-entry of information. The goal here is to create a smoother and more unified experience for users who have to work with a number of different systems. However, as with any integration, there are likely to be some challenges in achieving a truly smooth transfer of data between diverse systems.
Security is important, especially in a setting where sensitive information is often exchanged. I noticed the system is built to adhere to typical security and compliance standards, which makes sense given that requests for access to computing resources or software are often sensitive in nature. The use of role-based access control looks like a prudent measure to ensure that only those authorized can view and process a request.
The system can also be customized to some degree. There appears to be the ability to change how things are presented and grouped, making it easier to support different team requirements within a single system. This flexibility is important as different groups within a large organization will likely have varied requirements for IT support, and the system looks to have been designed with this need in mind.
An interesting aspect of this assistant is its ability to recognize recurring requests and potentially respond automatically. It could automate routine answers, which can free up IT staff to handle more complex issues. I imagine this has the potential to reduce the workload on help desks while simultaneously providing a more consistent response to commonly requested items.
Lastly, the assistant can analyze the tone of a request to gauge a user's emotion or urgency. This is interesting, as it opens up the possibility for more human-centered support. It might be able to pick up when someone is in distress and flag the request to receive immediate attention. This approach could potentially make the user experience more empathetic, but, again, it's important to note that the success of such a feature will depend on how well it's developed and deployed.
In general, the Service Catalog Assistant shows promise for improving how employees request and obtain access to technology resources. There are a number of features that could potentially improve the user experience, streamline the request process, and help IT manage resources more effectively. Whether it lives up to its full potential will depend on how it performs in practice and how well the design team can handle the inevitable challenges associated with implementing new AI features.
More Posts from :