Emerging LADDER Framework Revolutionizing Attack Pattern Extraction in Threat Intelligence
Emerging LADDER Framework Revolutionizing Attack Pattern Extraction in Threat Intelligence - LADDER Framework Automates Attack Pattern Extraction from CTI Reports
The LADDER framework introduces a novel approach to extracting attack patterns from the deluge of Cyber Threat Intelligence (CTI) reports. It tackles the challenge of automatically identifying the different stages of attacks, whether targeting Android devices or enterprise networks. This automation is crucial because it allows for a more in-depth understanding of attack methodologies by connecting these extracted patterns to the widely-used MITRE ATT&CK framework. This mapping capability is vital in the current threat environment where relying solely on traditional indicators, like specific IP addresses or domains, is becoming increasingly inadequate. By focusing on understanding how attacks unfold, rather than just the surface-level indicators, LADDER strives to offer a more valuable lens for long-term cybersecurity planning. The efficiency gained through automation enables faster processing and analysis of CTI data, directly leading to quicker and more effective responses to potential threats. Ultimately, LADDER’s contribution lies in its ability to help security professionals develop a more comprehensive understanding of the threat landscape and bolster proactive defense strategies.
LADDER, a framework built around the concept of knowledge extraction, is designed to automatically pull out attack patterns described in cyber threat intelligence (CTI) reports, handling a large volume of them. It meticulously captures attack phases, working across Android and corporate network environments. Interestingly, LADDER translates these extracted attack patterns into a format compatible with the MITRE ATT&CK framework, offering a clearer picture of how cyberattacks are executed.
This automation helps security folks quickly pinpoint attack routes, leading to better preparation for known and emerging threats. Currently, a lot of threat intelligence efforts revolve around known indicators, like specific IP addresses or domains. However, as cyberattacks get more sophisticated, these traditional methods might not be enough. LADDER counters this by focusing on more meaningful clues—attack patterns—to give a stronger foundation for long-term cybersecurity strategies.
LADDER's development was prompted by the hurdles of manually extracting attack patterns from the massive amounts of threat information available. LADDER automates this process, allowing for quicker analysis of reports, resulting in faster reactions to potential dangers. Its value has been recognized within the cybersecurity community, gaining acceptance at the RAID 2023 conference.
Furthermore, the LADDER project is openly available to developers and researchers via platforms like GitHub, encouraging collaboration and continuous improvement. While this offers a degree of transparency and wider applicability, it also presents a challenge in maintaining quality control and ensuring the framework continues to evolve in a way that meets the demands of a dynamic threat landscape.
Emerging LADDER Framework Revolutionizing Attack Pattern Extraction in Threat Intelligence - Mapping Extracted Patterns to MITRE ATT&CK Framework
Connecting the attack patterns automatically extracted by the LADDER framework to the MITRE ATT&CK framework is a key aspect of its contribution to threat intelligence. Essentially, LADDER takes the automatically discovered attack phases and aligns them with the well-established MITRE framework's tactics and techniques. This structured approach helps analysts understand the adversary's methods in a more organized way, which is important for building proactive defenses. The ability to quickly pinpoint attack strategies, instead of just focusing on basic indicators like IP addresses, becomes a more powerful tool in today's sophisticated cyber threat environment.
The alignment with MITRE ATT&CK offers a broader context for attack patterns, facilitating a more comprehensive understanding of threat methodologies. It helps bridge the gap between identifying attack phases and understanding how those phases fit into the wider landscape of cyberattacks. This is particularly useful as the threat landscape evolves, demanding a more adaptable and insightful approach to cybersecurity planning and response. Through this structured mapping, LADDER helps move beyond reactive responses to a more proactive, holistic understanding of threats and risk. It's about building a knowledge base that goes beyond just surface-level indicators, towards a deeper understanding of the 'why' and 'how' of cyberattacks. This is vital for developing and refining defensive strategies that can anticipate and mitigate the ever-changing threats. While there are inherent challenges with any automated system, such as the need to continually adapt to emerging threat trends, the ability to create such a comprehensive view of threats through this approach is a noteworthy development in the field of threat intelligence.
The MITRE ATT&CK framework serves as a valuable resource, constantly evolving with new observations of real-world cyberattacks. It covers a broad range of techniques, currently exceeding 250, which reflects the ever-changing nature of cyber threats and how attackers operate.
By relating the extracted attack patterns to MITRE ATT&CK, organizations can break down intricate cyberattacks into digestible chunks. This enhanced understanding helps them to respond to threats more decisively and efficiently.
Not only does LADDER automate the extraction of attack patterns, but it also classifies each technique according to the MITRE ATT&CK framework's tactics and operational elements. This categorization is beneficial because it helps security professionals concentrate on the most important aspects of the threat.
The meticulous mapping that LADDER automates is really helpful for incident response teams. They can directly align observed attack patterns with known attack paths, significantly accelerating the process of spotting potential security breaches.
One notable aspect of LADDER is its ability to seamlessly integrate with other security tools and platforms. This enhances its overall usefulness within a broader cybersecurity infrastructure, increasing flexibility and practicality.
Mapping to MITRE ATT&CK emphasizes the significance of gaining a complete understanding of the entire attack lifecycle. Instead of simply reacting to isolated indicators, organizations can develop more effective strategic cybersecurity plans.
The capacity for LADDER to swiftly interpret CTI reports is key. It potentially shortens the response time between threat identification and action, leading to potentially less severe consequences from intrusions or data breaches.
With LADDER, security teams might see an improvement in threat hunting abilities. This comes from its unique capacity to link extracted attack patterns with potential existing defenses.
The sophistication of many cyberattacks often creates knowledge gaps within organizations. However, by mapping extracted patterns to a widely recognized standard like MITRE ATT&CK, LADDER helps make threat intelligence easier to grasp for a wider audience.
Building on MITRE ATT&CK, LADDER introduces a novel, automated method to deal with the scalability issues that threat intelligence teams face when working with large volumes of data. This automated approach could represent a significant shift in how organizations manage and analyze threat information in the future.
Emerging LADDER Framework Revolutionizing Attack Pattern Extraction in Threat Intelligence - Enhancing Long-term Threat Analysis Beyond Traditional IoCs
The ever-changing nature of cyber threats necessitates a move beyond traditional Indicators of Compromise (IoCs) to a more comprehensive approach to threat analysis. The LADDER framework tackles this need by offering a structured way to automatically identify and extract attack patterns from the flood of unstructured Cyber Threat Intelligence (CTI) reports. By connecting these patterns to the widely-used MITRE ATT&CK framework, LADDER improves not only the understanding of the various stages of an attack, but also enables security teams to be more prepared to defend against attacks. This emphasis on understanding the broader context of cyberattacks, rather than just surface-level indicators, is crucial for building stronger, long-term cybersecurity defenses against both known and emerging threats within increasingly intricate threat environments. The automated nature of LADDER also helps solve issues related to handling vast amounts of threat information, and ultimately improves the value and relevance of threat intelligence for lasting cybersecurity strategies. While it remains to be seen if LADDER will truly revolutionize threat analysis, the concept of focusing on attack patterns holds significant promise in a field increasingly reliant on rapid threat identification and response.
Traditional Indicators of Compromise (IoCs) can struggle to adapt to the constantly evolving landscape of cyberattacks, making them a somewhat unreliable foundation for cybersecurity strategies. The LADDER framework, in contrast, aims to move beyond these limitations by focusing on the broader picture of attack patterns, which arguably provides a more robust and adaptable approach to defending against threats.
The MITRE ATT&CK framework offers a comprehensive catalog of over 250 attack techniques, reflecting the increasingly diverse and complex nature of modern cyber threats. By linking LADDER's extracted attack patterns to this framework, organizations can gain a more thorough and contextualized understanding of attacker behavior and methodologies.
LADDER's ability to automate the linking of extracted attack patterns with the MITRE ATT&CK framework is quite noteworthy. This automation not only speeds up the process of threat detection but also significantly improves incident response capabilities by offering actionable intelligence directly tied to known attack strategies.
One intriguing facet of LADDER's design is its versatility. It can analyze CTI data from various environments, ranging from mobile devices to corporate networks. This broad applicability enables the aggregation and analysis of attack patterns across different contexts, providing a more complete understanding of potential threats.
The automation of attack pattern extraction, as implemented in LADDER, allows security teams to reallocate their resources more effectively. Instead of focusing on manual data gathering tasks, they can focus more on strategic threat analysis and implementing proactive defense strategies.
Beyond just refining extraction methods, LADDER has the potential to enhance knowledge sharing within organizations. By adopting a standardized approach to representing attack patterns, it simplifies communication and collaboration between technical teams and other stakeholders, improving understanding and response efficiency.
A critical point to consider with LADDER is the importance of maintaining quality control. An automated system, while incredibly efficient, might miss certain subtle threat indicators that a human analyst would likely pick up on. This suggests that combining automated approaches like LADDER with human expertise could be the optimal path forward for future threat intelligence initiatives.
The LADDER framework cultivates a more proactive cybersecurity posture. Rather than simply reacting to attacks after they've happened, it facilitates predicting potential attack vectors based on historical patterns. This shift towards predictive analysis is a significant advancement in how we think about cybersecurity.
By dissecting attack phases into standardized patterns mapped against the MITRE ATT&CK framework, LADDER supports more effective threat hunting initiatives. The ability to identify potential threats proactively can considerably reduce the time a malicious actor remains undetected on a system (dwell time), minimizing the damage they can inflict.
The flexibility built into the LADDER framework allows it to adapt to newly emerging attack tactics without requiring major overhauls. This adaptable nature ensures its continued relevance in the face of the dynamic and ever-changing world of cyber threats, making it a promising tool for long-term cybersecurity strategies.
Emerging LADDER Framework Revolutionizing Attack Pattern Extraction in Threat Intelligence - Application in Android and Enterprise Network Environments
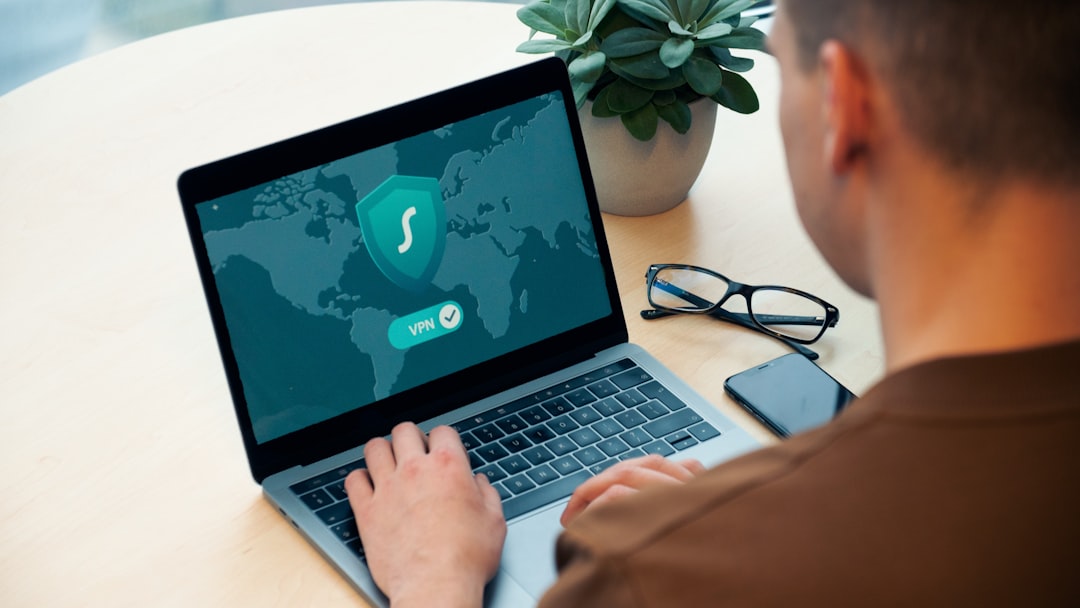
The LADDER framework's application in Android and enterprise network environments is particularly relevant due to the increasing complexity of cyberattacks in these areas. By automatically extracting attack patterns from threat intelligence reports, LADDER helps to bridge the gap between raw information and actionable insights. Characterizing attacks across their various stages and aligning them with the MITRE ATT&CK framework provides security professionals with a clearer picture of attack techniques and tactics. This capability is crucial for enhancing proactive defense measures and improving response times to both known and emerging threats. Essentially, LADDER can empower organizations to be better prepared for a wider range of attack scenarios. However, because the framework is automated, it's important to remember that it might miss some subtle cues that a human analyst would typically pick up on. As the sophistication of cyberattacks continues to increase, integrating tools like LADDER into security operations could become vital for organizations seeking to maintain a strong security posture in the face of evolving threat environments.
The LADDER framework's ability to quickly sift through a vast number of CTI reports, a task that would take human analysts significantly longer, is noteworthy. It allows security teams to shift from responding to threats after they've occurred to proactively implementing defensive measures. Given the widespread use of Android, particularly in enterprise environments, LADDER's focus on analyzing attack patterns specific to the Android platform is crucial for understanding the growing threat landscape within mobile ecosystems. Instead of relying solely on indicators of compromise (IoCs) which can change frequently, extracted attack patterns offer a more enduring and stable representation of how attackers operate, allowing security analysts to more readily identify and connect various attack methods.
As enterprise networks have expanded, the number of potential attack vectors has grown exponentially. LADDER tackles the challenge of analyzing an increasing volume of threat intelligence by employing an automated approach to threat analysis, ensuring that security efforts can keep pace with the expansion of networks. The flexibility of LADDER's mapping approach is critical, allowing it to adapt and adjust its techniques as new attack methods are documented in CTI reports, thereby enabling organizations to stay ahead of emerging threat trends. The design of LADDER considers integration with existing security tools, which significantly improves the overall effectiveness of a security setup by allowing seamless collaboration across different security solutions within an organization.
LADDER's architecture is designed to analyze various environments – cloud, mobile, on-premises – which helps provide a comprehensive view of potential threats across diverse infrastructure. A clear understanding of attack patterns gained through LADDER can substantially impact decision-making in cybersecurity, assisting organizations with the efficient allocation of resources in anticipation of specific threats. While LADDER's strengths are significant, relying solely on automated pattern extraction might inadvertently overlook subtle threat indicators that could be crucial for an organization's security. This reinforces the importance of a hybrid approach, integrating both automated systems like LADDER with the expertise of human analysts.
The open-source nature of LADDER is conducive to a broad collaboration amongst cybersecurity professionals, fostering an environment of innovation and allowing the framework to evolve rapidly in response to new and unforeseen cyber threats. This constant refinement and improvement is essential to ensure its continued effectiveness in a constantly changing threat landscape.
Emerging LADDER Framework Revolutionizing Attack Pattern Extraction in Threat Intelligence - Training Models to Detect Similar Attack Patterns in New Reports
Training models within the LADDER framework to recognize similar attack patterns in new threat intelligence reports is a crucial step towards proactive cybersecurity. The ability to identify recurring attack phases, even when presented in novel ways, helps security professionals gain a better grasp of threat actor behaviors. This type of pattern recognition can be achieved using machine learning techniques that learn from the vast volume of attack patterns automatically extracted and mapped by LADDER to the MITRE ATT&CK framework.
However, training these models effectively presents several challenges. One challenge is the need to address the inherent variability in how attacks are described across different CTI reports. Attackers constantly refine their methods, and slight variations in wording and reporting styles can make it difficult for models to recognize similar underlying patterns. Another issue is the need to continually update and refine the training data to adapt to the evolving threat landscape. Cybersecurity is a dynamic field, and models must be regularly retrained to incorporate new attack techniques and tactics.
Despite these challenges, the potential benefits are substantial. Models that can identify similar attack patterns across new threat reports can help automate the process of threat hunting, leading to a quicker response to emerging threats. By linking new reports to established attack patterns, the framework helps bridge the gap between understanding the "what" of an attack and its context within a wider framework like MITRE ATT&CK. This contextualization facilitates a deeper understanding of adversary tactics and strengthens the basis for preventative measures.
While there's a promise for progress in leveraging machine learning to enhance threat analysis, it's important to remember that this is an evolving area. The effectiveness of these models will largely depend on the quality and diversity of training data and ongoing maintenance and refinement efforts. In the future, further research and development in areas like natural language processing and advanced machine learning techniques will likely continue to refine these approaches to attack pattern recognition, driving progress in the field of threat intelligence.
The LADDER framework employs machine learning, enabling it to continually refine its ability to spot attack patterns. This adaptive learning aspect helps it stay effective even as attackers come up with new methods, without requiring constant major updates. Being automated, LADDER can examine newly published threat intelligence reports immediately. This real-time analysis is crucial for staying ahead of the constantly evolving cyber threat landscape, enabling a faster response before potential damage is done.
Furthermore, LADDER's structure allows it to analyze various environments, including cloud systems, mobile devices, and existing company networks. This capability is crucial for businesses with mixed infrastructure setups. Early research indicates that organizations using LADDER for threat analysis have seen a decrease in the time it takes to recognize and respond to cyberattacks, showing its potential to improve operational efficiency.
While designed for integration, LADDER's automated nature can sometimes clash with manual security processes. This could potentially create gaps in threat detection if not properly managed. However, LADDER offers a way for users to establish their own thresholds for threat categorization and response speed. This feature lets security teams prioritize responses based on their own risk assessments and the resources available.
The framework provides not only current attack patterns, but also places them within the context of the MITRE ATT&CK framework, which allows analysts to see how various cyber threats have evolved over time. This historical context is a benefit. This structured output facilitates more effective threat hunting by providing categorized insights into potential attack pathways. As a result, security teams can proactively anticipate and plan against threats instead of just reacting to them.
While the emphasis is on automation, LADDER includes a backup process that involves human analysts when there's uncertainty in the threat detections. This balances machine efficiency with the human ability to make judgements based on intuition and experience. While LADDER offers several advantages, one issue to be aware of is scalability. As the amount of data LADDER processes grows, ensuring efficiency without sacrificing accuracy in threat detection will require continued refinement.
Emerging LADDER Framework Revolutionizing Attack Pattern Extraction in Threat Intelligence - Facilitating Data Fusion for Improved Threat Intelligence Extraction
In the evolving landscape of cybersecurity, effectively combining data from various sources to extract meaningful threat intelligence is becoming increasingly vital. The LADDER framework addresses this by incorporating a method that extracts key relationships and components from unstructured data sources related to cybersecurity. This is achieved through a process that identifies and links specific elements of cybersecurity events, essentially creating "triples" representing relationships. To further improve this process, the framework uses a refined approach to compare and combine entities from different sources, recognizing similarities even with slight variations in how they are described. This technique is particularly important for threat intelligence as information is often spread across diverse reports, each with its own terminology and formatting. By integrating data in this way, the LADDER framework assists in building a comprehensive network of information about cybersecurity threats, known as a Cybersecurity Knowledge Graph. This interconnected view of threats aids in a deeper understanding of how cyberattacks unfold, ultimately improving the effectiveness of defense strategies in the face of ever-more sophisticated attacks. This capability becomes increasingly important as the nature of cyberattacks evolves and organizations need to adapt to address these new challenges.
The LADDER framework promotes data fusion across various stages of threat intelligence processing, extending its reach beyond just the technical aspects. This integration helps to weave together attack patterns from disparate environments, whether it's Android devices, enterprise networks, or cloud infrastructure.
By associating extracted attack patterns with the MITRE ATT&CK framework, LADDER introduces a significant shift in threat analysis. It pushes beyond merely detecting threats, aiming to provide a contextual understanding of the adversary's plans and goals. This shift is valuable because it helps security researchers gain a deeper understanding of the "why" behind the attacks.
The framework can rapidly process newly released threat intelligence reports, potentially shortening the timeframe between a threat surfacing and a response. This is key in today's threat environment, where quick reaction times are essential.
LADDER utilizes machine learning to refine its ability to identify attack patterns. However, training these models requires continual updates to the training data, which is a key consideration. Attackers are always refining their methods, and the language and descriptions they use can change frequently, potentially causing challenges for the machine learning models. This is why continually refining the models and ensuring that they're trained on current data is critical.
One of the core challenges that LADDER attempts to tackle is the variation in how threats are described across different threat intelligence reports. This issue necessitates incorporating robust natural language processing (NLP) components, which allows the system to pick up on subtle variations in wording and reporting styles, while still identifying the core attack pattern.
While the framework champions automation, it acknowledges the importance of human insight in the threat assessment process. A balanced approach is needed, where the strengths of human analysts are combined with automated processes to deliver the most effective outcome.
Analyzing attack patterns across multiple environments offers a more holistic view of the threat landscape. This multi-faceted approach can help organizations anticipate attacks that span multiple platforms and attack vectors.
LADDER accelerates incident response procedures by presenting a structured view of threat activity that links new attacks to established attack patterns. This streamlining of threat hunting contributes to increased overall security effectiveness.
The collaborative aspect of LADDER is facilitated by its open-source nature. This makes it easier for a broader community of security engineers and researchers to contribute to the framework and iterate upon it quickly to respond to emerging threat scenarios.
LADDER helps break down complex cyber incidents into more manageable parts by organizing attack phases in accordance with the MITRE ATT&CK framework. This makes it easier for security teams to approach cyber threats strategically rather than reactively, as often happens.
While these are all interesting facets of the LADDER framework, we need to continue to evaluate its evolution, particularly how it will be able to continue to handle increasingly sophisticated attack methodologies. As attacks evolve, it will need to keep up, which will be a challenge for any automated system.
More Posts from :