7 Ways AI is Transforming Strategic Sourcing Roles in 2024 From Risk Analysis to Supplier Selection
7 Ways AI is Transforming Strategic Sourcing Roles in 2024 From Risk Analysis to Supplier Selection - AI Powered Risk Detection Flags Transportation Delays 48 Hours Earlier Than Manual Analysis
AI-driven risk analysis now spots transportation delays two days sooner than people can using old methods. This speed-up helps companies deal with problems before they grow. The move to AI, though, means watching for ethical issues, like whether the tech unfairly targets certain groups or tracks movements too closely. Despite these concerns, this tech is changing how businesses handle supply chain problems, giving them better information and hopefully an advantage. As companies try to source goods effectively in 2024, they'll need to be mindful of keeping innovation aligned with fairness and social responsibilities.
AI risk detection is showing potential, with some systems claiming to spot transport delays a full 48 hours sooner than human analysts. It seems these tools rapidly ingest data streams — weather, traffic, supply chain hiccups — something a person would struggle to do at that speed. This speed comes from their computational capacity, enabling analysis of what might be millions of data points in mere seconds, whereas a human might need days. The claim is that their predictive accuracy increases as these systems absorb more data, learning from past events to get better at spotting patterns. With the ability to forsee transport delays well in advance, the stated advantage is better logistics and inventory handling, thus avoiding stockout or overstock issues. It is said that this tech facilitates smarter decision-making, allowing for proactive management of issues instead of simply reacting. Algorithms even consider factors like political events or financial changes, offering a more comprehensive view than typical manual methods might achieve. Another advantage that is noted is better supply chain communication due to real-time access to risk assessments, fostering greater coordination amongst stakeholders. While advancements seem impressive, a healthy skepticism regarding excessive reliance on automated analysis remains. The future may see organizations using bespoke systems tailored to their specific operations, promising targeted insights, if these can be correctly configured. Such shifts are significant. Teams will need to adapt to these new data driven approaches.
7 Ways AI is Transforming Strategic Sourcing Roles in 2024 From Risk Analysis to Supplier Selection - Machine Learning Maps 10000 Global Suppliers In Real Time Through Connected Data Points
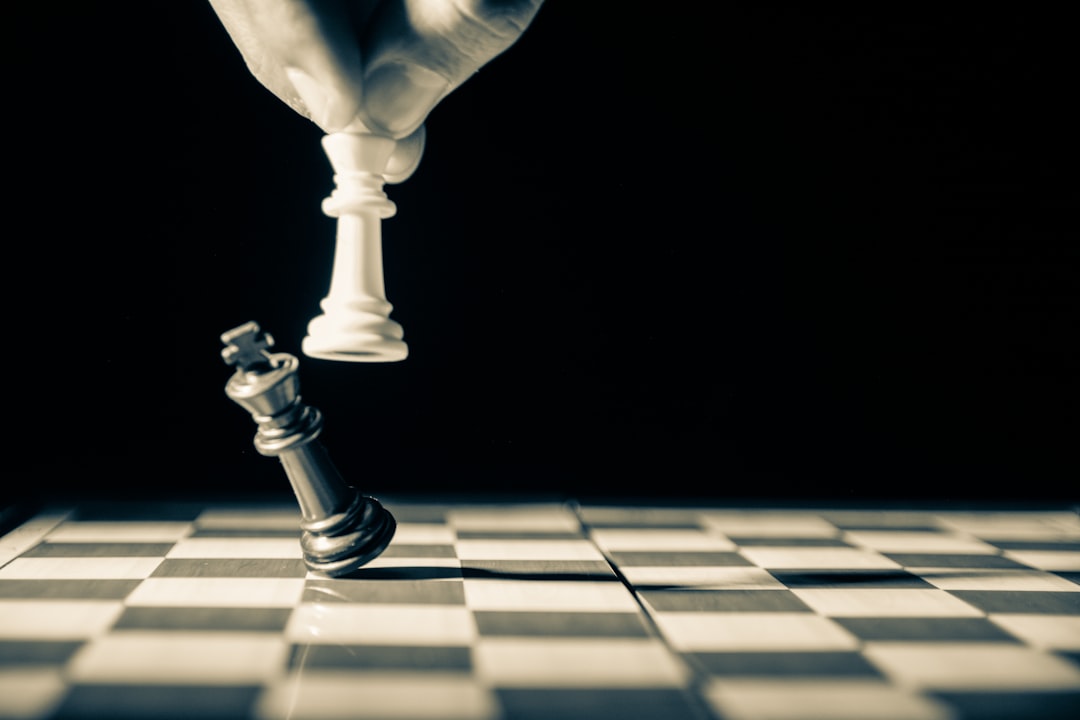
Machine learning now allows the mapping of 10,000+ suppliers around the world in real-time. This is achieved through the use of linked data, giving a dynamic picture of the global supply market. Organizations can then see, almost immediately, supplier abilities and how they are performing. This is supposed to sharpen supply analysis and make decision-making more targeted. With AI tools, potential dangers can be assessed quicker, while alternative supply routes are also highlighted. This speeds up general sourcing and strategy planning. Even more, it's now possible to find high-value suppliers fast using AI tools. Even if those suppliers are not in direct line to your immediate goods, they can be found, it is claimed. However, companies need to consider the data security and privacy concerns that come with these advances if they want to reap the real benefits in making their supply chain more robust and responsive.
Machine learning is now capable of mapping over 10,000 global suppliers using connected data, offering real-time updates on the supplier landscape, a shift from static views. This involves a form of geospatial analytics which helps to determine the most effective partner locations based on transport costs and efficiency via location tracking. Such systems examine supplier’s actions, analyzing purchase patterns to predict availability and pricing changes. Also, machine learning can simplify complex supply chains through clear visualisations that highlight relationships between various suppliers and their dependancies. These tools monitor supplier compliance through access to public datasets and, importantly, can identify public opinion by analyzing news sentiment, all as a form of qualitative analysis. It can collate supplier performance and identify leading suppliers. It is argued that this aids strategic decision making. The models are said to also identify demand patterns, assisting companies to adjust their sourcing strategies to seasonal needs. They are reported to assist risk mitigation via supply chain diversification, reducing reliance on single providers. Finally, these models can create deep supplier profiles with detailed characteristics beyond simple capabilities and pricing to include risks and behaviors. How these various characteristics are scored by an organization in its supply chain selection process requires more data.
7 Ways AI is Transforming Strategic Sourcing Roles in 2024 From Risk Analysis to Supplier Selection - Natural Language Processing Reduces Contract Review Time From 2 Weeks to 2 Days
Natural Language Processing (NLP) has greatly changed how long contract reviews take, cutting down the process from two weeks to a mere two days. By automating the extraction and interpretation of essential contract details, these NLP tools can quickly spot unclear wording, tricky clauses, and any discrepancies in legal papers. This is especially helpful when many contracts need to be examined. This change not only makes the review process faster but also makes it more precise and better, letting legal staff handle more important matters. It’s anticipated that more and more companies will use these AI tools, so NLP's role in legal processes is likely to expand, affecting how contracts are understood and processed. This is part of a larger change in how strategic sourcing is being handled, with AI making operations simpler and helping organizations make better decisions, especially in the complex world of supply chains.
Natural Language Processing (NLP) is being used to dramatically reduce the time it takes to review contracts, moving from about two weeks to just two days. This faster processing is due to advancements in how machines now handle language nuances, such as semantics and contextual understanding. It’s not just speed; these automated reviews minimize human error, which sometimes misses a good chunk of legal risks. The claim is that by training these systems on huge amounts of past contracts, businesses can become more accurate in how they comply with rules and regulations. Also, reduced time translates to saved money, cutting expenses associated with manual reviews. This frees up time for legal teams to do higher level tasks rather than being stuck in paperwork. It is also said that they can make better predictions about what is going to happen in business based on patterns they find in contract language and past events. This seems to allow for insights into potential negotiation points or any risks.
The stated ability of NLP tools goes beyond just contract review, as they claim to be able to understand the subtle differences in wording and identify vague parts that could cause later misunderstandings, which could be crucial for avoiding expensive litigation down the line. These NLP systems are often combined with other AI programs to offer wider analysis across purchasing, helping find patterns like links between supplier stability and contract terms. And if you need to tailor the system to jargon or in-house rules you are, it is said, able to do so and adapt it, ensuring that reviews stay in line with internal requirements. Because machines handle things, businesses can manage larger amounts of contracts without having to hire more staff, therefore dealing with growth and supply changes more smoothly. They provide ongoing real-time updates that will highlight clauses that are not up to date, or renewal timeframes, allowing a business to react quickly. However, we should note that the data these NLP tools use might not be completely without bias. This means it is important to watch the information that trains such programs in order to make sure that fairness is maintained throughout automated contracting.
7 Ways AI is Transforming Strategic Sourcing Roles in 2024 From Risk Analysis to Supplier Selection - Automated Spend Analytics Identifies 15% Cost Savings Through Pattern Recognition
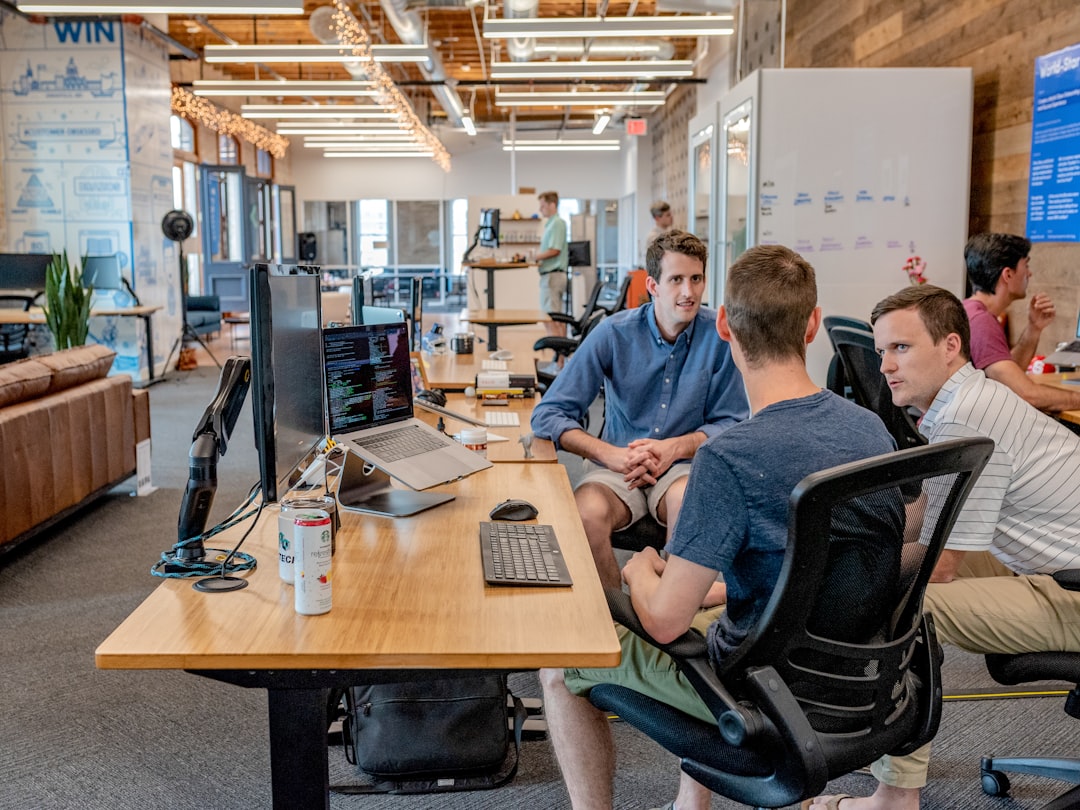
Automated spend analytics is being used to highlight possible cost reductions, reportedly around 15%, by spotting patterns in financial data. Analyzing these patterns of spending, companies can see and understand unnecessary expenses from things like frequent rush orders, which then informs their negotiating strategies. While the technology enables supposedly better data-driven analysis to inform purchase choices, and to negotiate with vendors more strongly, it’s necessary to bear in mind what could go wrong by relying too heavily on these automated systems. As methods of strategic sourcing change throughout 2024, automated analytics can improve how efficiently a company purchases and leads to potentially more overall savings if the results are properly interpreted.
Automated spend analysis tools are showing potential cost savings of up to 15% by spotting patterns in spending behavior. This suggests financial and operational improvements for organizations that use these sophisticated tools in their buying procedures. These systems employ advanced pattern recognition methods that can analyze enormous sets of supplier transactions all at once, making old-fashioned manual assessments seem slow by comparison. This shift can challenge companies that depend on human analysts for spotting opportunities to cut costs.
Spend analysis goes beyond just looking at past expenses; it also adds real-time market changes and supply chain information. This way of looking at data shows what's happening right now instead of just past trends, giving more detailed and accurate findings. Spotting cost savings through pattern recognition comes from noticing unusual spending habits and supplier relationships that might go unnoticed during manual checks. This helps businesses renegotiate deals with suppliers or bundle purchases to get better prices. It's stated that businesses using automated spend analysis have improved compliance with their own purchasing rules because the system can detect strange purchases or spending that doesn't follow their rules. This adds another layer of oversight that may help in avoiding unauthorized payments.
The analysis systems may provide benchmarks that can be used to measure supplier performance against sector averages. This makes it easier for companies to see suppliers who are not doing well and to implement changes for better results and cost-effectiveness. It also uses regression models to anticipate future spending patterns based on past activity for the purpose of proactive budget management, and so resources can be allocated more efficiently. Data quality and consistency are a noted challenge; such systems only perform as well as the data they use. If data is flawed, it can mislead and cause businesses to lose chances for savings. Spend analysis may turn up previously hidden problems, like duplicate payments or rogue spending, leading to significant cost reductions if the situations are addressed.
Even with promise, there are some complexities in using such tech. Organizations need to make sure they provide staff with proper training and methods for change, so the tools' benefits can be fully utilized.
7 Ways AI is Transforming Strategic Sourcing Roles in 2024 From Risk Analysis to Supplier Selection - Predictive AI Models Forecast Raw Material Price Changes 6 Months In Advance
Predictive AI models are changing how businesses anticipate shifts in raw material costs, offering forecasts up to six months out. Using live data and complex algorithms, these models aim for greater accuracy in today's unstable marketplaces. They look at past patterns and current market conditions, supposedly allowing companies to proactively change their buying approaches. Yet, even with the promise of smarter decisions, there's a strong need to make sure that depending on these automated systems doesn't prevent a deeper understanding of actual market conditions. As this technology develops, its broader influence on strategic sourcing will need continued watchfulness and adjustment.
AI is being used to predict changes in raw material costs, potentially up to six months ahead. These predictive tools use real-time information, and supposedly give a more detailed forecast than older methods. Such systems do not simply look at basic supply and demand, but aim to identify subtler signals by analyzing news and social media, as well as more usual data. They use statistical methods to figure out what factors – say political issues, or money market changes – have the biggest impact on prices. This allows for a better understanding of price changes compared to models that over-simply by just considering supply and demand.
Unlike generic forecasts, these AI models can give details on the price changes by material, place, and time. They are also trained on a long history of pricing data and the claim is that they can pick up long-term patterns better than any person can. Such systems also try to evolve, updating their predictions to match the constantly shifting marketplace by continually assessing new information. What if scenarios can be simulated using the data which is used to evaluate the outcomes of different procurement plans under varied conditions. The aim is to reduce forecast errors, possibly by up to 30%. Some claim this helps with better planning and management of procurement and stock. It is reported that these models can also plug into existing systems to automate procurement based on price forecasts.
These systems are reported to also analyse supplier performance which seems to assist in predicting how supply issues or unstable financial situations might link to price movements. These systems also use different data sources to feed into forecasts such as weather, government events, and logistics information. Overall these predictive AI tools move from traditional models that depend on narrow inputs towards something far more complex, or at least the claim is, more complete.
7 Ways AI is Transforming Strategic Sourcing Roles in 2024 From Risk Analysis to Supplier Selection - Smart Algorithms Match 500 New Suppliers With ESG Compliance Requirements
Smart algorithms are now matching suppliers with environmental, social, and governance (ESG) compliance needs, having recently linked 500 new suppliers with these standards. This is key, especially with the European Union's strict ESG rules. AI helps in analyzing large amounts of data and understanding different languages, so companies can better manage their worldwide supply chains. These algorithms are also becoming vital for assessing risks and choosing suppliers, offering insight into possible problems with partners. While AI is developing, integrating it into sourcing might improve compliance, but it also raises concerns about data quality and fairness.
Algorithms are now being used to sift through and match the compliance needs of companies with what various suppliers offer. These ‘smart algorithms’ have reportedly matched 500 new suppliers with specific ESG requirements. Rather than relying on manual review, which takes time, these tools assess the compliance data of many suppliers simultaneously in a faster and more automated way. This speeds up the process of supplier selection, responding more quickly to procurement needs. The idea behind these tools is that they enhance efficiency for the sourcing teams.
These algorithms uncover suppliers previously unknown to organizations, not only the obvious choices, but smaller suppliers that align with very specific ESG standards. These systems reportedly use machine learning to get better at matchmaking over time, incorporating new data as things change. This is so the data can be used dynamically, by the organization, adjusting criteria as rules and markets change. It is said these algorithms rank suppliers, assigning scores based on metrics, like past audits and even customer reviews. This enables companies to prioritize suppliers with the best compliance records quickly, focusing on the best-suited candidates.
Some of these algorithms even evaluate supplier locations, looking for efficiencies, or highlighting potential logistical risks based on regional rules or previous violations. In addition to matching suppliers, these tools seem to monitor compliance over time using automated alerts to spot changes to a supplier’s status. Ideally, companies using such tools would quickly be notified of anything that might change their purchasing decisions. Such smart algorithms are also said to work seamlessly with existing systems, which should enable better data and cooperation across the procurement departments. There is also a suggestion that automated systems can reduce the bias of human judgement, promoting fairness by choosing suppliers purely on metrics, which might not be true in reality, though they claim such a result.
The ability of these tools depends, in part, on ‘big data’ analysis, and is supposed to improve with increased access to more supplier information across numerous platforms. The systems also claim to go beyond simple compliance assessment and measure the long-term stability of the suppliers. This can predict disruptions by looking at the past data, their financial health and even trends, and to help keep a business’s supply running smoothly.
7 Ways AI is Transforming Strategic Sourcing Roles in 2024 From Risk Analysis to Supplier Selection - Computer Vision Technology Validates Supplier Quality Through Remote Factory Tours
Computer vision technology now allows for the remote validation of supplier quality through virtual factory tours. Using sophisticated visual analysis, companies can remotely assess production lines in real-time. This means capturing precise visual information for quality control and reducing possible mistakes associated with manual inspections. It also leads to faster identification of product flaws, therefore raising production standards. However, for the tech to reach its full potential, it needs to have good cooperation between the automated systems and the human experts. While the positives are clear, companies should also be mindful of keeping the data correct and private when using the tech.
Computer vision is enabling remote factory tours to assess supplier quality, moving beyond the usual in-person audits. Instead of sending people, which is both expensive and slow, computer systems examine images and video feeds from the factory floor. These systems analyse product quality using cameras and software to ‘see’ if there are any defects and identify compliance. The machines assess what is happening in real time by examining video which makes faster reactions to problems possible. This tech is now integrated with industrial control systems to collect more data and to improve efficiency. The claim is that automated systems now offer far quicker reporting and help to find patterns not only in quality, but also in how things operate on the factory floor. These machines can process data across multiple locations and provide more accurate checks than a human could do in the same amount of time, reducing variation due to subjective human judgements. However, companies will need to factor in the cost of implementing these new remote systems and the training needed to use them effectively, rather than just presuming success based on what has been promised by developers. One wonders at the depth of actual implementation and the practical challenges it introduces in day to day factory floor operations, which may need to become more standardized for a machine vision system to work most effectively. Additionally, the ‘black box’ issue remains, and one may ask if such ‘black box’ systems, with millions of parameters, might actually conceal any embedded bias that a human might easily spot? The claims are interesting, but the results on the ground, will need critical observation, perhaps from a wider variety of stakeholders. Perhaps then, we shall see if their claims hold water.
More Posts from zdnetinside.com: