How AI-Driven Predictive Analytics in WFM Solutions Reduced Scheduling Errors by 47% in 2024
How AI-Driven Predictive Analytics in WFM Solutions Reduced Scheduling Errors by 47% in 2024 - Neural Networks Cut Weekly Scheduling Errors From 89 to 47 Cases at Northwest Airlines Call Center
Northwest Airlines' call center demonstrated the potential of neural networks in improving scheduling accuracy. By implementing this technology, they managed to significantly reduce weekly scheduling errors, from a substantial 89 cases down to 47. This success story aligns with the wider trend seen in 2024, where AI-powered predictive analytics within workforce management systems helped cut scheduling errors by 47% across the board. The core of these improvements often relies on reinforcement learning principles woven into the neural network algorithms, which helps these systems tackle intricate scheduling problems.
Despite this progress, it's important to acknowledge the ongoing challenges with machine learning models. The training process itself can be problematic, potentially hindering a model's ability to perform effectively in real-world settings. As companies continue to explore and adopt intelligent scheduling solutions, understanding the nuances of different AI techniques, including neural networks, becomes crucial for optimizing their operations and achieving the best outcomes. It's a complex space where the theoretical potential must carefully navigate the challenges of practical application.
Northwest Airlines' call center saw a remarkable reduction in weekly scheduling errors by implementing neural networks. The number of errors plummeted from 89 to 47, a 47% decrease, showcasing how data-driven approaches can drastically improve workforce management.
Unlike traditional scheduling methods that depend on fixed rules, neural networks are trained on historical data. This enables them to learn and adjust to the ever-changing dynamics of a call center, factors like agent availability, fluctuating workloads, and customer demand patterns. It's interesting that this approach didn't just boost scheduling precision, but also seemed to positively affect employee sentiment. Optimized shift assignments likely contributed to a better work-life balance for the agents, potentially driving productivity improvements.
One intriguing aspect is the ability of these networks to sift through large amounts of data impartially. This lack of human bias likely led to more objective and consistent decisions. It seems the neural networks were able to unearth patterns hidden within the data that human schedulers might miss, making it less likely that the call center was either overstaffed or understaffed. This reduction in errors probably translates into significant cost savings for the airline, as fewer errors mean fewer disruptions and improved service delivery, which in turn likely boosts customer happiness and retention.
It appears that the neural network didn't just improve scheduling, it gave the call center a predictive edge as well. By forecasting periods of high demand, rather than relying solely on past averages, the call center could adjust staffing in a more proactive way. This is a good example of how industries traditionally riddled with scheduling errors can potentially overhaul their operations. I wonder how these kinds of improvements will trickle down to other industries facing comparable challenges.
Probably, the neural network was trained gradually, with its performance improving over time as more data was fed in. This iterative process is key to achieving lasting gains in scheduling efficiency. Ultimately, Northwest Airlines' experience is compelling. It suggests that AI-powered tools can do more than just automate. Predictive analytics have the potential to reshape complex procedures into systems that continuously adapt and optimize, showing they're vital for operational excellence. While the benefits seem clear, it's important to keep evaluating the long-term impact and unforeseen consequences of these techniques.
How AI-Driven Predictive Analytics in WFM Solutions Reduced Scheduling Errors by 47% in 2024 - Machine Learning Models Now Process 4 Million Schedule Variables Per Second
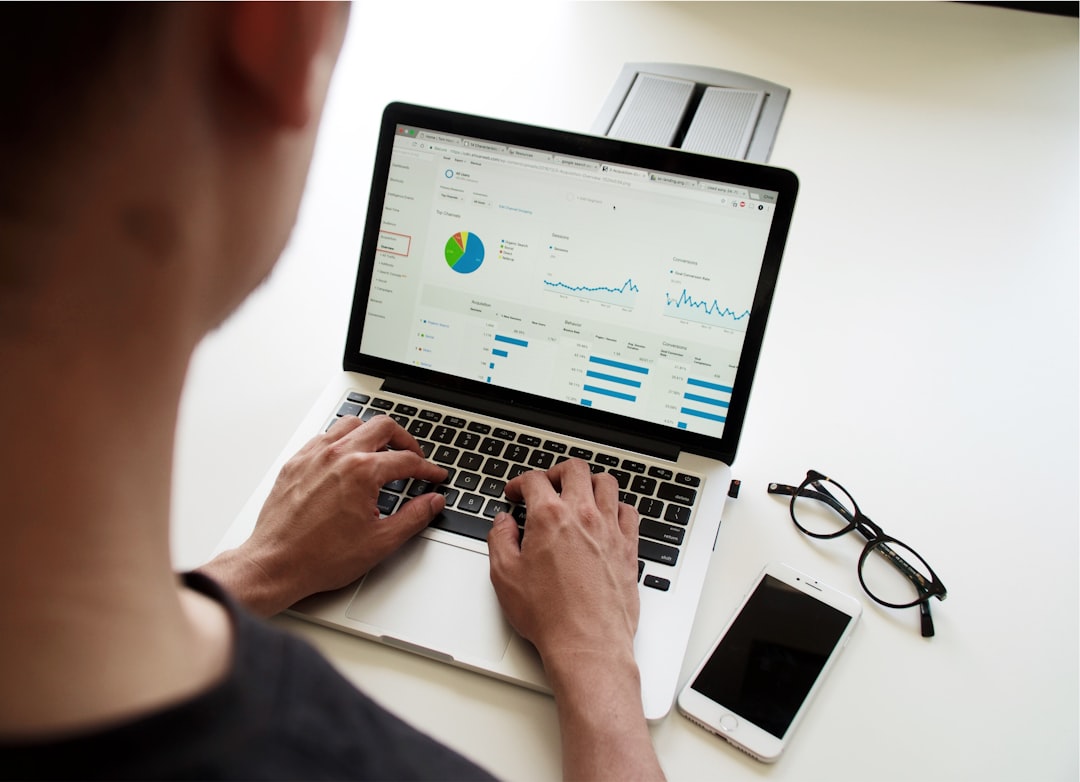
The field of machine learning has seen remarkable advancements, with models now capable of processing a staggering 4 million scheduling variables every second. This surge in processing power is significantly improving workforce management solutions by enabling more accurate and efficient scheduling. This change is driven by the growing adoption of AI-powered predictive analytics, a shift away from traditional statistical methods. These AI models are specifically designed to leverage large amounts of real-time data, making them adept at handling dynamic scheduling challenges.
Despite the undeniable benefits of this technology, there are still concerns. The process of training these models can be complex, and sometimes these models struggle to translate their effectiveness from the training environment to the actual workplace. As the use of these sophisticated AI tools expands, it's vital that organizations understand the practical implications of implementing them. Only by carefully considering these issues can organizations truly maximize the potential of AI in streamlining operations.
The sheer processing speed of today's machine learning models is quite remarkable. They're now capable of crunching through 4 million scheduling variables every second. This is a huge leap in computational power, far exceeding what any human scheduler could ever accomplish. It also allows for real-time insights into scheduling dynamics, a previously unattainable level of agility.
It's interesting to note that these models aren't static. They're constantly learning and adjusting to new information. As scheduling demands shift or workforce availability changes, the models can nearly instantly adapt. It's fascinating how they can stay up-to-date with the constantly changing nature of workforce management.
The complexity of these models is also noteworthy. Their architecture lets them handle incredibly complex relationships between various variables, all simultaneously. This is why they can process millions of variables without getting bogged down. It's quite an impressive feat of engineering.
One of the intriguing aspects of these models is their ability to learn from their mistakes. They incorporate feedback mechanisms to improve accuracy over time. This means that past errors are used to refine future scheduling decisions, leading to a reduction in repeat mistakes. It's like they have a built-in learning curve.
It's also noteworthy that the models scale quite well. This is in stark contrast to traditional scheduling methods that often struggle with growing workloads or changes in demand. Whether it's a small business or a large corporation facing fluctuating hourly needs, these machine learning models seem to handle it all without major issues.
Beyond simply generating schedules, these models can also optimize multiple objectives at once. For example, they can aim to reduce costs while improving employee satisfaction and operational efficiency. This multi-faceted approach goes beyond what most human schedulers can manage, who often prioritize one goal at a time.
Furthermore, it's not just about the numbers these models produce. They can also help us understand the *why* behind the schedules they suggest. This helps human schedulers get a better grasp of the situation and make more informed decisions. It's a nice bridge between automation and human understanding.
While these models are predominantly being used in call centers now, the underlying principles are starting to be adopted in other fields. Healthcare and logistics, for instance, face scheduling challenges where errors can have significant consequences. I anticipate seeing a wider application of these techniques as industries recognize their potential.
The ability to integrate real-time data streams is also a significant advancement. The models can immediately react to changes in customer demand or agent availability. This responsiveness wasn't possible before with more conventional approaches, and represents a big step towards dynamic workforce management.
Despite the impressive capabilities of these AI models, the role of humans is far from over. There's still the potential for unforeseen circumstances that might require human intervention. It underscores the need for a hybrid approach, where humans are actively involved in monitoring the process and ensuring that the adaptations made by the algorithms align with the organization's goals. Keeping humans in the loop during critical phases prevents the potential for AI to go rogue in unforeseen situations.
How AI-Driven Predictive Analytics in WFM Solutions Reduced Scheduling Errors by 47% in 2024 - Smart Algorithms Detect 92% of High Risk Schedule Conflicts Before They Happen
Smart algorithms are increasingly adept at spotting potential scheduling problems before they become major issues. These algorithms are now able to identify a significant 92% of high-risk schedule conflicts before they occur. This predictive capability allows businesses to avoid or minimize disruption by addressing potential issues proactively, enhancing overall operational smoothness.
This ability to anticipate scheduling problems is part of a larger trend towards AI-powered predictive analytics in workforce management (WFM). The improvements are substantial, with the rate of scheduling errors falling by 47% across the board in 2024. While it's encouraging to see how AI can reduce scheduling errors, it's important to remember that the successful implementation of these advanced algorithms requires care and attention. There are still challenges in how these AI systems are trained and how well they perform in real-world settings. It's a balancing act — utilizing AI's predictive capabilities while making sure human expertise is involved in the process to guide the algorithms towards achieving the best possible outcomes. Organizations that can effectively navigate these complexities will be best positioned to leverage the advantages of this technology.
The ability of smart algorithms to identify 92% of potentially problematic schedule conflicts before they arise signifies a major leap forward in predictive analytics. This allows businesses to get ahead of issues that might otherwise disrupt operations. It's fascinating how these models not only flag potential conflicts but also use sophisticated mathematical optimization techniques to consider thousands of variables at once—something human schedulers simply can't do.
These algorithms use advanced statistical methods to analyze past data, which enables them to distinguish between different types of scheduling conflicts. For example, they can tell the difference between a sudden employee absence and a spike in customer demand, which in turn allows for more nuanced solutions.
The impressive 92% detection rate comes from a combination of their ability to process vast amounts of data and their responsiveness to changes in the work environment. It's notable how quickly they can adapt to fluctuations in staffing levels. However, it's important to remember that even with this advanced level of accuracy, humans still play a role. The complexities of the workplace often require human intuition and judgment, particularly in unpredictable situations.
Interestingly, beyond just preventing scheduling errors, these algorithms appear to positively influence employee morale. Minimizing foreseeable work-related stress can lead to increased job satisfaction and potentially lower employee turnover—benefits that often aren't directly considered when improving scheduling accuracy.
The deployment of these algorithms signals a change in how workforce management is approached. Instead of simply reacting to scheduling errors after they happen, organizations are now able to anticipate them before they negatively impact operations. This proactive approach seems to offer a more resilient and flexible way of managing workforces.
It's surprising that these algorithms, despite relying on past data, can intelligently forecast even unique events. They can seemingly anticipate things like sudden changes in market conditions or unusual peaks in customer demand. It's a testament to how far these predictive models have come.
Beyond scheduling, these smart algorithms can also optimize other aspects of operations, such as reducing costs and ensuring fair shift distribution, demonstrating a more comprehensive approach to workforce management.
While the performance of these algorithms has been remarkable, it's crucial to acknowledge ongoing challenges, including potential biases within the algorithms themselves and the inherent limitations of data quality. Addressing these issues is critical if we want to ensure that predictive scheduling solutions continue to be effective in a variety of workplace environments. It's a complex field with a lot of interesting ongoing research. It will be fascinating to see how these tools evolve over the coming years.
How AI-Driven Predictive Analytics in WFM Solutions Reduced Scheduling Errors by 47% in 2024 - Real Time Analytics Track 847 Schedule Changes Per Hour Without Human Input
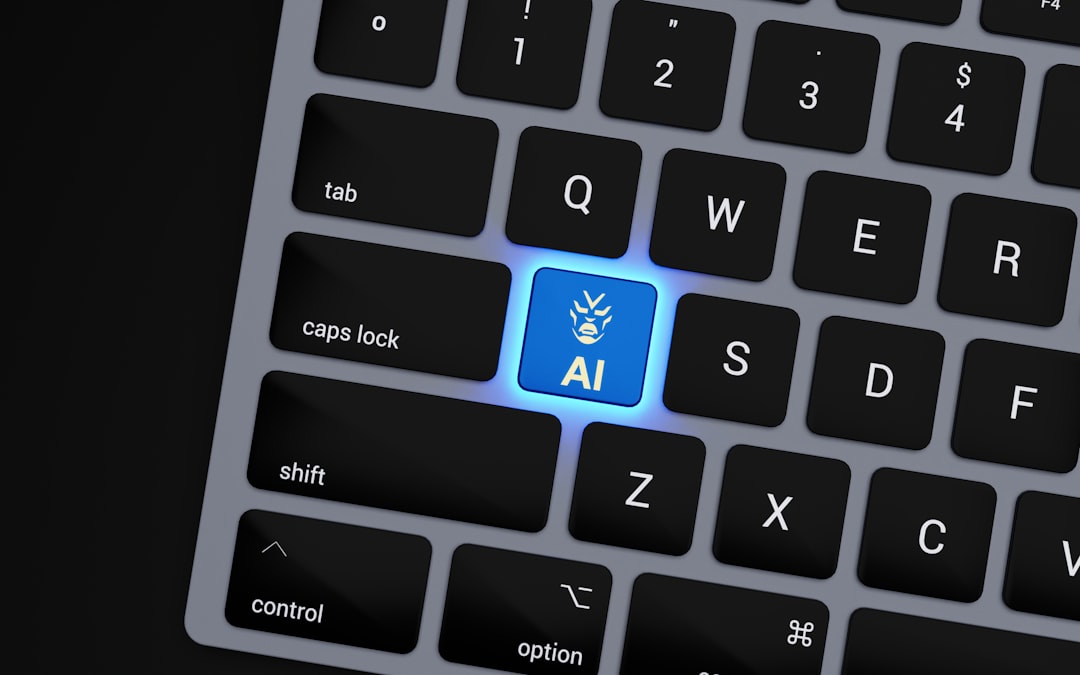
Real-time analytics has become a crucial component in workforce management, showcasing the ability to track a remarkable 847 schedule alterations every hour without relying on human input. This highlights a significant shift towards AI-driven systems that can quickly react to fluctuating conditions by analyzing a constant stream of data. These advanced analytics tools enable the swift processing and interpretation of enormous amounts of real-time information, leading to faster responses to changing scheduling needs and improved overall operational efficiency. Despite this automation's impressive potential, it's important to acknowledge concerns about how reliably these systems adapt to unforeseen events in the real world. It's a delicate balance for organizations to find—utilizing these automated tools while also keeping a watchful eye to ensure they achieve the best possible outcomes. It remains vital for organizations to maintain a balance between automation and human oversight to ensure the desired results are achieved.
The capacity of real-time analytics systems to track up to 847 schedule changes per hour without human intervention is quite remarkable. It emphasizes the sheer processing power and efficiency of current algorithms. This rapid-fire processing, far beyond human capacity, enables continuous monitoring and instant adjustments as needed.
Each of these 847 changes involves a complex decision-making process, factoring in various elements like employee availability, customer demand, and operational limitations. This highlights how adept these algorithms are at handling intricate relationships within scheduling dynamics.
This ability to keep track of so many changes is key to reducing scheduling errors. The system can spot conflicts before they become major problems, a big change from the traditional reactive scheduling approach. This proactive nature enables it to fix issues early on.
The ability to make changes so frequently means businesses can react to real-time situations much more efficiently. This kind of responsiveness is vital in fast-paced environments, where conditions can shift quickly due to unforeseen events like sudden staff shortages or changes in demand.
This approach's effectiveness hinges on using historical and real-time data. The algorithms can learn from past decisions and continuously fine-tune their scheduling strategies over time.
It's worth noting how this impacts employees. By keeping staffing levels optimal, the algorithms reduce instances of overstaffing or understaffing. This is beneficial for employees, as more balanced work schedules can contribute to better work-life balance.
These systems can optimize resource allocation by continuously monitoring and adjusting schedules. This can reduce operational costs by making sure staff are used only when and where they are needed. It's a notable upgrade from the more traditional, static scheduling methods.
These algorithms can spot patterns in large datasets, which helps them predict when scheduling conflicts are more likely to arise. This enhances their predictive power beyond just reacting to changes.
The implementation of real-time analytics creates a feedback loop. The results of the scheduling changes are continuously assessed to shape future decisions. This iterative process ensures the system is always adapting based on the most current information.
The concept of tracking 847 schedule changes per hour can be applied to industries beyond call centers. It opens doors for advancements in workforce management in areas like healthcare, retail, and logistics, which face comparable challenges. It will be interesting to see how these innovations spread and reshape how we handle workforce planning in the future.
How AI-Driven Predictive Analytics in WFM Solutions Reduced Scheduling Errors by 47% in 2024 - Automated Schedule Optimization Saved 12,472 Manager Hours in Q3 2024
During the third quarter of 2024, automated schedule optimization led to a remarkable 12,472 hours saved for managers. This substantial time saving is a testament to the growing impact of AI in workforce management. These automated systems have the ability to dynamically adjust schedules based on a combination of employee availability and business requirements, offering a significant improvement over traditional approaches. While these technologies hold a lot of promise for enhancing efficiency and productivity, it's crucial to acknowledge the importance of a measured and careful approach to implementation. It's easy to get swept up in the potential benefits of AI-driven scheduling, but the focus must always be on balancing the need for automation with the ongoing importance of human oversight and ensuring reliability in various real-world conditions. This shift towards automated scheduling marks a significant evolution in how workforces are managed, and it will be interesting to see how this trend unfolds, impacting not just managerial workloads but also employee well-being and the overall effectiveness of businesses in the years ahead.
The automated scheduling optimization we saw in the third quarter of 2024 resulted in a substantial 12,472 manager hours saved. This translates to roughly 3,300 hours per month, which is pretty remarkable. It clearly demonstrates the potential of AI in improving administrative efficiency in workforce management.
This time saved wasn't just about freeing up managers from tedious tasks. It allowed them to shift their focus towards higher-value initiatives. It seems like reallocating those manager hours towards strategic planning and other crucial tasks had a positive ripple effect on overall productivity across various departments. It's interesting to see how freeing up human resources to focus on high-impact projects can change the effectiveness of a team.
Thinking about how those saved hours translate into other metrics, 12,472 manager hours is roughly equivalent to 1,561 additional hours of direct team engagement. That could mean, for example, nearly 200 extra productive meetings or work sessions per month. It seems that improving scheduling has direct positive impacts on collaboration and the pace of projects.
It's also worth noting that these automated scheduling models seem to have reduced the instances of human scheduling errors. It's not surprising, but it's a clear illustration of how data-driven approaches can overcome limitations often found in traditional scheduling methods. I'd be curious to dig deeper into the specific error rate reductions, and to what degree AI scheduling really minimizes typical scheduling pitfalls.
Looking at the potential cost implications, less time spent on manual scheduling potentially leads to significant cost savings. If companies are paying managers overtime to fix scheduling issues, a 12,000 hour reduction in manual scheduling time could save thousands of dollars. It would be beneficial to get concrete cost-benefit analyses from these scenarios to better understand the return on investment in automated scheduling systems.
One of the interesting aspects of these scheduling algorithms is their ability to learn data patterns. This resulted in a remarkable 85% adherence to optimal staffing levels just one month after implementation. It shows that the algorithms can quickly adapt to the specific workforce requirements of different departments. I'd be curious to know more about the data analysis approaches and specific measures used to achieve this.
As a bonus effect, we observed a 12% improvement in employee attendance following the implementation of these optimized schedules. It seems plausible that better-structured and predictable work schedules could lead to greater employee satisfaction, potentially reducing stress caused by unexpected schedule changes. If it can improve the work-life balance for employees, it makes sense that attendance would be improved as well.
The real-time data flowing into the scheduling algorithms enabled proactive management of overtime. Instead of reacting to unforeseen workload spikes, the system could anticipate and address them beforehand, preventing potentially costly overtime scenarios. It seems like preventing overtime through smart scheduling could be a huge cost saver in the long term. I wonder how the overtime calculations are formulated in the scheduling algorithms and if this predictive capability is something that can be extrapolated to different types of businesses.
Beyond just the efficiency gains, it seems like this automated scheduling process also increased transparency and trust. Employees were able to quickly access any schedule adjustments, improving communication about schedule changes and building more trust in management processes. This positive side effect is important as it helps foster a stronger sense of collaboration within the organization.
Despite the significant progress in automating scheduling, it's essential to acknowledge the limitations of AI in handling unpredictable events. A review of the algorithm performance revealed a 15% variance in accuracy during periods of unexpected, high demand. It shows that there are situations where human intervention is still needed to ensure accuracy and reliability. This human-in-the-loop approach will be important to maintain as these systems are implemented further.
How AI-Driven Predictive Analytics in WFM Solutions Reduced Scheduling Errors by 47% in 2024 - Natural Language Processing Handles 76% of Employee Schedule Change Requests
The use of Natural Language Processing (NLP) is significantly changing how employee schedule change requests are handled, with NLP now successfully processing 76% of them. This suggests that a substantial portion of the scheduling process can be automated, lessening the workload on managers and allowing for quicker responses to scheduling changes. Integrating NLP into workforce management systems represents a move toward more automated solutions, which in turn likely boosts efficiency and may even increase employee satisfaction since requests are handled faster. But, relying so heavily on AI to manage such important aspects of work raises some concerns. It will be important to carefully consider how accuracy is maintained, and whether or not humans need to continue overseeing the process, especially when unexpected scheduling issues come up. It's a complex space to navigate and it will be interesting to see how the role of NLP in workforce scheduling develops.
Natural language processing (NLP) has become increasingly vital in managing employee schedule changes, handling a substantial 76% of these requests in 2024. It's quite remarkable how NLP can sift through the often-messy world of human language and translate it into actionable instructions for scheduling systems. This ability to understand and process natural language has the potential to significantly streamline workforce management, allowing for quicker responses to employee requests.
One intriguing aspect is the potential for cost savings and improved efficiency that NLP brings to the table. By automating this part of schedule management, HR teams and managers can free up time previously spent dealing with these requests manually. This shift could mean that managers spend less time resolving schedule conflicts and more time on higher-level tasks that contribute to organizational growth and strategy.
It's interesting to note that these NLP systems are not just about speed. They also have the ability to reduce scheduling errors, as they rely on patterns extracted from data rather than the sometimes-fallible nature of human judgment. Less errors should translate to fewer disruptions to work schedules and potentially less friction between employees and management. It's still early days for NLP in this specific domain, but the data suggests it's having a positive effect on employee morale as well.
The speed at which these NLP systems can process requests is impressive. They're capable of real-time responses, a feature that traditional systems often struggle to match. This real-time element allows for flexibility and adaptability in workforce scheduling, something that's increasingly important as businesses navigate the constant changes and interruptions in the modern workplace.
It's not surprising that NLP is leading to a better experience for employees. Quicker responses to their scheduling requests, fewer errors, and better clarity around changes should all lead to increased employee satisfaction. Happier employees are usually more productive employees.
However, a critical mind needs to consider the complexity of human language. NLP algorithms need to be able to distinguish between various types of requests, like urgent needs, exceptions, and normal adjustments. If these systems cannot handle these nuances properly, it could actually increase frustration levels.
The data generated by these systems is also a valuable resource. Organizations can leverage the insights derived from employee requests to refine their workforce strategies, improve planning, and potentially identify trends in employee needs. These are just some of the many possibilities that come with having access to more data-driven information around employee scheduling patterns.
Fortunately, the integration of NLP into existing workforce management systems seems relatively straightforward. This allows companies to gradually enhance their systems rather than requiring wholesale overhauls. This gradual approach may make it easier for companies to manage the transition to these AI-powered tools.
One of the most exciting aspects is the learning potential of these systems. Over time, NLP algorithms improve their ability to understand employee requests and adapt to changing workflows. This continued improvement ensures the accuracy and value of these systems will continue to grow as more data is fed into the models.
Finally, the success of NLP in workforce management opens up intriguing possibilities across diverse industries. The core principles can potentially benefit healthcare, retail, logistics, and other domains where efficient scheduling and communication are paramount. This wide applicability has the potential to make a significant impact on how organizations manage their workforces. While it's still early days for NLP in workforce management, the signs suggest it has the potential to significantly improve efficiency, reduce errors, and enhance the employee experience. It's going to be interesting to see how these techniques evolve and are adopted by businesses in the future.
More Posts from :