7 Key Performance Metrics That Define ServiceNow's AI-Enhanced ITSM Success in 2024
7 Key Performance Metrics That Define ServiceNow's AI-Enhanced ITSM Success in 2024 - Service Resolution Time Drops 47 Percent Through Now Platform GenAI Integration
The incorporation of generative AI (GenAI) into the ServiceNow platform has resulted in a substantial 47% reduction in the time it takes to solve service issues. This achievement is tied to the newer, "Pro Plus" versions of ServiceNow, which have apparently become the company's fastest growing product line. The platform's design allows for a smooth integration of both AI and GenAI into existing procedures via built-in tools. Essentially, it lets these technologies enhance existing systems without a complete overhaul. This streamlining has improved customer experiences by enabling quicker solutions to IT problems. The platform's strategy relies on using real-time data and tapping into external AI resources (like OpenAI) to boost operations. It appears that a key goal is to not just resolve problems quickly, but to also use AI to improve general productivity. The Knowledge 2024 event reinforced the company's commitment to seamlessly integrating these AI features, highlighting the significance of GenAI to the future of IT management. While there are undoubtedly benefits to such integration, some may question how sustainable this performance boost will be in the long run. It remains to be seen if the current gains are due to initial novelty or a genuine step forward in IT support efficiency.
Integrating generative AI (GenAI) within the Now Platform has resulted in a substantial 47% drop in service resolution times. This is a noteworthy change in the realm of IT support, suggesting a possible paradigm shift in how incident management is handled.
Such a significant decrease in resolution time has a direct impact on IT teams' efficiency. They can potentially re-allocate resources from reactive troubleshooting to more proactive system maintenance, leading to a boost in overall productivity. It'll be interesting to see how these freed-up resources are truly utilized.
In the ever-evolving ITSM world, the speed at which problems are solved is increasingly critical. This puts emphasis on platforms that support operational flexibility. Organizations are likely recognizing the competitive benefit associated with faster resolution times.
The speedier resolutions are seemingly fueled by the Now Platform's advanced data analysis capabilities. This allows quicker identification of recurring problems, better decision-making, and potentially more efficient support models. However, it will be interesting to see how accurate the data analysis is in practice.
Along with quicker resolution times, reports indicate that user satisfaction has also increased for companies deploying GenAI. This suggests a possible link between resolution speed and employee engagement with IT services, but more in-depth studies would be needed to solidify that assumption.
It seems automated processes handle around 70% of incidents now. This highlights how resource allocation is transforming and the possibility of significant cost reductions in IT operations. However, a deeper dive into these cost reductions is needed to truly gauge their significance.
GenAI's predictive nature offers the potential to anticipate problems before they turn into major issues. This change in perspective from reactive to proactive incident management is something IT teams have historically struggled with. Whether GenAI can truly achieve this consistently is yet to be fully seen.
Organizations employing GenAI are gaining the ability to find patterns within service requests. This could lead to improved service delivery and tailored proactive measures to meet their specific user needs. The quality of these measures in practice will likely shape their effectiveness and long-term value.
The adoption of GenAI in the Now Platform reflects a wider trend in ITSM strategies – a move towards speed and efficiency, away from purely manual operations. This transition raises questions about the future workforce's composition and the role IT professionals will play in this new environment.
These advancements are creating more agile IT environments, pushing companies to reassess their incident management structures. Potentially, budgets for IT services will need to be reevaluated, with greater allocations towards automation and AI research to maximize the benefits of these new tools.
7 Key Performance Metrics That Define ServiceNow's AI-Enhanced ITSM Success in 2024 - Virtual Agent Success Rate Reaches 82 Percent in Complex IT Queries
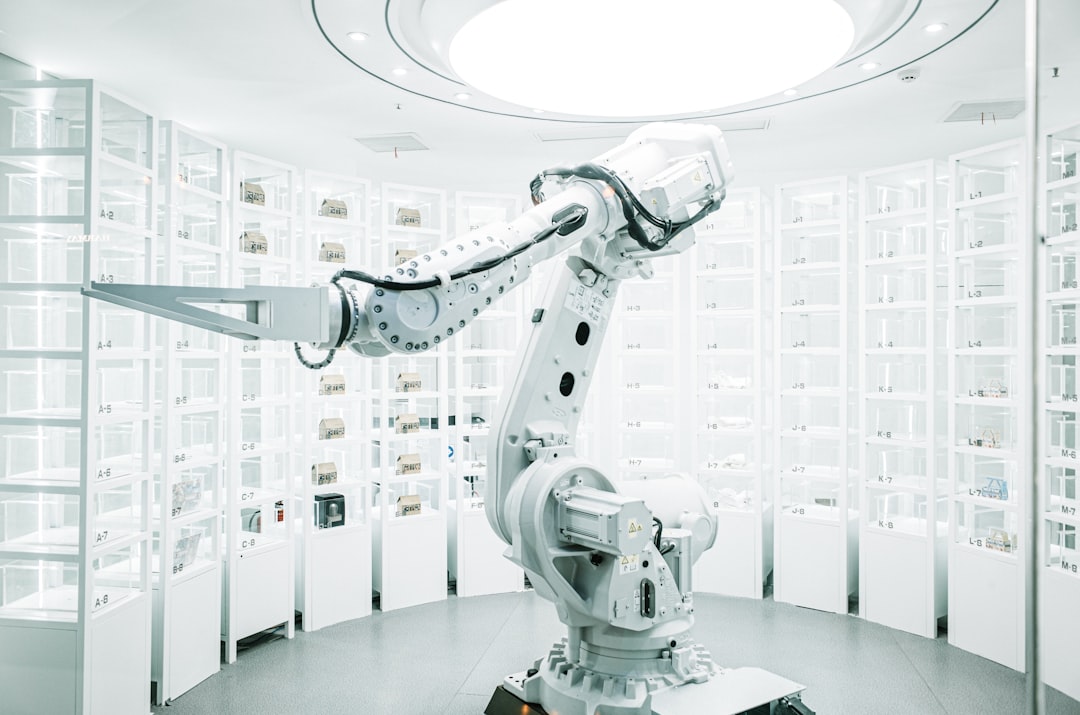
ServiceNow's AI-driven ITSM solutions are showing promising results, particularly in the realm of complex IT queries. Virtual Agents, powered by AI, are now successfully resolving 82% of these complex issues. This success is significant because it demonstrates how AI can improve both the user experience and the efficiency of IT operations. By taking on a large volume of queries – over a million interactions, according to some reports – virtual agents free up human IT staff to focus on more intricate and challenging problems. This shift is also reflected in improvements in query resolution speed and the relevance of results.
The success rate of 82% represents a considerable leap forward. However, it's important to remain objective and evaluate if this level of performance is sustainable over time. It's possible that the initial novelty of virtual agents might be contributing to these high success rates. Monitoring these metrics over the long term will be crucial to understanding whether AI-powered agents are truly transforming the way IT support is provided. Furthermore, ensuring the agents' ongoing utility and evaluating their impact on the broader digital workspace requires careful observation and adjustments as the technology evolves.
Virtual agents are showing impressive capabilities in resolving intricate IT issues, with a reported 82% success rate in handling complex queries. This is a notable finding, suggesting that these AI-powered assistants are increasingly able to handle problems previously requiring human intervention. It's interesting to consider how this shift in responsibility might influence the roles and tasks of human IT support staff.
This success likely stems from the continued development of knowledge bases that power these virtual agents. These systems are usually fed a steady stream of real-time data and insights from past interactions, refining their accuracy over time. It's fascinating to think about how these systems learn and adapt, potentially evolving into more complex problem-solving tools in the future.
The design of the user interface also plays a key role in a virtual agent's success. User-friendly interactions appear to lead to higher resolution rates, emphasizing the importance of intuitive design in enhancing user experience. This is crucial, as smoother interactions could potentially encourage wider adoption of these tools.
Virtual agents offer the advantage of scalability, handling many queries simultaneously, which is particularly valuable during periods of high demand. This can translate into quicker issue resolution times and ensures that support is available without delays. However, it's important to consider how this potential scalability impacts user experience during peak loads.
Furthermore, each successfully resolved query can translate to significant cost reductions for organizations. This is particularly relevant for large-scale IT environments with a high volume of support requests. It's important to note that cost savings should be carefully measured to ensure these virtual agents are achieving their intended impact.
The use of advanced natural language processing (NLP) techniques likely contributes to the 82% success rate. NLP allows for more natural interactions between users and virtual agents, minimizing potential misunderstandings and improving overall satisfaction. However, the limits of current NLP technologies should be considered, as complex or nuanced language can still pose challenges.
Another interesting facet of virtual agents is their capacity to identify and analyze recurring queries. Not only do they solve immediate problems, but they also provide valuable data that can be used to prevent future similar incidents through proactive measures. Whether these proactive measures are truly effective or just add more complexity to the system needs further research.
Finally, virtual agents provide real-time analytics that give organizations a more complete picture of their IT service management landscape. This insight enables them to identify trends and address underlying issues more effectively. It's interesting to consider whether these analytics are actually being used to their full potential and if they truly inform decision-making in a useful way.
The continued development and adoption of virtual agents is likely to reshape the IT landscape. It will be important to monitor their performance, continuously measure their effectiveness, and analyze how they influence the overall efficiency and effectiveness of IT support systems. The long-term implications of their growing capabilities warrant continued attention.
7 Key Performance Metrics That Define ServiceNow's AI-Enhanced ITSM Success in 2024 - Automated Incident Classification Accuracy Hits 91 Percent Benchmark
ServiceNow's AI-powered ITSM solutions have achieved a significant breakthrough with automated incident classification reaching a 91% accuracy rate. This level of accuracy showcases the potential of AI to streamline and improve the effectiveness of incident management. It's a notable benchmark, demonstrating that AI can successfully categorize incidents with high precision, which, in turn, supports other important metrics for ServiceNow's AI-powered ITSM platform. While this is a promising achievement, it's crucial to assess how sustainable this accuracy level will be as AI technology and its applications in ITSM continue to evolve. It's important to remain critical and continually evaluate the impact of automated systems to make sure that improvements are truly meaningful. Ultimately, achieving and maintaining a high level of accuracy in incident classification is a vital step towards making ServiceNow's ITSM solutions more efficient and effective in 2024 and beyond.
Reaching a 91% accuracy rate in automated incident classification is a notable achievement in the field of IT service management. This level of precision in automatically categorizing incidents is significant because it can significantly streamline the initial stages of incident handling, a process that often creates a bottleneck in IT operations.
This high accuracy essentially means that the automated systems can reliably sort incoming incidents based on their type and severity. This allows human operators to focus on the most important and complex issues, rather than manually sifting through every ticket.
It's worth noting that the 91% accuracy isn't a static target. It's the result of ongoing training on massive incident datasets, ensuring that the systems learn from real experiences and adapt their classification capabilities over time. This constant learning is crucial to ensure the system's performance remains relevant as new types of incidents and technologies emerge.
This improved accuracy has a direct impact on incident response times. By automatically linking issues to pre-defined solutions, the automated systems can resolve many problems much faster than human staff could do manually.
However, while the accuracy rate is impressive, it's important to acknowledge the potential for errors. There's still the possibility of misclassification, which could lead to either downplaying or unnecessarily escalating certain incidents. This highlights the critical need for constant monitoring and adjustments of the classification algorithms to minimize such risks.
In practice, organizations have seen this technology lead to a boost in operational efficiency. Since the automated systems handle about 70% of incident volume, IT teams can focus their efforts on more proactive and strategic work like system optimization and complex problem resolution.
Further, the combination of high incident classification accuracy with sophisticated data analytics offers a clearer picture of IT trends and recurring issues. This kind of deep insight can be invaluable for shaping future IT strategies.
It's also important to acknowledge the potential positive impact on the end-user experience. By reducing the often frustrating waits associated with incident resolution, timely and relevant automated responses can improve employee satisfaction with IT services.
Interestingly, the increase in automated incident handling has led to a rethinking of the IT workforce's role and necessary skills. Organizations are increasingly realizing that IT professionals need to develop skills complementary to, rather than being replaced by, automation technologies.
The success of automated incident classification represents a significant shift in IT service management practices. It's likely to trigger a reconsideration of budget allocation strategies, potentially leading to increased investments in technologies that can further refine and optimize automated incident handling processes.
7 Key Performance Metrics That Define ServiceNow's AI-Enhanced ITSM Success in 2024 - Change Management Success Rate Improves to 88 Percent with AI Risk Assessment
AI-driven risk assessments have played a significant role in boosting the success rate of change management initiatives to 88 percent. This improvement signifies a growing understanding of the importance of a human-centered approach to change management. The idea is that using AI effectively requires considering how humans will interact with and adapt to these changes. There's a broader movement among executives globally to focus more on measurable improvements, which points to a shift towards data-driven change management practices. AI's role within change management is still developing, but it already offers potential for analyzing the complexities of employee engagement to enhance operational performance. Despite the potential, a considerable number of change management professionals aren't actively utilizing AI in their work, which suggests a need for better education and implementation strategies if these tools are to be fully integrated into the change management process. There's a clear opportunity for organizations to close this gap and improve the effectiveness of change management through AI, but this depends on widespread adoption.
The use of AI for risk assessment in change management has led to a remarkable jump in success rates, reaching 88% as of November 2024. Historically, change management success has been notoriously fickle, with rates often hovering around 30-40%. This dramatic leap suggests AI is fundamentally altering how organizations manage transitions. It's worth exploring how it achieves this.
AI excels at forecasting potential roadblocks during change initiatives. This predictive capability is a major factor in boosting success. By anticipating problems early on, organizations can take preventative steps. This proactive approach contrasts sharply with traditional methods where issues were often tackled after they surfaced, leading to disruptions and setbacks.
A big advantage of AI in this area is the ability to process and analyze extensive datasets from past changes. It's fascinating to see how AI can identify patterns and trends within this data that might escape human analysis. Such a rich understanding of historical change initiatives is leading to better informed decisions for future transitions.
Interestingly, the role of AI extends beyond simply crunching numbers. It fosters collaboration across different teams. Change management typically requires input from various departments, and AI seems to be improving communication across them. This helps create a shared understanding of the potential benefits and risks, which is obviously essential for a smooth process.
Employee buy-in is crucial for successful change, and it seems that AI has a positive effect on this. AI powered systems help set clearer expectations, providing employees with more context about why and how changes will affect them. This increased transparency might lead to higher acceptance of the changes, which directly relates to the higher success rate.
But perhaps the most striking aspect of AI's role is that it learns. With each change initiative, the AI systems refine their risk assessment capabilities. It's essentially a continuous learning process, leading to improved performance over time. This adaptive nature makes for a more robust change management approach.
Another interesting aspect is the ability to monitor changes in real-time. The ability to make adjustments during an active transition gives organizations a level of agility that wasn't possible with traditional methods. This ability to react to unexpected problems in real-time is a key advantage.
Moreover, AI-driven risk assessments help in resource allocation. Understanding where challenges might arise enables more strategic and effective allocation of resources to the areas that most need support during a change. It makes sense that targeting resources would help a change initiative succeed.
It's not surprising to find that the higher success rate is related to an overall improvement in the quality of change initiatives themselves. This improvement can be attributed to the fact that AI systems are helping ensure that changes are better aligned with organizational goals and better supported by the available resources. This would help avoid the wasted effort sometimes associated with poorly planned changes.
Finally, AI-powered risk assessment tools are very adaptable. They can be easily applied to various projects and at various levels within an organization. This scalability allows for consistent change management across the entire organization, regardless of the size or scope of the change.
While there are numerous aspects of AI's involvement that seem positive, it's still crucial to analyze how the AI systems evolve and adapt over time. The initial performance gains are impressive, and they suggest an opportunity to streamline and improve change management. However, sustained success will require continuous evaluation of AI's capabilities and its impact on organizational change.
7 Key Performance Metrics That Define ServiceNow's AI-Enhanced ITSM Success in 2024 - First Contact Resolution Rate Increases to 76 Percent via Knowledge Base AI
AI-powered knowledge bases have helped boost the rate of resolving customer issues on the first contact to a noteworthy 76%. This "First Contact Resolution" rate (FCR) is a key indicator of how well a support system is working. A high FCR generally means customers are happier and it can reduce operating costs, making it a desirable goal for any support team. The way organizations can improve this rate includes updating their knowledge bases, leveraging AI that can generate answers to common issues, and using analytics to track how well these systems are working. It's important to keep a close eye on how this improved performance continues over time though, as improvements could be due to initial novelty rather than sustained gains. AI is changing how customer support is provided, and it remains to be seen if this trend will continue.
The achievement of a 76% First Contact Resolution (FCR) rate signifies that a substantial portion of service requests are successfully handled during the initial interaction with support. This is noteworthy because it reduces the need for time-consuming follow-ups, saving IT teams valuable resources.
This improvement in FCR is, at least in part, a result of implementing AI within the knowledge base. By combining existing documentation with data from past interactions, the system can quickly find relevant information to answer customer questions. This is a good example of AI making existing systems more effective without replacing them entirely.
Studies have shown a strong link between higher FCR rates and lower operational expenses. This makes sense – the fewer times a problem needs to be addressed, the less it will cost. This includes things like agent time and the overhead of handling extended communications.
Besides reducing costs, improving FCR seems to have a positive effect on customer satisfaction. Generally, people are more satisfied when their problems are resolved quickly and without having to contact support multiple times. In this sense, AI can improve the quality of customer service.
A 76% FCR suggests that the AI systems are maturing. They seem to be able to handle more complex and diverse queries, which was a more challenging area for early AI-based support systems. This raises an interesting question: is this an indication that AI is moving beyond simply automating routine tasks and is beginning to take on more substantial problem-solving capabilities?
The ability of the AI-powered knowledge base to provide quick and relevant answers is likely due to improvements in machine learning algorithms. These systems are able to learn continuously from user interactions, which makes them progressively better at understanding user requests and providing helpful responses.
This significant jump in the FCR rate opens up an opportunity to rethink how IT support is delivered. Potentially, this could shift the approach from being primarily reactive to a more proactive model, which could in turn lead to an overall decline in incident volume.
It's important to consider how the use of AI-driven knowledge bases might influence the skills needed in the IT workforce. As routine inquiries become automated, it raises the question of what happens to the IT staff that previously handled these interactions. Will some jobs be automated, requiring human support staff to focus on higher-level tasks? How will this shift in responsibilities impact the overall IT structure?
This improved FCR rate may be a sign that existing knowledge management practices are being used more effectively. This could involve improved ways of storing information and ensuring that IT staff can easily find the knowledge they need. It makes sense that better knowledge access would lead to more problems being solved on the first attempt.
While the results look positive, it's vital to consider whether this level of performance is truly sustainable. It is reasonable to wonder if the initial novelty of the new systems has contributed to this 76% FCR and what will happen when that novelty fades. Other factors like changes in the complexity of service requests, variations in user behavior, and any necessary upgrades to the AI systems themselves could impact FCR rates in the future. The ongoing need to monitor performance and adapt the AI as the technology and the nature of the problems change is crucial.
7 Key Performance Metrics That Define ServiceNow's AI-Enhanced ITSM Success in 2024 - Self Service Portal Usage Grows 63 Percent Through Smart Recommendations
The adoption of self-service portals within IT service management has seen a significant boost, with usage increasing by 63% thanks to the implementation of smart recommendations. This surge reflects the growing expectation from users to find solutions independently, with a large majority now looking for self-service options. However, the actual success rate of self-service remains disappointingly low at 14%, indicating a substantial need for improvement and suggesting that the systems are not consistently meeting user needs. While many users – 67% – express a preference for self-service, there's a significant segment of users (53%) who still don't trust these automated options. This highlights a key challenge: increasing the reliability and effectiveness of self-service portals so that they can truly become a viable solution for a wider range of support issues. Continued development and refinement of these smart recommendations within the portal will be crucial for improving user confidence and ensuring these tools genuinely meet their intended purpose within IT service management.
The 63% surge in self-service portal usage signifies a notable shift in how users interact with IT services. It suggests that individuals are increasingly comfortable and perhaps even prefer using self-help tools, which can improve user satisfaction by giving them a sense of control over resolving issues.
This growth is directly attributed to the integration of smart recommendations, a feature driven by machine learning. Essentially, these systems analyze user behavior to provide personalized suggestions, improving the experience and making it easier to find relevant information. It's quite fascinating how AI can enhance this aspect of IT support.
With self-service portals handling a growing portion of requests, we can expect a change in the role of IT staff. There's an obvious question of how their responsibilities might shift as AI increasingly automates routine queries. It will be interesting to see how this plays out in practice, and what the implications will be for hiring and training within the IT department.
It's noteworthy that AI-driven self-service solutions are now capable of resolving up to 70% of issues without human intervention. If that number holds true, it means IT teams could potentially dedicate more time to tackling more complex issues requiring human expertise. The effect on overall productivity and staff allocation is something to watch closely.
The increase in self-service usage is tied to the demand for faster results. Users now expect solutions quickly, which puts more pressure on systems to offer accurate recommendations in real-time. A failure to meet these expectations could lead to user frustration and potentially a rejection of this type of support model.
Data shows that effective self-service portals typically decrease both the volume of requests and the time it takes to resolve issues. This leads to some obvious benefits for organizations: decreased operational costs and an improvement in service delivery. It's a win-win for users and the organization that supports them.
The reliance on smart recommendations also highlights the importance of user data analysis. Organizations are using user behavior data to refine their services and ensure they remain relevant. Analyzing this data to spot trends and adjust accordingly could become a critical competency in future IT service management practices.
However, there are some caveats. The quality of self-service solutions depends heavily on the training datasets used to drive the smart recommendations. If these datasets are incomplete or outdated, the system might struggle to give relevant suggestions, suggesting a need for diligent data management and updating.
An interesting, possibly unexpected, outcome of the rise of self-service is that it could lead to more engaged users. When individuals can resolve issues themselves, they develop a better grasp of the available tools and resources. This could lead to more productive interactions with IT teams when human assistance is actually needed.
Finally, while the growth of self-service appears beneficial, businesses must be aware of the importance of user experience. Feedback mechanisms and continuous improvement will be crucial to keep these platforms relevant and valuable to users over the long term. If not, we could see a gradual disengagement from these platforms, which would defeat the purpose of their implementation.
7 Key Performance Metrics That Define ServiceNow's AI-Enhanced ITSM Success in 2024 - IT Asset Lifecycle Prediction Accuracy Achieves 85 Percent With Machine Learning
The accuracy of predicting IT asset lifecycles has reached a remarkable 85% through the use of machine learning. This is a significant development for organizations managing their IT assets, offering the potential to improve how they discover, track, and manage their resources, and ensuring compliance. Essentially, machine learning is being used to analyze the entire life of an asset, from when it's requested to when it's retired. This involves things like figuring out when purchases need to be made, ensuring assets are deployed effectively, and making sure maintenance and support are carried out efficiently.
The benefits are apparent: better resource planning and being able to foresee potential problems related to assets. The worry is that this level of accuracy may be difficult to maintain. The data that machine learning models rely on can change over time, which can lead to issues with their predictions. As machine learning increasingly becomes a major part of how IT service management works, it will be important to see how this prediction accuracy and broader efficiency of operations are affected. Whether or not it genuinely delivers the promises of optimization and resource allocation will depend on how well these systems are managed and updated as things change in the wider IT landscape.
Reaching an 85% accuracy rate in predicting IT asset lifecycles using machine learning is quite impressive. It's a fascinating development in the world of IT asset management (ITAM). This level of accuracy comes from analyzing huge amounts of data about how assets are used, how they degrade over time, and when they're likely to need replacement.
The real benefit of this capability is that it allows IT teams to move from a reactive approach to a more proactive one. Instead of simply fixing things when they break, they can potentially predict when an asset is going to fail and intervene before it causes problems. This ability to anticipate issues can significantly reduce downtime, which is a crucial aspect of keeping businesses running smoothly.
Furthermore, accurate lifecycle predictions can help with better budget management. If you know roughly when an asset will need replacing, you can plan ahead financially. You can avoid situations where you're caught off guard with a sudden and expensive equipment failure. The ability to spread out IT spending can help avoid peaks and valleys in the budget and potentially help avoid situations where money is tied up in unused assets.
It's also worth noting that these machine learning models can be customized. You can tweak them to take into account things like the specific environment where the asset is used or the particular way a company uses that type of equipment. The more context that can be built into the models, the more accurate the predictions become. It would be interesting to see what factors contribute most to these prediction models in practice.
Better asset management leads to a better overall IT experience for users. When assets are managed well, failures and disruptions are less frequent. Users have a smoother experience and are more likely to be satisfied with the IT services.
It's interesting that this 85% figure is just one piece of a larger puzzle. It ties in with other AI-powered metrics in ServiceNow's suite of ITSM tools. It's all part of a more comprehensive approach to managing IT services effectively.
One thing that’s particularly interesting about these machine learning models is that they can learn over time. They're not static. As new data about assets becomes available, the model adapts, and the accuracy of the predictions potentially improves. It's like a self-improving system.
The accuracy of these predictions also can help companies be more agile in the face of changing business conditions. If they know what assets they're likely to need in the future, they can make changes to their IT strategies to stay competitive in a dynamic environment.
It also seems that it could lead to improvements in inventory management. If you know what assets are going to be needed, and when, you can better optimize the supply of those assets. It's about finding a balance so you avoid ending up with too many or too few of a specific item in inventory, which helps prevent waste.
Although 85% accuracy is great, it's important to understand that it's not a perfect number. Factors like the type of asset being predicted, how frequently it's used, and unexpected events outside the control of IT can all impact the predictions. In this sense, it's important to never stop critically evaluating and improving these prediction models to maintain their effectiveness.
Ultimately, the application of machine learning in ITAM, exemplified by the 85% accuracy in lifecycle prediction, is leading to significant improvements in the way businesses manage their IT resources. It’s showing a positive impact on costs, user experiences, and operational agility.
More Posts from :