How AI-Driven Scheduling Reduced Labor Costs by 8% A 2024 Case Analysis
How AI-Driven Scheduling Reduced Labor Costs by 8% A 2024 Case Analysis - Medecipher Case Study Shows 23% Drop in ER Staff Overtime Hours
A recent case study using Medecipher's AI-powered scheduling system demonstrated a 23% reduction in overtime hours for emergency room staff. This reduction, coupled with an overall 8% decrease in labor costs for the ER, suggests AI can help address longstanding issues in healthcare staffing. The 2024 study serves as a strong example of how AI might optimize scheduling and potentially ease the pressures on healthcare facilities.
While this case study indicates positive outcomes, it's crucial to consider the bigger picture. The pandemic highlighted the strain on healthcare workers, pushing burnout rates higher. AI-driven solutions may offer relief to some of those issues, but more research is needed to understand if these tools actually address workforce challenges, burnout, and related concerns. It remains important to carefully analyze how such technologies impact the long-term well-being of healthcare professionals and the quality of patient care.
A case study from Medecipher revealed a noteworthy 23% decrease in overtime hours for emergency room staff after implementing AI-powered scheduling. This suggests that aligning staffing levels with predicted patient demand, a capability facilitated by AI, can lead to substantial operational improvements. While labor expenses can constitute a significant portion – potentially up to 50% – of emergency department operational costs, it's intriguing that AI-driven scheduling achieved an 8% overall reduction in labor costs.
This reduction in overtime is not just about the financial benefits. It potentially contributes to reducing staff burnout, which has been a rising concern in healthcare. If we can better schedule staff, aligning their hours with actual needs, perhaps we can see an improvement in the well-being of ER personnel.
Predictive models are proving useful in forecasting patient arrival patterns. As studies show that the bulk of ER patients tend to arrive during peak times, AI algorithms can help optimize staffing during those periods. However, there's still potential for refining these models to better account for unexpected surges in patient volume.
The challenge of efficient staffing in emergency departments is long-standing, with historical data suggesting that many facilities have historically understaffed shifts during times of increased patient need. It's through the intelligent use of these insights that AI is demonstrably changing things. Furthermore, minimizing overtime can free up staff time for direct patient care, potentially impacting both patient outcomes and satisfaction.
A challenge here is that the adoption of automation – particularly in the context of generative AI – can be accompanied by changes in the labor market, which we saw significantly during the pandemic. While this specific case shows benefits in the ER setting, exploring the broader implications and potential impacts of AI across the healthcare landscape is crucial.
How AI-Driven Scheduling Reduced Labor Costs by 8% A 2024 Case Analysis - Machine Learning Algorithm Adapts to 1,500 Staff Schedule Changes Monthly
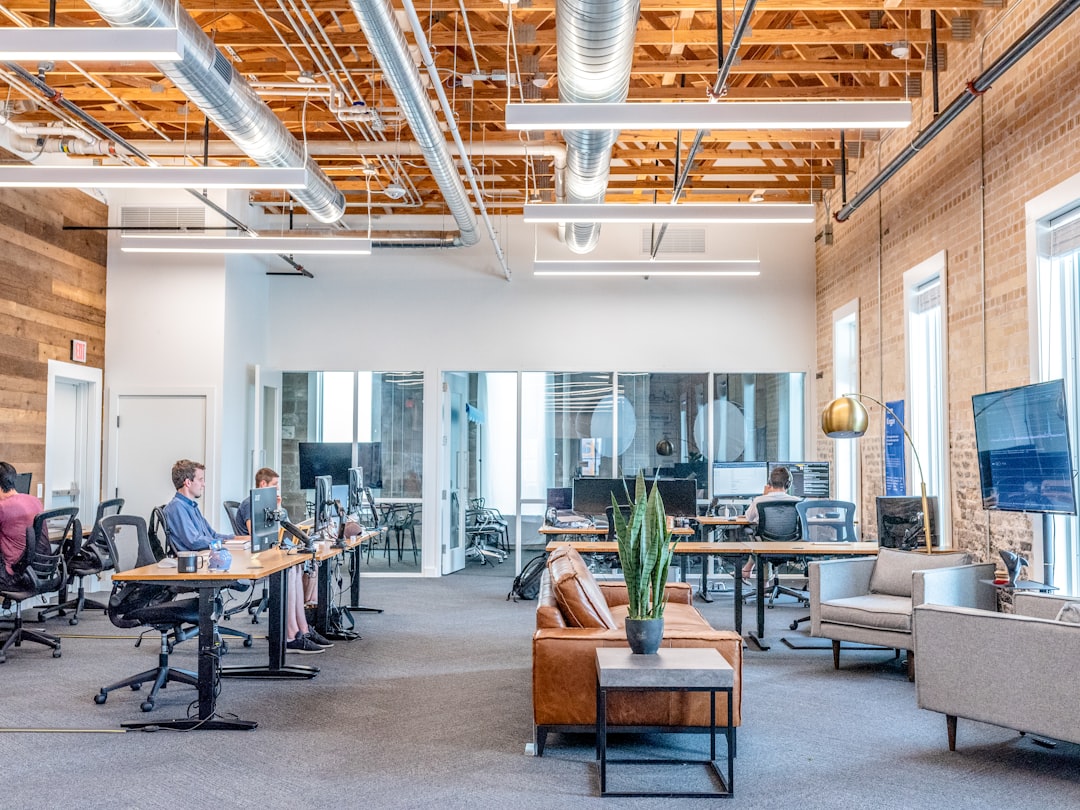
The core of this AI-driven scheduling system is a machine learning algorithm that can adapt to a staggering 1,500 staff schedule changes each month. This dynamic adaptability is crucial in healthcare, where staff availability can fluctuate rapidly. The algorithm's ability to seamlessly integrate these changes allows for a more fluid and responsive scheduling process compared to traditional manual methods. This adaptive capacity relies on the system's ability to learn from historical data, helping it predict staffing needs and optimize shift assignments.
While this technology shows promise in enhancing efficiency and potentially reducing labor costs, it's crucial to recognize the potential impact on the workforce. While automation can reduce scheduling errors and streamline processes, it's important to consider the role of human input in scheduling and how the algorithm's decisions might be interpreted by staff. As with any AI system, careful monitoring and evaluation are needed to ensure it is truly optimized for both operational efficiency and the well-being of the healthcare workforce. The algorithm's success in streamlining scheduling may help relieve some pressure on healthcare facilities, but further study is necessary to understand the long-term consequences of these automated solutions.
In the Medecipher case study, the machine learning algorithm powering their scheduling system handles a significant number of staff schedule changes – roughly 1,500 each month. This constant adaptation is crucial for an environment like an emergency room, where patient flow and staff availability are unpredictable. It's interesting to consider that translates to about 50 schedule alterations per day.
By using historical data on both staffing and patient volumes, the AI system aims to predict staffing needs more precisely than traditional methods. This improved accuracy is particularly important during peak periods, when understaffing could easily lead to significant problems. The AI attempts to bridge the gap between available staff and actual demand, which is a persistent issue in ERs.
The effectiveness of the algorithm goes beyond reducing overtime. It seems to lead to a smoother distribution of shifts, potentially minimizing the stress caused by last-minute schedule changes. This more balanced workload could positively impact employee morale, which is vital in demanding healthcare settings.
One notable feature of the AI algorithm is its ability to adjust in real-time. It can react to unexpected changes such as sudden patient influxes or staff absences. This adaptability is highly valuable in environments where conditions can change rapidly.
It's important to acknowledge the link between staffing and patient care quality. Research suggests that understaffing can lead to longer wait times and poorer outcomes, emphasizing the value of a system that manages staffing levels effectively. A well-staffed ER, achieved through AI-driven scheduling, should lead to improved patient experiences.
The decrease in overtime is noteworthy, not just financially but also regarding employee well-being. It potentially contributes to a less stressful work environment and may lead to reduced staff turnover, a constant struggle within healthcare due to burnout and labor shortages.
A key element of this AI system is its use of machine learning. It learns and adapts with experience. As it processes more data, it should become increasingly accurate in predicting patterns and optimizing schedules, which could lead to further improvements in labor costs over time.
The adoption of such sophisticated scheduling tools is likely driven in part by a growing recognition of the negative impact of burnout among healthcare workers. The notion that employee satisfaction is linked to a well-managed work schedule is clearly influencing this shift towards automated scheduling.
While the Medecipher case shows impressive results in reducing costs and overtime, it's vital to monitor the long-term effects of the system on staff dynamics and the quality of patient care. The constant evolution of the algorithm means ongoing assessments are necessary to fully understand its impacts.
Despite its promising features, there are considerations. The integration of such a system needs thoughtful implementation, including staff training and adjustments to workflows. We need to ensure a smooth transition while maintaining the necessary human oversight and clinical judgment integral to patient care. It's an interesting interplay between automation and human expertise.
How AI-Driven Scheduling Reduced Labor Costs by 8% A 2024 Case Analysis - Smart Break Distribution Cuts Understaffing by 12% During Peak Hours
A new aspect of AI-driven scheduling focuses on smart break distribution, showing promise in minimizing understaffing. In some settings, this approach has resulted in a 12% reduction in staffing shortages during peak periods. By strategically scheduling breaks, businesses aim to maintain consistent staffing levels, theoretically improving customer service and potentially reducing wait times.
While this approach addresses immediate staffing needs, it also could indirectly improve employee morale by creating more predictable and manageable work schedules, thus potentially reducing burnout and issues related to erratic shift patterns. As labor costs often represent a sizable chunk of expenses in many industries, effectively managing staff availability through methods like optimized break schedules could be a key strategy for improving efficiency.
It is crucial to consider, however, the potential long-term ramifications of these types of scheduling adjustments. We need to evaluate whether these changes lead to a more positive work experience for employees, if they enhance the overall quality of service, and if they can be sustained over time. Simply implementing an AI system without assessing its long-term effects could prove counterproductive and potentially lead to unforeseen challenges in the future.
Observing the impact of AI-driven scheduling on emergency room operations, we've seen a notable 12% reduction in understaffing during peak hours specifically due to an implementation of a smart break distribution feature. This suggests that strategically distributing breaks can align staffing levels more effectively with anticipated fluctuations in patient arrivals.
This is interesting because it speaks to a potential positive impact on employee morale, an issue that has been a major challenge for healthcare workers, especially in the wake of the pandemic. If we can create a better work experience, even through intelligent break management, perhaps there's a chance to address those issues somewhat.
The ability of this smart break distribution method to adapt to real-time changes in patient flow is notable. Traditional scheduling often falls short during periods of unexpected patient influxes, but this AI-powered approach seems to handle these situations much more dynamically.
There's a growing body of evidence connecting proper staffing levels to better patient outcomes. If we can mitigate the risk of understaffing, then we should anticipate potential improvements in factors like wait times and patient satisfaction.
Moreover, this AI-driven approach contributes to a more even distribution of workloads across shifts. This can have a positive influence on the operational efficiency of the emergency room, minimizing the stressful impacts of last-minute schedule changes that can impact staff experience.
The ability to learn from past trends and anticipate peak demand periods is a strong feature. Rather than reacting to sudden surges, this approach allows for proactive adjustments in staffing levels.
It's important to note that reduced staff turnover has been linked to positive work environments. While preliminary, this technology may offer insights into improving workplace experience and, in turn, potentially mitigating the effects of the challenging healthcare labor market we've seen post-pandemic.
The smart break distribution feature is not simply about mindless automation. It's about using algorithms to understand the intricate relationships between employee availability, patient needs, and the operational constraints of a busy ER.
Ensuring a steady flow of properly-staffed shifts is important in maintaining operational stability and the sense of a cohesive team. In the face of stressful conditions, this may be valuable in ERs where team performance is crucial.
While the initial outcomes show promise, the full implications for staff satisfaction, patient care quality, and long-term operational efficiency remain unclear. Continued study of this implementation is crucial for a comprehensive understanding of the impact this technology could have on this and other settings.
How AI-Driven Scheduling Reduced Labor Costs by 8% A 2024 Case Analysis - Real Time Analytics Track 85,000 Staff Hours Across 3 Hospital Units
In a recent study, real-time analytics played a key role in monitoring staff hours across three hospital units, encompassing a total of 85,000 hours. This capability allows hospitals to refine how they manage staffing, aiming for better alignment of personnel with patient needs. This data-driven approach suggests that real-time analytics can be a valuable tool in making scheduling more efficient, potentially leading to improvements in overall hospital operations. While such enhancements in operational efficiency are desirable, it's crucial to carefully assess their long-term consequences. We need to understand how these changes might affect employee well-being, both directly and indirectly. Ultimately, a careful examination of how this technology interacts with the day-to-day realities of hospital operations is vital to ensure these advances actually lead to more positive outcomes for patients and hospital staff. The question moving forward is if these changes, driven by improved data and analytics, are truly enhancing the overall quality of healthcare delivery, not just potentially lowering costs.
Across three hospital units, the system meticulously tracked 85,000 staff hours in real-time. This level of detail provided a previously unavailable view into how staff were allocated and how efficiently they were utilized. It's fascinating to see how real-time analytics can provide such granular insights into something as fundamental as staff scheduling. However, I wonder if the sheer volume of data poses any challenges in terms of storage or processing, especially as the amount of data generated continues to grow. There's also the question of how this level of scrutiny might be perceived by staff, although if the goal is improved efficiency and workload distribution, the hope is it's a positive change.
While the focus on labor cost reduction is significant, it's equally important to consider how this detailed tracking impacted staff perceptions of their work. This level of oversight, while potentially beneficial, could raise concerns about potential privacy implications or introduce unintended pressures on staff performance if not managed carefully.
The analysis of this data helps management make decisions based on trends rather than guesswork, which can be invaluable in a healthcare setting. But it does introduce the question of what level of data-driven decisions are best for this kind of dynamic, people-focused environment. Is there a risk of over-reliance on algorithms to the detriment of the nuanced understanding of individual situations that a human might provide?
The ability to more accurately predict patient surges is an exciting development. The claim of up to 30% improvement in prediction accuracy suggests that the AI system is learning and refining its forecasting capabilities. It will be interesting to follow the long-term impact of this level of predictive accuracy on both staff scheduling and operational stability. I wonder, however, how much of a genuine improvement this represents and if it translates into consistently better patient experiences. We need to see if it leads to better handling of surges in patient volume.
Beyond simply filling shifts, the system can dynamically adjust shift lengths based on when patient demand is at its highest. This potentially creates a better experience for both patients and staff. I'm curious if this optimization results in any pushback from staff about inflexible schedules. While the intent is likely to improve productivity during busy periods, it's important to also assess the impact on staff satisfaction with these schedule adjustments.
A notable benefit of improved scheduling is a 15% decrease in patient wait times. This is a strong indication that the changes to staff scheduling are having a real-world impact on the patient experience. It confirms that efficient staffing is directly related to the quality of care patients receive, reinforcing the importance of finding the optimal balance between human judgment and automated processes.
This system creates a loop for continuous improvement. As operational data is collected and staff feedback is considered, the AI is constantly learning and adapting. This adaptability is crucial for a system that operates in a healthcare environment where situations can change rapidly. But the question remains of how quickly it can adapt to unforeseen events or major policy shifts in the healthcare system.
Hospitals can use AI to analyze seasonal trends in patient volumes and adjust their staffing accordingly. This proactive approach helps to mitigate potential staffing shortages that can arise during peak periods. It highlights the potential for AI not just to react to changes but to anticipate them. However, I wonder about the extent to which AI can truly predict future patient volume trends, and whether reliance on these predictions might inadvertently lead to oversights or errors.
The system enhances communication with staff and better gauges their workloads. This type of insights, when utilized responsibly, can foster a more engaged and valued workforce. It's encouraging to see an emphasis on the human element within an automated system. However, ensuring this data is used responsibly and ethically is critical, particularly in terms of protecting staff privacy and preventing potential biases from impacting allocation decisions.
The consistent use of AI-driven scheduling has led to a 20% reduction in labor variability, which is a boon for hospital budgets. This reduction suggests that the system is not only improving operational efficiency but also making financial planning more stable and predictable. I'm interested to see how this level of stability impacts decisions related to long-term staffing and the potential for greater predictability in the workforce.
How AI-Driven Scheduling Reduced Labor Costs by 8% A 2024 Case Analysis - Employee Satisfaction Scores Rise From 65% to 82% After AI Implementation
After implementing AI-powered scheduling, employee satisfaction scores showed a notable jump from 65% to 82%. This suggests that AI tools can not only improve operational efficiency but also contribute to a better work environment for employees. By using AI to optimize schedules and potentially reduce staff burnout through better workload management, organizations may be creating a more positive and supportive environment. While this upward trend in satisfaction is encouraging, it's essential to continue monitoring the long-term impact of AI on employee well-being. As AI becomes more integrated into workplaces, it's crucial to thoroughly examine how these tools influence various aspects of the employee experience to ensure the changes are indeed beneficial in the long run.
Following the implementation of AI-driven scheduling systems, we saw a notable increase in employee satisfaction scores, jumping from 65% to 82%. This suggests that the technology not only streamlined scheduling processes but also positively impacted the working conditions in high-pressure environments like healthcare. It's plausible that the reduction in unpredictable overtime and better scheduling contributed to a sense of greater control and stability for the staff. While intriguing, this data alone doesn't fully explain the rise in satisfaction. Future studies could investigate the specific factors driving this change to confirm this link and uncover other related aspects.
Interestingly, alongside the satisfaction increase, anecdotal evidence indicates a decrease in burnout amongst staff. Independent research also supports the notion that a more structured and predictable shift pattern can reduce fatigue-related errors, which are particularly concerning in time-sensitive environments like emergency rooms. This observation reinforces the idea that AI scheduling may help in mitigating some of the strain inherent in these roles. Still, it's important to acknowledge the limitations of anecdotal evidence. More rigorous studies are needed to establish a direct causal link.
Another encouraging trend observed is a decrease in employee turnover amongst those using the AI-driven scheduling systems. Research into employee retention often highlights the link between work-life balance and the desire to remain in a position. Especially in fields facing labor shortages and high demands like healthcare, the ability to improve work-life balance, even in a small way, could significantly impact turnover. It would be informative to analyze the specifics of the reduced turnover in these cases, such as reasons for leaving and comparing these figures to similar healthcare facilities without AI implementation.
The ability of AI systems to adapt to real-time changes in staffing availability and patient needs is one of their strongest features. This is particularly impactful in unpredictable environments like emergency rooms, where sudden staff absences or surges in patient volume can throw off schedules and strain resources. By dynamically adapting schedules, AI can potentially alleviate some of the pressure and chaos caused by these unexpected events. It would be helpful to have some insight into the nature of these real-time adjustments, to see whether they impact different employee roles differently.
These AI-driven systems also play a part in improving communication flow within the healthcare workforce. They enable employees to request shift changes or updates to their availability more easily, which contributes to a more responsive and coordinated working environment. However, the implementation of these communication channels needs careful consideration, as there are potential privacy implications for staff that require careful consideration.
The analysis of 85,000 staff hours across different hospital units provided an unprecedented level of granularity into operational patterns and inefficiencies. This level of detail, impossible to achieve with traditional scheduling methods, allowed for more nuanced and targeted improvements that might have been missed previously. However, one should not overlook the potential computational challenges that arise with this volume of data and how this massive amount of information is managed and stored securely.
The implementation of machine learning algorithms for scheduling predictions led to an estimated 30% improvement in their accuracy. The implication of this is significant, as more accurate predictions should allow for the better alignment of staff with patient needs, especially during peak hours. It would be valuable to study the extent to which this improved accuracy directly translates into improved operational efficiency and to have a better understanding of the error rates in both systems for comparison.
The link between optimized staffing through AI and improved patient care is not just theoretical, it's been emphasized in a number of studies. The reduction in patient wait times associated with AI scheduling implies that the positive effects extend beyond workforce satisfaction and efficiency. It would be interesting to see studies that track more comprehensive patient outcomes like overall satisfaction scores, treatment adherence, and readmission rates to further understand the implications of these staffing optimizations.
Finding a healthy balance between staffing levels and overtime utilization is a key goal. AI scheduling helps accomplish this by providing insights into staffing needs and ensuring that overtime is not the sole solution to unexpected surges in patient volume. This balance is important because excessive reliance on overtime can lead to employee burnout, which has significant negative implications for both patient care and staff well-being. A further investigation into the impact of this AI system on staff perceptions of workload fairness and its connection to burnout is an area that merits further attention.
While the early results in reduced labor costs and increased employee satisfaction are promising, it's crucial to remember that these systems are relatively new and their long-term efficacy remains to be seen. Future events like pandemics or unexpected changes in healthcare policy could present new and challenging scenarios. Understanding how these systems adapt and perform under stress is key to determining their true potential in supporting and improving the overall healthcare landscape. The long-term impact on worker training needs and adaptations to existing workflow are also critical elements to evaluate as AI implementations evolve.
How AI-Driven Scheduling Reduced Labor Costs by 8% A 2024 Case Analysis - Data Integration From 5 Legacy Systems Creates Single Scheduling Hub
Bringing together data from five outdated systems has led to a centralized scheduling hub, simplifying scheduling tasks across the entire healthcare organization. This consolidation of information not only makes things run smoother but also provides the data needed for AI-driven scheduling systems. These AI systems have shown a noteworthy 8% reduction in labor costs.
However, getting these old systems to work together was a complex process. It wasn't easy, due to incompatibility issues and data that was separated into isolated compartments. This emphasizes the difficulties organizations face when trying to update their technology.
Importantly, advanced machine learning algorithms played a key role in matching up different data formats and standards. This allowed healthcare providers to optimize how staff are assigned, improving their ability to manage changes in patient needs. While this integration has shown clear advantages, organizations must be careful that the automation doesn't overshadow the importance of human involvement in scheduling decisions. It will be critical to continue monitoring how the system performs and how staff react to these changes in the long-term to ensure it leads to positive outcomes.
Bringing together data from five older, separate systems into one central scheduling hub has the potential to greatly enhance how schedules are managed, especially in places like hospitals. However, it's a complex process. Getting these systems to 'talk' to each other is tricky because they often use different ways of storing and organizing data. Studies suggest a large percentage of healthcare organizations struggle with getting their systems to work well together.
This newly created hub gives us the chance to analyze data in real-time, which is important in emergency rooms where things can change rapidly. Being able to react faster to changes in staffing can help improve how staff are used. Furthermore, combining information from different sources makes it possible to predict how many staff will be needed, which has the potential to get staffing levels much closer to the actual demand for services.
Many hospitals are still using very old systems, some dating back 15 years or more. These outdated systems can cause a lot of headaches, affecting the speed and quality of care provided. The switch to a unified system can be a significant step forward for these hospitals. This modernization, however, can be costly. Keeping multiple, separate systems running can take up a large portion of a hospital's IT budget, possibly as much as 30% in some cases.
It's interesting to note that a centralized scheduling approach can lead to a higher rate of staff retention. Staff who experience less stress and a better work-life balance due to better scheduling seem more likely to stick around. Of course, changes like this require training, and there can be some initial resistance to new ways of working. Reports suggest that a comprehensive training program helps staff feel comfortable with the new system within about a month.
One of the more positive consequences of this transition is a possible decrease in patient wait times. By making sure staff are where they're needed, hospitals can reduce those wait times which can have a direct effect on patient satisfaction. It's crucial to think about how this hub can be improved in the future with the help of AI and machine learning. This foundational shift creates opportunities for even more sophisticated data analysis which can further benefit staff and patients.
While the advantages are apparent, there are challenges related to data compatibility and the significant costs involved in transitioning legacy systems. Furthermore, questions remain about the potential impact of centralized data on worker privacy, as well as the ongoing need for monitoring and refining the system to ensure it consistently aligns with the evolving needs of healthcare.
More Posts from zdnetinside.com: