ServiceNow's Agent Client Collector Enhancing Predictive AIOps with Comprehensive Log Management
ServiceNow's Agent Client Collector Enhancing Predictive AIOps with Comprehensive Log Management - ACC's Log Compilation Enhances ServiceNow's Predictive AIOps
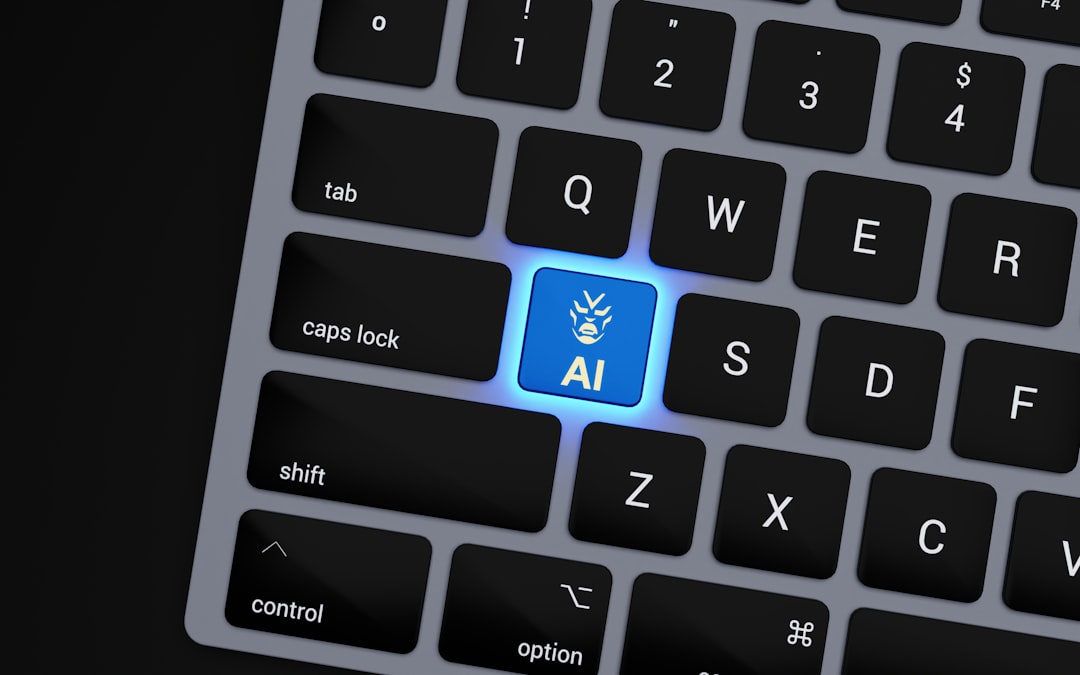
ServiceNow's Predictive AIOps gains a significant boost from its Agent Client Collector (ACC) due to the ACC's ability to gather and process logs. This integration connects log data with IT Operations Management, creating a more holistic view of what's going on across a company's infrastructure, whether it's in a data center or the cloud. The ACC's role in gathering and combining data from various sources into one platform provides a powerful foundation for ServiceNow's Predictive AIOps. Organizations using this setup are seeing positive outcomes. They're experiencing fewer major incidents, and when issues do arise, they're resolving them faster. This improvement is driven by ServiceNow's capacity to take the flood of log data and other operational data and transform it into usable information. By continuously collecting data and applying predictive modeling, ServiceNow's AIOps aims to prevent issues before they impact services, reducing the need for manual intervention and streamlining incident response. In essence, the ACC acts as a central point for gathering operational information, allowing teams to better anticipate and proactively handle service disruptions.
ServiceNow's Agent Client Collector (ACC) plays a vital role in enhancing ServiceNow's Predictive AIOps by bringing log data into the mix. It's capable of consolidating logs from various ServiceNow instances, creating a central hub for monitoring and issue resolution. This centralized approach speeds up the process of spotting problems across multiple systems, leading to reduced downtime.
The ACC's ability to handle both structured and unstructured log data is interesting. It boosts the predictive models' accuracy by providing a fuller picture of system health, something which wouldn't be as apparent with just one type of log data. This is key, since combining different sources can help detect more nuanced issues.
ACC has the advantage of connecting log events with performance metrics, uncovering hidden patterns traditional monitoring tools might miss. This correlation is crucial for getting ahead of potential problems and averting service disruptions before they become major outages. Its compatibility with diverse log formats, like JSON and XML, makes it very adaptable to environments with various technologies. This feature is attractive because it encourages leveraging existing logging infrastructure instead of requiring massive new tooling.
ACC employs machine learning algorithms to analyze vast log datasets for anomalies. By identifying unusual patterns humans might miss, engineers can act faster to fix them. Its real-time alerts, activated when pre-set boundaries are crossed, allow engineers to quickly respond to incidents, minimizing downtime and streamlining workflow. This automated log analysis, in the context of ServiceNow's Predictive AIOps, takes a significant burden off the shoulders of the engineering teams, automating a tedious process. This is particularly beneficial when dealing with a high volume of logs, and it also decreases the chance of human error.
The ACC builds a foundation for accurate predictions of future performance and potential service disruptions by establishing baselines of typical operational behavior based on historical log data. Visualizations of log data through dashboards enable clearer communication between engineers, helping them swiftly spot and understand the impact of issues on service delivery. Ultimately, deploying the ACC can positively impact customer satisfaction, due to the reduced frequency and faster resolution of service interruptions, leading to a more dependable experience and increased confidence in the underlying IT systems. There are potential tradeoffs in using this approach however, including complexity and accuracy in correlation of log data to problems.
ServiceNow's Agent Client Collector Enhancing Predictive AIOps with Comprehensive Log Management - Sensu Framework Integration Enables Customizable Monitoring
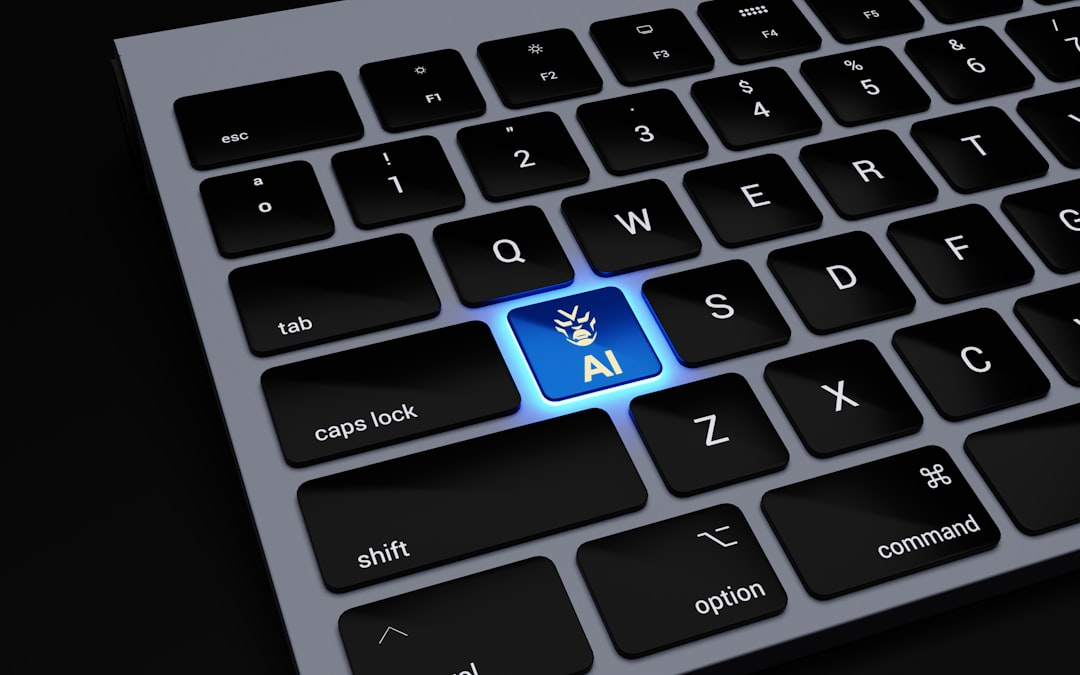
ServiceNow's Agent Client Collector (ACC) gains a boost in flexibility thanks to its integration with the Sensu framework. This integration enables customizable monitoring, allowing organizations to tailor monitoring checks to their unique environments. This feature is helpful when dealing with a diverse mix of technologies and infrastructure components. The ability to modify or create custom monitoring checks makes it easier to adapt to changes in IT systems and ensures that specific monitoring needs are addressed effectively.
While this customization empowers users, it also adds a layer of complexity to the system. Setting up and maintaining custom monitoring checks might be more challenging compared to simpler, pre-defined checks. Teams must also be prepared to handle the potentially increased complexity in troubleshooting when issues arise.
Furthermore, by utilizing the Sensu observability pipeline, the ACC can bridge the gap between various monitoring tools within the ServiceNow platform. This consolidation creates a more complete picture of system health by bringing together different sources of data into a single view. This unification contributes to a more comprehensive understanding of operational environments. This can make it easier to spot patterns and trends which might be missed if monitoring information is scattered across several systems. It's important to recognize that achieving the intended benefit of this consolidation requires careful planning and configuration. Despite this additional complexity, the ability to consolidate monitoring from various sources enhances operational efficiency. However, it's crucial for organizations to carefully consider the complexity of implementing and maintaining this framework to reap the advantages of customized monitoring.
The ServiceNow Agent Client Collector (ACC) leverages the Sensu framework, opening up a world of customization possibilities in the realm of monitoring. This integration allows engineers to tap into a rich library of over 100 plugins, adapting monitoring to fit the specific needs of their diverse environments. This focus on customization can lead to more pertinent data and, ultimately, more useful insights.
This integration isn't just about metrics; it combines them with log data, which creates a more comprehensive understanding of system behavior. By examining both sides of the coin—metrics and logs—potential patterns and inconsistencies that might be missed with a single-source approach can come to light.
Furthermore, Sensu boasts a highly efficient event processing system, able to tackle a massive influx of data—a capability essential for today's rapidly evolving IT infrastructures. It doesn't just handle stable systems, either. Sensu can dynamically monitor transient assets, like containers in cloud environments that are constantly popping up and disappearing. This is vital in keeping monitoring relevant in highly dynamic setups.
Engineers have the flexibility to build custom monitoring checks using Ruby, allowing them to create very precise scripts tailored to specific application needs or intricate business logic. This fine-grained control empowers more focused alerting, leading to a reduction in noise. The Sensu framework also supports parallel execution of these checks, significantly reducing the overall time needed for monitoring tasks and quickening the pace of incident detection.
In contrast to many monitoring tools that limit users to a rigid set of scripts, Sensu embraces flexibility by supporting check definitions in multiple languages. Engineers can choose whatever suits their needs and expertise, making the system more adaptable to their workflows.
Sensu also offers real-time dashboarding capabilities that make monitoring data readily visible. This instantaneous visualization streamlines decision-making processes, a crucial feature during incident response. The fact that Sensu is open-source, with a vibrant community contributing to its development, also makes it more adaptable and resilient to changes in technology. This continuous stream of updates and improvements from the community keeps the tool relevant in the fast-paced world of IT.
Its cross-platform design makes Sensu a versatile solution for both on-premises environments and cloud setups. This means businesses can achieve consistency in their monitoring strategy, regardless of the complexity and heterogeneity of their infrastructure. While the ACC and its Sensu underpinnings offer a lot of potential, there are also questions about potential issues. For example, customizing the framework may take considerable skill, and using it effectively for complex environments might introduce some intricacies in correlation of log data to problems. But, all in all, this flexible, customizable approach offers a powerful foundation for improving observability and supporting the larger AIOps initiative within ServiceNow.
ServiceNow's Agent Client Collector Enhancing Predictive AIOps with Comprehensive Log Management - ACC Deployment Across Infrastructure for Comprehensive Data Collection
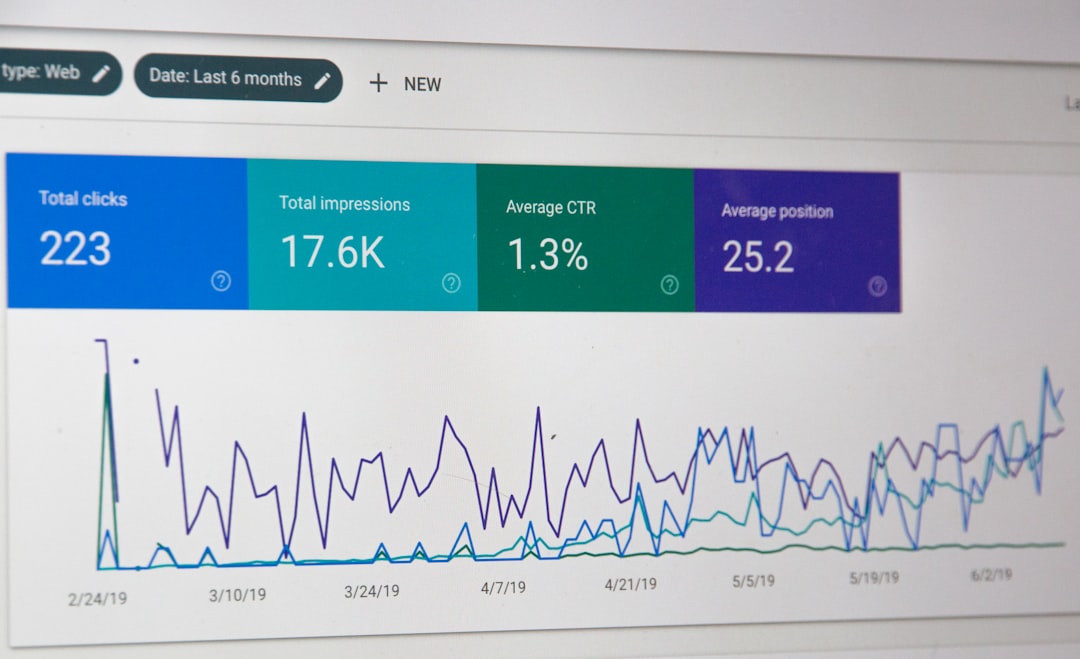
The ServiceNow Agent Client Collector (ACC) plays a crucial role in gathering data across a company's infrastructure for comprehensive analysis. It's installed on various system components, both in data centers and the cloud, making it a valuable tool for creating a holistic view of operational data. The ACC gathers logs and combines them with performance metrics, providing a more detailed picture of the system's health. This ability to collect and consolidate information from various sources is vital for enabling proactive actions to prevent problems and speed up the resolution of issues that do arise. The underlying Sensu framework makes the ACC adaptable and customizable, a positive feature that can also be a source of challenges. While being able to tailor monitoring to specific needs is very helpful, configuring and maintaining customized checks can add to the complexity of the system and may make debugging issues a more difficult process. Ultimately, the ACC offers substantial advantages in monitoring and incident management. However, it's important to weigh the added complexities involved in leveraging its full potential to make sure the gains outweigh the operational challenges.
The Agent Client Collector (ACC) is designed to work across various parts of a company's infrastructure, including servers and devices at the edge. It's essentially an agent that's installed on these components, making it possible to gather data and keep an eye on how things are running. The fact that it builds on the Sensu framework means it's flexible enough to adapt to the way a company monitors its systems, whether they want to use the standard checks Sensu provides or create their own.
From there, the ACC does its work by running commands on the machines where it's installed and then sending the results of those commands to the ServiceNow system through something called a MID Server. The data that's collected, which can include both events and metrics, gets stored in ServiceNow's database. This is interesting because it helps to provide a unified view of monitoring, discovery, and log collection all in one place. One benefit is that it builds on top of how ServiceNow already does credential-based discovery, giving it a more complete picture of things.
It seems like the ACC is meant to enhance existing tools rather than replace them. For example, it lets ServiceNow use its AI-based log analytics capabilities to make sense of the flood of log data coming from across an infrastructure.
Moreover, it helps with tasks like figuring out what hardware and software a company is using because it can gather information about the operating systems on machines where it's installed. It can be beneficial for investigations by being able to fetch important data about metrics and logs, making it easier to solve incidents.
The ACC is engineered to operate across a mixed bag of environments, such as those that still use traditional data centers and cloud-based infrastructure. This gives it a unique capability to offer a consolidated view of all that infrastructure from a single pane of glass. This could be useful if you need to see the big picture and make decisions accordingly. But, it does come with the complexity of needing to set up how you want to collect and integrate your data, and any problems could have wider-reaching implications. It is, however, a step forward in gaining a holistic view of a company's infrastructure for a wide variety of monitoring needs.
ServiceNow's Agent Client Collector Enhancing Predictive AIOps with Comprehensive Log Management - Single Agent Solution Supporting Multiple IT Management Use Cases
The ServiceNow Agent Client Collector (ACC) is a single agent that handles many IT management tasks. This includes things like keeping track of system performance, gathering log data, and managing hardware and software assets. Because it uses the Sensu framework, you can easily adapt the ACC to your specific monitoring needs, making it well-suited for diverse infrastructure environments. The ACC gathers data from a variety of places, both within a company's data centers and out in the cloud, allowing for a more complete view of what's going on. This expanded view allows for better anticipation and identification of potential issues, plus faster incident handling. However, the flexibility and adaptability also create some complexities in setting up and managing the agent, which organizations need to be aware of. If you can handle that, the ACC has the potential to improve operational effectiveness and improve how transparent your IT infrastructure is.
1. **Centralized Data Gathering:** The Agent Client Collector (ACC) is interesting because it can gather logs and operational metrics from various systems at the same time, providing a single view that gives a much better understanding of what's going on in IT. It's like having all the different monitoring tools talking to each other, reducing the chance of missing something important.
2. **Adaptability Across Environments:** ACC is designed to work equally well in both the cloud and traditional data centers, which is great for companies with a mix of infrastructure. This means their monitoring approach can be consistent, regardless of the complexity of their setup.
3. **Real-Time Data Handling:** Built on the Sensu framework, ACC can handle a large volume of data in real-time, which is essential these days. It makes a difference for companies that need instant feedback on how their systems are performing, especially when combined with log data.
4. **Automated Anomaly Detection:** ACC has built-in machine learning tools that can analyze massive amounts of log data. This can be really useful for finding issues that might otherwise be missed, like unusual patterns or behavior. This proactive approach can help avoid larger problems.
5. **Customization with Sensu Framework:** The integration with Sensu provides access to more than 100 built-in plugins for monitoring, allowing users to tailor their checks to their specific technology needs. This leads to more specific and actionable information.
6. **Flexible Scripting Languages:** Instead of being tied to a single scripting language, ACC allows engineers to choose from multiple languages for defining custom checks. This is helpful because engineers can use what they already know best, improving the overall usability and adaptability of the system.
7. **Monitoring Short-Lived Resources:** The ACC can monitor things like containerized apps in cloud environments, which are constantly appearing and disappearing. This feature ensures monitoring stays relevant even when dealing with highly dynamic infrastructure.
8. **Quick Action with Visualizations:** It provides real-time dashboards for easy visualization of data, speeding up decision-making during critical incidents. This can be crucial when trying to address problems quickly.
9. **Predictive Capability:** ACC helps to build up a picture of "normal" system behavior by looking at past log data. This can aid in predicting future issues before they impact services, allowing IT teams to be proactive instead of reactive.
10. **Complexity Considerations:** While ACC is a powerful tool, it also introduces more complexity, particularly in setup and management. Companies need to consider whether the customization it offers outweighs the added effort needed to keep it running smoothly.
ServiceNow's Agent Client Collector Enhancing Predictive AIOps with Comprehensive Log Management - AI-Driven Event Consolidation Reduces Alert Noise Significantly
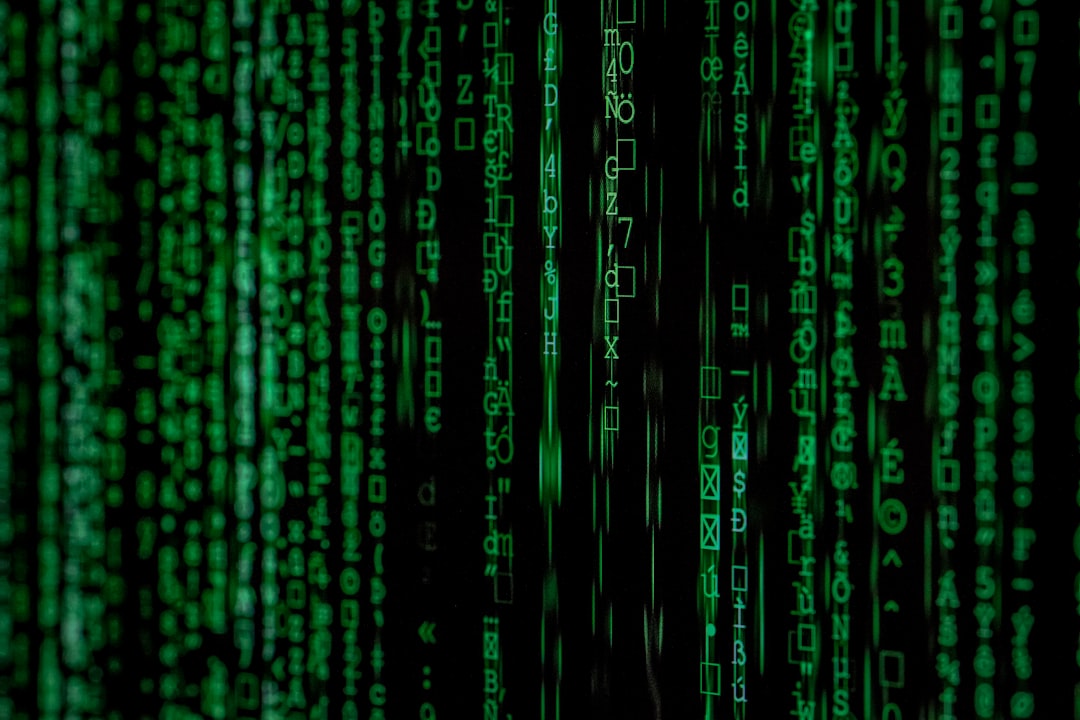
AI-powered event consolidation plays a crucial role in reducing the overwhelming number of alerts that can flood IT teams. By intelligently grouping and analyzing events from different monitoring tools, it filters out the noise, delivering a focused set of alerts that are actually important. This consolidation can dramatically decrease alert volume, sometimes by as much as 99%, freeing up engineers to tackle problems rather than wade through a constant stream of notifications. The ability to effectively tag and categorize events also streamlines the process of pinpointing the source of service disruptions. This combination of noise reduction and improved event analysis results in more efficient operations and smoother incident management. It helps IT teams make quicker, better-informed decisions when problems do arise. While these are valuable benefits, implementing and managing such a complex system effectively can be difficult, so careful consideration is needed before adoption.
AI-powered event consolidation is proving to be a game-changer in the realm of incident management, particularly when it comes to significantly reducing the overwhelming amount of alerts that can flood IT teams. By automatically grouping related alerts, these systems effectively filter out the noise, allowing engineers to focus on the truly critical issues instead of being bombarded by a constant stream of possibly irrelevant notifications. This ability to discern meaningful alerts from the sea of data helps teams react more efficiently, potentially resulting in substantially faster incident resolution.
The speed at which these AI systems process data is also remarkable. They're able to analyze log information in real time, instantly detecting anomalies and triggering alerts, which is crucial in today's fast-paced IT environments. This ability to quickly identify and address issues can lead to a substantial reduction in the time it takes to resolve problems, potentially cutting resolution times in half or even more compared to traditional approaches.
Beyond immediate responses, AI can also aid in understanding the underlying causes of recurring problems. By analyzing historical log data, AI algorithms can pinpoint patterns that contribute to repeated incidents. This is beneficial because it helps engineers get to the root of the issue rather than just addressing the surface symptoms, leading to more robust system stability over time. This also plays a role in shortening the mean time to acknowledge (MTTA) of issues. By focusing engineers' attention on relevant issues, they spend less time sifting through the noise and more time working on real problems, which could lead to a substantial decrease in MTTA.
Furthermore, AI systems are capable of handling both high-level summaries of system performance and detailed, granular data analysis. This flexibility is really helpful for gaining broad system understanding while allowing engineers to drill down for specific details when needed. This two-sided view provides a much richer operational insight into system behavior.
An important aspect of AI-driven event management is the capability to prioritize alerts. AI can intelligently rank alerts based on their historical impact and level of urgency. This capability ensures that truly critical incidents are quickly brought to the attention of engineers for immediate action, leading to improved service quality and potentially higher user satisfaction due to fewer disruptions and faster recovery times. These prioritized alerts are also a critical piece in improving predictive capabilities. When combined with event consolidation, AI can effectively analyze past data to predict potential issues with increased accuracy. By learning from historical data, these systems are able to flag problems before they impact services, allowing engineers to be proactive in preventing incidents instead of reacting after the fact.
Most AI-driven alert management systems are designed to work seamlessly with existing monitoring frameworks, which means organizations can integrate this technology into their existing IT infrastructure without needing a complete overhaul. This ease of integration is a plus as it lowers the barrier to adopting these advanced capabilities. One of the other positive impacts of reducing alert noise is that it mitigates the constant pressure engineering teams experience from being overloaded with alerts. This reduction in alert fatigue can potentially increase morale and job satisfaction for engineers because it reduces the feeling of being constantly bombarded by information. Finally, AI systems are not static; they are continuously learning. They are designed to refine their algorithms based on feedback they receive on the actions taken in response to the alerts they generate. This ongoing learning process results in ever-improving noise reduction and incident management strategies. While promising, the effectiveness of these AI systems also hinges on the quality of the data they are fed. There may be situations where the correlation of events is not accurately represented, leading to incorrect conclusions. Overall, however, AI-driven event consolidation holds immense potential to revolutionize how IT teams manage incidents.
ServiceNow's Agent Client Collector Enhancing Predictive AIOps with Comprehensive Log Management - Real-Time Data Ingestion Powers Predictive Incident Management
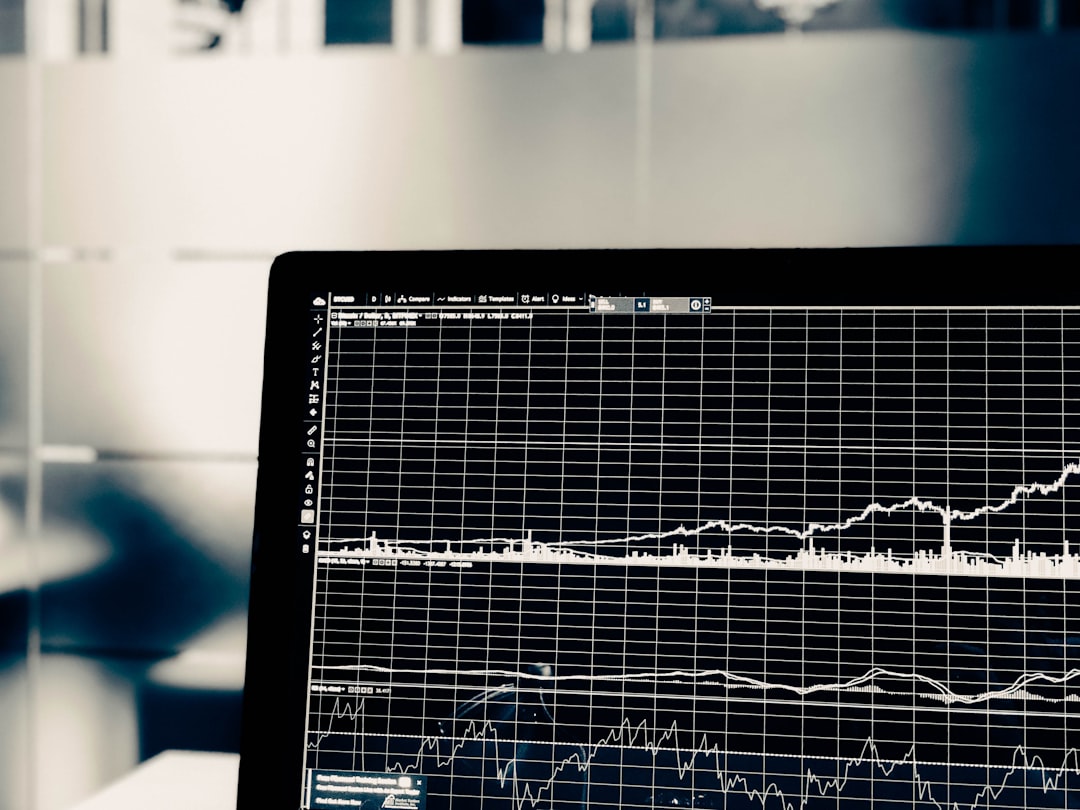
Real-time data ingestion is crucial for predictive incident management because it enables organizations to capture and process a large volume of operational data as it happens. Systems like ServiceNow's Agent Client Collector are examples of how this works, constantly gathering and analyzing log information, which is key to recognizing irregularities in real time. This immediate feedback allows for better detection and quicker response, leading to a more streamlined process of categorizing and handling issues. By using machine learning algorithms on this live data, past patterns can be utilized to guide actions that help prevent service outages from occurring. However, there are hurdles associated with this constant flow of information—ensuring data accuracy and managing the model's complexity require a careful and balanced implementation.
Real-time data ingestion, particularly of logs, is proving vital for making predictive incident management a reality. It's all about handling massive amounts of log data practically instantaneously. This allows systems to instantly recognize unusual patterns or behaviors that deviate from the norm—a process we call anomaly detection. It's critical for systems that must remain online at all times, as the quicker the system can spot a problem, the better it can manage the situation.
It's remarkable the accuracy that's achievable with modern anomaly detection algorithms. These approaches can often detect anomalies with a rate exceeding 90%, which helps significantly in the effort to anticipate issues before they become significant problems. We also see the benefit in the unification of data into a single interface, which does a lot to remove any blind spots teams might experience when managing events in fragmented systems. With a single source for viewing everything, there's a natural improvement in incident response. It also encourages teams to connect the dots when investigating a problem. Finding a problem that is isolated to one component is helpful, but connecting that issue to others is helpful in understanding its bigger impact.
Systems for real-time log ingestion need to be very scalable, as the volume of data being managed can become enormous, especially as companies grow. Many systems can manage hundreds of thousands of logs every minute, which is a big advantage if you need to handle large and complex environments. Interestingly, this focus on real-time processing has also resulted in systems capable of a greater reduction in the number of false alarms. While alarms are important, an overwhelming quantity can lead to engineers ignoring important signals. A significant reduction in false alerts, sometimes as much as 80%, is a major win for reducing alert fatigue.
The benefit of real-time data also extends to historical analytics, which is often combined with it. Organizations can store all of this real-time data in what's called a data lake, creating a huge reservoir of information that can be used to predict future issues. This capability is incredibly valuable, as it allows teams to be more proactive in preventing problems. The same real-time processing that helps quickly spot problems also drastically speeds up the process of understanding the root cause of problems. This is great because it reduces recovery times for service interruptions.
Modern systems have to adapt quickly, and log ingestion is no exception. We see systems designed to instantly react to changes in infrastructure, such as adding new services or switching between clouds. It's a huge advantage to not have to manually reconfigure monitoring with every infrastructure change, ensuring a consistent level of observability. Much of this is aided by using machine learning, which can learn from these constant data streams, allowing for more accurate predictions about system behavior. While all of this seems beneficial, there are also some potential downsides, and careful planning and management are needed to balance the gains with any potential operational burden.
More Posts from :