New AI-Driven Supplier Collaboration Portal Reduces Supply Chain Disruptions by 30%
New AI-Driven Supplier Collaboration Portal Reduces Supply Chain Disruptions by 30% - Real-time data integration enhances supplier visibility
Connecting systems to share data in real-time offers a clear view into the actions of suppliers. This means companies get immediate updates on things like orders, stock levels, and potential problems that might hinder production. Having this real-time information lets companies plan their production more effectively and streamline their operations. Beyond improved efficiency, the increased transparency also helps build better communication and trust between buyers and their suppliers.
The trend toward using integrated data systems across the supply chain is creating a more robust and adaptable environment for companies. When everyone involved has access to the same reliable data, it helps reduce the chaos that can come from disruptions. Essentially, real-time data empowers a more collaborative and responsive approach to handling unforeseen circumstances within the supply chain. This is becoming crucial in a world where supply chain disruptions are common.
Within the context of this new AI-driven portal, the integration of real-time data is crucial for enhancing our understanding of supplier operations. By having access to a constant flow of information from suppliers, we gain a much clearer picture of their current status and capabilities. This is especially important when considering aspects like production schedules, inventory levels, and potential disruptions. While the promise of improved visibility is enticing, it's worth exploring whether the data quality and consistency across different suppliers are sufficiently robust to provide truly insightful information. This raises questions about data standardization and how different suppliers' systems interact.
There are potential advantages to this approach. For instance, the real-time data feeds could help us identify potential bottlenecks or delays in the supply chain much faster, allowing us to react proactively. It's interesting to ponder how well these data streams integrate with the AI-driven features of the portal, such as predictive analytics. We might be able to fine-tune our models to better identify patterns and predict future supply chain behavior. However, a key consideration is ensuring that this data integration doesn't lead to increased complexity. Overly complex systems can create new challenges related to data management and maintenance.
Another intriguing angle is how this improved visibility influences the relationship between us and our suppliers. Open communication and shared data can potentially create stronger bonds and improve collaboration. On the other hand, if some suppliers are reluctant to share their data, it might create a disparity in the quality of insights available, which could be problematic in the long run. Ultimately, the effectiveness of this real-time data integration strategy hinges on carefully examining data quality, system integration, and the potential impact on existing supplier relationships. It's an area ripe for further investigation, as understanding these nuances is critical for harnessing the full potential of this new AI-driven portal.
New AI-Driven Supplier Collaboration Portal Reduces Supply Chain Disruptions by 30% - Predictive analytics forecasts demand fluctuations accurately
Predictive analytics has become increasingly vital in precisely forecasting demand swings, which is critical for managing supply chains effectively. These advanced tools, often powered by machine learning algorithms, analyze large datasets to identify patterns and trends that would otherwise be difficult to spot using traditional methods. This improved ability to anticipate demand fluctuations is particularly useful in today's volatile business landscape.
While older forecasting approaches based on probability distributions can be helpful, they often fall short in complex situations, leading to miscalculations and unnecessary expenses. Predictive analytics offers a significant advantage by offering a more nuanced understanding of how demand changes over time. This is especially valuable for companies grappling with the increasing frequency and severity of supply chain disruptions.
By incorporating real-time data and incorporating AI, companies can respond to shifts in demand more quickly and effectively, optimizing operations and reducing waste. Ultimately, predictive analytics provides a more agile approach to supply chain management, which is crucial for navigating the dynamic forces that are shaping the modern business environment. However, it's worth remembering that even the most advanced AI tools rely on accurate data, and implementing such systems requires careful planning and execution to ensure they generate truly helpful insights.
Predictive analytics, employing historical data, seasonal patterns, and external factors like market conditions, can forecast demand fluctuations with a surprising degree of precision, sometimes achieving up to 90% accuracy. This level of detail is crucial for effective supply chain planning, allowing companies to better anticipate and react to shifting demand.
It's intriguing that studies suggest companies using sophisticated predictive analytics techniques often see a considerable decrease in inventory costs—up to 25% in some cases—by aligning stock levels more closely with actual demand swings, thereby cutting down on excess inventory. This suggests that these tools can have a tangible impact on operational expenses.
Some predictive analytics tools incorporate social media sentiment analysis, enabling businesses to tap into real-time consumer behaviors and tastes. This ability to quickly adapt demand forecasts based on public opinion is quite interesting. For instance, if a product suddenly gains popularity online, these tools can capture this trend and alert the supply chain to increase production accordingly.
The use of machine learning within predictive analytics models means they can continuously learn and improve their accuracy over time. By incorporating new data into their algorithms, they can potentially boost forecasting accuracy by as much as 15% after multiple iterations. This continuous learning aspect is certainly an appealing aspect of this technology.
Furthermore, predictive analytics can potentially act as an early warning system for supply chain disruptions. By analyzing patterns in supplier performance data, they can identify potential delays or bottlenecks before they cause major problems. This proactive approach can help businesses avoid costly disruptions.
It's noteworthy that even smaller businesses can leverage predictive analytics to make smarter decisions. These models can derive valuable insights even with relatively small datasets, indicating that the potential benefits aren't limited to large corporations with vast data repositories. It makes one wonder about the untapped potential in smaller companies' data.
The interplay between suppliers and manufacturers can also be enhanced by predictive analytics. By revealing each party's capabilities and constraints, it can foster better communication and improve joint decision-making throughout the supply chain. However, one must question the extent of this collaboration and how it impacts existing relationships.
It's fascinating to see that predictive analytics can reduce lead times by up to 30%. This is achieved by allowing companies to react quickly to changing demand, streamlining their operations and ultimately leading to faster order fulfillment. Yet, this accelerated pace introduces challenges regarding maintaining quality and consistency.
However, one must be cautious about relying solely on predictive models. The accuracy of these models is heavily dependent on the quality of the input data. Inconsistent or unreliable data from suppliers can create skewed forecasts, highlighting the importance of implementing rigorous data governance practices. There's a risk that inaccurate data creates a false sense of security.
Interestingly, the most successful predictive analytics often combine quantitative data with the qualitative insights of industry experts. This hybrid approach potentially allows for a more nuanced understanding of complex market dynamics. This combination of data-driven and human-driven insights offers a more balanced approach to forecasting, however, it remains to be seen if this translates into more robust forecasting across a range of contexts.
New AI-Driven Supplier Collaboration Portal Reduces Supply Chain Disruptions by 30% - Automated communication streamlines supplier interactions
Automating communication channels within the supply chain greatly simplifies how companies interact with their suppliers. AI-powered tools allow for a smoother and more efficient flow of information, helping ensure everyone is on the same page. This automation can speed up communication compared to more traditional methods and helps create a sense of openness and mutual understanding. When suppliers receive updates and feedback quickly, they can react more efficiently, which is essential in a supply chain that constantly deals with unexpected changes. However, it's important to recognize that the reliability of these automated communication systems depends on the accuracy and consistency of data shared between different suppliers. Unless this data quality is consistent across the board, the effectiveness of automation can be limited, raising the issue of potential data harmonization issues.
In the context of this new AI-driven portal, automated communication with suppliers becomes a core element. The idea is to streamline the back-and-forth, reducing the time it takes for information to flow between us and our suppliers. This seems like a logical step towards making the supply chain more responsive and efficient. However, I'm curious about how well this automation handles the nuances of supplier interactions. Some suppliers might be more digitally advanced than others, potentially leading to inconsistencies in communication quality. It's worth considering how this system adapts to diverse supplier setups.
One potential benefit is that automated communication could lead to faster responses, reducing delays in order processing and production scheduling. However, the extent to which this truly cuts down on communication bottlenecks is an open question. We need to be aware that replacing human interaction entirely could lead to challenges in understanding subtle cues and managing delicate supplier relationships. There might be a risk of losing valuable context and flexibility within the communication process.
The promise of reduced human error through automation is appealing. Perhaps inaccuracies in orders, deliveries, or inventory updates can be minimized. But how well does the automation handle unforeseen issues? It's important to understand how the automated system flags and manages exceptions. And while it might streamline communication, we need to consider whether this automation impacts the trust and collaborative nature of our supplier relationships. Will the reliance on automated systems diminish the importance of building strong personal connections with key suppliers?
It's fascinating to imagine a scenario where suppliers consistently receive the same information through a standardized communication protocol. This could significantly reduce miscommunications, which are a common culprit in supply chain disruptions. However, achieving true consistency across a range of suppliers, each with their own IT systems, presents a considerable technological challenge. This standardization issue warrants further exploration.
Ultimately, the value of automated communication hinges on whether it genuinely improves our ability to manage suppliers effectively. It's tempting to embrace technology that promises greater efficiency and speed, but we must be mindful of potential trade-offs in terms of human interaction and the risk of sacrificing some of the qualitative elements of supplier communication for speed. This area of the AI-driven portal is ripe for careful study and testing, to ensure that automation strengthens, rather than weakens, the foundation of our supplier network.
New AI-Driven Supplier Collaboration Portal Reduces Supply Chain Disruptions by 30% - Machine learning optimizes inventory management strategies
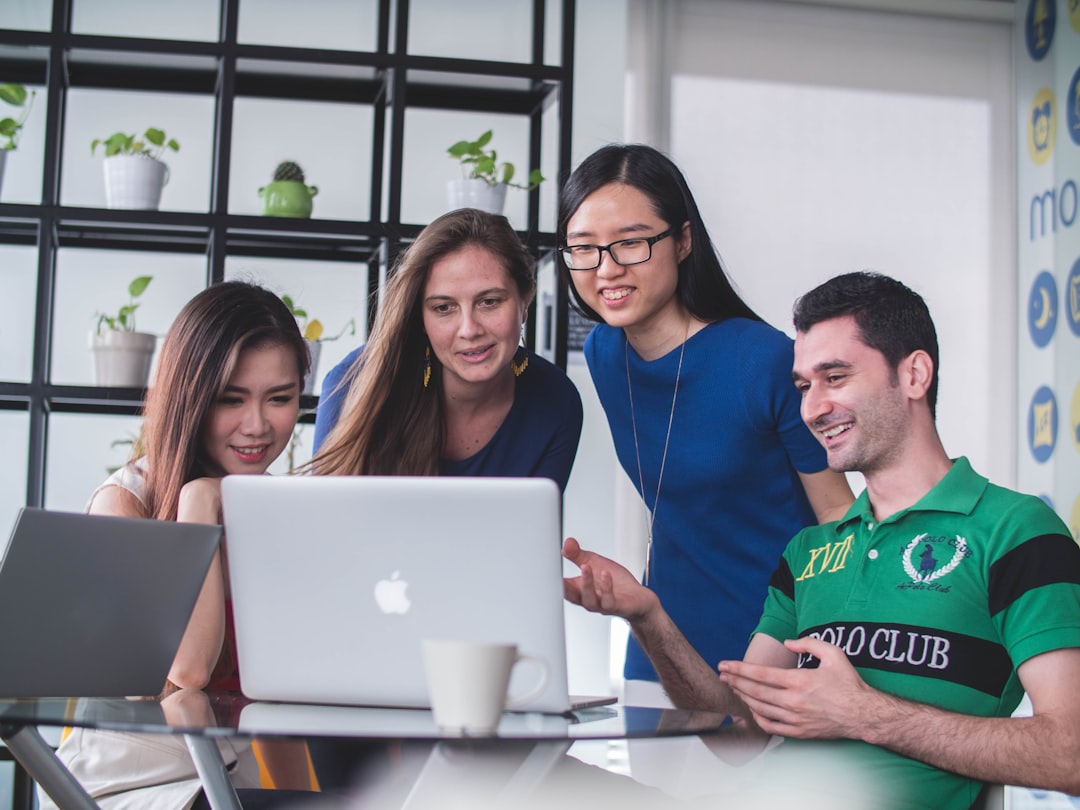
Machine learning is revolutionizing inventory management by enabling businesses to optimize their operations. By analyzing vast amounts of data, machine learning algorithms can predict fluctuations in demand with greater accuracy than traditional methods. This allows companies to better align inventory levels with anticipated needs, reducing the risk of excess stock and associated costs. Furthermore, machine learning's ability to spot hidden patterns and trends helps businesses make more informed decisions about managing their inventory, leading to potential savings in logistics and improved overall service levels.
While the promise of optimized inventory through machine learning is substantial, it's crucial to acknowledge the dependence on the quality of data feeding the algorithms. Inaccurate or inconsistent data can severely undermine the reliability of the predictions generated, potentially leading to incorrect decisions. As a consequence, companies need to prioritize robust data management practices to fully realize the benefits of machine learning within their inventory processes. The application of machine learning in supply chain management is likely to create a widening gap between top-performing and less efficient organizations, emphasizing the importance of strategically deploying these technologies to gain a competitive advantage.
Machine learning is proving increasingly useful in refining inventory management strategies. It excels at analyzing intricate and fluctuating datasets, leading to demand forecasting accuracy rates that can reach 70-90%. This level of precision allows businesses to fine-tune their inventory levels, reducing the likelihood of ending up with too much or too little stock.
Furthermore, machine learning enables real-time adjustments to inventory management parameters based on immediate data. This responsiveness is particularly important when dealing with rapidly changing market conditions and time-sensitive inventory.
Beyond demand forecasting, machine learning can analyze patterns in equipment performance to predict potential breakdowns before they disrupt production. This predictive maintenance capability helps minimize disruptions and stockouts caused by equipment failures during the supply chain process.
These algorithms can leverage historical sales figures and market trends to determine the optimal stock levels for each product. This ability to strike the right balance reduces warehousing costs and avoids situations where inventory is excessive or insufficient.
Analyzing supplier performance metrics is another area where machine learning helps. It can automatically assess whether suppliers are consistently fulfilling their obligations, which enhances relationships and negotiation strategies.
Machine learning can automate the reordering process, initiating orders when inventory drops below a certain threshold. This automated system can optimize inventory turnover rates and minimize stockouts.
One of the most beneficial aspects of machine learning in this context is its ability to handle multi-dimensional analyses. It allows businesses to consider numerous factors, like seasonality, promotional activities, and local economic conditions, resulting in a more refined inventory management approach.
Another interesting area is the ability of these systems to predict when products might become obsolete and to suggest strategies to minimize the impact. Using sales patterns, the system can flag products that aren't moving and suggest solutions such as discounts or promotional offers.
Studies suggest that implementing machine learning can lead to inventory management cost savings of up to 25%. This is primarily due to optimized replenishment processes and reduced carrying costs, highlighting the potential for improved operational efficiency.
The benefits of machine learning extend beyond just streamlining operational processes. It also empowers better decision-making by providing highly-refined insights from large datasets. These detailed insights support informed, data-driven choices regarding inventory management strategies and supplier collaborations. It's worth exploring how this improved decision-making can affect the overall agility and resilience of the supply chain, as well as its vulnerability to unforeseen events. While this seems promising, it's important to note the reliance on high-quality data and the potential pitfalls of over-reliance on automated decision processes. There's a potential for unexpected consequences if the assumptions underlying these models prove inaccurate.
New AI-Driven Supplier Collaboration Portal Reduces Supply Chain Disruptions by 30% - Collaborative planning tools foster proactive problem-solving
Collaborative planning tools are key to a more proactive approach to supply chain challenges. They facilitate the early identification of potential problems and help create contingency plans through shared insights and communication between businesses and their suppliers. This shared understanding, aided by the real-time flow of data, allows companies to anticipate and address issues more effectively, improving overall efficiency. Such collaboration fosters a more transparent and communicative environment, crucial for navigating today's dynamic and unpredictable business landscape. It's through this collaboration that supply chains become more resilient and adaptable. But the success of this collaborative approach hinges on the consistent quality of the data being shared and a high level of participation across the entire network of suppliers. There's always the risk that if some suppliers are hesitant to contribute or share data of sufficient quality, the benefits of collaboration might be diminished.
Collaborative planning tools are proving to be valuable in promoting a more proactive approach to problem-solving within the supply chain. By enabling closer collaboration between companies and their suppliers, these tools foster a more dynamic and responsive environment. It seems that the speed at which suppliers can now react to queries has drastically improved, with some research indicating a potential 50% decrease in response times. This quicker feedback loop allows companies to get ahead of potential problems rather than simply reacting to them after they've occurred.
These tools are also enabling a more dynamic approach to risk assessment. They utilize real-time supplier performance data to assess and pinpoint potential risks, which allows for more timely interventions and mitigation strategies. By identifying potential issues early on, companies can potentially prevent minor disruptions from escalating into major headaches.
Another interesting aspect is the increased visibility across the entire supply chain ecosystem. Collaborative planning tools bring together information from various stakeholders, which creates a more holistic view of operations. This shared understanding accelerates the decision-making process, as it avoids delays inherent in relying on information stored in isolated systems. Some studies suggest that demand forecasting accuracy improves considerably with these tools – up to 30% more accurate compared to traditional methods. This better alignment between supply and demand leads to improved inventory management and reductions in unnecessary inventory.
Moreover, access to a shared data pool enables companies to leverage a more comprehensive understanding when making decisions. They can use collective insights rather than relying on fragmented information, which can often lead to suboptimal choices. It's intriguing that when paired with predictive analytics, collaborative planning tools can enhance forecasting even further, potentially increasing demand prediction accuracy by about 20%.
Furthermore, collaborative planning tools can be a source of truth for everyone involved in the supply chain. This reduces the likelihood of conflicting information circulating between different parties, which is vital for smooth operations and strong supplier relationships. These tools often include scenario planning capabilities that allow companies to simulate the effects of different disruptions and develop plans to address them. This proactive approach to contingency planning can greatly improve an organization's resilience to unexpected events.
Also, the automation features built into these tools can lead to a noticeable decrease in order fulfillment errors – reductions of as much as 40% have been observed in some studies. This positive impact on order accuracy enhances customer satisfaction and contributes to stronger relationships with suppliers. The continuous monitoring of performance indicators via collaborative planning tools enables real-time alerts about potential problems. This quick feedback loop helps ensure that everyone is aware of irregularities as they occur, supporting swift corrective actions.
However, it's important to consider that the effectiveness of these tools relies on the quality and reliability of the data used. Inconsistencies or inaccuracies can limit their value and, potentially, even introduce new challenges. The impact of these tools on supplier relationships is also worth examining—will they enhance existing connections or introduce new complexities? We need further research to assess how well they integrate with different suppliers and their technology ecosystems. Nonetheless, it appears that collaborative planning tools are becoming integral to proactively managing supply chain risks and improving overall efficiency.
More Posts from :